Prostate cancer radiomics and the promise of radiogenomics
Introduction
Prostate cancer is the most prevalent male malignancy in the US, with more than 1 in 6 men expected to be diagnosed with the disease in their lifetime. Treatment recommendations are currently based on risk stratification using PSA, Gleason score and T-category, which categorize men as low, intermediate, and high risk (1). Typically, men with low risk are offered active surveillance rather than immediate definitive treatment and most of these men do well in long term (2,3). However, some of these men may harbor more aggressive disease that remains undetected. Recently, an analysis of 17,943 patients with low-risk prostate cancer (i.e., candidates for active surveillance) treated with radical prostatectomy (RP) in the US between 2010–2011 showed that upgrading, upstaging, or nodal metastases occurred in 45% of men and that the deferral of radical prostatectomy for more than 12 months was associated with an 1.7-fold increased risk of non-organ confined disease after surgery (4). Additionally, in men with low risk prostate cancer, the deferral of radical prostatectomy is associated with significantly worse radical prostatectomy outcomes, including more pathology upgrading and a higher rate of biochemical progression (5). Therefore, additional tools beyond clinical staging are needed to improve risk stratification and optimize effective use of active surveillance (3). Similarly, patients at risk for developing metastatic cancer would benefit from intensified therapy at an earlier time point. For the radiotherapy (RT) patient, RT technique and the use and length of concurrent/adjuvant androgen deprivation therapy (ADT) would be optimized. Up to 50% of these men demonstrate biochemical failure suggesting that additional strategies for defining and treating patients based on improved risk stratification are required (6,7).
Genomic analyses have significantly increased our understanding of prostate cancer heterogeneity and greatly improved patient risk classification thus directly impacting treatment decision-making [Decipher (8), Myriad (9,10), Genomic Health Institute (GHI)]. Gene expression signatures have demonstrated effective personalization of therapy by genetic risk stratification of the patient, these coupled with clinical risk factors can be useful in predicting biochemical failure and early metastasis post radical treatment (8,11,12). These approaches help select men that may benefit most from adjuvant RT after radical prostatectomy (13).
Prostate cancer, however, exhibits spatial heterogeneity that can confound current pre-treatment clinical-pathological and genomic assessment. The use of multiparametric MRI (mpMRI) is rapidly gaining momentum in the management of prostate cancer because of its improved diagnostic potential and its ability to combine functional and anatomical information. mpMRI is widely used to measure perfusion via dynamic contrast enhanced (DCE)-MRI, diffusion via diffusion weighted imaging (DWI) and anatomical information [T2-weighted (T2w) MRI]. There is no other imaging modality that has shown the same sensitivity and specificity for distinguishing intraprostatic cancer of higher grade (GS 7 or above) when combined with mpMRI guided prostate biopsies (14,15). The automated analysis and interpretation of mpMRI, however, is quite challenging as each exam results in thousands of images and there is lack of consensus of how to optimally extract the relevant information.
“Radiomics”, as it refers to the extraction and analysis of large number of advanced quantitative imaging features from medical images using high throughput methods (16,17) is perfectly suited to extract and provide an engine for effective sifting through the multiple series of prostate mpMRI and quantify the regions of interest. The central hypothesis of the radiomic approach is that the imaging features capture distinct phenotypic differences of tumors and may have prognostic power (18). Radiomics offers important advantages for assessment of tumor heterogeneity and the approach has the potential to enable quantitative measurements for intra- and intertumoral heterogeneity. Consequently, the concept of using imaging data to identify sub-regions, or ‘habitats’, within tumor lesions has been described (19). MR-based habitat imaging is accomplished by combining images acquired with different pulse sequences. Radiomics of these habitats results in distinct combinations of quantitative parameters that reveal regions with different physiologies and phenotypes.
Radiomics is a quite young discipline, but there are series of efforts in the community for addressing the clinical issues about (I) detection/segmentation of the suspicious lesion; and (II) assessment of the aggressiveness of prostate cancer. The goal of the latter is in particular to identify patients who can be spared biopsies and/or patients at high risk for metastatic disease; and the aim of the former is to diagnose cancerous vs. non-cancerous tissue and thus provide targets for biopsies or radiation boost. While there are few examples of radiogenomics, a discipline connecting radiomic and genomic data, in glioblastoma (GBM) (20-22), renal cell carcinoma (23), hepatocellular carcinoma (24), lung and head and neck cancers (18), our group at University of Miami is taking the lead to use the concepts of radiogenomics in prostate cancer.
The article is structured as follows: first, we introduce the radiomics pipeline, tailored for analysis of prostate mpMRI; in the process we describe our particular implementations of radiomics modules. Second, we summarize the efforts in the radiomics field related to prostate cancer diagnosis and assessment of aggressiveness. Finally, we describe our results from radiogenomic analysis, based on MRI-targeted biopsies and the potential of future applications of the technique.
Radiomics pipeline
The steps of the radiomics process for analysis of prostate mpMRI are shown in Figure 1.
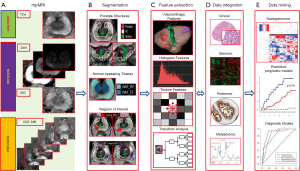
mpMRI exam of the prostate
mpMRI exam of the prostate typically includes acquisition of T2w, DWI and DCE-MRI data. An Apparent diffusion coefficient (ADC) map is calculated on the MRI scanner’s console. The acquired images are transferred to an image processing station. There is a variety of medical image computing platforms, both commercial [MIM (MIM, Cleveland, Ohio, USA) and Mirada (Mirada Medical USA, Denver, CO, USA) and open source [3D SLICER, http://slicer.org and electronic Physician Annotation Device (ePAD)] (25). In our study, we used MIM imaging workstation to implement custom routines in the platform.
Volume selection and segmentation
Selection of the volumes for the analysis impacts the entire downstream process. Prostate is a unique organ with distinct zonal morphology. As a consequence, the peripheral (PZ) and transition (TZ) zones have different imaging characteristics. In Figure 2, the T2w and ADC values in PZ and TZ were analyzed and the distribution of values are different for the two prostate regions. Thus PZ and TZ should be considered separately in identification of the prostate habitats. The peri-urethral area is characterized by high vascularity, which can generate false positives on DCE. In our implementation, the urethra is contoured and excluded from subsequent analysis. Tumor regions of interest (ROIs) are either automatically delineated or manually drawn based on radiologists’ assessment or pathology evaluation. Regions of normal appearing tissues (NAT) in PZ and TZ are selected outside of the ROIs.
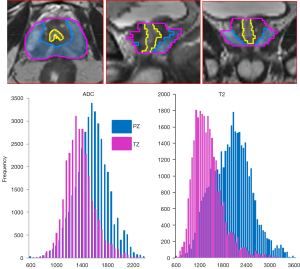
Segmenting the volumes of interest is of critical importance as the subsequent radiomics features are generated from the segmented volumes. In our radiomics pipeline in MIM, we manually contour the prostate, PZ and urethra. TZ is determined algebraically by subtracting the PZ from the prostate volume. Efforts are underway for automation of this process by utilizing a prostate atlas. In a collaborative efforts with MIM, we developed an automated atlas-based segmentation method for generating prostate and PZ contours (26).
Tumor (ROI) delineation is challenging both for automatic or manual methods because tumors may have indistinct borders. In our implementation we utilize the habitats concept to identify the suspicious lesions map the tumor heterogeneity. The approach is based on combination of co-registered images from multiple modalities, with each one contributing a piece of orthogonal information. In mpMRI, DCE-MRI identifies regional distributions of blood flow, and lack of blood flow. On the other hand, ADC, measured via diffusion MRI, is a powerful method to interpolate the density of diffusion barriers (i.e., cells) and hence provides information that may be biologically, but not physically, related to DCE-MRI.
In our implementation, we combine DCE-MRI and ADC to construct habitat maps which represent high, medium and low risk for cancer. First, an unsupervised pattern recognition approach is used to decompose DCE-MRI data as a product of several temporal patterns and their relative contribution (27). The ‘tumor’ pattern, associated with rapid washin and gradual washout of the contrast is identified. Let A be the map of distribution of this pattern: thresholds for areas of high, mid and low risk for aggressive tumor are estimated as [mean(A) + k*stdev(A)], where k=2, 1.5 and 1. Similarly, the ADC map is thresholded at 800, 1,000 and 1,200 µm2/s based on literature and empirical observations in our group (28-33). Finally, the areas of intersections between the corresponding maps from DCE-MRI and ADC are considered the volumes of high, mid and low probability for high risk cancer.
Radiomic features
Extraction of the radiomics features is the engine of the radiomics process. The broad categories of radiomics features as applied to prostate are summarized in Table 1. These features can be generally divided in 4 categories: the first category (C1) summarizes features descriptive of the volume size, shape, etc.; the second (C2), third (C3) and fourth (C4) category can be described as first-, second- and higher order statistical outputs. First-order statistic features are related to the intensity histogram of a given volume: mean, median, standard deviation, minimum, maximum, quartiles, kurtosis, skewness, etc. The second-order statistics are related to texture analysis features, also known as Haralick texture descriptors (34). On the grey level co-occurrence matrix (GLCM), various statistics can be computed: energy, entropy, correlation, homogeneity, contrast, etc. GLCM captures the frequency of co-occurrence of similar intensity levels over the region, which describes the texture of the region of interest. Another technique in this category is fractal-based texture analysis (35) which examines the difference between pixels at different length scales (offset differences). And lastly, the higher-order methods extract repetitive or non-repetitive patterns using kernel functional transformation. Some popularly used texture descriptors are Wavelets, Laplace, Fourier transforms; Gabor filters, Minkowski functionals, etc.
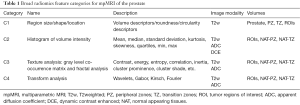
Full table
We have implemented texture features extraction using a MIM Java plugin that interfaces to an in-house software developed in C++ using Insight Segmentation and Registration Toolkit (ITK) (36,37). The ITK is an open-source, cross-platform system developed in C++ that provides tools for the development of image analysis. In our implementation, the maps of the calculated texture features are returned MIM, co-registered with mpMRI and characterized within the volumes if interest.
Data integration
Integrating imaging with clinical and molecular data in contemporary clinical databases is crucial for clinical decision making. The challenge is that the information in the images is neither explicit nor in standard computer-accessible formats. We partnered with MIM to develop a systematic procedure for semi-automated processing of mpMRI into an explicit, standard computer accessible format. The goal is to integrate the quantitative imaging features in an existing clinical RedCap database. We are refining quantitative imaging methods that enhance tumor characterization and biopsy positioning through the application of spatially explicit quantitative image analysis that recognizes tumor heterogeneity and defines regionally distinct habitats (18).
Data mining
Radiomics data are in a format that is amicable for building descriptive and predictive models relating image features to outcome, as well as gene-protein signatures. Resultant models may include imaging, molecular, and clinical data, and provide valuable diagnostic, prognostic or predictive information. The process of conversion of digital medical images into mineable high-dimensional data is motivated by the concept that biomedical images contain information that reflects underlying pathophysiology and that these relationships can be revealed via quantitative image analyses.
Review of applications of radiomics to prostate cancer
The published manuscripts, related to radiomics of prostate mpMRI are summarized chronologically in Table 2. The data is grouped based on selected volumes for analysis, type of segmentation (manual vs. automatic), mpMRI modality, types of extracted features, and overall goal of the analysis. Below we discuss the papers, grouped based on the analysis goal: prostate cancer diagnosis, assessment for aggressiveness or both.
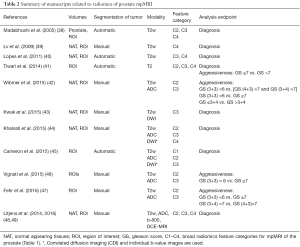
Full table
Prostate cancer diagnosis
Algorithms for automatic identification of the prostate cancer usually compute series of features and develop a supervised classifier based on ‘ground truth’ volumes. The classifier is first trained on subsets of the data and then applied to the rest.
Madabhushi et al. (38) presented a method for detecting prostate cancer from high resolution MRI of prostatectomy samples. Besides first and second-order statistical methods, the authors used Gabor filters, Gradient based features and discrete cosine transform to achieve high specificity (98%), but low sensitivity (36–42%).
These ideas were further developed in subsequent work by Litjens et al. (48,49) where a new classifier was introduced to separate prostate cancer and benign confounders on MRI. The pathology annotations were propagated to MR images by registration of whole-mount slides and manual annotation of cancer, benign prostatic hyperplasia (BPH), prostatic intraepithelial neoplasia (PIN), inflammation. Signal intensities and texture features (Gauss, Gabor and Hess filters) were extracted from T2w, ADC, b-value =800 sec/mm2 and series of DCE maps. The authors conclude that the best features for discrimination depend on the benign disease and cancer grade.
Texture features, based on local binary patterns (50) are used in the computer-aided diagnosis system (CAD) in Kwak et al. (43) based on T2w and high b-value (=2,000 sec/mm2) in DWI. MRI-US fusion targeted biopsies results are used as “ground truth”. A three-stage feature selection method was used to determine the most discriminative features. The Area under ROC curve (AUC) in distinguishing cancer from MR-positive benign lesions was 0.83. The performance of the CAD was independent from the prostate zone.
Khalvati et al. (44) presented a study using computed high b-value DWI (CHB-DWI) and new diffusion imaging modality, called correlated diffusion imaging (CDI) in addition to T2w and DWI in a cohort of 20 patients. In this study, four first-order features (mean, standard deviation, skewness and kurtosis), 72 second-order features (from texture analysis), 8 features from Kirsch filters and 12 from Gabor filters were computed in a 3×3 pixels sliding window. The voxels were tagged as cancerous or non-cancerous and 96 features were computed. A feature selection analysis for each individual modality was used to select the features which gave the best tissue type discrimination. The results show that in all the cases, adding CDI, CHB-DWI and the b-values images increased significantly the sensitivity and specificity.
The aim of the Cameron et al. (45) paper was to provide a computer-aided detection of the prostate cancer lesion using a large number of quantitative imaging features. High-level feature categories, including Morphology, Asymmetry, Physiology and Size (MAPS) were combined. Using a cohort of 13 patients, the initial identification of candidate tumors was automatically performed using ADC first: all voxels with and ADC value less than 700 µm2/s were grouped into connected volumes. Forty-two imaging features were used to train a naive Bayes classifier. The performance was evaluated using leave-one cross validation, resulting in accuracy, sensitivity and specificity of 87%, 86% and 88%, respectively.
Prostate cancer aggressiveness
In a review article, Gillies et al. (51) state: “It is axiomatic that images can be used to guide biopsies”. Quantitative imaging directed biopsies are expected to reduce underdiagnosis, with the potential to limit biopsies to regions at significant risk of containing determinate disease, thereby reducing the potential morbidity and frequency of the procedure. Moreover, since mpMRI directed biopsies result in higher rates of detection of significant cancers, the association between prostate biopsy results and patient outcome should be strengthened over time. The papers below are enabling diagnosis by utilizing radiomic analysis to determine the aggressiveness of the cancer.
Lv et al. (39) explored the potential of quantitative characterization of prostate MR images by extracting fractal features of texture and intensity distributions for differential diagnoses of prostate cancer. In a retrospective study of 55 subjects who underwent biopsy, 27 histologically positive prostate cancer patients were identified along with 28 controls with no histological abnormality. Manually segmented ROIs were placed on T2-weighted MR images in low signal regions in sextants with positive biopsy. There were a total of 130 ROIs: 65 cancerous and 65 controls. Texture fractal dimension (TFD), a measure of image roughness, was applied to the 2D ROIs. In addition, histogram fractal dimension (HFD) was recorded as a measure of the complexity of the image histogram. As expected, TFD and HFD were lower in cancerous ROIs, reflecting the typically lower heterogeneity in those regions compared to normal peripheral zone tissue AUC was used to evaluate the ability of TFD and HFD to distinguish cancerous from normal tissue, resulting in AUCs of 0.691 and 0.966, respectively.
Lopes et al. (40) likewise used fractal analysis to classify voxels as tumor or non-tumor on prostate T2-weighted MR images of 27 patients. In addition to utilizing fractal dimension (FD) and multifractional Brownian motion (mBm) modeling features, classical Haralick textural features were calculated along with Gabor filtering and wavelet frame decomposition. Voxel classification was performed using two different classification algorithms (SVM and AdaBoost), with ground truth provided by histologic maps of prostate specimens. Classification using mBm combined with FD resulted in AUC =0.92, compared to AUC =0.75 using FD alone [which may be compared to the TFD AUC result of Lv et al., (39) above]. Furthermore, classification results using fractal geometry were found to be superior to those using classical texture features, Gabor, and wavelet, which together had a lower AUC =0.88.
Wibmer et al. (42) investigated Haralick texture features of prostate mpMRI for diagnosis purposes. Using T2-weighted and diffusion-weighted MRI from 147 patients, 5 different features described in Table 1, C3 (entropy, inertia, energy, correlation and homogeneity) were computed in cancerous tissue, reported on MRI using pathology as reference, and in non-cancerous tissue. Three different types of analyses were carried out using the generalized estimating equations (GEE) method: GS 3+3 =6 vs. GS (3+4) =7 or GS (4+3) =7, GS (3+3) =6 vs. GS >7 and GS ≤ GS (3+4) =7 vs. GS > GS (4+3) =7. The results showed that all the five features differed significantly between cancerous and non-cancerous tissue in PZ on both T2w and ADC and. In TZ, inertia and correlation on T2w and all the features on ADC differed significantly (P value <0.05).
The follow-up study by Fehr et al. (47) on the same cohort of patients used a software-based automatic classification by GS using T2w and ADC. First-order features, related to the intensity volume histogram (mean, standard deviation, skewness and kurtosis) were also included. To balance the sample in terms of GS distribution, two oversampling methods, synthetic minority oversampling technique (SMOTE) and Gibbs sampling (sample generation through conditionally independent features) were used. Finally, 3 main feature selection and classification methods were evaluated: t-test Support Vector Machine (t-test SVM), Adaptive Boosting (AdaBoost) and Recursive Feature Selection Support Vector Machine (RFE-SVM). The authors report accuracy of 93% in discrimination of GS (3+3) =6 versus GS ≥7 for the RFE-SVM method using SMOTE (AUC of the same classifiers for PZ=0.99). The same methods resulted in a 92% accuracy and an AUC of 0.99 for PZ for discrimination of GS (3+4) =7 vs. GS (4+3) =7.
The aim of the article by Vignati et al. (46) was to use contrast and homogeneity from the computation of texture features on T2w and ADC to predict prostate cancer aggressiveness and to compare to traditional ADC metrics (mean, median, 10th and 25th percentile). A cohort of 93 patients who underwent mpMRI before prostatectomy were used and clinically significant tumors (≥0.5 mL) were outlined on histological sections and transferred to contours in T2w and ADC (ROIs). Spearman correlation between features in ROIs with the corresponding GS was computed. Homogeneity and contrast on T2w were found to be much better than ADC classic parameters in correlating with GS: −0.654 for contrast and 0.645 for homogeneity compared to −0.569 to ADC 10th percentile which is the highest correlation value for ADC first-order statistics parameters. AUC values for differentiating tumor foci with pathologic GS 6 from those with pathologic GS ≥7 gave the same conclusion with 0.945 and 0.962 for contrast and homogeneity on T2 and 0.854 for 10th percentile ADC.
Prostate cancer diagnosis and aggressiveness
Tiwari et al. (41) combined radiomics information with metabolic data from MR Spectroscopy (MRS) to develop computerized decision support system (DSS) for distinguishing (I) benign vs. cancerous and (II) high- (GS >7) vs. low- (GS ≤7) Gleason Score prostate cancer. The radiomic features are similar to the ones in Madabhushi et al. (38). The study reported accuracy of the detection of 86% (whole mount prostatectomy samples are used as “ground truth”).
Radiogenomics
The integration of quantitative imaging data (radiomics) to detect correlations with genomic signatures is commonly known as radiogenomics (51). The underlying hypothesis is that mpMRI radiomics features can be used to derive “radiophenotypes” that both correlate to and complement existing validated clinical and genomic risk stratification biomarkers. We performed an integrated analysis of quantitative mpMRI and gene expression in prostate cancer samples from patients undergoing mpMRI-US guided fusion prostate biopsies. This retrospective study was Health Insurance Portability and Accountability Act (HIPAA) compliant and approved by the institutional review board at University of Miami with a waiver of written informed consent. Between September 2012 and March 2014, 37 patients underwent MRI-US guided prostate biopsies at the University of Miami and 20 (54%) patients were positive for prostate cancer. Six patients had more than 2 positive biopsies and were selected for this study. The patients ranged in age from 61-85 years old and PSA values ranged from 4.2 to 10.8. Five of 6 patients had T1c and one patient had a cT2b tumor by palpation. Biopsies were reviewed by a board certified genitourinary pathologist at the University of Miami. The mean time-interval between mpMRI and biopsy was 49±16 days.
MRI-US guided fusion biopsy
The key in radiogenomic analysis is to be able to connect the gene expression of the prostate tissue with the radiomics features from the location of the tissue. The co-registration of the two types of features is of paramount importance as the prostate tumors are heavily heterogeneous. We utilize MRI-US image fusion biopsies carried out in Artemis system (Eigen, CA, USA) (52). The procedure is illustrated in Figure 3. Regions of Interest (ROIs) based on established mpMRI analysis criteria and informed by software showing habitats suspicious for harboring tumor were contoured in ProFuse (Eigen, Sun Valley, CA, USA) multi-modality image fusion software, Figure 3A. The prostate volume is outlined (yellow). A 3D transrectal ultrasound (TRUS) is acquired just prior to biopsy by reconstructing sweeps of 2D to 3D. The prostate volume is semiautomatically segmented (53) on TRUS and both these volumes are fused after specification of four or more corresponding points along the gland boundary and MRI targets are visualized on TRUS (Figure 3B). The lesion is targeted using ultrasound monitoring and the needle trajectory is visualized (Figure 3C). Tissue from identified targets was obtained for pathology and gene expression analysis.
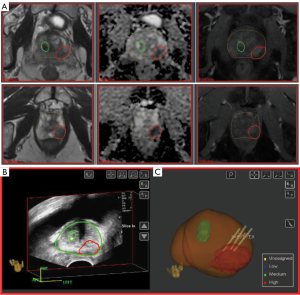
RNA extraction and microarray hybridization
From the original study (n=19), RNA was available for microarray from 17 biopsies (6 unique patients). As previously described (8,54), after histopathological re-review by an expert genitourinary pathologist, tumor was macro-dissected from surrounding stroma from 3–4 10-µm tissue sections from a region with maximum tumor content for total RNA extraction. RNA extraction and microarray hybridization was performed as previously described (8,54). Human Exon 1.0 ST GeneChips (Affymetrix, Santa Clara, CA, USA) were utilized that profile coding and non-coding regions of the transcriptome using approximately 1.4 million probe selection regions (PSRs), hereinafter referred to as features.
All of the samples with available tissue and RNA, passed initial quality control. The positive versus negative AUC was used as an additional metric to assess microarray quality by measuring the signal between positive control probes, which measure the expression of housekeeping genes, and negative control probes, which measure anti-genomic sequences and hence should exhibit background intensity levels. The percent present observed in these biopsy samples was higher than the typical range seen in formalin fixed paraffin embedded FFPE radical prostatectomy samples.
Radiomics features
The analysis followed the radiogenomic workflow presented in Figure 1. Using propriety extensions in MIM, we created a radiomics pipeline for extraction of 49 features: The volumes of prostate, PZ, TZ and ROI were estimated in MIM (n=4); Mean, median and stdev of the intensities of T2w and ADC in NAT_PZ, NAT_TZ and ROI were calculated (n=18). In addition for the ROI, the top and bottom 5 percentile (Q5 and Q95), skewness, kurtosis and integral were recorded for both T2w and ADC (n=10). The ‘extended Tofts model’ (55,56) was applied to the averaged DCE-MRI curves within NAT_PZ, NAT_TZ and ROI. Using synthetic Parker fixed population average Arterial Input Function (AIF) (57) we have shown that a valid compartmental modeling can be carried out even at the lower temporal resolution of the data (58). Three features from the pharmacokinetic analysis (Ktrans – Volume transfer constant between plasma and Extracellular Extravascular Space (EES), kep – Rate constant between EES and plasma and ve – the fraction of the EEC) were estimated for NAT_PZ, NAT_TZ and ROI (n=9). The volumes of the probability maps and their intersections with ROI were also included (n=6). Two features were also included in the analysis: extra capsular extension and location of the lesion (n=2). Each of these parameters was used in combination to define specific nomenclature for each unique feature. (For example, NAT.PZ_ADC_Mean refers to the mean ADC value in the contour of NAT in PZ).
Radiogenomic analysis
The Decipher prostate cancer classifier was developed to predict early metastasis in patients that have undergone radical prostatectomy. Decipher has undergone extensive validation in a number of independent cohorts from several institutes(8,13,59-63) and shown clinical utility in the stratification of at-risk patients who may benefit from radiation therapy from those who may benefit from deferring the secondary therapy (9,64-66). Expression patterns of the 22 gene Decipher panel in the biopsies from the analyzed cohort are illustrated as a heatmap in Figure 4. Hierarchical clustering of the gene probes segregated the patient cohort into samples with Gleason 6 and Gleason 8–9 disease. Gleason 7 samples segregated in both low and high risk clusters, in keeping with the genetic heterogeneity of this subtype. In addition to GS, the Decipher expression patterns also segregated by risk category, suggesting strong correlation between Gleason and Decipher score. Decipher scores and Gleason score were also consistent with previous evaluations of tumor specimens from RP (59). These results indicate that mpMRI habitat guided biopsies capture distinct phenotypic differences of tumors.
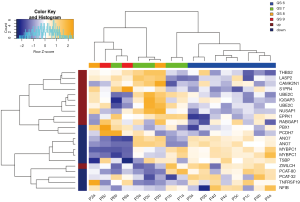
Genes from Decipher were assessed for their relationship to the radiomic features. To reveal the relationship between genomic and radiomic features, Pearson’s correlation distances between the genomic and radiomics features were calculated. Two-way hierarchical clustering of these distances is illustrated as a heatmap in Figure 5. This analysis grouped Decipher genes into two groups of expression patterns with the left side containing mostly those markers that were previously shown to be associated with tumors from patients that developed metastasis after RP, and the right side containing mostly those markers associated with tumors from patients that did not develop metastasis after RP.
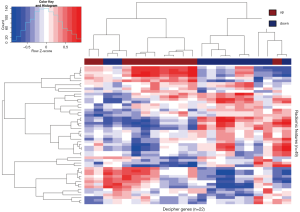
This is the first demonstration of a correlation between quantitative imaging features and genomic risk stratification in prostate cancer. This method provides a novel approach for targeting diagnostic biopsies that can directly impact clinical treatment strategies by defining highest risk disease. This data supports validation of MP-MRI based radiomics as a novel method for characterizing prostate cancer multi-focality in a large independent cohort.
Discussion
If a patient’s prostate cancer is indolent, he could be assigned to an active surveillance protocol and thus be spared from treatment-induced morbidity and avoiding significant costs. If a patient’s disease is aggressive, an appropriate treatment option must be chosen—radiotherapy or prostatectomy? Finally, if a patient is at risk of developing metastatic disease, can a precision-tailored, intensified treatment be assigned at an earlier time? Urologists and radiation oncologists struggle with these questions as the standard clinical prognostic factors of Gleason score (GS), PSA and tumor-category are insufficient for selecting an optimal patient management strategy.
The nascent field of radiomics has the potential to describe the tumor morphology using computer accessible imaging features that can be directly related to diagnosis or risk assessment. The goal of the “MRI-Guided Biopsy Selection of Prostate Cancer Patients for Active Surveillance versus Treatment: The Miami MAST Trial” (ClinicalTrials.gov: NCT02242773) is to investigate the impact of mpMRI and MRI-US fusion biopsy to identify higher grade or volume tumors early on for better selection of patients for active surveillance. This single arm trial is generating data from MRI-US fusion biopsies that are targeting the suspicious areas seen on mpMRI as well as standard template biopsies, sampling the remaining gland. The rationale is that follow-up patient data (imaging and biopsy) in clinical trials like the Miami MAST Trial will add additional information that may lead to better stratification, treatment, and prognosis of patients as well as gaining better insights into imaging features that are non-invasive biomarkers of underlying molecular pathways. These integrated markers will improve patient risk assessment and in general, improve prospective clinical trials.
As with any developing discipline, it is possible that the field will undergo a process of evolution as radiomics classification schemes are further validated and applied to larger clinical patient datasets at multiple institutions. There is large variability in imaging hardware in clinic: different MRI instruments (field strength and manufacturers); coils (endorectal or body coils). There is even larger variability in imaging sequences and methods for data reconstruction. And finally—there is a plethora of image processing approaches. For instance, the same DCE-MRI data was distributed among several academic medical centers. The participants applied pharmaco-kinetic modeling and the estimation of Ktrans, the volume transfer coefficient that measures capillary permeability (55), resulted in within-subject coefficient of variation of 0.59 (67). As the radiomics field matures, the level of standardization across medical centers will increase. There are multiple on-going efforts for standardization and for a full list of the organizations and initiatives, please refer to Gillies et al. (51).
Radiogenomics provides a noninvasive and repeatable way for investigating phenotypic information. This is the basis of the increasing role of radiomics in personalized medicine. While traditionally the discussion about personalized medicine has been centered around genomic and proteomic characterization of the tumor, the application of these techniques is hindered by uncertainties related to the location of the investigated tissue. Tumors are spatially and temporally heterogeneous; acquiring a few tissue samples without imaging guidance does not allow for complete characterization of the tumor. Prostate cancer is multifocal and heterogeneous, and thus it is challenging to determine prostate regions to biopsy that are most likely to be determinate of outcome.
We describe the extraction of quantitative mpMRI and gene expression features from prostate regions of interest informed by mpMRI directed habitat biopsies. Radiomic features are extracted from habitat biopsy location and considered in combination with features outside of the biopsy locations. The described radiogenomics pipeline will accelerate required future validation studies. The number of extracted radiomic features is also small relative to other published studies (18). With an increase in the dataset, other features such as tumor shape and texture might be added to the radiomic characterization of prostate habitats. The data indicate that our platform would significantly improve the biopsy targeting of prostate habitats through better recognition of indolent versus aggressive disease, thereby facilitating a more personalized approach to prostate cancer management. On the one hand, the expectation of non-invasively identifying habitats with high probability of housing aggressive cancers would result in directed biopsies that are more informative and actionable. Conversely, providing evidence for lack of disease would reduce the incidence of non-informative biopsies. In RT of prostate cancer, dose escalation for prostate cancer has been shown to reduce biochemical failure (68). While dose escalation also has been shown to reduce the need for androgen deprivation in intermediate to high risk patients (69-71), when the entire prostate is dose escalated, the complication risk rises. Dose escalation only to determinate prostate habitats has the potential to improve tumor control with less toxicity than when the entire prostate is dose escalated.
Acknowledgments
Funding: This work was supported by National Cancer Institute [R01CA189295 to A.P., R01CA190105 to R.J.G/A.P.]; and Bankhead Coley Cancer Research Program [1BT03 to A.P.; Imaging Core: A.P./R.S.].
Footnote
Provenance and Peer Review: This article was commissioned by the Guest Editors (Laurence E. Court, Arvind Rao and Sunil Krishnan) for the series “Radiomics in Radiation Oncology” published in Translational Cancer Research. The article has undergone external peer review.
Conflicts of Interest: M.T., N.E., and E.D. are currently and were employees of GenomeDx Biosciences during the execution of the study. The other authors have no conflicts of interest to declare.
Ethical Statement: The authors are accountable for all aspects of the work in ensuring that questions related to the accuracy or integrity of any part of the work are appropriately investigated and resolved.
Open Access Statement: This is an Open Access article distributed in accordance with the Creative Commons Attribution-NonCommercial-NoDerivs 4.0 International License (CC BY-NC-ND 4.0), which permits the non-commercial replication and distribution of the article with the strict proviso that no changes or edits are made and the original work is properly cited (including links to both the formal publication through the relevant DOI and the license). See: https://creativecommons.org/licenses/by-nc-nd/4.0/.
References
- D'Amico AV, Moul J, Carroll PR, et al. Cancer-specific mortality after surgery or radiation for patients with clinically localized prostate cancer managed during the prostate-specific antigen era. J Clin Oncol 2003;21:2163-72. [Crossref] [PubMed]
- Klotz L, Zhang L, Lam A, et al. Clinical results of long-term follow-up of a large, active surveillance cohort with localized prostate cancer. J Clin Oncol 2010;28:126-31. [Crossref] [PubMed]
- Klotz L, Vesprini D, Sethukavalan P, et al. Long-term follow-up of a large active surveillance cohort of patients with prostate cancer. J Clin Oncol 2015;33:272-7. [Crossref] [PubMed]
- Weiner AB, Patel SG, Eggener SE. Pathologic outcomes for low-risk prostate cancer after delayed radical prostatectomy in the United States. Urol Oncol 2015;33:164.e11-7. [Crossref] [PubMed]
- O'Brien D, Loeb S, Carvalhal GF, et al. Delay of surgery in men with low risk prostate cancer. J Urol 2011;185:2143-7. [Crossref] [PubMed]
- Nichol AM, Warde P, Bristow RG. Optimal treatment of intermediate-risk prostate carcinoma with radiotherapy: clinical and translational issues. Cancer 2005;104:891-905. [Crossref] [PubMed]
- Buyyounouski MK, Pickles T, Kestin LL, et al. Validating the interval to biochemical failure for the identification of potentially lethal prostate cancer. J Clin Oncol 2012;30:1857-63. [Crossref] [PubMed]
- Erho N, Crisan A, Vergara IA, et al. Discovery and validation of a prostate cancer genomic classifier that predicts early metastasis following radical prostatectomy. PloS one 2013;8:e66855 [Crossref] [PubMed]
- Badani KK, Thompson DJ, Brown G, et al. Effect of a genomic classifier test on clinical practice decisions for patients with high-risk prostate cancer after surgery. BJU international 2015;115:419-29. [Crossref] [PubMed]
- Crawford ED, Scholz MC, Kar AJ, et al. Cell cycle progression score and treatment decisions in prostate cancer: results from an ongoing registry. Curr Med Res Opin 2014;30:1025-31. [Crossref] [PubMed]
- Freedland SJ, Gerber L, Reid J, et al. Prognostic utility of cell cycle progression score in men with prostate cancer after primary external beam radiation therapy. Int J Radiat Oncol Biol Phys 2013;86:848-53. [Crossref] [PubMed]
- Cuzick J, Berney DM, Fisher G, et al. Prognostic value of a cell cycle progression signature for prostate cancer death in a conservatively managed needle biopsy cohort. Br J Cancer 2012;106:1095-9. [Crossref] [PubMed]
- Den RB, Yousefi K, Trabulsi EJ, et al. Genomic classifier identifies men with adverse pathology after radical prostatectomy who benefit from adjuvant radiation therapy. J Clin Oncol 2015;33:944-51. [Crossref] [PubMed]
- Isebaert S, Van den Bergh L, Haustermans K, et al. Multiparametric MRI for prostate cancer localization in correlation to whole-mount histopathology. J Magn Reson Imaging 2013;37:1392-401. [Crossref] [PubMed]
- Fütterer JJ, Briganti A, De Visschere P, et al. Can Clinically Significant Prostate Cancer Be Detected with Multiparametric Magnetic Resonance Imaging? A Systematic Review of the Literature. Eur Urol 2015;68:1045-53. [Crossref] [PubMed]
- Kumar V, Gu Y, Basu S, et al. Radiomics: the process and the challenges. Magn Reson Imaging 2012;30:1234-48. [Crossref] [PubMed]
- Lambin P, Rios-Velazquez E, Leijenaar R, et al. Radiomics: extracting more information from medical images using advanced feature analysis. Eur J Cancer 2012;48:441-6. [Crossref] [PubMed]
- Aerts HJ, Velazquez ER, Leijenaar RT, et al. Decoding tumour phenotype by noninvasive imaging using a quantitative radiomics approach. Nat Commun 2014;5:4006. [PubMed]
- Gatenby RA, Grove O, Gillies RJ. Quantitative imaging in cancer evolution and ecology. Radiology 2013;269:8-15. [Crossref] [PubMed]
- Gevaert O, Mitchell LA, Achrol AS, et al. Glioblastoma multiforme: exploratory radiogenomic analysis by using quantitative image features. Radiology 2014;273:168-74. [Crossref] [PubMed]
- Jamshidi N, Diehn M, Bredel M, et al. Illuminating radiogenomic characteristics of glioblastoma multiforme through integration of MR imaging, messenger RNA expression, and DNA copy number variation. Radiology 2014;270:1-2. [Crossref] [PubMed]
- Diehn M, Nardini C, Wang DS, et al. Identification of noninvasive imaging surrogates for brain tumor gene-expression modules. Proc Natl Acad Sci U S A 2008;105:5213-8. [Crossref] [PubMed]
- Shinagare AB, Vikram R, Jaffe C, et al. Radiogenomics of clear cell renal cell carcinoma: preliminary findings of The Cancer Genome Atlas-Renal Cell Carcinoma (TCGA-RCC) Imaging Research Group. Abdom Imaging 2015;40:1684-92. [Crossref] [PubMed]
- Segal E, Sirlin CB, Ooi C, et al. Decoding global gene expression programs in liver cancer by noninvasive imaging. Nat Biotechnol 2007;25:675-80. [Crossref] [PubMed]
- Rubin DL, Rodriguez C, Shah P, et al. iPad: Semantic annotation and markup of radiological images. AMIA Annu Symp Proc 2008;626-30. [PubMed]
- Nelson AS, Piper J, Curry K, et al. TU-CD-BRA-04: Evaluation of An Atlas-Based Segmentation Method for Prostate and Peripheral Zone Regions On MRI. Med Phys 2015;42:3606. [Crossref]
- Stoyanova R, Huang K, Sandler K, et al. Mapping Tumor Hypoxia In Vivo Using Pattern Recognition of Dynamic Contrast-enhanced MRI Data. Transl Oncol 2012;5:437-47. [Crossref] [PubMed]
- Somford DM, Hambrock T, Hulsbergen-van de Kaa CA, et al. Initial experience with identifying high-grade prostate cancer using diffusion-weighted MR imaging (DWI) in patients with a Gleason score </= 3 + 3 = 6 upon schematic TRUS-guided biopsy: a radical prostatectomy correlated series. Invest Radiol 2012;47:153-8. [PubMed]
- Litjens GJ, Hambrock T, Hulsbergen-van de Kaa C, et al. Interpatient variation in normal peripheral zone apparent diffusion coefficient: effect on the prediction of prostate cancer aggressiveness. Radiology 2012;265:260-6. [Crossref] [PubMed]
- Hoeks CM, Vos EK, Bomers JG, et al. Diffusion-weighted magnetic resonance imaging in the prostate transition zone: histopathological validation using magnetic resonance-guided biopsy specimens. Invest Radiol 2013;48:693-701. [Crossref] [PubMed]
- Hambrock T, Somford DM, Huisman HJ, et al. Relationship between apparent diffusion coefficients at 3.0-T MR imaging and Gleason grade in peripheral zone prostate cancer. Radiology 2011;259:453-61. [Crossref] [PubMed]
- Hambrock T, Hoeks C, Hulsbergen-van de Kaa C, et al. Prospective assessment of prostate cancer aggressiveness using 3-T diffusion-weighted magnetic resonance imaging-guided biopsies versus a systematic 10-core transrectal ultrasound prostate biopsy cohort. Eur Urol 2012;61:177-84. [Crossref] [PubMed]
- Bittencourt LK, Barentsz JO, de Miranda LC, et al. Prostate MRI: diffusion-weighted imaging at 1.5T correlates better with prostatectomy Gleason Grades than TRUS-guided biopsies in peripheral zone tumours. Eur Radiol 2012;22:468-75. [Crossref] [PubMed]
- Haralick RM, Haralic RM. Statistical and structural approaches to texture. Proc IEEE 1979;67:786-804. [Crossref]
- Oczeretko E, Borowska M, Kitlas A, et al. Fractal analysis of medical images in the irregular regions of interest. Athens: IEEE Publications;2008:1-6.
- Yoo TS, Ackerman MJ, Lorensen WE, et al. Engineering and algorithm design for an image processing Api: a technical report on ITK--the Insight Toolkit. Stud Health Technol Inform 2002;85:586-92. [PubMed]
- Yoo TS, Metaxas DN. Open science--combining open data and open source software: medical image analysis with the Insight Toolkit. Med Image Anal 2005;9:503-6. [Crossref] [PubMed]
- Madabhushi A, Feldman MD, Metaxas DN, et al. Automated detection of prostatic adenocarcinoma from high-resolution ex vivo MRI. IEEE Trans Med Imaging 2005;24:1611-25. [Crossref] [PubMed]
- Lv D, Guo X, Wang X, et al. Computerized characterization of prostate cancer by fractal analysis in MR images. J Magn Reson Imaging 2009;30:161-8. [Crossref] [PubMed]
- Lopes R, Ayache A, Makni N, et al. Prostate cancer characterization on MR images using fractal features. Med Phys 2011;38:83-95. [Crossref] [PubMed]
- Tiwari P, Kurhanewicz J, Madabhushi A. Multi-kernel graph embedding for detection, Gleason grading of prostate cancer via MRI/MRS. Med Image Anal 2013;17:219-35. [Crossref] [PubMed]
- Wibmer A, Hricak H, Gondo T, et al. Haralick texture analysis of prostate MRI: utility for differentiating non-cancerous prostate from prostate cancer and differentiating prostate cancers with different Gleason scores. Eur Radiol 2015;25:2840-50. [Crossref] [PubMed]
- Kwak JT, Xu S, Wood BJ, et al. Automated prostate cancer detection using T2-weighted and high-b-value diffusion-weighted magnetic resonance imaging. Med Phys 2015;42:2368-78. [Crossref] [PubMed]
- Khalvati F, Wong A, Haider MA. Automated prostate cancer detection via comprehensive multi-parametric magnetic resonance imaging texture feature models. BMC Med Imaging 2015;15:27. [Crossref] [PubMed]
- Cameron A, Khalvati F, Haider MA, et al. MAPS: A Quantitative Radiomics Approach for Prostate Cancer Detection. IEEE Trans Biomed Eng 2016;63:1145-56. [Crossref] [PubMed]
- Vignati A, Mazzetti S, Giannini V, et al. Texture features on T2-weighted magnetic resonance imaging: new potential biomarkers for prostate cancer aggressiveness. Phys Med Biol 2015;60:2685-701. [Crossref] [PubMed]
- Fehr D, Veeraraghavan H, Wibmer A, et al. Automatic classification of prostate cancer Gleason scores from multiparametric magnetic resonance images. Proc Natl Acad Sci U S A 2015;112:E6265-73. [Crossref] [PubMed]
- Litjens GJ, Elliottb R, Shih N, et al. Distinguishing prostate cancer from benign confounders via a cascaded classifier on multi-parametric MRI. Proc SPIE 2014;9035:903512 [Crossref]
- Litjens GJ, Elliott R, Shih NN, et al. Computer-extracted Features Can Distinguish Noncancerous Confounding Disease from Prostatic Adenocarcinoma at Multiparametric MR Imaging. Radiology 2016;278:135-45. [Crossref] [PubMed]
- Ojala T, Pietikainen M, Maenpaa T, et al. Multiresolution gray-scale and rotation invariant texture classification with local binary patterns. IEEE 2002;971-87.
- Gillies RJ, Kinahan PE, Hricak H. Radiomics: Images Are More than Pictures, They Are Data. Radiology 2016;278:563-77. [Crossref] [PubMed]
- Narayanan R, Kurhanewicz J, Shinohara K, et al. Mri-ultrasound registration for targeted prostate biopsy. IEEE Internaional Symposium on Biomedical Imaging - From Nano to Macro; 2009 Jun 28-Jul 01;Boston, USA. New York: IEEE, 2009:991-4.
- Ladak HM, Mao F, Wang YQ, et al. Prostate boundary segmentation from 2D ultrasound images. Medical Physics 2000;27:1777-88. [Crossref] [PubMed]
- Nakagawa T, Kollmeyer TM, Morlan BW, et al. A tissue biomarker panel predicting systemic progression after PSA recurrence post-definitive prostate cancer therapy. PloS one 2008;3:e2318 [Crossref] [PubMed]
- Tofts PS. Modeling tracer kinetics in dynamic Gd-DTPA MR imaging. J Magn Reson Imaging 1997;7:91-101. [Crossref] [PubMed]
- Tofts PS, Brix G, Buckley DL, et al. Estimating kinetic parameters from dynamic contrast-enhanced T(1)-weighted MRI of a diffusable tracer: standardized quantities and symbols. J Magn Reson Imaging 1999;10:223-32. [Crossref] [PubMed]
- Parker GJ, Roberts C, Macdonald A, et al. Experimentally-derived functional form for a population-averaged high-temporal-resolution arterial input function for dynamic contrast-enhanced MRI. Magn Reson Med 2006;56:993-1000. [Crossref] [PubMed]
- Tofts PS, Stoyanova R. Modelling slow DCE data from prostate: rate constant (kep) and Extracellular Extravascular Space (EES: ve) both distinguish hypoxic regions in the tumour. European Society for Magnetic Resonance in Medicine and Biology (ESMRMB) Congress; Leipzig. 2011.
- Karnes RJ, Bergstralh EJ, Davicioni E, et al. Validation of a genomic classifier that predicts metastasis following radical prostatectomy in an at risk patient population. J Urol 2013;190:2047-53. [Crossref] [PubMed]
- Klein EA, Yousefi K, Haddad Z, et al. A Genomic Classifier Improves Prediction of Metastatic Disease Within 5 Years After Surgery in Node-negative High-risk Prostate Cancer Patients Managed by Radical Prostatectomy Without Adjuvant Therapy. Eur Urol 2015;67:778-86. [Crossref] [PubMed]
- Den RB, Feng FY, Showalter TN, et al. Genomic prostate cancer classifier predicts biochemical failure and metastases in patients after postoperative radiation therapy. Int J Radiat Oncol Biol Phys 2014;89:1038-46. [Crossref] [PubMed]
- Ross AE, Feng FY, Ghadessi M, et al. A genomic classifier predicting metastatic disease progression in men with biochemical recurrence after prostatectomy. Prostate Cancer Prostatic Dis 2014;17:64-9. [Crossref] [PubMed]
- Prensner JR, Zhao S, Erho N, et al. RNA biomarkers associated with metastatic progression in prostate cancer: a multi-institutional high-throughput analysis of SChLAP1. The Lancet Oncology 2014;15:1469-80. [Crossref] [PubMed]
- Lobo JM, Dicker AP, Buerki C, et al. Evaluating the clinical impact of a genomic classifier in prostate cancer using individualized decision analysis. PloS one 2015;10:e0116866 [Crossref] [PubMed]
- Michalopoulos SN, Kella N, Payne R, et al. Influence of a genomic classifier on post-operative treatment decisions in high-risk prostate cancer patients: results from the PRO-ACT study. Curr Med Res Opin 2014;30:1547-56. [Crossref] [PubMed]
- Badani K, Thompson DJ, Buerki C, et al. Impact of a genomic classifier of metastatic risk on postoperative treatment recommendations for prostate cancer patients: a report from the DECIDE study group. Oncotarget 2013;4:600-9. [Crossref] [PubMed]
- Huang W, Li X, Chen Y, et al. Variations of dynamic contrast-enhanced magnetic resonance imaging in evaluation of breast cancer therapy response: a multicenter data analysis challenge. Transl Oncol 2014;7:153-66. [Crossref] [PubMed]
- Kuban DA, Levy LB, Cheung MR, et al. Long-term failure patterns and survival in a randomized dose-escalation trial for prostate cancer. Who dies of disease? Int J Radiat Oncol Biol Phys 2011;79:1310-7. [Crossref] [PubMed]
- Demanes DJ, Brandt D, Schour L, et al. Excellent results from high dose rate brachytherapy and external beam for prostate cancer are not improved by androgen deprivation. Am J Clin Oncol 2009;32:342-7. [Crossref] [PubMed]
- Dattoli M, Wallner K, True L, et al. Long-term outcomes for patients with prostate cancer having intermediate and high-risk disease, treated with combination external beam irradiation and brachytherapy. J Oncol 2010;2010.
- Bauman G, Haider M, Van der Heide UA, et al. Boosting imaging defined dominant prostatic tumors: a systematic review. Radiother Oncol 2013;107:274-81. [Crossref] [PubMed]