Development of a prediction model for the association between thyroid dysfunction and breast cancer
Highlight box
Key findings
• The predictive model based on thyroid function-related hormone levels can effectively predict benign and malignant breast tumors, indicating the clinical value of thyroid function in breast cancer diagnosis.
• With increasing age, especially during perimenopause, thyroid hormones gradually replace the role of sex hormone levels and play a relatively important role in the process affecting the occurrence and development of breast cancer.
What is known and what is new?
• Patients with thyroid dysfunction also have an increased risk of developing breast cancer.
• A model-based approach to explore the relationship between thyroid dysfunction and benign/malignant breast tumors was established.
What is the implication, and what should change now?
• The findings of this study provide a new theoretical basis for breast cancer screening and prevention, can contribute to reducing the incidence of breast cancer from the source, and facilitate the early intervention of breast cancer.
Introduction
According to the data released by the International Agency for Research on Cancer (IARC) of the World Health Organization (WHO) in 2020, breast cancer has surpassed lung cancer and became the most common cancer among women worldwide (1,2). There are approximately 2.6 million new cases of breast cancer each year globally, with 420,000 new cases in China (1,2). In recent years, the incidence of breast cancer has been increasing annually at a rate of 3% to 4%, and there is a younger trend of breast cancer patients (2). In a global context, China accounts for 12.20% of the newly diagnosed breast cancer cases worldwide, and breast cancer-related deaths among women account for 9.60% of malignant tumor-related deaths, ranking second (2). Thyroid diseases are also more common in women, with a male-to-female ratio of approximately 1:4 (2). In clinical practice, there is a growing number of breast cancer patients who also have thyroid dysfunction. There is a certain mutual pathogenic relationship between thyroid dysfunction and breast cancer. It is indicated that elevated serum levels of free triiodothyronine, free thyroxine, free triiodothyronine/free thyroxine, and thyroid-stimulating hormone are risk factors for the occurrence of breast cancer (3). Huang et al. (4) reported that the risk of breast cancer was significantly higher in the hypothyroidism cohort compared to the non-hypothyroidism cohort, especially among individuals aged 40 to 64 years. Despite extensive research on breast malignant tumors and thyroid dysfunction diseases, there is currently no conclusive evidence to establish a causal relationship between the two.
The incidence of thyroid disease is closely related to gender, age, genetic factors, iodine intake, history of radiation exposure, as well as other endocrine and immune factors of patients (5). There are many overlapping risk factors between thyroid disease and known risk factors for breast cancer (6). Both the thyroid and the breast are hormone-responsive organs, regulated by the hypothalamic-pituitary system, and changes in endocrine function are closely associated with the occurrence of diseases in both organs. Estrogen and thyroid hormones mutually influence each other in the development, physiology, and pathology of breast cancer and thyroid disease. Estrogen affects the development, physiology, and pathology of the thyroid, thereby leading to the occurrence of thyroid disease. Especially in premenopausal women, the association between tumors such as papillary carcinomas and breast cancer is more significant (7). Conversely, thyroid hormones also have an impact on the incidence of breast cancer (8,9). The relationship between hypothyroidism or hypothyroidism and the risk factor for breast cancer remains unclear (10-12). However, in clinical practice, there is a growing number of breast cancer patients who also have thyroid dysfunction.
Herein, this study aims to identify new risk factors and diagnostic markers for breast cancer. We analyzed the incidence of thyroid dysfunction between patients with benign and malignant tumors by analyzing the laboratory data of breast cancer patients from the Affiliated Tumor Hospital of Xinjiang Medical University from 2015 to 2019. Moreover, we used a model-based approach to explore the relationship between thyroid dysfunction and benign/malignant breast tumors. We present this article in accordance with the TRIPOD reporting checklist (available at https://tcr.amegroups.com/article/view/10.21037/tcr-23-2164/rc).
Methods
Study participants
This retrospective study collected data from breast cancer patients and benign breast tumor patients who received inpatient treatment at the Affiliated Tumor Hospital of Xinjiang Medical University from 2015 to 2019. Inclusion criteria included: (I) patients with clinical pathology diagnosis of breast cancer or benign breast tumors; (II) patients were newly diagnosed cases without prior surgery, chemotherapy, radiotherapy, or other tumor treatments; (III) patients with complete laboratory examination data. Patients with incomplete clinical data or laboratory examination data were excluded.
The study was conducted following the Declaration of Helsinki (as revised in 2013). The study was approved by the Ethics Committee of Affiliated Tumor Hospital of Xinjiang Medical University (No. K-2023045) and informed consent was waived due to the retrospective nature of the study.
Data collection
The general information and laboratory examination data were collected. The general information included gender, age, height, weight, occupation, marital status, etc. The laboratory examination data on thyroid function were collected, including thyroid-stimulating hormone, thyronine, thyroxine, anti-thyroid peroxidase antibody, serum-free thyroxine, serum-free triiodothyronine, and thyroglobulin.
Construction and validation of a prediction model
We constructed a prediction model using Python. The model construction included four stages: data processing, feature selection, model construction, and model evaluation. During the data processing, we first collected sample data, then removed invalid data, and performed data standardization. The least absolute shrinkage and selection operator (LASSO) analysis was conducted based on the classification probabilities outputted by the model and the clinical information to select and optimize the features. Next, we employed two methods, namely the Random Forest algorithm and support vector machine (SVM), for model construction. Finally, the predictive performance of the model was assessed using the receiver operating characteristic (ROC) curves, decision analysis, and calibration curves.
Statistical analysis
The proportions of each category were analyzed as categorical data using the Chi-square test. Measurement data were described using mean ± standard deviation and compared using the two-sample t-test. A significance level of P<0.05 indicated a statistical difference.
Results
Demographic data and basic data of participants
This study involved a total of 14,526 patients, including 9,287 cases of benign breast tumors and 5,239 cases of malignant tumors. There were a total of 5,447 cases (37.50%) aged 40 years and below, 4,456 cases (30.70%) aged 41 to 50 years, 3,010 cases (20.70%) aged 51 to 60 years, and 1,613 cases (11.10%) aged 61 years and above. The distribution of benign and malignant breast tumors significantly varied across different age groups, as indicated in Table 1 (χ2=5,502.454, P<0.001). Patients with benign breast tumors were predominantly observed in cases aged 40 years and below (54.3%) and 41–50 years (34.10%), whereas malignant breast tumors were more prevalent among individuals aged 51–60 years (40.30%) and above 60 years (27.40%). Thus, the incidence of breast benign tumors was the highest in cases aged 40 and below, and it tended to decline with age, while the incidence of breast malignant tumors showed an increasing trend with age. Additionally, there were statistically significant differences in the levels of thyroid-stimulating hormone (χ2=16.757, P<0.001), thyronine (χ2=10.689, P=0.005), free thyroxine (χ2=20.555, P<0.001), free triiodothyronine (χ2=43.009, P<0.001), and thyroglobulin (χ2=41.889, P<0.001) between the benign and malignant breast tumors.
Table 1
Variable | Patients with benign breast tumors, n (%) | Patients with malignant breast tumors, n (%) | Total | χ2 | P |
---|---|---|---|---|---|
Ethnicity | 2.980 | 0.08 | |||
Han | 6,621 (71.3) | 3,664 (69.9) | 10,285 | ||
Others | 2,666 (28.7) | 1,575 (30.1) | 4,241 | ||
Age (years) | 5,502.454 | <0.001 | |||
40 and below | 5,041 (54.3) | 406 (7.7) | 5,447 | ||
41–50 | 3,168 (34.1) | 1,288 (24.6) | 4,456 | ||
51–60 | 900 (9.7) | 2,110 (40.3) | 3,010 | ||
61 and above | 178 (1.9) | 1,435 (27.4) | 1,613 | ||
Thyroid-stimulating hormone (μIU/mL) (range, 0.6–5.4) | 16.757 | <0.001 | |||
High | 1,490 (16.0) | 960 (18.3) | 2,450 | ||
Low | 109 (1.2) | 81 (1.5) | 190 | ||
Normal | 7,688 (82.8) | 4,198 (80.1) | 11,886 | ||
Thyronine (nmol/L) (range, 1.5–3.2) | 10.689 | 0.005 | |||
High | 28 (0.3) | 19 (0.4) | 47 | ||
Low | 395 (4.3) | 284 (5.4) | 679 | ||
Normal | 8,864 (95.4) | 4,936 (94.2) | 13,800 | ||
Thyroxine (nmol/L) (range, 66.0–136.0) | 3.352 | 0.19 | |||
High | 16 (0.2) | 13 (0.2) | 29 | ||
Low | 181 (1.9) | 122 (2.3) | 303 | ||
Normal | 9,090 (97.9) | 5,104 (97.4) | 14,194 | ||
Anti-thyroid peroxidase antibody (IU/mL) (range, 0–32.0) | 2.641 | 0.10 | |||
High | 1,357 (14.6) | 818 (15.6) | 2175 | ||
Normal | 7,930 (85.4) | 4,421 (84.4) | 12,351 | ||
Serum free thyroxine (pmol/L) (range, 12.0–22.0) | 20.555 | <0.001 | |||
High | 170 (1.8) | 132 (2.5) | 302 | ||
Low | 210 (2.3) | 169 (3.2) | 379 | ||
Normal | 8,907 (95.9) | 4,938 (94.3) | 13,845 | ||
Serum free triiodothyronine (pmol/L) (range, 4.0–6.6) | 43.009 | <0.001 | |||
High | 75 (0.8) | 38 (0.7) | 113 | ||
Low | 19 (0.2) | 52 (1.0) | 71 | ||
Normal | 9,193 (99.0) | 5,149 (98.3) | 14,342 | ||
Thyroglobulin (ng/mL) (range, 3.67–79.67) | 41.889 | <0.001 | |||
High | 151 (1.6) | 161 (3.1) | 312 | ||
Low | 1,611 (17.3) | 996 (19) | 2,607 | ||
Normal | 7,525 (81.0) | 4,082 (77.9) | 11,607 |
The 5,239 malignant breast cancer patients and 9,287 benign breast tumor patients were randomly allocated to the training set and validation set in a ratio of 7:3. The baseline data between the training and validation sets were compared. As shown in Table 2, there were no significant differences in thyroid function, hormone levels, and age between the training and validation sets, indicating comparability.
Table 2
Variables | Cases with benign breast tumors (n=9,287) | Cases with malignant breast tumors (n=5,239) | |||||
---|---|---|---|---|---|---|---|
The training set (n=5,256) | The validation set (n=2,253) | P | The training set (n=2,946) | The validation set (n=1,262) | P | ||
Thyroid-stimulating hormone (μIU/mL) | 2.96±3.15 | 2.98±3.73 | 0.56 | 3.21±4.94 | 3.16±4.47 | 0.69 | |
Thyronine (pmol/L) | 1.76±0.32 | 1.76±0.32 | 0.95 | 1.76±0.33 | 1.79±0.50 | 0.60 | |
Thyroxine (nmol/L) | 98.38±17.45 | 98.98±18.74 | 0.80 | 101.71±20.11 | 102.48±22.88 | 0.14 | |
Anti-thyroid peroxidase antibody (IU/mL) | 38.59±86.00 | 40.02±92.30 | 0.62 | 42.99±94.18 | 37.43±82.98 | 0.10 | |
Serum free thyroxine (pmol/L) | 16.32±2.97 | 16.47±2.70 | 0.41 | 16.45±2.89 | 16.63±5.04 | 0.51 | |
Serum free triiodothyronine (pmol/L) | 4.74±0.82 | 4.75±0.71 | 0.78 | 4.64±0.73 | 4.73±2.04 | 0.87 | |
Thyroglobulin (ng/mL) | 13.44±25.40 | 14.12±28.98 | 0.33 | 16.46±36.40 | 18.22±43.92 | 0.99 | |
Progesterone (nmol/L) | 12.69±18.99 | 12.68±19.01 | 0.18 | 6.68±14.11 | 6.30±13.77 | 0.79 | |
Testosterone (nmol/L) | 0.77±0.48 | 0.78±0.46 | 0.72 | 0.70±0.46 | 0.70±0.50 | 0.35 | |
Follicle-stimulating hormone (mIU/mL) | 13.43±20.64 | 13.88±21.33 | 0.47 | 32.51±30.59 | 34.46±32.88 | 0.69 | |
Luteinizing hormone (mIU/mL) | 11.75±12.91 | 12.02±13.03 | 0.29 | 19.30±15.81 | 20.23±16.66 | 0.47 | |
Estradiol (pmol/L) | 390.70±412.09 | 394.48±435.09 | 0.79 | 267.88±489.57 | 245.39±452.21 | 0.37 | |
Prolactin (mIU/mL) | 455.63±330.21 | 460.69±311.55 | 0.058 | 402.20±387.56 | 413.34±459.87 | 0.50 | |
Age (years) | 39.42±10.00 | 39.51±10.20 | 0.22 | 53.60±10.50 | 52.90±10.30 | 0.85 |
Data are presented as mean ± standard deviation and compared using the two-sample t-test.
Construction of the prediction model
We examined the distribution of data and evaluated the contribution of filtering indicators to the model. The box plot in Figure 1 reveals a relatively concentrated distribution of data for benign tumors, whereas malignant tumors exhibited some outliers. Generally, the distribution of thyroid hormones was consistent in both benign and malignant tumors.
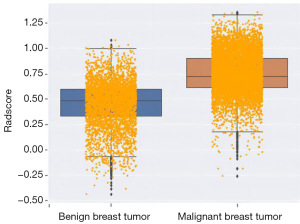
The feature importance of each factor in the model is presented in Figure 2. The ranking of importance of these factors on the malignant or benign nature of breast tumors was as follows: age > follicle-stimulating hormone > luteinizing hormone > prolactin > progesterone > thyroid hormone > testosterone > ethnicity. The respective contribution ratios were 36.01%, 12.93%, 11.36%, 9.83%, 9.82%, 9.51%, 9.32%, and 1.21%, respectively.
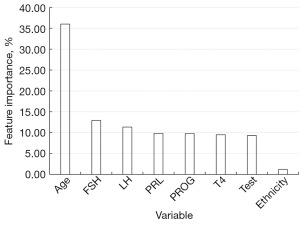
Validation of the prediction model
Figures 3-6 illustrate the validation of the model with different methods. Figure 3 shows the confusion matrix of the prediction model established based on thyroid function, hormones, age, and ethnicity. The prediction model had an accuracy of 83.70%, a precision of 90.69%, a sensitivity of 84.74%, and a specificity of 81.50%. Figure 4 displays the ROC curve with an area under the curve of 0.9012, close to 1, indicating a good predictive performance.
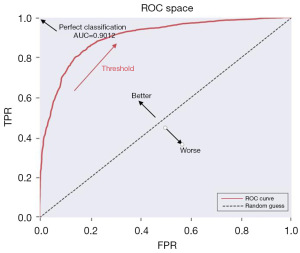
Furthermore, the convergence plot (Figure 5) and the random forest model (Figure 6) were employed to evaluate the performance of the prediction model based on thyroid function, estrogen levels, age, and ethnicity. The training set achieved a score of 1.00, and the validation set achieved a score of 0.84. The LASSO intercept was 0.64. This indicates that the prediction model has good performance.
Discussion
Both the breast and the thyroid gland are target organs for pituitary hormones, and alterations in endocrine function are closely associated with the onset and progression of diseases. Recent epidemiological studies have indicated a positive relationship between thyroid hormone abnormalities and breast diseases (13-16). In the current study, we constructed a predictive model based on factors such as age, ethnicity, thyroid function, and estrogen levels. This model can well predict the occurrence and development of benign and malignant breast tumors.
In this study, we found a certain relationship between age and the occurrence and development of breast cancer. Patients with benign breast tumors were mainly in the age group below 40 years (54.30%) and the 41–50 age group (34.10%), while patients with malignant breast tumors were more prevalent in the age group of 50–60 years (40.30%) and the age group of over 60 years (27.40%). The probability of developing malignant breast tumors increased with age. Thyroid hormone levels may become an important factor affecting the occurrence and development of breast cancer in postmenopausal women.
The relationship between abnormal thyroid function and breast cancer is very complex and is influenced by various factors (17). There may be several reasons for this (18). First, abnormal thyroid function affects the growth and development of breast tissue by regulating the expression and function of estrogen receptors, thereby leading to abnormal cell growth and differentiation in breast tissue and increasing the risk of breast cancer. Second, abnormal thyroid function can affect the proliferation and metastasis of breast cancer cells. Third, sex hormones can affect thyroid function, leading to abnormal thyroid function and increasing the risk of breast cancer. Conversely, abnormal thyroid function may also affect the synthesis and metabolism of sex hormones, further increasing the risk of breast cancer. This study found that with increasing age, especially during perimenopause, the effects of thyroxine and thyroid-stimulating hormones on the occurrence and development of breast cancer gradually replace the role of sex hormone levels, playing a relatively important role.
In this study, a series of advanced computational methods and techniques were used to analyze and predict the benign and malignant nature of breast cancer. By thoroughly exploring key aspects such as feature selection, model construction, and model evaluation, the aim was to construct an efficient, accurate, and interpretable predictive model. Firstly, a deep exploration of feature selection, a critical step, was conducted. In data analysis, feature selection plays a vital role as it helps researchers identify numerous features most relevant to the target variable, thus improving the predictive accuracy and interpretability of the model (19,20). In this study, the t-test and LASSO method were used for feature selection. The t-test is a commonly used statistical method that helps researchers identify features with significant differences from the target variable. On the other hand, LASSO is a feature selection method based on L1 regularization, enabling the compression of coefficients for irrelevant features to zero, thereby achieving automatic feature selection (21,22).
In the model construction, two advanced machine learning algorithms, Random Forest and SVM, were used. Random Forest is an ensemble learning method that improves the accuracy and stability of predictions by constructing multiple decision trees based on the decision tree (23,24). SVM is a classification algorithm based on kernel methods, which can classify data by constructing an optimal hyperplane (25). In this study, the parameters of SVM were optimized to further improve the predictive performance of the model.
In terms of model evaluation, multiple evaluation metrics and methods were used to assess the performance of the model. Among them, the ROC curve and the area under the curve value are two commonly used evaluation metrics, which can help evaluate the classification performance and accuracy of the model (26,27). In addition, the calibration curves and decision curves were also used in the study to evaluate the prediction accuracy and clinical utility of the model. Through these evaluation methods, a more comprehensive understanding of the performance and strengths and weaknesses of the model can be obtained (28,29).
This study has several limitations. Firstly, it used a retrospective case-control design, which is characterized by lower levels of evidence and credibility compared to prospective cohort studies. Moreover, multiple factors influence the development and occurrence of breast cancer. In this study, a prediction model was used to assess the impact of thyroid hormones and age on breast cancer development. While this provides some insights for future research, further studies should include a more comprehensive and rigorous analysis of influencing factors. Additionally, the age distribution of the patients was not even, which may lead to potential bias.
Conclusions
This study found that the predictive model based on thyroid function-related hormone levels can effectively predict benign and malignant breast tumors, underscoring the clinical significance of thyroid function indicators in diagnosing breast cancer. In addition, with increasing age, especially during perimenopause, thyroid hormones gradually replace the role of sex hormone levels and significantly affect the occurrence and development of breast cancer. Our findings may provide a new theoretical basis for breast cancer screening and prevention, can contribute to reducing the incidence of breast cancer from the source, and facilitate precise early intervention for breast cancer. In future research, the research team plans to further explore the molecular mechanisms and biomarkers of breast cancer. In-depth research utilizing more bioinformatics methods and data can help clinicians better understand the pathogenesis and progression of breast cancer through molecular mechanisms and biomarkers.
Acknowledgments
Funding: This study was supported by the fund from
Footnote
Reporting Checklist: The authors have completed the TRIPOD reporting checklist. Available at https://tcr.amegroups.com/article/view/10.21037/tcr-23-2164/rc
Data Sharing Statement: Available at https://tcr.amegroups.com/article/view/10.21037/tcr-23-2164/dss
Peer Review File: Available at https://tcr.amegroups.com/article/view/10.21037/tcr-23-2164/prf
Conflicts of Interest: All authors have completed the ICMJE uniform disclosure form (available at https://tcr.amegroups.com/article/view/10.21037/tcr-23-2164/coif). The authors have no conflicts of interest to declare.
Ethical Statement:
Open Access Statement: This is an Open Access article distributed in accordance with the Creative Commons Attribution-NonCommercial-NoDerivs 4.0 International License (CC BY-NC-ND 4.0), which permits the non-commercial replication and distribution of the article with the strict proviso that no changes or edits are made and the original work is properly cited (including links to both the formal publication through the relevant DOI and the license). See: https://creativecommons.org/licenses/by-nc-nd/4.0/.
References
- Britt KL, Cuzick J, Phillips KA. Key steps for effective breast cancer prevention. Nat Rev Cancer 2020;20:417-36. [Crossref] [PubMed]
- He SY, Li H, Cao MM, et al. Age Distribution and Trend of Disease Burden for Female Breast Cancer Worldwide and in China. Chinese Journal of Oncology 2023;32:1-7.
- Mu R, Ma Z, Lu C, et al. Role of succinylation modification in thyroid cancer and breast cancer. Am J Cancer Res 2021;11:4683-99. [PubMed]
- Huang CH, Wei JC, Chien TC, et al. Risk of Breast Cancer in Females With Hypothyroidism: A Nationwide, Population-Based, Cohort Study. Endocr Pract 2021;27:298-305. [Crossref] [PubMed]
- Dworakowska D, Grossman AB. Thyroid disease in the time of COVID-19. Endocrine 2020;68:471-4. [Crossref] [PubMed]
- Shi J, Guan Y, Liang D, et al. Cost-effectiveness evaluation of risk-based breast cancer screening in Urban Hebei Province. Sci Rep 2023;13:3370. [Crossref] [PubMed]
- Modi S, Jacot W, Yamashita T, et al. Trastuzumab Deruxtecan in Previously Treated HER2-Low Advanced Breast Cancer. N Engl J Med 2022;387:9-20. [Crossref] [PubMed]
- Liu J, Gao J, Bo DX, et al. Meta-analysis of the relationship between hypothyroidism and the risk of breast cancer. Chinese Journal of Cancer Control and Prevention 2018;25:911-6.
- Halada S, Casado-Medrano V, Baran JA, et al. Hormonal Crosstalk Between Thyroid and Breast Cancer. Endocrinology 2022;163:bqac075. [Crossref] [PubMed]
- Yuan S, Kar S, Vithayathil M, et al. Causal associations of thyroid function and dysfunction with overall, breast and thyroid cancer: A two-sample Mendelian randomization study. Int J Cancer 2020;147:1895-903. [Crossref] [PubMed]
- Han M, Wang Y, Jin Y, et al. Benign thyroid disease and the risk of breast cancer: An updated systematic review and meta-analysis. Front Endocrinol (Lausanne) 2022;13:984593. [Crossref] [PubMed]
- Baldini E, Lauro A, Tripodi D, et al. Thyroid Diseases and Breast Cancer. J Pers Med 2022;12:156. [Crossref] [PubMed]
- Brandt J, Borgquist S, Manjer J. Prospectively measured thyroid hormones and thyroid peroxidase antibodies in relation to risk of different breast cancer subgroups: a Malmo Diet and Cancer Study. Cancer Causes Control 2015;26:1093-104. [Crossref] [PubMed]
- Chen S, Wu F, Hai R, et al. Thyroid disease is associated with an increased risk of breast cancer: a systematic review and meta-analysis. Gland Surg 2021;10:336-46. [Crossref] [PubMed]
- Zurabashvili M, Kvanchakhadze R. Evaluation Of Thyroid Disease Detection Among Female Population With Breast Pathologies In Kvemo Kartli Region (Georgia). Georgian Med News 2021;138-42. [PubMed]
- Ni T, Zhao HN. Research progress on the relationship between breast cancer and thyroid diseases. Cancer Progress 2021;19:1640-2.
- Henderson BE, Feigelson HS. Hormonal carcinogenesis. Carcinogenesis 2000;21:427-33. [Crossref] [PubMed]
- Rinaldi S, Plummer M, Biessy C, et al. Thyroid-stimulating hormone, thyroglobulin, and thyroid hormones and risk of differentiated thyroid carcinoma: the EPIC study. J Natl Cancer Inst 2014;106:dju097. [Crossref] [PubMed]
- Doshi-Velez F, Kim B. Towards A Rigorous Science of Interpretable Machine Learning. (2017-02-19) [2022-10-12]. https://arxiv.org/abs/1702.08608
- Guyon IM. An introduction to variable and feature selection. The Journal of Machine Learning Research 2003;3:1157-82.
- Lundberg SM, Lee SI. A unified approach to interpreting model predictions. Long Beach, USA: Proceedings of the 31st International Conference on Neural Information Processing Systems, 2017:4768-77.
- Tibshirani R. Regression shrinkage and selection via the lasso. Journal of the Royal Statistical Society, Series B 1996;58:267-88. [Crossref]
- Jordan MI, Mitchell TM. Machine learning: Trends, perspectives, and prospects. Science 2015;349:255-60. [Crossref] [PubMed]
- Zaharia M, Xin RS, Wendell P, et al. Apache Spark: a unified engine for big data processing. Communications of the Acm 2016;59:56-65. [Crossref]
- Sokolova M, Lapalme G. A systematic analysis of performance measures for classification tasks. Information Processing & Management 2009;45:427-37. [Crossref]
- Linden A. Measuring diagnostic and predictive accuracy in disease management: an introduction to receiver operating characteristic (ROC) analysis. J Eval Clin Pract 2006;12:132-9. [Crossref] [PubMed]
- Kourou K, Exarchos TP, Exarchos KP, et al. Machine learning applications in cancer prognosis and prediction. Comput Struct Biotechnol J 2014;13:8-17. [Crossref] [PubMed]
- Steyerberg EW, Vickers AJ, Cook NR, et al. Assessing the performance of prediction models: a framework for traditional and novel measures. Epidemiology 2010;21:128-38. [Crossref] [PubMed]
- Van Calster B, Wynants L, Verbeek JFM, et al. Reporting and Interpreting Decision Curve Analysis: A Guide for Investigators. Eur Urol 2018;74:796-804. [Crossref] [PubMed]