Screening of postoperative adjuvant chemotherapy-related serum metabolic markers in breast cancer patients based on 1H NMR metabonomics
Highlight box
Key findings
• Metabolic biomarkers associated with the efficacy of adjuvant chemotherapy for breast cancer were identified using 1H nuclear magnetic resonance metabolomics.
What is known and what is new?
• There is no effective method to dynamically and non-invasively monitor the efficacy of chemotherapy.
• Metabolic alterations associated with the efficacy of adjuvant chemotherapy for breast cancer patients were revealed.
What is the implication, and what should change now?
• The study identified non-invasive biomarkers that can be used to monitor the efficacy of long-term chemotherapy for breast cancer, offering valuable insights for personalized treatment and efficacy assessment in breast cancer management.
• Further expand the sample collection to validate the clinical diagnostic value of chemotherapy-related metabolic markers.
Introduction
Breast cancer (BC) has the highest incidence and mortality among women (1). According to the 2020 report of International Agency for Research on Cancer (IARC), BC has surpassed lung cancer to become the tumor type with the highest incidence rate in the world, accounting for 11.7% of the 2.3 million new cases (2). The incidence of BC in China is relatively low compared with other countries, but the absolute mortality rate is high because of the high population (3).
BC is highly heterogeneous and each BC subtype [including luminal A, luminal B, human epidermal growth factor receptor 2 (HER2) and triple-negative] is characterized by different clinical response and prognosis due to the unique molecular characteristics of the subtypes (4). Adjuvant chemotherapy is a commonly used treatment method after surgery for all molecular subtypes of BC, as it can effectively reduce the risk of micrometastasis and improve the survival rate of patients (5,6). Findings from a retrospective cohort study on the impact of chemotherapy on the prognosis of elderly BC showed that patients receiving chemotherapy had better overall survival (OS) and breast cancer specific survival (BCSS) prognoses. Subjects with HER2+ or multiple lymph node metastases exhibited a better response to chemotherapy (7). Combination of hormone therapy and chemotherapy significantly reduces the risk of hormone receptor expression in patients with recurrent BC (8). In addition, a combination of trastuzumab and chemotherapy significantly improves the OS of metastatic BC patients with over-expressed HER2 (9). Triple negative breast cancer (TNBC) is a BC characterized by negative expression of estrogen receptor (ER), progesterone receptor (PR) and HER2 receptors, which is highly invasive and has a poor prognosis (10). Chemotherapy is the main treatment method for TNBC. Therefore, exploring the patient’s responses to chemotherapy and evaluating drug efficacy are crucial for determining the choice of chemotherapy regimens.
Chemotherapy efficacy is generally evaluated based on a 5-year survival period. Some biochemical indicators, such as carcinoembryonic antigen (CEA) and cancer antigen 15-3 (CA15-3), are often used as auxiliary evaluation indicators. However, due to the low sensitivity of those indicators, its clinical application is limited (11). Currently, there is no effective method to dynamically and noninvasively monitor the efficacy of chemotherapy.
Metabonomics, as a new type of omics after genetics, transcriptomics and proteomics, is a field involved in research on cancer phenotype (12). Metabonomics involves identification of tumor markers in biological fluids through high-throughput, non-invasive analytical technologies to explore the role of metabolic changes on the pathogenesis of cancer. Yu et al. conducted a study to evaluate the metabolites in the urine of chemotherapy patients through capillary electrophoresis mass spectrometry (CE-MS), and the results showed that the levels of glycine, cysteine, histidine, cystine, and tryptophan in chemotherapy-sensitive patients were significantly reduced compared with subjects who had low response to chemotherapy (13). Giskeødegård et al. studied the relationship between metabolite profiles of advanced BC tissues and the 5-year survival rates of patients. The findings showed significant metabolic differences in tumor tissue between surviving and non-surviving cancer patients. High levels of lactate and glycine are associated with poor prognosis in ER-positive BC patients (14). Small molecular substances in tissues or body fluids have been used to construct individual unique fingerprints, identify healthy controls and cancer groups, and evaluate the response of diseases to treatment (15-17).
In the present study, nuclear magnetic resonance (NMR)-based metabonomics combined with multivariate analysis was used to evaluate the serum metabolic profiles of postoperative BC patients before and after chemotherapy. The aim of the study was to identify the biomarkers for predicting the efficacy of chemotherapy and verify the predictive value of the metabolic markers. The results of the study provide a potential, long-term dynamic monitoring index for evaluation of chemotherapy efficacy in BC subjects. We present this article in accordance with the STARD reporting checklist (available at https://tcr.amegroups.com/article/view/10.21037/tcr-23-2352/rc).
Methods
Participants
A total of 51 healthy controls and 61 BC patients (13 of the 61 BC patients were luminal A; 35 were luminal B; 8 were HER2+ and 58 were TNBC) received surgical treatment participated in our study. Of the 61 postoperative patients, 46 patients received postoperative adjuvant chemotherapy and had good curative effects clinically evaluated by 5-year survival. The inclusion criteria were as follows: all patients were pathologically diagnosed with BC; all patients received regular chemotherapy after surgery. Thirteen patients received letrozole-based therapy, seven received monoclonal antibody-based therapy, and the remaining patients received anthracycline-based and taxane-based therapy. Clinical data of all patients were complete and corresponding immunohistochemical examinations were performed. All the patients had no recurrence within 5 years after chemotherapy. Sixty-one serum samples were collected from 61 patients (before chemotherapy), and 46 serum samples were collected at the end of the third postoperative chemotherapy cycle (after chemotherapy). The BC patient samples were obtained from the breast surgery cases of the First Affiliated Hospital of Guangdong Pharmaceutical University during 2018–2022. Fifty-one participants from the healthy control group did not have cancer or metabolic diseases and underwent regular routine check-ups. The study was approved by the Medical Ethics Committee of the First Affiliated Hospital of Guangdong Pharmaceutical University (2020, No. 29) and informed consent was obtained from all individual participants. The study was conducted in accordance with the Declaration of Helsinki (as revised in 2013). Tumor node metastasis (TNM) of tumors was statistically based on the 8th edition of the American Joint Committee on Cancer (AJCC) American Cancer Society staging. The details of the patients are shown in Table 1.
Table 1
Characteristics | Control (n=51) | BC patients before chemotherapy (n=61) | BC patients after chemotherapy (n=46) |
---|---|---|---|
Age (years) | 41.02±1.65 | 55.54±1.61** | 51.37±1.65 |
Menopausal status | |||
Post | 10 (19.61) | 38 (62.30)** | 24 (52.17) |
Pre | 41 (80.39) | 23 (37.70) | 22 (47.83) |
Tumor size | |||
Tis | 7 (11.48) | ||
T1 | 14 (22.95) | 14 (30.43) | |
T2 | 32 (52.46) | 24 (52.17) | |
T3 | 2 (3.27) | 2 (4.35) | |
T4 | 6 (9.84) | 6 (13.04) | |
Node status | |||
Node negative | 38 (62.3) | 26 (56.52) | |
Node positive | 23 (37.7) | 20 (43.48) | |
Clinical stage | |||
0 | 7 (11.48) | ||
I | 8 (13.11) | 8 (17.39) | |
II | 32 (52.46) | 30 (65.22) | |
III | 8 (13.11) | 8 (17.39) | |
IV | 6 (9.84) | ||
Molecular type | |||
Luminal A | 13 (21.31) | 7 (15.22) | |
Luminal B | 35 (57.38) | 28 (60.87) | |
HER2-positive | 8 (13.11) | 7 (15.22) | |
Triple-negative | 5 (8.20) | 4 (8.70) | |
CEA (µg/L) | 1.46±0.11 | 4.45±1.12* | 1.93±0.14# |
CA15-3 (U/mL) | 8.59±0.51 | 17.11±1.56*** | 12.95±0.89# |
The values were described with mean ± SEM or frequency (%). P value by Student’s t-test and Chi-squared test (control vs. before chemotherapy: *, P<0.05; **, P<0.01; ***, P<0.001; after vs. before chemotherapy: #, P<0.05). BC, breast cancer; Tis, tumor in situ; HER2, human epidermal growth factor receptor 2; CEA, carcinoembryonic antigen; CA15-3, cancer antigen 15-3; SEM, standard error of mean.
Samples collection and storage
All patients fasted for 12 hours before sample collection to avoid dietary influence. Fasting peripheral blood was collected in 3 mL of anticoagulant-free vacuum blood collection tubes, left for 30 minutes, centrifuged at 1,500 rpm at 4 ℃ for 10 minutes to obtain the supernatant, and then stored at −80 ℃ until batch NMR analysis was performed.
Serum preparation and 1H NMR spectroscopy
Serum samples stored at −80 ℃ were thawed and centrifuged at 12,000 rpm for 10 minutes at 4 ℃. 300 µL of supernatant was added to a 5 mm NMR tube, then 150 µL of PBS (0.2 mol/L Na2HPO4/NaH2PO4, pH=7.4) and 100 µL of D2O were added for dilution. The sample was stored at 4 ℃ before testing.
NMR metabonomics analysis was performed on a Bruker AVANCE III 500 MHz superconducting NMR spectrometer (Bruker, Inc., Switzerland). Spectra were collected using a standard pulse sequence of Carr-Purcell-Meiboom-Gill [CPMG, recycle delay-90-(τ-180-τ)n-acquisition]. The experimental temperature was set to 298 K and the total spin-echo time was 100 ms (2nτ). A spectral width of 10 kHz was used to acquire 128 data points for each sample. Topspin 4.0 (Bruker BioSpin, Germany) software was used for manual phase and baseline correction of all the NMR samples. The lactate peak was scaled at a chemical shift of δ1.33. In order to facilitate subsequent analysis, the AMIX software (V4.0.2, Bruker BioSpin) was used to divide the spectra in the range δ0.5–9.0 (excluding δ4.7–5.2 to remove the effect of residual water peak) into integration intervals in terms of 0.004 ppm.
Multivariate and ROC analysis
Screening of different metabolites between groups was performed by using supervised orthogonal partial least squares discriminant analysis (OPLS-DA). The integrated data of metabolites were imported into the MetaboAnalyst 5.0 (https://www.metaboanalyst.ca/) for multivariate analysis. All metabolites were tested for significance using Student’s t-test in SPSS 22.0 software. The associated metabolic pathways were mapped manually based on the Kyoto Encyclopedia of Genes and Genomes (KEGG) pathways through CorelDRAW (Corel Inc., Canada) software. The receiver operating characteristic (ROC) curves was generated by using GraphPadPrism (Version 8.0.1, China), and the corresponding area under the curve (AUC), specificity and sensitivity were calculated.
Statistical analysis
All calculations were based on GraphPad Prism (Version 8.0.1, China) software and SPSS (IBM Corp., NY, USA) software.
Results
A total of 112 subjects were enrolled to this study, including 51 healthy controls and 61 BC patients. Out of the 61 BC patients, 46 patients received postoperative chemotherapy. Menopause status was significantly different between the control subjects and BC patients. The levels of CEA and CA15-3 were up-regulated in BC patients before chemotherapy compared with the healthy controls, but they significantly reduced after chemotherapy, indicating the efficiency of adjuvant chemotherapy for BC patients. Details on tumor size, lymph node status, clinical stage and molecular subtypes of BC patients are presented in Table 1.
Serum 1H NMR spectra of healthy controls and BC patients before and after chemotherapy were acquired (Figure 1). The metabolites in the three groups were concentrated in the δ0.5–9.0 peak region. A total of 23 endogenous metabolites were identified according to Human Metabolome Database (http://hmdb.ca/) and previous studies (18,19).
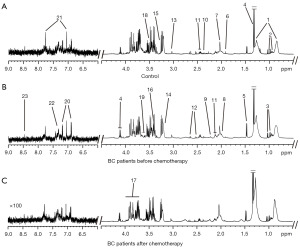
OPLS-DA models were constructed to identify the metabolic changes in BC patients and to screen the biomarkers caused by chemotherapy (Figure 2A,2B). Cross-validation (CV) was performed to evaluate the validity of the OPLS-DA models (Figure 2C,2D). The differential metabolites were obtained based on variable important in projection (VIP) values (Figure 2E,2F). Based on the VIP value (VIP >1), it was found that citrate, GPC/PC, formate, pyruvate, acetate, glycine, creatine, glutamine, valine, 1-methylhistidine, lactate and phenylalanine had great contributions to the classification of healthy controls and BC patients before chemotherapy. Compared with BC patients before chemotherapy, chemotherapy caused significant changes in the metabolites of formate, alanine, acetate, 1-methylhistidine, pyruvate, glycoprotein and lactate (VIP >1).
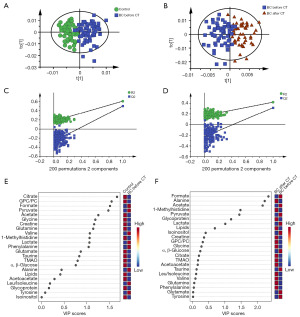
Sequentially, an unpaired Student’s t-test was conducted on the metabolites with VIP >1 (Figure 3). Among the 12 metabolites mentioned above, 11 showed statistical significance in BC patients compared with the healthy control group. The serum levels of acetate, glutamine, glycine, citrate, creatine, GPC/PC and 1-methylhistidine were significantly reduced, whereas valine, lactate, pyruvate and formate were significantly up-regulated. It was found that the serum metabolic characteristics of BC patients showed significant differences after chemotherapy. The levels of lactate, acetate, pyruvate, and formate were down-regulated, whereas 1-methylhistidine was up-regulated by chemotherapy. Chemotherapy restored lactate and pyruvate to the levels of the healthy group. It was noted that acetate reduced in BC patients before chemotherapy, but it further decreased after chemotherapy, indicating that chemotherapy did not regulate the metabolic disorder of acetate in BC. Therefore, it was concluded that chemotherapy has a reverse regulatory effect on the four metabolites of lactate, pyruvate, 1-methylhistidine and formate, which were considered potential biomarkers associated with BC chemotherapy.
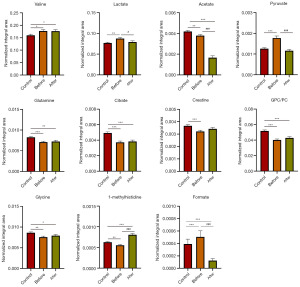
Heatmaps were generated to show the metabolic differences between the controls and BC patients before chemotherapy (Figure 4A), as well as the metabolic difference between BC patients before and after chemotherapy (Figure 4B). Warm color and cold color represented higher and lower serum metabolite levels, respectively. The metabolic changes and the chemotherapy-related biomarkers were visually observed, which was consistent with the findings in Figure 3.
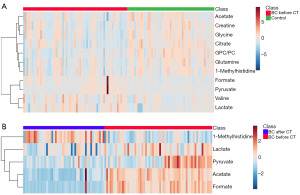
ROC curves were generated to show the predictive abilities of the identified metabolites and their combinations for BC diagnosis (Figure 5) and chemotherapy efficacy (Figure 6). In this study, nine metabolites had good predictive significance for BC (AUC value >0.66), including pyruvate, 1-methylhistidine, lactate, glutamine, glycine, creatine, formate, citrate and GPC/PC. ROC analysis showed that the AUC value of these nine metabolites was 0.967 (Figure 5J), which was similar to the predictive ability of the combination of these nine metabolites and age (Figure 5K). The values of the four chemotherapy-related metabolites in predicting the chemotherapy efficacy were also evaluated. It was showed that a combination of the four metabolites of lactate, pyruvate, 1-methylhistidine and formate had a good predictive ability for chemotherapy with AUC of 0.958, sensitivity of 98.36% and specificity of 91.30% (Figure 6E).
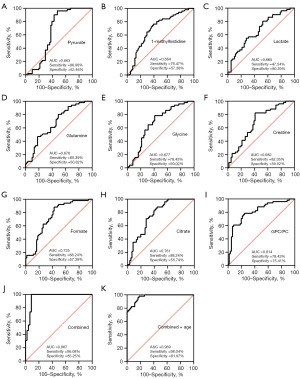
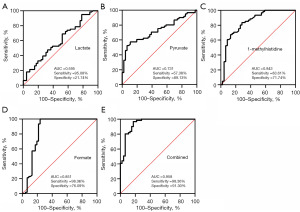
The correlations between chemotherapy-related metabolic markers (lactate, pyruvate, 1-methylhistidine and formate) and clinical indicators (lymph node metastasis, clinical stage and tumor size) were also evaluated (Table S1). By comparing the changes of these four chemotherapy-related metabolic markers in patients with different clinical indicators, we found that there was no significant difference in the concentration of these four metabolites between the lymph node metastasis negative and positive groups. Similarly, tumor size and clinical staging did not significantly affect these four metabolites. This result indicates that the metabolic changes caused by chemotherapy are mainly related to the therapeutic effect of chemotherapy, and there is no significant correlation with these clinical indicators. Therefore, the chemotherapy related metabolic markers screened in this experiment can be used to evaluate the chemotherapy efficacy of patients with different clinical indicators. In addition, Figure 7 shows the metabolic pathways involved in the aforementioned metabolic changes. It was found that choline metabolism, glycolysis, serine-glycine metabolism, TCA cycle and glutamine changed in the serum of BC patients. Chemotherapy significantly regulated the metabolism in glycolysis, formate, and 1-methylhistidine.
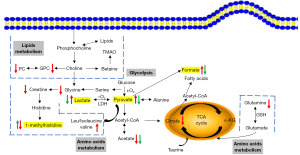
Discussion
Adjuvant chemotherapy is currently one of the routine treatments for postoperative BC to prevent micrometastasis and reduce tumor recurrence (20). The clinical efficacy of chemotherapy is usually evaluated by a 5-year survival period. Currently, there is no effective method for long-term, dynamic and non-invasive monitoring of chemotherapy efficacy. Metabonomics is an omics method for cancer prognosis and efficacy evaluation based on detecting the stable and quantifiable metabolites in body fluids (21). Serum metabolic markers associated with the efficacy of chemotherapy were identified through a comparison of the serum metabolic characteristics between the healthy group and BC patients before and after chemotherapy.
The results showed that a combination of the nine metabolites, namely pyruvate, 1-methylhistidine, lactate, glutamine, citrate, formate, glycine, creatine and GPC/PC, showed excellent performance in distinguishing healthy controls and BC patients, with sensitivity of 96.08% and specificity of 85.25%. Multivariate and statistical analyses showed that chemotherapy reversely regulated the metabolism of lactate, pyruvate, 1-methylhistidine and formate, and restored the levels of lactate and pyruvate to those of control subjects. ROC analysis showed that the combination of these four metabolites had a good predictive effect on chemotherapy efficacy (sensitivity of 98.36% and specificity of 91.30%). It was found that the chemotherapy-related metabolic markers are not correlated with clinical indicators, which revealed that they can be used to evaluate the chemotherapy efficacy of patients with different clinical indicators.
Energy metabolism plays an important role in maintaining the growth and proliferation of cancer cells (22). Cancer cells exhibit upregulation of hypoxia inducible factor-1α (HIF-1α) under hypoxic conditions, converting aerobic phosphorylation into a glycolysis pathway (23). Circulating glucose undergoes glycolysis to release lactate and adenosine triphosphate (ATP) to supplement the energy required for the synthesis of macromolecular substances such as lipids and nucleic acids (24). The serum level of lactate in BC patients was up-regulated compared with the healthy controls. Lactate is considered as a tumor marker (25). Acidification of the tumor microenvironment helps to promote tumor progression, invasion and migration (26,27). After chemotherapy, it was found that serum lactate level decreased and was regulated back to the normal level. Pyruvate is the final product of glycolysis and the main source of carbon flux for tricarboxylic acid (TCA) cycle. Jobard et al. observed high level of pyruvate in metastatic BC compared with early BC (28). In this study, serum level of pyruvate in BC patients after chemotherapy was down-regulated and it had no significant difference compared with the level in controls. These findings indicated that chemotherapy regulates glycolytic metabolic pathways.
Citrate is synthesized through carboxylation of ketoglutaric acid (α-KG) and subsequently cleaved by citrate lyase to form acetyl-CoA, which is involved in protein acetylation, lipid synthesis and TCA cycle (29). The result showed a decrease in citrate level in BC patients compared with the level in controls. Citrate is involved in various physiological processes, such as insulin secretion (30), immune and inflammatory reactions, and antibacterial defense (31). Therefore, dysregulation of citrate metabolism is associated with cancer and inflammation. Although tumors were surgically removed in the BC patients enrolled in this study, dysregulated metabolism of citrate indicated that some metabolic characteristics of tumors still exist. Notably, there was no significant change in citrate level in BC patients before and after chemotherapy, indicating that chemotherapy had no significant regulatory effect on TCA metabolic pathway.
The levels of several amino acids, including glutamine, glycine, creatine and 1-methylhistidine decreased in BC patients compared with healthy controls. These four amino acids are nonessential and can be synthesized endogenously. A recent study reported the low serum levels of amino acids in BC patients (32). Glutamine, a nitrogen and carbon source for the synthesis of lipids and amino acids, can be converted into glutamate and further converted into α-ketoglutarate used for ATP synthesis (33). Healthy cells synthesize glutamine through glutaminase (GLS), whereas tumor cells cannot meet the energy requirements for rapid proliferation through synthesis, which leads to the consumption of serum glutamine (34).
Glycine provides carbon units for single-carbon metabolism used for synthesis of macromolecules such as lipids and nucleic acids, and provides energy for the growth and proliferation of cancer cells (35). Glycine is also converted to creatine through various pathways. Creatine is mainly present in cells in the phosphorylated form and has a high antioxidant capacity. One study has shown that creatine supplementation therapy alleviates inflammatory diseases, metabolic disorders and malnutrition (36). The reduction of creatine reveals metabolic dysregulation in BC patients, which may be a serum metabolic marker in BC patients. However, in the serum of BC patients after chemotherapy, we found a reduction in glycine and creatine, but the difference was not significant.
The serum level of 1-methylhistidine was significantly reduced in BC patients. 1-methylhistidine is a metabolite of histidine metabolism. Methylated histidine is involved in multiple processes by regulating gene expression and enzyme activity (37). Administration of histidine to liver injury model mice reduced the level of inflammatory factors in liver (38). In the present study, chemotherapy increased the serum level of 1-methylhistidine compared with the level before chemotherapy, which showed that chemotherapy possibly improved inflammation.
GPC/PC is the main component of cell biomembrane, and they play key roles in cell transmission and cycle stability (39). Compared with controls, the change of GPC/PC in BC patients indicates dysregulation of lipid metabolism. Previous findings have shown that a continuous decrease in choline stimulates nuclear factor kappa B (NF-κB) to induce cell survival, leading to inflammation and tumorigenesis (40). Low levels of choline-containing metabolites have been detected in the serum of lung cancer patients (41). In the current study, the serum choline metabolism remained dysregulated in BC patients after solid tumor resection, whereas chemotherapy had no significant regulatory effect on the disorder of choline metabolism pathway.
The oxidation and synthesis of fatty acids in mitochondria provide a large amount of ATP for cell growth. Formate is a metabolite of short-chain fatty acids and is obtained from the oxidation of the third carbon of serine in the mitochondria. In addition, formate can be used for synthesis of serine through single-carbon metabolism in the cytoplasm (42). The present results showed that the serum formate level in BC patients was higher than those after chemotherapy. Meiser et al. observed higher levels of formate in mice with intestinal adenoma and BC compared with normal mice, which is consistent with the findings of this study, indicating that high levels of formate is a potential marker of oxidative cancer. A study has also shown that reducing formate levels can effectively prevent the invasion of cancer cells (43). The decrease of serum formate level in BC patients after chemotherapy suggests that chemotherapy may affect the oxidation energy supply of fatty acids and induce the apoptosis of residual tumor cells.
Analysis of the relationship between the four chemotherapy-related metabolites and the clinical indicators demonstrated that the levels of the four metabolites were relatively stable in BC patients with different clinical indicators. This implies that the metabolic changes caused by chemotherapy mainly reflect the effects of chemotherapy drugs and are not related to the clinical indicators. However, there are some limitations to this paper, such as the small sample size we included. We will further expand the sample collection to validate the clinical diagnostic value of chemotherapy-related metabolic markers.
Conclusions
A metabonomics method based on 1H NMR was used to study the serum metabolic profiles of healthy control group and BC patients before and after chemotherapy, and screen four metabolic markers having good predictive effects on chemotherapy. It was found that the chemotherapy-related metabolites of lactate, pyruvate, 1-methylhistidine and formate were not correlated with the clinical indicators. However, they were associated with molecular types, and more sensitive to chemotherapy in Luminal B and HER2+ types. Therefore, this study identified non-invasive biomarkers that can be used to monitor the long-term chemotherapy efficacy of BC.
Acknowledgments
The authors would like to thank the First Affiliated Hospital of Guangdong Pharmaceutical University for providing serum samples.
Funding: This study was supported by
Footnote
Reporting Checklist: The authors have completed the STARD reporting checklist. Available at https://tcr.amegroups.com/article/view/10.21037/tcr-23-2352/rc
Data Sharing Statement: Available at https://tcr.amegroups.com/article/view/10.21037/tcr-23-2352/dss
Peer Review File: Available at https://tcr.amegroups.com/article/view/10.21037/tcr-23-2352/prf
Conflicts of Interest: All authors have completed the ICMJE uniform disclosure form (available at https://tcr.amegroups.com/article/view/10.21037/tcr-23-2352/coif). The authors have no conflicts of interest to declare.
Ethical Statement: The authors are accountable for all aspects of the work in ensuring that questions related to the accuracy or integrity of any part of the work are appropriately investigated and resolved. The study was conducted in accordance with the Declaration of Helsinki (as revised in 2013). The study was approved by the Medical Ethics Committee of the First Affiliated Hospital of Guangdong Pharmaceutical University (2020, No. 29) and informed consent was obtained from all individual participants.
Open Access Statement: This is an Open Access article distributed in accordance with the Creative Commons Attribution-NonCommercial-NoDerivs 4.0 International License (CC BY-NC-ND 4.0), which permits the non-commercial replication and distribution of the article with the strict proviso that no changes or edits are made and the original work is properly cited (including links to both the formal publication through the relevant DOI and the license). See: https://creativecommons.org/licenses/by-nc-nd/4.0/.
References
- Bos PD, Zhang XH, Nadal C, et al. Genes that mediate breast cancer metastasis to the brain. Nature 2009;459:1005-9. [Crossref] [PubMed]
- Sung H, Ferlay J, Siegel RL, et al. Global Cancer Statistics 2020: GLOBOCAN Estimates of Incidence and Mortality Worldwide for 36 Cancers in 185 Countries. CA Cancer J Clin 2021;71:209-49. [Crossref] [PubMed]
- Li T, Mello-Thoms C, Brennan PC. Descriptive epidemiology of breast cancer in China: incidence, mortality, survival and prevalence. Breast Cancer Res Treat 2016;159:395-406. [Crossref] [PubMed]
- Díaz-Beltrán L, González-Olmedo C, Luque-Caro N, et al. Human Plasma Metabolomics for Biomarker Discovery: Targeting the Molecular Subtypes in Breast Cancer. Cancers (Basel) 2021;13:147. [Crossref] [PubMed]
- Waks AG, Winer EP. Breast Cancer Treatment: A Review. JAMA 2019;321:288-300. [Crossref] [PubMed]
- Wang Y, Yin Q, Yu Q, et al. A retrospective study of breast cancer subtypes: the risk of relapse and the relations with treatments. Breast Cancer Res Treat 2011;130:489-98. [Crossref] [PubMed]
- Wu Y, Qi Y, Yang J, et al. Effect of adjuvant chemotherapy on the survival outcomes of elderly breast cancer: A retrospective cohort study based on SEER database. J Evid Based Med 2022;15:354-64. [Crossref] [PubMed]
- Anampa J, Makower D, Sparano JA. Progress in adjuvant chemotherapy for breast cancer: an overview. BMC Med 2015;13:195. [Crossref] [PubMed]
- Slamon DJ, Leyland-Jones B, Shak S, et al. Use of chemotherapy plus a monoclonal antibody against HER2 for metastatic breast cancer that overexpresses HER2. N Engl J Med 2001;344:783-92. [Crossref] [PubMed]
- Yin L, Duan JJ, Bian XW, et al. Triple-negative breast cancer molecular subtyping and treatment progress. Breast Cancer Res 2020;22:61. [Crossref] [PubMed]
- Di Gioia D, Heinemann V, Nagel D, et al. Kinetics of CEA and CA15-3 correlate with treatment response in patients undergoing chemotherapy for metastatic breast cancer (MBC). Tumour Biol 2011;32:777-85. [Crossref] [PubMed]
- Holmes E, Wilson ID, Nicholson JK. Metabolic phenotyping in health and disease. Cell 2008;134:714-7. [Crossref] [PubMed]
- Yu L, Jiang C, Huang S, et al. Analysis of urinary metabolites for breast cancer patients receiving chemotherapy by CE-MS coupled with on-line concentration. Clin Biochem 2013;46:1065-73. [Crossref] [PubMed]
- Giskeødegård GF, Lundgren S, Sitter B, et al. Lactate and glycine-potential MR biomarkers of prognosis in estrogen receptor-positive breast cancers. NMR Biomed 2012;25:1271-9. [Crossref] [PubMed]
- Park J, Shin Y, Kim TH, et al. Plasma metabolites as possible biomarkers for diagnosis of breast cancer. PLoS One 2019;14:e0225129. [Crossref] [PubMed]
- Slupsky CM, Steed H, Wells TH, et al. Urine metabolite analysis offers potential early diagnosis of ovarian and breast cancers. Clin Cancer Res 2010;16:5835-41. [Crossref] [PubMed]
- Catchpole GS, Beckmann M, Enot DP, et al. Hierarchical metabolomics demonstrates substantial compositional similarity between genetically modified and conventional potato crops. Proc Natl Acad Sci U S A 2005;102:14458-62. [Crossref] [PubMed]
- Chen W, Li Q, Hou R, et al. An integrated metabonomics study to reveal the inhibitory effect and metabolism regulation of taurine on breast cancer. J Pharm Biomed Anal 2022;214:114711. [Crossref] [PubMed]
- Hu JM, Sun HT. Serum proton NMR metabolomics analysis of human lung cancer following microwave ablation. Radiat Oncol 2018;13:40. [Crossref] [PubMed]
- Li J, Zhang BN, Fan JH, et al. A nation-wide multicenter 10-year (1999-2008) retrospective clinical epidemiological study of female breast cancer in China. BMC Cancer 2011;11:364. [Crossref] [PubMed]
- Spratlin JL, Serkova NJ, Eckhardt SG. Clinical applications of metabolomics in oncology: a review. Clin Cancer Res 2009;15:431-40. [Crossref] [PubMed]
- Vander Heiden MG, DeBerardinis RJ. Understanding the Intersections between Metabolism and Cancer Biology. Cell 2017;168:657-69. [Crossref] [PubMed]
- Semenza GL. Hypoxia-inducible factors: mediators of cancer progression and targets for cancer therapy. Trends Pharmacol Sci 2012;33:207-14. [Crossref] [PubMed]
- Ferreira LM. Cancer metabolism: the Warburg effect today. Exp Mol Pathol 2010;89:372-80. [Crossref] [PubMed]
- de la Cruz-López KG, Castro-Muñoz LJ, Reyes-Hernández DO, et al. Lactate in the Regulation of Tumor Microenvironment and Therapeutic Approaches. Front Oncol 2019;9:1143. [Crossref] [PubMed]
- Walenta S, Wetterling M, Lehrke M, et al. High lactate levels predict likelihood of metastases, tumor recurrence, and restricted patient survival in human cervical cancers. Cancer Res 2000;60:916-21. [PubMed]
- Yokota H, Guo J, Matoba M, et al. Lactate, choline, and creatine levels measured by vitro 1H-MRS as prognostic parameters in patients with non-small-cell lung cancer. J Magn Reson Imaging 2007;25:992-9. [Crossref] [PubMed]
- Jobard E, Pontoizeau C, Blaise BJ, et al. A serum nuclear magnetic resonance-based metabolomic signature of advanced metastatic human breast cancer. Cancer Lett 2014;343:33-41. [Crossref] [PubMed]
- Icard P, Coquerel A, Wu Z, et al. Understanding the Central Role of Citrate in the Metabolism of Cancer Cells and Tumors: An Update. Int J Mol Sci 2021;22:6587. [Crossref] [PubMed]
- Iacobazzi V, Infantino V. Citrate--new functions for an old metabolite. Biol Chem 2014;395:387-99. [Crossref] [PubMed]
- O'Neill LA, Pearce EJ. Immunometabolism governs dendritic cell and macrophage function. J Exp Med 2016;213:15-23. [Crossref] [PubMed]
- Shen J, Yan L, Liu S, et al. Plasma metabolomic profiles in breast cancer patients and healthy controls: by race and tumor receptor subtypes. Transl Oncol 2013;6:757-65. [Crossref] [PubMed]
- Choi YK, Park KG. Targeting Glutamine Metabolism for Cancer Treatment. Biomol Ther (Seoul) 2018;26:19-28. [Crossref] [PubMed]
- Lukey MJ, Katt WP, Cerione RA. Targeting amino acid metabolism for cancer therapy. Drug Discov Today 2017;22:796-804. [Crossref] [PubMed]
- Amelio I, Cutruzzolá F, Antonov A, et al. Serine and glycine metabolism in cancer. Trends Biochem Sci 2014;39:191-8. [Crossref] [PubMed]
- Gualano B, Artioli GG, Poortmans JR, et al. Exploring the therapeutic role of creatine supplementation. Amino Acids 2010;38:31-44. [Crossref] [PubMed]
- Casadei-Gardini A, Del Coco L, Marisi G, et al. (1)H-NMR Based Serum Metabolomics Highlights Different Specific Biomarkers between Early and Advanced Hepatocellular Carcinoma Stages. Cancers (Basel) 2020;12:241. [Crossref] [PubMed]
- Yan SL, Wu ST, Yin MC, et al. Protective effects from carnosine and histidine on acetaminophen-induced liver injury. J Food Sci 2009;74:H259-65. [Crossref] [PubMed]
- Zong L, Pi Z, Liu S, et al. Metabolomics analysis of multidrug-resistant breast cancer cells in vitro using methyl-tert-butyl ether method. RSC Adv 2018;8:15831-41. [Crossref] [PubMed]
- Holmes-McNary MQ, Baldwin AS Jr, Zeisel SH. Opposing regulation of choline deficiency-induced apoptosis by p53 and nuclear factor kappaB. J Biol Chem 2001;276:41197-204. [Crossref] [PubMed]
- Zhang X, Zhu X, Wang C, et al. Non-targeted and targeted metabolomics approaches to diagnosing lung cancer and predicting patient prognosis. Oncotarget 2016;7:63437-48. [Crossref] [PubMed]
- Meiser J, Tumanov S, Maddocks O, et al. Serine one-carbon catabolism with formate overflow. Sci Adv 2016;2:e1601273. [Crossref] [PubMed]
- Meiser J, Schuster A, Pietzke M, et al. Increased formate overflow is a hallmark of oxidative cancer. Nat Commun 2018;9:1368. [Crossref] [PubMed]