A signature of four ferroptosis-related genes in laryngeal squamous cell carcinoma
Highlight box
Key findings
• In this study, we developed a prognostic model for laryngeal squamous cell carcinoma by integrating patient gene expression and clinical characteristics.
What is known and what is new?
• While numerous prognostic models for laryngeal cancer exist, there is a lack of validation.
• In our research, we established a novel prognostic model based on public databases, evaluating the expression of the signature of four genes in the cell lines of head and neck squamous cell carcinoma.
What is the implication, and what should change now?
• This model facilitates the calculation of survival probabilities, assisting clinicians in planning follow-up strategies and contributing to precision medicine.
Introduction
Laryngeal squamous cell carcinoma (LSCC) is a common malignant tumor of the head and neck squamous cell carcinoma (HNSCC), which originates from the mucosal epithelium of the larynx. It is estimated that 177,422 new patients are diagnosed, and approximately 94,771 die globally in 2018 (1). LSCC, marked by swift advancement, local spread, and lymph node spreading, severely hinders patients’ ability to speak, swallow, and breathe. This ultimately results in rapid cancer-related death (2). Significant advancements have been made in treating LSCC over the past decades. Based on surgery as the primary option, many more viable non-surgical options exist, such as radiotherapy and systemic therapy (3). Unfortunately, the prognosis of laryngeal carcinoma has not improved. The 5-year survival rate has declined from 66% to 63% over the past 40 years, although the overall incidence is decreasing (3). Therefore, it is crucial to probe the pathogenesis, assess the prognosis, and explore effective new therapeutic targets for laryngeal carcinoma.
Ferroptosis is a kind of regulated cell death (RCD) that is mainly related to iron-mediated oxidative damage and subsequent cell membrane damage (4). Inhibiting cell membrane transporters and blocking the activation of intracellular antioxidant enzymes are the ways to activate the process of ferroptosis. Enriched ferroptosis correlates with aggravated immunosuppression and thus favors tumor growth (5). Nevertheless, interfering with the metabolism of cystine by agents can induce ferroptosis and disrupt tumor cell growth (6). The dual role in tumors suggests that we may treat the tumor by ferroptosis (4). Recently, the most commonly used anti-cancer drug, cyclophosphamide (CTX), has been identified with its primary antitumor mechanism for inducing ferroptosis in cancer cells (7). RB1-inducible coiled-coil 1 related signaling pathway makes tumor cells sensitive to ferroptosis, and targeting RB1-inducible coiled-coil 1 may benefit tumor therapy (8).
A growing number of studies have explored the role of genes associated with ferroptosis in HNSCC. Ferroptosis may participate in the progression of HNSCC through the immune microenvironment and TP53 mutation (9). Interleukin-6 could facilitate ferroptosis resistance in HNSCC and thus may be utilized as a target for tumor prevention and therapy (10). Recent research has introduced a novel prognostic signature comprising seven ferroptosis-related genes for predicting the prognosis of HNSCC, which suggested that targeting ferroptosis could represent a potential therapeutic strategy for HNSCC (11). However, only some studies have focused on the role of ferroptosis-related genes in LSCC. Another research has revealed that the ferroptosis-related gene SLC3A2 negatively regulates ferroptosis through the mammalian target of rapamycin (mTOR) pathway in LSCC (12). The mechanisms of how ferroptosis-related genes regulate the development and advancement of LSCC still needs to be determined. On that account, to further work out the relationship between ferroptosis and LSCC development is helpful to explore the novel therapy scheme and improve the overall survival (OS) rate of LSCC (13,14).
In this study, differentially expressed genes (DEGs) between LSCC and adjacent normal tissues in the public database were analyzed. Ferroptosis-related genes (drivers, suppressors, and markers) were obtained from the FerrDb database (15). After comparison, the ferroptosis-related differential genes were obtained and analyzed by functional enrichment analysis to explore the potential mechanism. After screening the prognostic genes, we established a prognostic model and constructed a nomogram with clinical information to predict the prognosis of LSCC patients. We present this article in accordance with the MDAR and TRIPOD reporting checklists (available at https://tcr.amegroups.com/article/view/10.21037/tcr-23-2046/rc).
Methods
Data acquisition
The RNA expression data obtained under accession number Gene Expression Omnibus (GEO): GSE127165 (16), which consisted of expression profiling by high throughput sequencing of 57 LSCC and paired adjacent normal tissues, were downloaded from the GEO database (https://www.ncbi.nlm.nih.gov/geo). Additionally, the transcriptome RNA sequencing data [fragments per kilobase million (FPKM)] and corresponding clinical pathologic information of LSCC patients were sourced from The Cancer Genome Atlas (TCGA) database (https://www.cancer.gov/tcga/). 259 ferroptosis-related genes, including drivers, suppressors, and markers, were obtained from the FerrDb database (http://www.zhounan.org/ferrdb/) (15). We selected them as FerrDb genes. This study was conducted in accordance with the Declaration of Helsinki (as revised in 2013).
Identification of differentially expressed ferroptosis genes
The identification of DEGs between tumor and paired adjacent normal tissues was conducted using the GEO dataset GSE127165. This analysis was performed in R (version 4.2.1) utilizing the “limma” package. (17). The DEGs were determined using the criteria of |log2 fold change (FC)| >1 and an adjusted P value <0.05. The R package “pheatmap” was employed to visualize the extent of differences in the two GEO datasets. Then, the ferroptosis-related differentially expressed genes (FDGs) were obtained using Venny (version 2.1) (18) to overlap the intersection of DEGs and FerrDb genes.
Functional enrichment analysis
The FDGs were submitted to Metascape Online (19) (https://metascape.org/gp/index.html#/main/step1), which incorporates a core set of default ontologies like Gene Ontology (GO) biological processes, Kyoto Encyclopedia of Genes and Genomes (KEGG) pathways, Canonical pathways, etc., to conduct functional analysis and construct a protein-protein interaction (PPI) network. Molecular complex detection (MCODE) was performed to unveil the densely connected regions further, employing a cutoff value of P<0.05.
Establishment of the prognostic model of the ferroptosis-related genes
LSCC patients from TCGA database was used to establish a prognostic model of ferroptosis-related gene. By using the R packages “survival” and “survminer”, a univariate Cox analysis of OS was first performed to identify the survival-related genes among the FDGs with a prognosis value (P<0.05) and incorporated into the subsequent least absolute shrinkage and selection operator (LASSO) regression analysis. LASSO regression analysis was employed to mitigate the risk of overfitting and identify the most relevant prognostic genes. The prognostic model was calculated based on the normalized gene expression level and corresponding regression coefficients. Kaplan-Meier (K-M) survival curves were used to show the prognostic value of genes. Then, LASSO regression was performed for these genes to generate a prognostic model. The risk score of prognosis was determined by using a linear combination of the regression coefficient in the LASSO regression and the expression level of the gene. The Tumor IMmune Estimation Resource (TIMER, https://cistrome.shinyapps.io/timer/) database was used to obtain the expression of genes in pan-cancer.
The assessment of the prognosis model
LSCC patients from TCGA datasets were divided into high-risk and low-risk groups by the median of the risk score. The K-M survival curve was performed to assess prognostic significance. The receiver operating characteristic (ROC) curve was created by using the R package “timeROC”, and the area under the curve (AUC) in1-, 3-, and 5-year OS was used to evaluate the performance of the prognostic model.
Nomogram construction and evaluation
We performed univariate and multivariate Cox analyses of patient clinical factors, including gender, age, TNM stage, histological grade, margin status, and person neoplasm cancer status, to determine the significance of each factor in predicting OS in patients with LSCC. Based on the results of multivariate Cox regression, the R package “rms” was used to construct a nomogram to provide the 1-, 3- and 5-year survival probabilities. The calibration curve evaluated the predictive ability of the nomogram.
Analysis of public databases
The Cancer Cell Line Encyclopedia (CCLE) database (https://portals.broadinstitute.org/ccle) (20) and The Human Protein Atlas (HPA) database (https://www.proteinatlas.org/) (21) were utilized to confirm the four prognostic model genes expression across HNSCC through RNA-Seq results.
Cell culture and qRT-PCR
The human keratinocyte HaCaT cell line (RRID: CVCL_0038) was obtained from the National Institutes of Health (Bethesda, MD, USA). The human laryngeal carcinoma cell lines HEp-2 (RRID: CVCL_1906) and AME-HN-8 (CVCL_5966) were obtained from the FuHeng BioLogy (Shanghai, China). The cell lines were cultured in Dulbecco’s Modified Eagle Medium (DMEM) supplemented with 10% fetal bovine serum (both from GIBCO, CA, USA), 100 U/mL penicilin and 100 µg/mL streptomycin. All cells were maintained at 37 ℃ in 5% CO2. Total RNA was extracted from cell lines using the total RNA rapid extraction kit (Fastagen, Shanghai, China), and RNA concentration was calculated. The cDNA was synthesized using the ReverTra Ace qPCR RT Kit (Toyobo, Osaka, Japan). Quantitative real-time polymerase chain reaction (qRT-PCR) was performed using the Taq Pro Universal SYBR qPCR Master Mix (Vazyme, Nanjing, China). The primers are listed in Table 1. GAPDH was used as an internal control.
Table 1
Gene name | Primer type | Primer sequence |
---|---|---|
NCF2 | Forward primer | 5'-ACTACTGCCTGACTCTGTGGTG-3' |
Reverse primer | 5'-CCTCCACTTGGCTGCCTTTCTT-3' | |
PLIN4 | Forward primer | 5'-CCCACGCTTGGCAATGCTGCA-3' |
Reverse primer | 5'-TGTTCCGCCGACAGCACCTTTG-3' | |
RGS4 | Forward primer | 5'-ACATCGGCTAGGTTTCCTGCTG-3' |
Reverse primer | 5'-CAGGTTTTCCAGTGATTCAGCCC-3' | |
TFRC | Forward primer | 5'-ATCGGTTGGTGCCACTGAATGG-3' |
Reverse primer | 5'-ACAACAGTGGGCTGGCAGAAAC-3' | |
GAPDH | Forward primer | 5'-GTCTCCTCTGACTTCAACAGCG-3' |
Reverse primer | 5'-ACCACCCTGTTGCTGTAGCCAA-3' |
qRT-PCR, quantitative real-time polymerase chain reaction.
Statistical analysis
R software (version 4.2.1) and GraphPad Prism9 software (GraphPad Software Inc., LaJolla, CA, USA) were used to perform data analysis. The statistical value P<0.05 indicated that the difference was statistically significant.
Results
FDGs in LSCC
The information of GEO and TCGA databases used is listed in Table 2. Through the comparison of tumor tissues and adjacent non-neoplastic tissues in GEO: GSE127165, a total of 2,112 DEGs were identified. Among these, 1,110 genes exhibited upregulation, while 1,002 showed downregulation in tumor tissues (table available at https://cdn.amegroups.cn/static/public/tcr-23-2046-1.pdf). The DEGs obtained from the GEO datasets were intersected with the 259 FerrDb genes to obtain FDGs. There were 36 genes overlapped between the two datasets (Figure 1A).
Table 2
Data sources | Platform | Samples | Scanned items | PMID | Clinical files |
---|---|---|---|---|---|
GEO: GSE127165 | GPL20301 | 57 | LncRNA + mRNA | 32487167 | No |
TCGA: LSCC | N/A | 111 | mRNA | N/A | Yes |
GEO, Gene Expression Omnibus; TCGA, The Cancer Genome Atlas; LSCC, laryngeal squamous cell carcinoma; N/A, not applicable.
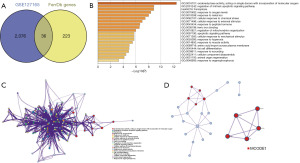
Functional enrichment analysis
The distinctive biological processes and pathway features associated with the 36 FDGs were subsequently analyzed. The findings were visualized through both bar graphs and PPI networks. The GO and KEGG analyses showed that enrichments were mainly focused on oxidoreductase activity, regulation of intrinsic apoptotic signaling pathway, and ferroptosis, etc. (Figure 1B). Furthermore, the significant module was identified by the PPI network and MCODE (Figure 1C). SLC3A2, SLC7A5, CAV1, PANX1, and SLC7A11 made up this module (Figure 1D).
Construction of a ferroptosis-related prognostic model
FPKM values, along with corresponding patient information, were acquired from the TCGA datasets of LSCC patients. By utilizing these data, we conducted further analysis on 36 overlapping FDGs. Univariate Cox regression analysis showed that four FDGs were significantly associated with prognosis (Table S1, Figure 2A). And this four FDGs were later identified as prognostic signatures through a LASSO regression analysis (Figure 2B). K-M survival curves of the four genes showed that the high expression of NCF2, RGS4, and TFRC had shorter OS than the patients of low expression, while the low expression of PLIN4 had shorter OS than the patients of high expression (Figure 2C-2F). Therefore, a prognostic model was formulated utilizing the coefficients in the LASSO regression analysis and the FPKM value from TCGA datasets (Table 3). A risk score for each patient was determined using the following formula: 0.0389 × (expression of NCF2) + (−0.3114) × (expression of PLIN4) + 0.085 × (expression of RGS4) + 0.0044 × (expression of TFRC). The TIMER database previewed the expression of these four prognostic signatures in pan-cancer. All four signatures were found to be differently expressed in HNSCC (Figure 2G-2J).
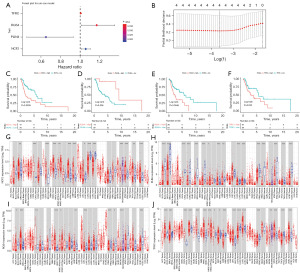
Table 3
Gene | Coef |
---|---|
NCF2 | 0.0389 |
PLIN4 | −0.3114 |
RGS4 | 0.085 |
TFRC | 0.0044 |
LASSO, least absolute shrinkage and selection operator.
Estimations of the prognosis model in the TCGA datasets
The patients from the TCGA datasets were divided into the high-risk group (n=55) and the low-risk group (n=56) by the median value of the risk score. The patients in the high-risk group had a significantly worse prognosis than the low-risk group (log-rank test P=0.0023, Figure 3A). The AUC values of the 1-, 3- and 5-year OS rates with the prognostic model were 0.74, 0.71, and 0.75, respectively (Figure 3B). The distribution of the risk score is shown in Figure 3C,3D.
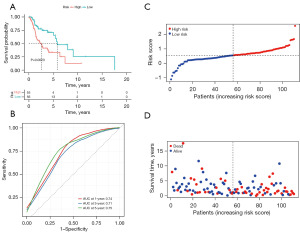
Establishment and evaluation of the nomogram
The univariate and multivariate Cox regression analyses showed that the risk score and clinical factors of M stage and person neoplasm cancer status were the independent significant prognostic factors in predicting OS in patients with LSCC (Table S2 and Figure S1). We subsequently constructed a predictive nomogram with these factors (Figure 4A). Besides, the C index calculated by R for the nomogram was 0.805, suggesting that the nomogram had a superior predictive performance. The calibration curves were used to evaluate the accuracy of the nomogram, in which a standard curve represented the best prediction. The predicted outcomes of 1-, 3- and 5-year OS rates showed excellent consistency (Figure 4B-4D).
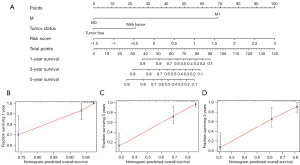
Validation of the expression properties of the four prognostic model genes using public databases and qRT-PCR
To evaluate the expression profile of four prognostic model genes, we examined RNA expression data from HNSCC cell lines in the CCLE and HPA databases. Notably, NCF2 and TFRC exhibit significant expression levels, whereas PLIN4 shows lower expression. Additionally, RGS4 displays relatively low expression overall but is prominently expressed in the LSCC cell line SNU-46 (Figure 5A-5H).
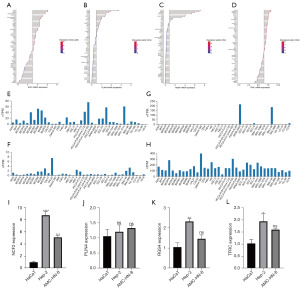
The mRNA expression levels of these four prognostic model genes were measured in HaCaT cells, HEp-2 cells, and AME-HN-8 cells. qRT-PCR confirmed that the mRNA levels of NCF2, RGS4, and TFRC were significantly higher in HEp-2 cells than in HaCaT cells, and there was no significant difference in the mRNA level of PLIN4 between HaCaT cells and HEp-2 cells. The mRNA level of NCF2 was significantly higher in AME-HN-8 cells than in HaCaT cells, and there were no significant differences in the mRNA levels of PLIN4, RGS4, and TFRC between HaCaT cells and AME-HN-8 cells (Figure 5I-5L).
Discussion
Cancer cells can undergo several regulated forms of cell death during tumor development, including apoptosis, autophagy, and necrosis (22). As a RCD, ferroptosis is involved in the process of tumor cell death and can inhibit tumors through lipid peroxidation (23). Therefore, inducing ferroptosis is expected to eradicate cancer cells, and researchers focus on how to develop anti-cancer drugs induced by ferroptosis (24). Ferroptosis plays an essential role in HNSCC. Epstein-Barr virus is closely related to the occurrence of nasopharyngeal carcinoma. A recent study revealed that Epstein-Barr virus infection could reduce the sensitivity of nasopharyngeal carcinoma cells to ferroptosis by activating the p62-Keap1-NRF2 signaling pathway in conjunction with upregulated expression of SLC7A11 and GPX4 (25). In mutant p53 hypopharyngeal squamous carcinoma, the combination therapy of low-concentration paclitaxel and RSL3 induces ferroptosis and significant cell death (26). By the principal component analysis algorithm, one research (27) evaluated the ferroptosis regulation patterns of oral squamous cell carcinoma and found that patients with a high score of ferroptosis, which means the high expression of ferroptosis-specific gene signature, were associated with a favorable prognosis, a ferroptosis-related immune-activation phenotype, potential sensitivities to the chemotherapy and immunotherapy. Based on three ferroptosis-related genes, another research developed a ferroptosis-related model to predict the prognosis of LSCC (28). However, our research methods and contents are different from theirs.
In the current study, we used the DEGs from GEO: GSE127165, which generated 57 LSCC and paired adjacent normal tissues using high throughput sequencing. After intersecting with the 259 FerrDb genes, 36 FDGs were obtained, and functional analysis was subsequently performed based on them. Oxidoreductase activity, intrinsic apoptotic signaling pathway regulation, and ferroptosis, among others, were major enriched pathways. By activating PPAR-γ, xanthine oxidoreductase (XOR) promotes cell differentiation and produces an antitumorigenic and antiproliferative action (29). Moreover, the low XOR activity, together with an increase in purine biosynthesis, provides a selective advantage to the growth of tumor tissue. Apoptosis can be induced in cancer cells through intrinsic and extrinsic pathways, and it relies on activating distinct signaling pathways which are often deregulated in cancer (29). In summary, these 36 FDGs were intricately linked to the processes of tumor initiation and progression.
Next, we used univariate Cox and LASSO regression analyses to screen out four significant genes for the OS of LSCC. Based on the expression values of these genes and their regression coefficients, we established a new prognostic model integrating the four ferroptosis-related genes. The K-M and ROC curves suggested that the model had good predictive value. Nomograms have been well developed as a prognostic assessment tool and have been proven to be more accurate than conventional staging systems in several cancers (30). We constructed a nomogram in combination with clinical factors. The C index (0.805) and the calibration curves confirmed that the model had a good predictive performance. Finally, we evaluated the mRNA level of the four model genes between HNSCC cells and HaCaT cells.
According to our study, NCF2, PLIN4, RGS4, and TFRC were the four FDGs in LSCC samples. NCF2 encodes neutrophil cytosolic factor 2, the 67-kilodalton cytosolic subunit of the multi-protein NADPH oxidase complex found in neutrophils (31). NCF2 may promote ferroptosis (32). NCF2 may be a biomarker for esophageal squamous cell carcinoma and non-small cell lung cancer (33,34). PLIN4 (perilipin 4) is a member of the perilipin family, and these proteins coat the intracellular lipid storage droplets (LSD) (35). PLIN4 can be upregulated in cells treated with ferroptosis inducer erastin and may promote ferroptosis in a GSH-dependent manner (36). It can be used as a biomarker that reveals poor prognosis and may serve as a therapeutic target for breast cancer (37). Regulators of G protein signaling (RGS) family members are regulatory molecules that act as GTPase activating proteins (GAPs) for G alpha subunits of heterotrimeric G proteins, and regulator of G protein signaling 4 (RGS4) belongs to this family (38). RGS4 may inhibit ferroptosis because it could be down-regulated in erastin-treated samples (39), and it plays a crucial role in tumor immunity and the prognosis of Ewing sarcoma (40). Transferrin receptor (TFRC) has dual roles in ferroptosis (41,42). In liver cancer, O-GlcNAcylation could enhance sensitivity to RSL3-induced ferroptosis via the YAP/TFRC pathway (43). In laryngeal carcinoma, lncRNA LINC00888 upregulation could accelerate the growth and mobility of cancer cells through the regulation of miR-378g/TFRC (44). Similarly, in our study, the expression of these four genes was significantly different between tumor tissues and adjacent non-neoplastic tissues in GEO datasets. Moreover, our study confirmed that high NCF2, RGS4, and TFRC and low expression of PLIN4 were associated with worse outcomes in 111 LSCC patients from the TCGA datasets.
There are some limitations in our study. Firstly, our research is limited to bioinformatics analysis of the data from the public database and cell experiments, lacking validation with a larger sample of cases. In addition, except for changes at the RNA level, the spatial expression at the protein level and in-depth mechanism could be investigated further.
Conclusions
This study defined a novel prognostic model of four ferroptosis-related genes that were closely related to the prognosis of laryngeal cancer. Our model showed better predictive value. These efforts may provide some new insights into the treatment of LSCC. Nevertheless, further research on the underlying mechanisms is warranted.
Acknowledgments
We sincerely acknowledge the contributions from the GEO project, TCGA project, FerrDb database, and Metascape Online.
Funding: This study was supported by
Footnote
Reporting Checklist: The authors have completed the MDAR and TRIPOD reporting checklists. Available at https://tcr.amegroups.com/article/view/10.21037/tcr-23-2046/rc
Data Sharing Statement: Available at https://tcr.amegroups.com/article/view/10.21037/tcr-23-2046/dss
Peer Review File: Available at https://tcr.amegroups.com/article/view/10.21037/tcr-23-2046/prf
Conflicts of Interest: All authors have completed the ICMJE uniform disclosure form (available at https://tcr.amegroups.com/article/view/10.21037/tcr-23-2046/coif). The authors have no conflicts of interest to declare.
Ethical Statement: The authors are accountable for all aspects of the work in ensuring that questions related to the accuracy or integrity of any part of the work are appropriately investigated and resolved. The study was conducted in accordance with the Declaration of Helsinki (as revised in 2013).
Open Access Statement: This is an Open Access article distributed in accordance with the Creative Commons Attribution-NonCommercial-NoDerivs 4.0 International License (CC BY-NC-ND 4.0), which permits the non-commercial replication and distribution of the article with the strict proviso that no changes or edits are made and the original work is properly cited (including links to both the formal publication through the relevant DOI and the license). See: https://creativecommons.org/licenses/by-nc-nd/4.0/.
References
- Bray F, Ferlay J, Soerjomataram I, et al. Global cancer statistics 2018: GLOBOCAN estimates of incidence and mortality worldwide for 36 cancers in 185 countries. CA Cancer J Clin 2018;68:394-424. [Crossref] [PubMed]
- Zhao K, Chen L, Xie Y, et al. m6A/HOXA10-AS/ITGA6 axis aggravates oxidative resistance and malignant progression of laryngeal squamous cell carcinoma through regulating Notch and Keap1/Nrf2 pathways. Cancer Lett 2024;587:216735. [Crossref] [PubMed]
- Steuer CE, El-Deiry M, Parks JR, et al. An update on larynx cancer. CA Cancer J Clin 2017;67:31-50. [Crossref] [PubMed]
- Chen X, Kang R, Kroemer G, et al. Broadening horizons: the role of ferroptosis in cancer. Nat Rev Clin Oncol 2021;18:280-96. [Crossref] [PubMed]
- Liu T, Zhu C, Chen X, et al. Ferroptosis, as the most enriched programmed cell death process in glioma, induces immunosuppression and immunotherapy resistance. Neuro Oncol 2022;24:1113-25. [Crossref] [PubMed]
- Stockwell BR, Friedmann Angeli JP, Bayir H, et al. Ferroptosis: A Regulated Cell Death Nexus Linking Metabolism, Redox Biology, and Disease. Cell 2017;171:273-85. [Crossref] [PubMed]
- Shi H, Hou B, Li H, et al. Cyclophosphamide Induces the Ferroptosis of Tumor Cells Through Heme Oxygenase-1. Front Pharmacol 2022;13:839464. [Crossref] [PubMed]
- Xue X, Ma L, Zhang X, et al. Tumour cells are sensitised to ferroptosis via RB1CC1-mediated transcriptional reprogramming. Clin Transl Med 2022;12:e747. [Crossref] [PubMed]
- Fan X, Ou Y, Liu H, et al. A Ferroptosis-Related Prognostic Signature Based on Antitumor Immunity and Tumor Protein p53 Mutation Exploration for Guiding Treatment in Patients With Head and Neck Squamous Cell Carcinoma. Front Genet 2021;12:732211. [Crossref] [PubMed]
- Li M, Jin S, Zhang Z, et al. Interleukin-6 facilitates tumor progression by inducing ferroptosis resistance in head and neck squamous cell carcinoma. Cancer Lett 2022;527:28-40. [Crossref] [PubMed]
- He F, Chen Z, Deng W, et al. Development and validation of a novel ferroptosis-related gene signature for predicting prognosis and immune microenvironment in head and neck squamous cell carcinoma. Int Immunopharmacol 2021;98:107789. [Crossref] [PubMed]
- Wu F, Xiong G, Chen Z, et al. SLC3A2 inhibits ferroptosis in laryngeal carcinoma via mTOR pathway. Hereditas 2022;159:6. [Crossref] [PubMed]
- Xu L, Li W, Liu D, et al. ANXA3-Rich Exosomes Derived from Tumor-Associated Macrophages Regulate Ferroptosis and Lymphatic Metastasis of Laryngeal Squamous Cell Carcinoma. Cancer Immunol Res 2024;12:614-30. [Crossref] [PubMed]
- Xu M, Hu X, Xiao Z, et al. Silencing KPNA2 Promotes Ferroptosis in Laryngeal Cancer by Activating the FoxO Signaling Pathway. Biochem Genet 2024; Epub ahead of print. [Crossref] [PubMed]
- Zhou N, Bao J. FerrDb: a manually curated resource for regulators and markers of ferroptosis and ferroptosis-disease associations. Database (Oxford) 2020;2020:baaa021. [Crossref] [PubMed]
- Wu Y, Zhang Y, Zheng X, et al. Circular RNA circCORO1C promotes laryngeal squamous cell carcinoma progression by modulating the let-7c-5p/PBX3 axis. Mol Cancer 2020;19:99. [Crossref] [PubMed]
- Ritchie ME, Phipson B, Wu D, et al. limma powers differential expression analyses for RNA-sequencing and microarray studies. Nucleic Acids Res 2015;43:e47. [Crossref] [PubMed]
- Oliveros JC, Venny. An interactive tool for comparing lists with Venn's diagrams. 2007-2015. Available online: https://bioinfogp.cnb.csic.es/tools/venny/index.html
- Zhou Y, Zhou B, Pache L, et al. Metascape provides a biologist-oriented resource for the analysis of systems-level datasets. Nat Commun 2019;10:1523. [Crossref] [PubMed]
- Barretina J, Caponigro G, Stransky N, et al. The Cancer Cell Line Encyclopedia enables predictive modelling of anticancer drug sensitivity. Nature 2012;483:603-7. [Crossref] [PubMed]
- Thul PJ, Lindskog C. The human protein atlas: A spatial map of the human proteome. Protein Sci 2018;27:233-44. [Crossref] [PubMed]
- Lu B, Chen XB, Ying MD, et al. The Role of Ferroptosis in Cancer Development and Treatment Response. Front Pharmacol 2018;8:992. [Crossref] [PubMed]
- Liang C, Zhang X, Yang M, et al. Recent Progress in Ferroptosis Inducers for Cancer Therapy. Adv Mater 2019;31:e1904197. [Crossref] [PubMed]
- Stockwell BR. Ferroptosis turns 10: Emerging mechanisms, physiological functions, and therapeutic applications. Cell 2022;185:2401-21. [Crossref] [PubMed]
- Yuan L, Li S, Chen Q, et al. EBV infection-induced GPX4 promotes chemoresistance and tumor progression in nasopharyngeal carcinoma. Cell Death Differ 2022;29:1513-27. [Crossref] [PubMed]
- Ye J, Jiang X, Dong Z, et al. Low-Concentration PTX And RSL3 Inhibits Tumor Cell Growth Synergistically By Inducing Ferroptosis In Mutant p53 Hypopharyngeal Squamous Carcinoma. Cancer Manag Res 2019;11:9783-92. [Crossref] [PubMed]
- Gu W, Kim M, Wang L, et al. Multi-omics Analysis of Ferroptosis Regulation Patterns and Characterization of Tumor Microenvironment in Patients with Oral Squamous Cell Carcinoma. Int J Biol Sci 2021;17:3476-92. [Crossref] [PubMed]
- Han F, Li W, Chen T, et al. Ferroptosis-related genes for predicting prognosis of patients with laryngeal squamous cell carcinoma. Eur Arch Otorhinolaryngol 2021;278:2919-25. [Crossref] [PubMed]
- Battelli MG, Polito L, Bortolotti M, et al. Xanthine oxidoreductase in cancer: more than a differentiation marker. Cancer Med 2016;5:546-57. [Crossref] [PubMed]
- Lin C, Hu R, Sun F, et al. Ferroptosis-based molecular prognostic model for adrenocortical carcinoma based on least absolute shrinkage and selection operator regression. J Clin Lab Anal 2022;36:e24465. [Crossref] [PubMed]
- Lopdell TJ, Tiplady K, Couldrey C, et al. Multiple QTL underlie milk phenotypes at the CSF2RB locus. Genet Sel Evol 2019;51:3. [Crossref] [PubMed]
- Yang WS. Regulation of ferroptotic cancer cell death by GPX4. Cell 2014;156:317-31. [Crossref] [PubMed]
- Qin K, Zheng Z, He Y, et al. High expression of neutrophil cytosolic factor 2 (NCF2) is associated with aggressive features and poor prognosis of esophageal squamous cell carcinoma. Int J Clin Exp Pathol 2020;13:3033-43. [PubMed]
- Yang S, Tang D, Zhao YC, et al. Potentially functional variants of ERAP1, PSMF1 and NCF2 in the MHC-I-related pathway predict non-small cell lung cancer survival. Cancer Immunol Immunother 2021;70:2819-33. [Crossref] [PubMed]
- Moszyńska A, Gebert M, Collawn JF, et al. SNPs in microRNA target sites and their potential role in human disease. Open Biol 2017;7:170019. [Crossref] [PubMed]
- Zhang X, Du L, Qiao Y, et al. Ferroptosis is governed by differential regulation of transcription in liver cancer. Redox Biol 2019;24:101211. [Crossref] [PubMed]
- Yousuf U, Sofi S, Makhdoomi A, et al. Identification and analysis of dysregulated fatty acid metabolism genes in breast cancer subtypes. Med Oncol 2022;39:256. [Crossref] [PubMed]
- Song Z, Li F, He C, et al. In-depth transcriptomic analyses of LncRNA and mRNA expression in the hippocampus of APP/PS1 mice by Danggui-Shaoyao-San. Aging (Albany NY) 2020;12:23945-59. [Crossref] [PubMed]
- Dixon SJ, Patel DN, Welsch M, et al. Pharmacological inhibition of cystine-glutamate exchange induces endoplasmic reticulum stress and ferroptosis. Elife 2014;3:e02523. [Crossref] [PubMed]
- Zhao R, Li Z, Huang Y, et al. A Novel Ferroptosis-Related Gene Signature for Prognosis Prediction in Ewing Sarcoma. Anal Cell Pathol (Amst) 2022;2022:6711629. [Crossref] [PubMed]
- Gao M, Monian P, Pan Q, et al. Ferroptosis is an autophagic cell death process. Cell Res 2016;26:1021-32. [Crossref] [PubMed]
- Alvarez SW, Sviderskiy VO, Terzi EM, et al. NFS1 undergoes positive selection in lung tumours and protects cells from ferroptosis. Nature 2017;551:639-43. [Crossref] [PubMed]
- Zhu G, Murshed A, Li H, et al. O-GlcNAcylation enhances sensitivity to RSL3-induced ferroptosis via the YAP/TFRC pathway in liver cancer. Cell Death Discov 2021;7:83. [Crossref] [PubMed]
- Cui P, Dai X, Liu R, et al. LncRNA LINC00888 upregulation predicts a worse survival of laryngeal cancer patients and accelerates the growth and mobility of laryngeal cancer cells through regulation of miR-378g/TFRC. J Biochem Mol Toxicol 2021;35:e22878. [Crossref] [PubMed]