Construction of a new prognostic model for colorectal cancer based on bulk RNA-seq combined with The Cancer Genome Atlas data
Highlight box
Key findings
• This risk score (RS) has potential for the prognostic prediction of colorectal cancer (CRC).
What is known and what is new?
• CRC is a common malignant tumor of digestive tract, which seriously endangers human life and health. CRC is now the fourth most common malignancy worldwide and the fifth leading cause of cancer death.
• The expression levels of RETNLB, CLCA4, UGT2A3, SULT1B1, CCL24, BMP5 and ATOH1 can predict the risk of CRC.
What is the implication, and what should change now?
• Through bioinformatics, we hope to construct RS to predict the occurrence of CRC.
Introduction
According to the 2022 report from the National Cancer Center (NCC), colorectal cancer (CRC) ranks second in China in terms of morbidity and fourth in terms of mortality among all cancer types, with CRC patients comprising approximately 10% of the total cancer population (1). CRC is a common malignant tumor in the gastrointestinal tract. Initially, it often presents with mild symptoms. However, as the disease progresses, individuals may experience changes in bowel habits, bloody stools, diarrhea, alternating diarrhea and constipation, and localized abdominal pain. In the later stages, systemic symptoms such as anemia and weight loss may occur. CRC is primarily a genetic disorder that develops from precursor colonic lesions/polyps through various tumorigenic pathways (2), and its occurrence is closely related to factors such as smoking (3), high salt intake (4,5), lack of physical exercise (6,7), obesity (8), and family history of the disease. As reported, although the incidence of CRC has slightly decreased in individuals aged over 50 years old, there is an upward trend in the incidence among those under 50, indicating a trend towards younger onset (9). At present, surgical resection, chemotherapy, and drug-assisted therapy are commonly used treatment methods for CRC in clinical practice. Radical surgical resection can reduce the mortality rate for early CRC patients (10,11), and adjuvant systemic therapy after surgical resection can reduce the risk of recurrence and significantly improve the overall survival rate of them (12). Early screening can effectively curb the development of CRC, but a large number of patients in China are still diagnosed with advanced CRC. Immune checkpoint blockade (ICB) shows promise in treating advanced CRC (13), but drug resistance remains a challenge (14), contributing to a high mortality rate. Therefore, developing new immunotherapy targets to improve the survival rate of CRC patients is currently the top priority.
Over the past 5 years, there have been 109,684 publications in the field of bioinformatics analysis, with 35,202 specifically focused on cancer research. With the development of technology, various tools and techniques have emerged to help researchers in exploring the underlying factors contributing to disease occurrence, such as single-cell sequencing, gene chips, etc. At present, bioinformatics analysis is predominantly applied for identifying disease-related key genes (15), constructing risk models related to care indicators (16), and discovering potential drug targets (17). Moreover, researchers worldwide also upload relevant sequencing data to public databases, such as Gene Expression Omnibus (GEO), The Cancer Genome Atlas (TCGA), ArrayExpress databases, etc., which facilitates data sharing and further propels the progress of bioinformatics analysis. At present, the integration of data from multiple datasets or databases is a common approach in bioinformatics analysis to extract disease-related data (18-20).
In this study, CRC-related single-cell, transcription and clinical data were downloaded from publicly available databases, namely GEO and TCGA. Through a series of analyses including differential analysis, CIBERSORTx analysis, least absolute shrinkage and selection operator (LASSO) analysis, Cox univariate analysis and survival analysis, we identified key genes that could serve as prognostic indicators for the prognosis of CRC. Risk score (RS) was established to develop a prognostic risk model. The model was also validated in the validation set, and the correlation between RS and immune-related clinical information was analyzed. Our aim in conducting this analysis is to construct a new prognostic risk model that can effectively evaluate the prognosis of CRC patients, so as to provide early interventional treatment for high-risk populations and ultimately improve the survival rate of CRC patients. We present this article in accordance with the TRIPOD reporting checklist (available at https://tcr.amegroups.com/article/view/10.21037/tcr-23-2281/rc).
Methods
Data acquisition and processing
The CRC-related single-cell RNA sequencing (scRNA-seq) data were obtained from the GSE201348 dataset in the GEO database (https://www.ncbi.nlm.nih.gov/geo/). Two cancer samples (GSM6061645 and GSM6061686) and two normal samples (GSM6061709 and GSM6061713) were selected for subsequent analysis. The “TCGAbiolinks” R package (v 2.25.3) was used to download and process the mRNA data of colon adenocarcinoma (COAD) and rectum adenocarcinoma (READ) from the TCGA database, and consequently the corresponding transcripts per million (TPM) data were obtained and standardized (622 cancer samples, 51 normal samples). Additionally, the GSE12945, GSE29623, and GSE38832 datasets were downloaded from the GEO database using the “GEOquery” R package for validation. Gene IDs in these datasets were converted to gene symbols using GPL96 annotation, and duplicated genes were averaged. The study was conducted in accordance with the Declaration of Helsinki (as revised in 2013).
Single-cell analysis
We performed single-cell analysis on four samples selected from the GSE201348 dataset using the “Seurat” R package (v 4.3.0). Quality control of cells was conducted based on the following criteria: (I) selection of genes detected in cells between 200 and 6,000; (II) inclusion of cells with a mitochondrial ratio below 5%. Cell subset annotation was performed using the PanglaoDB database (https://panglaodb.se/).
Cell-cell communication
We used the “CellChat” package (v 1.6.1) to analyze cell-cell communication, and the selected database information was “Secreted Signaling”.
CIBERSORTx analysis
Cibersort analysis was conducted on the cancer samples (622 samples) from the READ and COAD datasets (TCGA-CRC queue) on the CIBERSORTx website (https://cibersortx.stanford.edu/). K-means clustering of the cancer samples was performed using the “factoextra” R package (v 1.0.7). The expression of cibersortx isoforms in 22 immune cells was analyzed using the “ggpubr” R package (v 0.6.0).
Screening of differentially expressed genes (DEGs) and functional analysis
Differential analysis was conducted using the “edgeR” R package. A Wayne diagram was generated using the “VennDiagram” package. Functional enrichment analysis was performed on the metascape website (https://metascape.org/).
Screening of prognostic genes for CRC
Cox univariate analysis was performed on DEGs using the “survival” R package (v 3.5.5). A forest map was plotted using the “forestplot” R package (v 3.1.1). LASSO regression analysis was performed using the “glmnet” package (v 4.1.7). Survival analysis was carried out using the “survival” R package. Receiver operating characteristic (ROC) analysis was conducted using the “survivalROC” R package (v 1.0.3.1). The results of the aforementioned analysis were visualized using the “ggsci” R package (v 3.0.0). Finally, a nomogram was created using the “rms” package (v 6.7.1).
Single-sample gene set enrichment analysis (ssGSEA)
ssGSEA was performed on the genes using the “clusterProfiler” package (v 4.8.1) to reveal the top-ranked pathways.
Correlation analysis
Correlation analysis was performed using the “cor.test” function, “ggplot2”, or “ggpubr” built-in in R language. A correlation heatmap was generated using the “pheatmap” R package (v 1.0.12).
Statistics
The data were statistically analyzed and plotted using the R language, presented as mean ± standard deviation (). Pairwise comparison (intra-group comparison) of differences between groups was conducted using the rank sum test and chi-square test, while the whole group comparison was conducted using the Kruskal-Wallis test. Kruskal-Wallis analysis was performed using the “ggpubr” R package (v 0.6.0). The “ggstatsplot” R package (v 0.11.0) was used for chi-square test. P<0.05 was considered for determining a significant difference.
Results
Single-cell analysis
Four samples selected from the bulk RNA-seq GSE201348 were subjected to scRNA-seq analysis. After quality control, a total of 10,619 cells (total 22,830 genes) were included in subsequent analysis. These cells were clustered into 22 clusters (Figure 1A), and 12 cell types were obtained when these clusters were annotated using the PanglaoDB database, namely: cholangiocytes, goblet, epithelial, smooth muscle, macrophages, transit amplifying, fibroblasts, T, endothelial, plasma, dendritic and enteric glia (Figure 1B). The distribution of these 12 cell types in the cancer and normal samples is depicted in Figure 1C, revealing a larger number of epithelial, goblet, and smooth muscle in the normal samples compared to that in the cancer samples. Additionally, differential analysis was conducted on GSE201348, after which 5,210 DEGs were screened out based on P<0.05. Figure 1D shows the expression of the top ten DEGs in different cell types in the two groups of samples, with DST showing significant differences in most cell types.
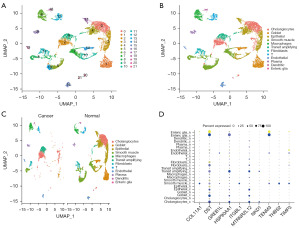
Cell-cell communication
Cell-cell communication analysis revealed that smooth muscle, epithelial, and cholangiocytes were the top three cell types with the highest number and intensity of interactions (Figure 2A), suggesting that they may play significant roles in CRC development. When cells acted as signalers, they were clustered into four categories based on different interaction patterns between cells. Notably, cholangiocytes, goblet, and epithelial were clustered into pattern 1, and pattern 1 showed a strong correlation with proteinase-activated receptors (PARs), GUCA and GAS pathways (Figure 2B). Correlation analysis further highlighted a strong correlation between cholangiocytes and the GAS pathways (Figure 2C). When cells acted as signal recipients, they were also clustered into four categories according to distinct interaction patterns between cells. In this case, cholangiocytes, goblet, epithelial, smooth muscle, and transit amplifying were clustered into pattern 1, which exhibited a strong correlation with PARs, VISFATIN, BMP, and GUCA pathways (Figure 2D). Correlation analysis showed that epithelial had a strong correlation with GUCA (Figure 2E). Obviously, the PARs signaling pathway ranked first in terms of the overall cellular communication intensity (the sum of reception and output), signifying its crucial role whether cells act as a signal sender or receiver (Figure 2F).
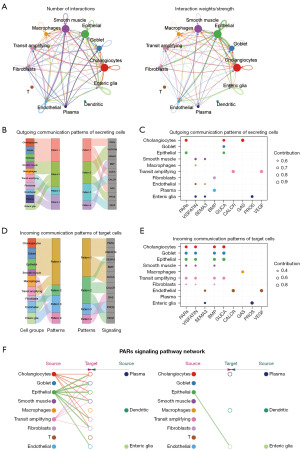
CIBERSORTx analysis
We conducted a cibersort analysis using the TCGA-CRC queue (Figure 3A). K-means clustering of the samples revealed the highest slope change when k=3. When the samples were clustered into three categories, the grouping differences were found to be significant (Figure 3B,3C). Based on the k-means clustering results, we classified the samples and conducted survival analysis, and the results showed significant differences (P=0.03) (Figure 3D). Furthermore, we analyzed the expression of k1, k2, and k3 in the 22 types of immune cells (Figure 3E). The analysis revealed non-significant differences in B cells memory and neutrophils, while significant differences were observed in the remaining 20 immune cells. Therefore, we divided the samples into three categories: k1, k2, and k3 for subsequent bioinformatics analysis, representing three different subtypes of cancer.
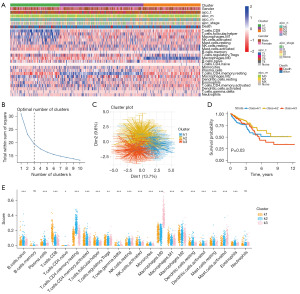
Screening of key genes and functional analysis
Differential analysis was conducted on the TCGA-CRC queue based on the standard of P<0.05 and |log2fold change (FC)| >1, and 4,408 DEGs were selected accordingly (Figure 4A). Given the significant differences observed in survival analysis between k1 and k2, we conducted differential analysis specifically on the samples from k1 and k2 based on P<0.05 as the standard, and ultimately identified 1,899 DEGs (Figure 4B). The intersection of DEGs was selected from the scRNA-seq, TCGA-CRC queue, and two subtypes of cancer, and 77 key genes were identified (Figure 4C). Functional enrichment analysis was conducted on these genes (Figure 4D), and a network diagram was plotted (Figure 4E). The results showed that the 77 key genes were mainly involved in steroid metabolic process, completion activation, and classical pathway, etc.
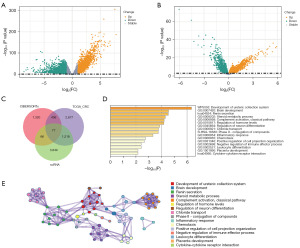
Establishment of RS
Cox univariate analysis was performed on the key genes (Figure 5A), and 14 genes were identified with significant relation to survival. Subsequently, LASSO regression analysis was performed on these 14 genes (Figure 5B,5C), and 7 biomarkers were screened out along with their corresponding coefficients (Figure 5D). We calculated the RS of cancer samples in the TCGA-CRC database based on RS = ∑ (coefficienti × expressioni) to predict prognosis [RS = (−0.092) × expression level of ATOH1 + (−0.089) × expression level of BMP5 + (−0.41) × expression level of CCL24 + (−0.23) × expression level of CLCA4 + (−0.0049) × expression level of RETNLB + (−0.031) × expression level of SULT1B1 + (−0.029) × expression level of UGT2A3]. We conducted survival analysis based on the RS and divided the samples into high and low evaluation groups based on the optimal cutoff value (cutoff =−0.6133481). The results demonstrated a significant difference (P<0.001) between the high and low evaluation groups (Figure 5E). Additionally, ROC analysis was conducted on the cancer samples in the TCGA-CRC database using the survival time of 1, 3, and 6 years as cutoff points (Figure 5F), revealing that the RS could effectively predict the survival of cancer patients. Figure 5G illustrates the RS, survival information, and expressions of the seven biomarkers for the cancer samples in the TCGA-CRC database.
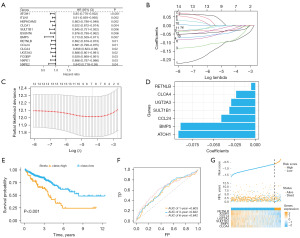
RS validation
We validated the prognostic value of the risk model using GSE12945, GSE29623, and GSE38832 datasets. According to survival analysis, these datasets were divided into high/low survival groups based on the optimal cutoff values (−2.403191, −2.271374, and −2.098198, respectively). The results demonstrated that the high survival group had significantly lower overall survival rates compared to the low survival group, with P values of 0.02, 0.05, and 0.03, respectively (Figure 6A-6C). The 1-, 3- and 6-year area under curve (AUC) values of the ROC curve of the risk model in the GSE12945 dataset were 0.821, 0.632 and 0.661, respectively (Figure 6D). Correspondingly, the AUC values were 0.685, 0.572, and 0.509 in the GSE29623 dataset (Figure 6E), and 0.651, 0.549, and 0.526 in the GSE38832 dataset, respectively (Figure 6F). Additionally, we presented the RS, survival information, and biomarker expression of each sample in each dataset (Figure 6G-6I). These data indicate that the constructed prognostic risk model can accurately evaluate the prognosis of CRC patients. Furthermore, the prediction model based on these seven biomarkers is capable of predicting the survival rate of CRC patients (Figure 7A-7C).
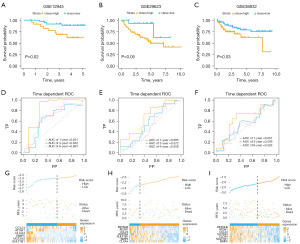
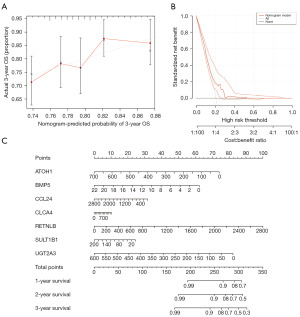
ssGSEA
Based on the TCGA dataset, we performed ssGSEA on the seven biomarkers that constitute the RS. The results showed that RETNLB was associated with the hippo signaling pathway-multiple species (Figure 8A); SULT1B1 was associated with the peroxisome (Figure 8B); UGT2A3 was involved in endocytosis (Figure 8C); CLCA4 was implicated in the salmonella infection (Figure 8D); CCL24 was linked to the coronavirus disease 2019 (COVID-19) (Figure 8E); BMP5 was implicated in endocytosis (Figure 8F); and ATOH1 played a role in the cAMP signaling pathway (Figure 8G).
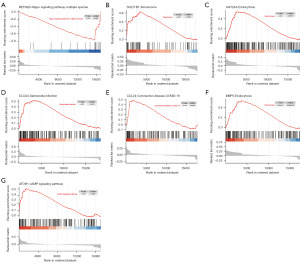
Immunological correlation analysis
A correlation analysis was conducted on the 22 immune cells with RS and its constituent genes (Figure 9A), revealing that RS was significantly negatively correlated with plasma cells, activated CD4+ memory T cells, macrophages M2, resting dendritic cells, resting mast cells, and eosinophils, but positively correlated with T cells follicular helper, resting natural killer (NK) cells, activated NK cells, macrophages M0, macrophages M1 and activated mast cells. Through literature review, four immune indicators (APM, CYT, TIS, and TILS) and eight immune checkpoints (CD274, CTLA4, HAVCR2, LAG3, PDCD1, PDCA1LG2, SIGLEC15, and TIGIT) were retrieved, and their correlation with RS and its constituent genes were analyzed (Figure 9B). The results demonstrated significant negative correlations of RS with CYT and TILs, and notable positive correlation between RS and SIGLEC15. According to the RS, the cancer samples in the TCGA-CRC database were divided into high/low evaluation sample groups, and the scores of these groups in the 22 types of immune cells were plotted (Figure 9C). Significant differences were observed between the high and low evaluation groups in B cells memory, plasma cells, resting T cells CD4 memory, resting NK cells, activated NK cells, and resting dendritic cells. Figure 9D-9K further illustrates that the RS displayed significant negative correlations with plasma cells, resting T cells CD4 memory, monocytes, and eosinophils, while exhibiting significant positive correlations with T cells follicular helper, resting NK cells, macrophages M0, and activated mast cells. In conclusion, the seven biomarkers selected in the risk model play an important role in regulating the tumor immune microenvironment of CRC patients.
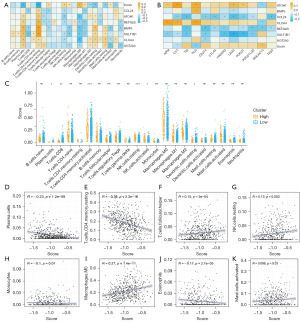
Correlation between RS and the clinical information from TCGA
The correlation between the risk model and the clinical pathological characteristics of CRC patients was assessed. The Kruskal-Wallis test was used to compare differences in risk models across tumor node metastasis (TNM) stages and RS across stages. Figure 10A shows no significant difference in RS among pathological T stages (P=0.24); Figure 10B demonstrates a significant difference in RS among pathological N stages (P<0.001), specifically between N0 and N1, N0 and N2, and N1 and N2; Figure 10C reveals a significant difference in RS among pathological M stages (P=0.002), with a significant difference between M0 and M1; Figure 10D presents a significant difference in RS among tumor stages (P=0.003), specifically between I and III, I and IV, II and III, and II and IV. Based on the above results, RS is associated with pathological N-stage, pathological M-stage, and tumor stage, but not with pathological T-stage.
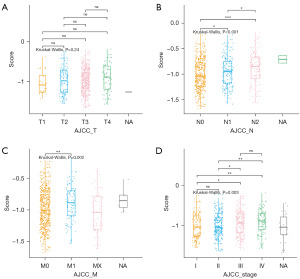
Discussion
CRC is a common malignant tumor in the digestive tract, usually occurring in the colon and rectum. It can be divided into colon cancer and rectal cancer based on its location of onset. These two types of tumors share common histological characteristics and pathogenesis, hence collectively referred to as CRC for research. CRC can be broadly classified into ulcerative, protruding, and infiltrative types, while its histological classification includes adenocarcinoma, adenosquamous carcinoma and undifferentiated carcinoma (21,22). This study addresses the need for effective and reliable predictive biomarkers for monitoring the progression of advanced CRC. The objective is to construct a new prognostic risk model through bioinformatics analysis, with the aim of assessing the prognosis of CRC patients and identifying potential targets to enhance the outcomes of immunotherapy.
We obtained single-cell sequencing data related to CRC from the GEO database for cell annotation and differential analysis. Meanwhile, READ and COAD data were downloaded from the TCGA database for differential analysis. Immune infiltration analysis and k-means clustering were performed on the cancer samples from the READ and COAD data, and the cancer samples were reclassified into three categories (k1, k2, and k3). Subsequently, differential analysis was conducted on the k1 and k2 groups of samples based on the results of survival analysis. Finally, by taking intersection of the differential analysis results, we identified 77 key genes. Functional analysis revealed enrichment of these genes in the steroid metabolic process, complement activation, and classical pathway, etc. Brain metastasis is believed to occur through the entry of circulating tumor cells into brain microvessels. The development of brain metastasis is an important factor for the poor prognosis of patients with advanced cancer. Notably, patients with CRC are particularly prone to developing brain metastasis (23). In animal experiments, hypothalamic oxytocin-producing neurons were found to regulate the progression of colitis-associated cancer (CAC) in mice (24). Inhibition of the renin-angiotensin system pathway has shown efficacy in reducing tumor growth and metastasis, and inhibitors targeting this pathway have demonstrated promising results in in clinical practice (25). Moreover, the (pro) renin receptor has been reported in promoting CRC progression by inhibiting NEDD4L-mediated Wnt3 ubiquitination and regulating intestinal microbiota (26). It is also considered as a potential therapeutic target for pancreatic cancer, CRC, brain cancer and other cancers (27). Elevated levels of intestinal bile acid are a risk factor for CRC (28), as bile acids can be converted by gut microbiota in the small intestine into tumor-promoting secondary bile acids that can promote CRC (29). Bile acids have also been reported as both tumor inducers and promoters in esophageal cancer, CRC and hepatocellular carcinoma, while exhibiting inhibitory effects on breast cancer at specific concentrations (30). Researchers found that loss of intracellular complement C5a/C5aR1 can destabilize β-catenin and significantly block the development of CRC (31), indicating the regulatory role of C5aR1 in the occurrence of CRC through immune regulation (32).
In order to screen out hub genes related to CRC prognosis among the key genes, we conducted Cox univariate analysis on the key genes and identified 14 genes related to CRC prognosis. Subsequently, we conducted LASSO analysis and ultimately selected seven biomarkers (RETNLB, CLCA4, UGT2A3, SULT1B1, CCL24, BMP5, and ATOH1) for constructing a prognostic risk model. According to existing literature reports, RETNLB is a tumor promoter in oral squamous cell carcinoma and is significantly correlated with poor prognosis in CRC patients (33); CLCA4 can reduce the proliferation, migration, and invasion of CRC cells by inhibiting the PI3K/AKT pathway (34-36); CLCA4 serves as a tumor suppressor in esophageal cancer (37), but promotes tumor development in head and neck squamous cell carcinoma (38); UGT2A3 inhibits the proliferation and metastasis of CRC cells (39); similarly, SULT1B1 is a distinct biomarker for CRC (40); CCL24 promotes the occurrence of various cancers, including CRC, non-small cell carcinoma, and nasopharyngeal carcinoma, through M2 macrophage polarization, angiogenesis, invasion and migration, and eosinophil recruitment (41); BMP5 induces a reduction in migration and invasion of breast cancer cells (42), and BMP5 gene deletion delays the occurrence of prostate cancer and skin cancer in mice (43); ATOH1 has been less studied in the mechanism of cancer, but its association with intestinal health has been identified.
The RS has demonstrated a good predictive ability for the prognosis of CRC patients. Clinical correlation analysis has confirmed that RS is associated with pathological N stage, pathological M stage, and tumor staging, but not with pathological T stage. Immunotherapy has emerged as a promising approach in cancer treatment which helps improve the prognosis of cancer patients (44). Infiltration of immune regulatory cells such as regulatory T cells, regulatory macrophages, and myeloid suppressor cells into the tumor tissue can lead to an anti-tumor immune response, thus having a negative impact on the prognosis of cancer patients (45). These immunomodulatory cells are characterized by high expression levels of their immune checkpoints. ICB enhances immune responses or relieves immune suppression by targeting immune checkpoints, which are ligand receptor pairs (46) that inhibits or stimulates immune responses. Immune checkpoints related to tumor cells mediate immune evasion and contribute to maintaining various malignant behaviors, including self-renewal, epithelial mesenchymal transition, metastasis, drug resistance, anti-apoptosis, angiogenesis, and enhancement of energy metabolism (47,48). Remarkable progress has been made in the clinical application of ICB therapy for advanced malignant tumors, with anti PD-1/PD-L1 drugs receiving approval as second-line treatment for metastatic CRC (mCRC) (49). Immune correlation analysis confirmed that RS was negatively correlated with plasma cells, activated T cell CD4 memory, macrophage M2, resting dendritic cells, resting mast cells, eosinophils, CYT, and TILs. RS was positively correlated with T cells follicular helper, resting NK cells, activated NK cells, macrophages M0, macrophages M1, activated mast cells and SIGLEC15. These findings suggest that RS may play an important role in immunotherapy, and these identified biomarkers may serve as targets for improving immunotherapy.
This study also has certain limitations. The analysis of CRC patient data relied on data downloaded from public databases, and the lack of access to individual clinical samples and information restricts the validation in an in vitro setting.
Conclusions
In this study, CRC-related single-cell, transcriptome and clinical data were obtained from publicly available GEO and TCGA databases. Key genes associated with CRC prognosis were identified through differential analysis, CIBERSORTx analysis, LASSO analysis, Cox univariate analysis and survival analysis, leading to the development of a prognostic risk model encompassing seven genes and the establishment of an RS. The correlation of RS with tumor immune microenvironment, pathological N stage, pathological M stage and tumor staging was investigated. We hope that through this analysis, a new prognostic risk model is constructed for evaluating the prognosis of CRC patients, and potential targets are identified for improving immunotherapy.
Acknowledgments
Funding: This work was supported by
Footnote
Reporting Checklist: The authors have completed the TRIPOD reporting checklist. Available at https://tcr.amegroups.com/article/view/10.21037/tcr-23-2281/rc
Peer Review File: Available at https://tcr.amegroups.com/article/view/10.21037/tcr-23-2281/prf
Conflicts of Interest: Both authors have completed the ICMJE uniform disclosure form (available at https://tcr.amegroups.com/article/view/10.21037/tcr-23-2281/coif). Both authors have no conflicts of interest to declare.
Ethical Statement: The authors are accountable for all aspects of the work in ensuring that questions related to the accuracy or integrity of any part of the work are appropriately investigated and resolved. The study was conducted in accordance with the Declaration of Helsinki (as revised in 2013).
Open Access Statement: This is an Open Access article distributed in accordance with the Creative Commons Attribution-NonCommercial-NoDerivs 4.0 International License (CC BY-NC-ND 4.0), which permits the non-commercial replication and distribution of the article with the strict proviso that no changes or edits are made and the original work is properly cited (including links to both the formal publication through the relevant DOI and the license). See: https://creativecommons.org/licenses/by-nc-nd/4.0/.
References
- Maomao C, He L, Dianqin S, et al. Current cancer burden in China: epidemiology, etiology, and prevention. Cancer Biol Med 2022;19:1121-38. [Crossref] [PubMed]
- Sullivan BA, Noujaim M, Roper J. Cause, Epidemiology, and Histology of Polyps and Pathways to Colorectal Cancer. Gastrointest Endosc Clin N Am 2022;32:177-94. [Crossref] [PubMed]
- Weber MF, Sarich PEA, Vaneckova P, et al. Cancer incidence and cancer death in relation to tobacco smoking in a population-based Australian cohort study. Int J Cancer 2021;149:1076-88. [Crossref] [PubMed]
- Park ES, Yu T, Lee HJ, et al. Shinan Sea Salt Intake Ameliorates Colorectal Cancer in AOM/DSS with High Fat Diet-Induced C57BL/6N Mice. J Med Food 2021;24:431-5. [Crossref] [PubMed]
- Ge S, Feng X, Shen L, et al. Association between Habitual Dietary Salt Intake and Risk of Gastric Cancer: A Systematic Review of Observational Studies. Gastroenterol Res Pract 2012;2012:808120. [Crossref] [PubMed]
- Friedenreich CM, Ryder-Burbidge C, McNeil J. Physical activity, obesity and sedentary behavior in cancer etiology: epidemiologic evidence and biologic mechanisms. Mol Oncol 2021;15:790-800. [Crossref] [PubMed]
- Gu MJ, Huang QC, Bao CZ, et al. Attributable causes of colorectal cancer in China. BMC Cancer 2018;18:38. [Crossref] [PubMed]
- De Pergola G, Silvestris F. Obesity as a major risk factor for cancer. J Obes 2013;2013:291546. [Crossref] [PubMed]
- Siegel RL, Torre LA, Soerjomataram I, et al. Global patterns and trends in colorectal cancer incidence in young adults. Gut 2019;68:2179-85. [Crossref] [PubMed]
- Chen Y, Xi D, Zhang Q. Laparoscopic Radical Resection versus Routine Surgery for Colorectal Cancer. Comput Math Methods Med 2022;2022:4899555. [Crossref] [PubMed]
- Lin JS, Piper MA, Perdue LA, et al. Screening for Colorectal Cancer: Updated Evidence Report and Systematic Review for the US Preventive Services Task Force. JAMA 2016;315:2576-94. [Crossref] [PubMed]
- Galluzzi L, Humeau J, Buqué A, et al. Immunostimulation with chemotherapy in the era of immune checkpoint inhibitors. Nat Rev Clin Oncol 2020;17:725-41. [Crossref] [PubMed]
- Morad G, Helmink BA, Sharma P, et al. Hallmarks of response, resistance, and toxicity to immune checkpoint blockade. Cell 2022;185:576. [Crossref] [PubMed]
- Tong H, Wei H, Smith AO, et al. The Role of m6A Epigenetic Modification in the Treatment of Colorectal Cancer Immune Checkpoint Inhibitors. Front Immunol 2022;12:802049. [Crossref] [PubMed]
- Wang Q, Zhao S, Gan L, et al. Bioinformatics analysis of prognostic value of PITX1 gene in breast cancer. Biosci Rep 2020;40:BSR20202537. [Crossref] [PubMed]
- Thomas M, Sakoda LC, Hoffmeister M, et al. Genome-wide Modeling of Polygenic Risk Score in Colorectal Cancer Risk. Am J Hum Genet 2020;107:432-44. [Crossref] [PubMed]
- Fang L, Liu Q, Cui H, et al. Bioinformatics Analysis Highlight Differentially Expressed CCNB1 and PLK1 Genes as Potential Anti-Breast Cancer Drug Targets and Prognostic Markers. Genes (Basel) 2022;13:654. [Crossref] [PubMed]
- Ren N, Liang B, Li Y. Identification of prognosis-related genes in the tumor microenvironment of stomach adenocarcinoma by TCGA and GEO datasets. Biosci Rep 2020;40:BSR20200980. [Crossref] [PubMed]
- Zhao J, Guo C, Ma Z, et al. Identification of a novel gene expression signature associated with overall survival in patients with lung adenocarcinoma: A comprehensive analysis based on TCGA and GEO databases. Lung Cancer 2020;149:90-6. [Crossref] [PubMed]
- Liu XS, Zhou LM, Yuan LL, et al. NPM1 Is a Prognostic Biomarker Involved in Immune Infiltration of Lung Adenocarcinoma and Associated With m6A Modification and Glycolysis. Front Immunol 2021;12:724741. [Crossref] [PubMed]
- Ono Y, Yilmaz O. Emerging and under-recognised patterns of colorectal carcinoma morphologies: a comprehensive review. J Clin Pathol 2024;77:439-51. [Crossref] [PubMed]
- Matsumoto T, Shimizu M, Iida M, et al. Primary low-grade, B-cell, mucosa-associated lymphoid tissue lymphoma of the colorectum: clinical and colonoscopic features in six cases. Gastrointest Endosc 1998;48:501-8. [Crossref] [PubMed]
- Achrol AS, Rennert RC, Anders C, et al. Brain metastases. Nat Rev Dis Primers 2019;5:5. [Crossref] [PubMed]
- Pan S, Yin K, Tang Z, et al. Stimulation of hypothalamic oxytocin neurons suppresses colorectal cancer progression in mice. Elife 2021;10:e67535. [Crossref] [PubMed]
- Tabatabai E, Khazaei M, Parizadeh MR, et al. The Potential Therapeutic Value of Renin-Angiotensin System Inhibitors in the Treatment of Colorectal Cancer. Curr Pharm Des 2022;28:71-6. [Crossref] [PubMed]
- Wang J, Ding Y, Li D, et al. (Pro)renin receptor promotes colorectal cancer progression through inhibiting the NEDD4L-mediated Wnt3 ubiquitination and modulating gut microbiota. Cell Commun Signal 2023;21:2. [Crossref] [PubMed]
- Wang J, Nishiyama A, Matsuyama M, et al. The (pro)renin receptor: a novel biomarker and potential therapeutic target for various cancers. Cell Commun Signal 2020;18:39. [Crossref] [PubMed]
- Fu T, Coulter S, Yoshihara E, et al. FXR Regulates Intestinal Cancer Stem Cell Proliferation. Cell 2019;176:1098-1112.e18. [Crossref] [PubMed]
- Ocvirk S, O'Keefe SJD. Dietary fat, bile acid metabolism and colorectal cancer. Semin Cancer Biol 2021;73:347-55. [Crossref] [PubMed]
- Režen T, Rozman D, Kovács T, et al. The role of bile acids in carcinogenesis. Cell Mol Life Sci 2022;79:243. [Crossref] [PubMed]
- Ding P, Xu Y, Li L, et al. Intracellular complement C5a/C5aR1 stabilizes β-catenin to promote colorectal tumorigenesis. Cell Rep 2022;39:110851. [Crossref] [PubMed]
- Ding P, Li L, Li L, et al. C5aR1 is a master regulator in Colorectal Tumorigenesis via Immune modulation. Theranostics 2020;10:8619-32. [Crossref] [PubMed]
- Di Rosa M, Di Cataldo A, Broggi G, et al. Resistin-like beta reduction is associated to low survival rate and is downregulated by adjuvant therapy in colorectal cancer patients. Sci Rep 2023;13:1490. [Crossref] [PubMed]
- Wei L, Chen W, Zhao J, et al. Downregulation of CLCA4 expression is associated with the development and progression of colorectal cancer. Oncol Lett 2020;20:631-8. [Crossref] [PubMed]
- Li H, Huang B. <em>miR-19a</em> targeting <em>CLCA4</em> to regulate the proliferation, migration, and invasion of colorectal cancer cells. Eur J Histochem 2022;66:3381. [Crossref] [PubMed]
- Chen H, Liu Y, Jiang CJ, et al. Calcium-Activated Chloride Channel A4 (CLCA4) Plays Inhibitory Roles in Invasion and Migration Through Suppressing Epithelial-Mesenchymal Transition via PI3K/AKT Signaling in Colorectal Cancer. Med Sci Monit 2019;25:4176-85. [Crossref] [PubMed]
- Song X, Zhang S, Li S, et al. Expression of the CLCA4 Gene in Esophageal Carcinoma and Its Impact on the Biologic Function of Esophageal Carcinoma Cells. J Oncol 2021;2021:1649344. [Crossref] [PubMed]
- Li B, Jiang YP, Zhu J, et al. MiR-501-5p acts as an energetic regulator in head and neck squamous cell carcinoma cells growth and aggressiveness via reducing CLCA4. Mol Biol Rep 2020;47:2181-7. [Crossref] [PubMed]
- Wu H, Zhong W, Zhang R, et al. G-quadruplex-enhanced circular single-stranded DNA (G4-CSSD) adsorption of miRNA to inhibit colon cancer progression. Cancer Med 2023;12:9774-87. [Crossref] [PubMed]
- Lian W, Jin H, Cao J, et al. Identification of novel biomarkers affecting the metastasis of colorectal cancer through bioinformatics analysis and validation through qRT-PCR. Cancer Cell Int 2020;20:105. [Crossref] [PubMed]
- Lim SJ. CCL24 Signaling in the Tumor Microenvironment. Adv Exp Med Biol 2021;1302:91-8. [Crossref] [PubMed]
- Jin Y, Park S, Park SY, et al. G9a Knockdown Suppresses Cancer Aggressiveness by Facilitating Smad Protein Phosphorylation through Increasing BMP5 Expression in Luminal A Type Breast Cancer. Int J Mol Sci 2022;23:589. [Crossref] [PubMed]
- Tremblay M, Viala S, Shafer ME, et al. Regulation of stem/progenitor cell maintenance by BMP5 in prostate homeostasis and cancer initiation. Elife 2020;9:e54542. [Crossref] [PubMed]
- Johdi NA, Sukor NF. Colorectal Cancer Immunotherapy: Options and Strategies. Front Immunol 2020;11:1624. [Crossref] [PubMed]
- Iglesias-Escudero M, Arias-González N, Martínez-Cáceres E. Regulatory cells and the effect of cancer immunotherapy. Mol Cancer 2023;22:26. [Crossref] [PubMed]
- Zhang Y, Zheng J. Functions of Immune Checkpoint Molecules Beyond Immune Evasion. Adv Exp Med Biol 2020;1248:201-26. [Crossref] [PubMed]
- Song E, Mao T, Dong H, et al. VEGF-C-driven lymphatic drainage enables immunosurveillance of brain tumours. Nature 2020;577:689-94. [Crossref] [PubMed]
- Dersh D, Hollý J, Yewdell JW. A few good peptides: MHC class I-based cancer immunosurveillance and immunoevasion. Nat Rev Immunol 2021;21:116-28. [Crossref] [PubMed]
- Weng J, Li S, Zhu Z, et al. Exploring immunotherapy in colorectal cancer. J Hematol Oncol 2022;15:95. [Crossref] [PubMed]