The prognostic value of immune escape-related genes in lung adenocarcinoma
Highlight box
Key findings
• A prognostic model consisting of four genes performed well in the prognostic prediction of lung adenocarcinoma (LUAD).
What is known and what is new?
• Immune escape-related genes (IERGs) play a key role in the development and metastasis of LUAD.
• IERGs were used to construct a prognostic model, which was found to be associated with the prognosis of LUAD.
What is the implication, and what should change now?
• This study highlights the importance of IERGs in predicting prognosis of LUAD.
Introduction
Among the different tumor types, lung cancer has one of highest incidences, with approximately 1.8 million people worldwide being diagnosed with this disease every year. Over the past few years, the incidence rate of lung adenocarcinoma (LUAD), a subtype of lung cancer responsible for nearly 50% of lung cancer deaths, has increased (1,2). The emergence of low-dose spiral computed tomography (CT) has changed the pattern of lung cancer screening. An increasing number of patients are being diagnosed with cancer, and when lesions are completely removed, these patients face a good prognosis (3,4). Meanwhile, the development and application of radiotherapy, chemotherapy, molecular targeted drugs, and immune checkpoint inhibitors (ICIs) has also significantly benefited the outcome for these patients (5). However, there are still a considerable number of patients with lung cancer who cannot benefit significantly from these treatments, and more effective and precise treatment methods need to be further explored.
Immune escape, a key factor affecting the efficacy of ICIs, is involved in the emergence and progression of LUAD. The heterogeneous set of immune escape processes shapes the evolution of precursor lesions entering invasive phase, helps promote progression from the early stage to metastatic disease, and defines the main immunological characteristics of the specific subsets of lung cancer molecules (6). Lawson et al. (7) discovered and introduced 182 immune escape-related genes (IERGs), among which CEP55 and AHSA1 are highly relevant to our research.
Previous studies have shown that elevated expression of CEP55 and AHSA1 may induce tumor development, but the clinical significance of these two immune escape gene products in LUAD remains unclear. In this study, we used bioinformatics methods to systematically analyze RNA sequencing (RNAseq) data from normal and LUAD samples obtained from The Cancer Genome Atlas (TCGA) database and, in combination with clinical characteristics, further clarify the roles of CEP55 and AHSA1 in LUAD. We focused on the expression profile of CEP55 and AHSA1 in LUAD and their predictive value in bioinformatics analysis. We then successfully constructed a nomogram based on the expression of CEP55 and AHSA1 in the samples and evaluated the prognostic significance of survival time, survival status, clinical information, and other data in the samples. Finally, the protein expression levels of these two immune escape genes in LUAD tissues were validated using the Human Protein Atlas (HPA) database. This study provides a theoretical basis for novel immunotherapy strategies and contributes to the personalized treatment of patients with LUAD. We present this article in accordance with the TRIPOD reporting checklist (available at https://tcr.amegroups.com/article/view/10.21037/tcr-23-2295/rc).
Methods
Data collection
Clinical information and sequencing RNA data were downloaded from the Gene Expression Omnibus (GEO) TCGA databases, and sequencing data were subjected to missing data imputation and standardization processing. Clinical samples of patients with a survival time of fewer than 30 days and lacking study-critical information were removed. The inclusion criteria were as follows: (I) a pathological type of LUAD and (II) complete clinical information (including survival time, survival status, disease staging, age, and gender) and gene expression matrix. Meanwhile, the exclusion criteria were as follows: (I) samples with incomplete clinical data, (II) samples with excessively incomplete gene expression values, (III) and samples with excessive expression bias. For the training queue, 499 samples were used from TCGA database; for the validation queue, 380 samples from GSE72094 and 83 samples from GSE30219 were collected from the GEO database. The demographic data and clinical characteristics of all queues are shown in Table 1. According to the study by Lawson et al., 182 IERGs were obtained (7). This study was conducted in accordance with the Declaration of Helsinki (as revised in 2013).
Table 1
Clinical feature | TCGA-LUAD (n=499) | GSE72094 (n=380) | GSE30219 (n=83) |
---|---|---|---|
Sex | |||
Female | 260 | 214 | 18 |
Male | 239 | 166 | 65 |
Age, years | |||
<65 | 216 | 104 | 55 |
≥65 | 283 | 276 | 28 |
Pathological staging | |||
Stage 1/2 | 393 | 310 | 82 |
Stage 3/4 | 106 | 70 | 1 |
Survival time, days | |||
<365 | 97 | 52 | 5 |
≥365 | 402 | 328 | 78 |
TCGA, The Cancer Genome Atlas; LUAD, lung adenocarcinoma.
Weighted gene coexpression network analysis
For the TCGA-LUAD dataset, the median absolute deviation (MAD) of each gene was calculated separately, and the top 50% genes with the smallest MAD were removed. Weighted gene coexpression network analysis (WGCNA) conducted via the “goodSamplesGenes” package in R software version 1.17.0.1 (The R Foundation for Statistical Computing) was used to remove outliers and samples. WGCNA was additionally used to construct a scale-free coexpression network, modules with a distances less than 0.25 were merged, and the correlation between module feature vectors and gene expression was calculated to obtain module membership (MM). The samples in the TCGA-LUAD dataset were divided into cancer and noncancer groups, and we associated the grouping information with clinical features. Through calculation of the correlation between each module and clinical features, the module with the strongest positive and negative correlation was selected as the central module for further analysis. The gene significance (GS) and MM of each gene feature in the central module were measured, based upon which the key genes were screened. These key genes were intersected with 182 immune escape genes to obtain differential IERGs.
Functional enrichment analysis
The “clusterProfiler” R software package was used to perform gene ontology (GO) analysis and Kyoto Encyclopedia of Genes and Genomes (KEGG) analysis on IERGs, with which the relevant pathways of action of IERGs were enriched.
Establishment of a prognostic risk model
Survival status, survival time, and gene expression data were integrated into the R software package “glmnet”, and regression analysis was performed, with the least absolute shrinkage and selection operator (LASSO) Cox method being used to further screen for key prognostic genes related to the IERGs. In addition, 10-fold cross-validation was used to obtain the optimal model.
R software was used to calculate the optimal cutoff value for the risk score. The minimum sample size was set to greater than 25% and the maximum sample size to less than 75%. Patients were divided into high-risk and low-risk groups, and the prognostic differences between the two groups were further analyzed using the survival function in R software. The log rank test method was used to evaluate the significance of the prognostic differences between the different groups of samples. The R package “pROC” was used for receiver operating characteristic (ROC) analysis to obtain the area under the curve.
Immune infiltration analysis and clinical analysis
Microenvironment cell population (MCP) counter analysis was used to evaluate the relative abundance of immune cell types in the high-risk and low-risk groups. The Estimation of Stromal and Immune Cells in Malignant Tumor Tissues Using Expression Data (ESTIMATE) algorithm was used to evaluate the immune score and matrix score between the high-risk and low-risk groups. The effectiveness of predictive models under different clinical conditions was evaluated by combining the clinical information and risk score of patients with LUAD.
Construction of the nomogram
Data including survival time, survival status, and clinical information were integrated by using the R software package “RMS”, and a nomogram was established using Cox analysis to evaluate the prognostic significance of these features in the sample.
Immunohistochemical verification in the HPA database
The protein expression levels of the differentially expressed genes (DEGs) in lung cancer samples were investigated by using the HPA database (https://www.proteinatlas.org/). The whole process of data analysis is shown in Figure 1.
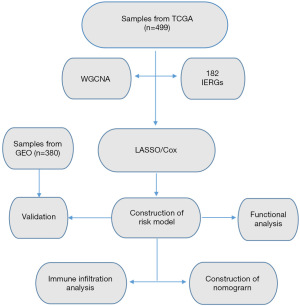
Validation of the prognostic risk models
The risk model was validated via the external datasets GSE72094 and GSE30219.
Statistical analysis
Various packages in R software were used for data analysis and graphics rendering: the “glmnet” package was used to conduct LASSO regression analysis, combining survival status, survival time, and gene expression data; the “survival” package was used to create a survival curve; the “survfit” survival function was used to investigate the differences in prognosis across groups; the “pROC” package was used for ROC analysis to obtain the AUC; and the “rms” package was used to establish the nomograms to assess the prognostic significance of several features in the samples. P<0.05 indicated a statistically significant difference.
Results
WGCNA and the screening of key genes
We conducted WGCNA using the top 25% of the variance expression profile in the TCGA-LUAD queue. The soft threshold in the queue was 3 (Figure 2A,2B). Subsequently, dynamic module identification was performed, with no fewer than 100 genes per module (Figure 2C), resulting in 24 coexpressed modules (Figure 2D). Twenty-four coexpression modules were clustered into cancer and noncancer groups, with the brown module having the strongest positive correlation with the cancer group score [correlation (Cor) =0.74; P=6.5e−99] and the red module having the strongest positive correlation with the noncancer group score (Cor=0.81; P=1.7e−132) (Figure 2E,2F). The brown and red modules were selected as the central modules. We then measured the GS and MM of each gene feature in the central module (Figure 2G,2H). Finally, with MM >0.6 and GS >0.6 as thresholds, the genes in the module were screened as potential key genes. The key genes were intersected with 182 immune escape genes to obtain 20 IERGs (Figure 2I).
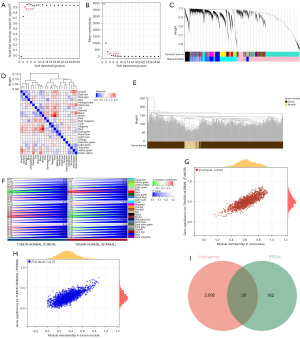
Functional enrichment analysis
GO enrichment analysis of 20 differentially expressed IERGs revealed that in biological processes (BP), IERGs mainly regulated immune system processes, immune responses, and interferon-γ enrichment in mediated signaling pathways, apoptotic signaling pathways, regulation of cellular communication, lymphocyte activation, and immune system process regulation, among others. In terms of molecular function (MF), IERGs appeared mainly enriched in cytokine binding, protein deacetylase activity, nucleosome DNA binding, deacetylase activity, nucleosome binding, ubiquitin binding, and inhibition of transcription factor binding. For cellular components (CC), IERGs were mainly enriched in protein-containing complexes, membrane protein complexes, transcriptional repressor complexes, and tumor necrosis factor receptor superfamily complexes (Figure 3A). KEGG enrichment analysis revealed that IERGs were closely related to T helper 17 cell (Th17) differentiation, necroptosis, human immunodeficiency virus type 1 infection, adipocyte cytokine signaling pathway, antigen processing and presentation, programmed cell death ligand 1 (PD-L1) expression and programmed cell death 1 (PD-1) pathway in tumors, cytokine-cytokine receptor interaction, Th1 and Th2 cell differentiation, and tumor necrosis factor signaling pathway (Figure 3B).
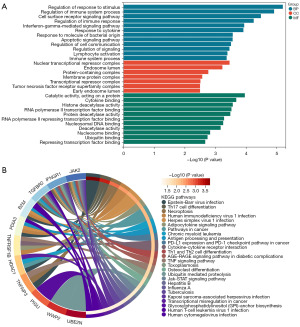
Construction of a risk model
We used the R software package “glmnet” to integrate survival data and gene expression data and identified 4 key prognostic genes from 20 IERGs using LASSO regression analysis. The lambda value was 0.04, and the model formula was as follows: risk score = 0.295 × AHSA1+0.111 × CEP55 – 0.029 × SUSD6 – 0.073 × WWP2 (Figure 4A,4B). By examining the relationships between various risk scores and patient survival times, survival outcomes, and changes in gene expression, we observed that when risk scores increase, patient survival rates sharply decline. The AHSA1 and CEP55 genes were both found to be risk factors, with their expression increasing with the increase of risk score (Figure 4C). Patients were categorized into high- and low-risk groups according to the best cutoff value of the risk score, and the prognostic differences between the two groups were examined. Ultimately, we observed significant prognostic differences (P<0.01), with the high-risk group having a shorter survival time (Figure 4D). To evaluate the risk score and the reliability of the model, time-dependent ROC curves were plotted, and the final AUC values for 1 year and 3 years were 0.70 and 0.63, respectively (Figure 4E).
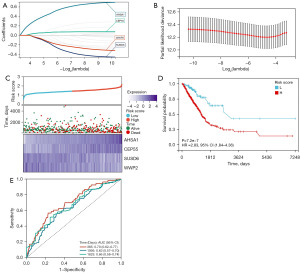
Immune infiltration analysis and clinical analysis
We subsequently conducted immune analysis to determine the differences in immune status between the high- and low-risk groups. The ESTIMATE analysis showed that the immune score, matrix score, and ESTIMATE score in the low-risk group were all significantly higher than those in the high-risk group (P<0.01) (Figure 5A). The MCP-counter immune infiltration algorithm analysis showed that the abundance of T lymphocytes, B cells, myeloid dendritic cells, neutrophils, and endothelial cells was significantly higher in the low-risk group than in the high-risk group (Figure 5B). These results indicate significant differences in immune status between the high- and low-risk groups. By matching the clinical information in the queue with the risk score, we found that the higher the risk score, the later the staging, while there was no correlation between age or gender and the risk score (Figure 5C-5E).
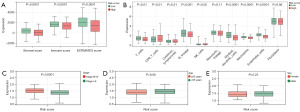
Construction and calibration of the nomogram
By combining the risk score with gender, age, and pathological staging, we constructed a nomogram and calibration curve for predicting prognosis, as shown in Figure 6A,6B. The survival prediction concordance index was 0.70 (95% CI: 0.654–0.743; P<0.01). According to the Kaplan-Meier curve in the column chart in Figure 6, they are significant prognostic differences between the groups (P<0.01), with the survival time of the high-risk group being shorter (Figure 6C). The time-dependent ROC curve indicates that the AUC values for 1 and 3 years are 0.76 and 0.73 (Figure 6D), suggesting that the comprehensive column chart can accurately predict the prognosis patients with LUAD.
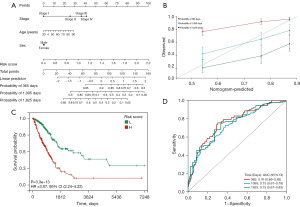
Immunohistochemical verification in the HPA database
The protein expression levels of the key prognostic genes in LUAD tissues was examined via the HPA database (https://www.proteinatlas.org/) (8). The immunohistochemical results showed that AHSA1 had a low expression in normal lung tissue and a high expression in lung cancer tissue (Figure 7A,7B), while CEP55 had a low expression in normal lung tissue and a high expression in lung cancer tissue (Figure 7C,7D).
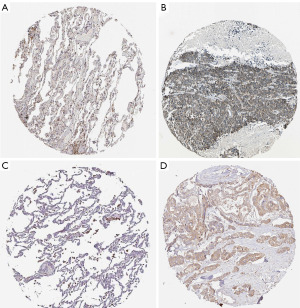
Validation of risk model
The risk model was validated using the GEO datasets GSE72094 and GSE30219, and the expression heatmaps of key genes, as well as the Kaplan-Meier survival curve and ROC curve, were obtained. The expression heatmaps were basically consistent with the gene expression of the model, and as the risk score increased, the survival time of patients in the validation queue decreased, and the ROC curve predicted the survival rate well (Figure 8A-8C).
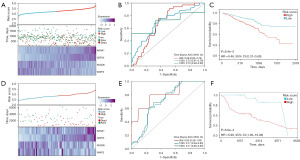
Discussion
An increasing number of studies are indicating that immune escape participates in each stage of LUAD development, promoting tumor progression to varying degrees. The immune system has a dual effect of both promoting and inhibiting tumors. In the early stages of cancer occurrence (i.e., during immune editing), the immune system is able to recognize and destroy tumor cells through the antigens they produce (9,10). However, due to genetic instability and the continuous division and mutation of tumor cells, tumor cells ultimately impair the immune system’s ability to eradicate them through immunosuppressive effects or loss of target antigen expression, resulting in immune escape and the worsening of clinical stage (11,12).
Immune checkpoints are substances or pathways that control the immune system’s activation and functionality. PD-1/PD-L1 is one of the key immune checkpoint pathways, and PD-1 and PD-L1 are proteins expressed on the surface of T cells and cancer cells, respectively (13). Han et al. found that their interaction prevents the activation and proliferation of T cells, allowing cancer cells to evade immune responses (14). Anti-PD-1/PD-L1 therapy, such as with that nivolumab or pembrolizumab, can suppress immune escape reactions by binding and inhibiting the interaction between PD-1 and PD-L1, thereby achieving the goal of treating tumors (15).
Li et al. found that N6 methyladenosine (m6A) promotes the expression of oncogenes by modifying and regulating RNA splicing, decay, nuclear export, stability, and translation, thereby inducing immune escape (16). M6A-related drugs such as m6A methyltransferases METTL3 and METTL14 exert anticancer activity in tumor therapy (17,18). The currently known immune escape mechanisms include CTL-associated antigen-4 (CTLA-4), immunoglobulin and ITIM domain (TIGIT), and T-cell immunoglobulin 3 (TIM-3) immune escape responses mediated by immune perception deficits via inhibitory immune checkpoint receptor (ICP-R) expression, such as that of V-domain immunoglobulin suppressor of T cell activation (VISTA) and lymphocyte activation genes (LAG-3) expressed on T cells and/or natural killer (NK) cells (19,20).
The tumor immunotherapy developed based on the immune escape-related mechanisms mentioned above can provide certain therapeutic effects in the field of solid cancers such as lung cancer. However, due to the intrinsic and/or acquired resistance mediated by tumor cell immune escape strategies, only a portion of patients can achieve sustained response rates. Thus, more investigation into the genes linked to immune escape in LUAD may aid in understanding the function of immune escape in the onset and progression of LUAD and offer prospective targets for lung cancer immunotherapy.
This study used bioinformatics methods to explore the significance of IERGs on the prognosis of patients with LUAD. We preliminarily screened 20 differentially expressed immune escape genes and used the LASSO Cox regression analysis to screen for core IERGs with independent prognostic relevance. A risk score prognostic model was constructed, and patients were divided into high- and low-risk groups based on the optimal cutoff value of the risk score. Survival analysis showed that patients in the low-risk group had better overall survival than those in the high-risk group in the TCGA patient cohort, which was confirmed in the GEO database. Clinical correlation analysis showed that the risk score was closely related to the gender, age, and pathological staging of patients with LUAD. A nomogram was created using the aforementioned risk factors in order to forecast the prognosis of patients with LUAD. The results showed an exceptional level of conformity with the patients’ actual survival rate. These findings demonstrate that the prognostic model performs well in terms of prediction for lung cancer populations with low overall survival.
Subsequently, we conducted GO analysis and KEGG analysis on the IERGs to further enrich their related pathways of action. We used ESTIMATE and MCP-counter methods to determine the immune status of the high-risk and low-risk groups. The immune score, matrix score, and ESTIMATE score in the low-risk group were significantly higher than those in the high-risk group. Finally, we conducted immunohistochemical analysis to further validate the low expression and high expression of two proteins in normal lung tissue and in lung cancer tissue, respectively, as identified by the LASSO regression analysis of LUAD tissue.
We found that the numerous genes used to create the risk model in this study are strongly associated with the onset and progression of cancers. CEP55, a member of the centrosome protein family and one of the expression products of immune escape–related genes, is an important factor in the regulation of mitosis and cytoplasmic division. Its main function is to anchor microtubules and polymerize related proteins, participate in the formation of spindles, and then regulate cell proliferation, with studies indicating that it is overexpressed in various tumors (21-23). Overexpression of CEP55 directly interacts with the p110 catalytic subunit of PI3K, which upregulates the PI3K/AKT pathway, leading to cell transformation, proliferation, epithelial-to-mesenchymal transition, invasion, and migration, thereby promoting cancer development (24,25). In addition, CEP55 has been shown to induce tumorigenesis by regulating the metaphase of meiotic oocytes and accelerating TRP53+/- overexpression (26,27). Therefore, CEP55 may become a predictive biomarker for tumor prognosis and a potential target for immunotherapy.
Luo et al. observed and validated the high expression of CEP55 in lung cancer cell lines through experiments conducted in non-small cell lung cancer (NSCLC) cells (23). They reported that targeting CEP55 with miR-195-5p can inhibit the proliferation of NSCLC cells and induce cell apoptosis. Li et al. found that miR-144-3p can also be used to inhibit the development of NSCLC by inhibiting CEP55 expression (28). CEP55 is also associated with tumors other than lung cancer. In breast cancer, CEP55 contributes to disrupting cell fate determination in aneuploid cells during mitosis, which can be targeted through MEK1/2-PLK1 inhibition. The progression of endometrial cancer (EC) is closely related to abnormal expression of CEP55, and downregulation of CEP55 expression can inhibit the proliferation, invasion, and migration of EC; delay cell cycle; and accelerate tumor cell apoptosis (29). The results of our study are consistent with previous research on CEP55, which is highly expressed in various tumors, including LUAD, and is a promising clinical target for cancer treatment. However, its therapeutic effect and mechanism still require further investigation.
Hsp90 is involved in several metabolic and developmental processes of tumor cells. AHSA1 is the major activator of Hsp90 ATPase and it critical to regulating the molecular chaperone cycle and protein folding of Hsp90 (30,31). As another important immune escape gene expression product, AHSA1 has been observed to be upregulated in many tumor tissues, and its aberrant expression may be related to the occurrence and metastasis of tumors (10). In this study, KEGG enrichment analysis showed that AHSA1 is closely related to the expression of PD-L1 in tumors, the PD-1 pathway, Th1 and Th2 cell differentiation, and the tumor necrosis factor signaling pathway. This also suggests that AHSA1 may figure prominently in tumor occurrence and prognosis. Zhang et al. reported that AHSA1 is highly expressed in LUAD and is associated with poor prognosis, which is consistent with the results of our study (32). In addition, the upregulation of AHSA1 expression is also associated with the development of hepatocellular carcinoma; it has been shown to promote the proliferation and metastasis of hepatocellular carcinoma in vivo by recruiting ERK1/2 and contributing to the phosphorylation and inactivation of CALD1, which is associated with poor prognosis in those with hepatocellular carcinoma (33). Previous studies have shown that CD8+ T cells are one of key combatants of tumor cells (34-36). However, when infiltrating cancer tissues, they are generally in a dysfunctional state, which known as T-cell exhaustion. The results of immune infiltration algorithm analysis in our study (Figure 5B) revealed that CD8+ T-cell exhaustion in the high-risk group (i.e., the group in which CEP55 and AHSA1 were upregulated) was higher than that in the low-risk group, indicating that the CEP55- and AHSA1-positive expression may induce the body to produce more CD8+T cells to fight against tumor cells. Meanwhile, the frequent expression of inhibitory receptors such as PD-1 on depleted CD8+T cells and higher CD8+ T-cell exhaustion in LUAD may suggest that combined PD-1/PD-L1 therapy confers superior antitumor effects, but this needs to be examined in further clinical or in vitro trials (37).
The LASSO regression analysis in our study further showed that the expression levels of SUSD6 and WWP2 in LUAD tissues were lower than those in normal adjacent tissues, indicating that inhibiting their expression may enhance the treatment effect on LUAD. However, there is a dearth of evidence regarding their roles and mechanisms in the occurrence and development of LUAD and other malignant tumors. We therefore did not focus on SUSD6 and WWP2 in this study. Subsequent in vitro experiments and other research should be completed to ascertain the potential value of precision LUAD treatment.
In recent years, with the innovation of emerging molecular targeted therapies and the use of conventional chemotherapy and radiotherapy, the prognosis of patients with LUAD has improved, but their prognosis is still poor. Therefore, it is crucial to study the key biomarkers of immunotherapy and provide precise, efficient, and personalized treatment. In this study, we sought to clarify the role of immune escape–related mechanisms in the occurrence, development, and treatment of LUAD by focusing on the expression of IERGs in patients with LUAD. First, through consensus clustering, two molecular subgroups with significant differences in prognosis and immune status were identified, and the differences in immune status between different subgroups were analyzed. Second, based on the clustering results, we examined the related biological mechanisms and partially clarified the potential mechanisms. Finally, the association of the identified IERGs on prognosis was determined. Our findings may help to improve the prognosis of patients with LUAD by advancing the development of immunotherapy.
Conclusions
Our results suggest that the prognosis of LUAD is closely related to the DEGS of tumor immune escape. Based on these genes, we developed a four-gene model related to LUAD prognosis and established a clinical prognosis prediction column chart. The genes in the model may be viable targets for the immunotherapy of LUAD and aid in individualized treatment. However, this study has a few limitations, including the small number of samples used in the analysis and the lack of in vivo and in vitro experiments to verify the accuracy of its predictions. In future studies, larger sample sizes should be used, findings should be verified by clinical experiments, and biological functions and mechanisms of action should be further explored to better guide clinical practice.
Acknowledgments
Funding: None.
Footnote
Reporting Checklist: The authors have completed the TRIPOD reporting checklist. Available at https://tcr.amegroups.com/article/view/10.21037/tcr-23-2295/rc
Peer Review File: Available at https://tcr.amegroups.com/article/view/10.21037/tcr-23-2295/prf
Conflicts of Interest: All authors have completed the ICMJE uniform disclosure form (available at https://tcr.amegroups.com/article/view/10.21037/tcr-23-2295/coif). The authors have no conflicts of interest to declare.
Ethical Statement: The authors are accountable for all aspects of the work in ensuring that questions related to the accuracy or integrity of any part of the work are appropriately investigated and resolved. This study was conducted in accordance with the Declaration of Helsinki (as revised in 2013).
Open Access Statement: This is an Open Access article distributed in accordance with the Creative Commons Attribution-NonCommercial-NoDerivs 4.0 International License (CC BY-NC-ND 4.0), which permits the non-commercial replication and distribution of the article with the strict proviso that no changes or edits are made and the original work is properly cited (including links to both the formal publication through the relevant DOI and the license). See: https://creativecommons.org/licenses/by-nc-nd/4.0/.
References
- Herbst RS, Morgensztern D, Boshoff C. The biology and management of non-small cell lung cancer. Nature 2018;553:446-54. [Crossref] [PubMed]
- Yu B, Li T, Chen J, et al. Identification of activated pathways in lung adenocarcinoma based on network strategy. J Cancer Res Ther 2020;16:793-9. [Crossref] [PubMed]
- National Lung Screening Trial Research Team. Reduced lung-cancer mortality with low-dose computed tomographic screening. N Engl J Med 2011;365:395-409. [Crossref] [PubMed]
- Feng W, He Z, Shi L, et al. Significance of CD80 as a Prognostic and Immunotherapeutic Biomarker in Lung Adenocarcinoma. Biochem Genet 2023;61:1937-66. [Crossref] [PubMed]
- Yang CY, Yang JC, Yang PC. Precision Management of Advanced Non-Small Cell Lung Cancer. Annu Rev Med 2020;71:117-36. [Crossref] [PubMed]
- Anichini A, Perotti VE, Sgambelluri F, et al. Immune Escape Mechanisms in Non Small Cell Lung Cancer. Cancers (Basel) 2020;12:3605. [Crossref] [PubMed]
- Lawson KA, Sousa CM, Zhang X, et al. Functional genomic landscape of cancer-intrinsic evasion of killing by T cells. Nature 2020;586:120-6. [Crossref] [PubMed]
- Thul PJ, Lindskog C. The human protein atlas: A spatial map of the human proteome. Protein Sci 2018;27:233-44. [Crossref] [PubMed]
- Dunn GP, Bruce AT, Ikeda H, et al. Cancer immunoediting: from immunosurveillance to tumor escape. Nat Immunol 2002;3:991-8. [Crossref] [PubMed]
- Dunn GP, Old LJ, Schreiber RD. The three Es of cancer immunoediting. Annu Rev Immunol 2004;22:329-60. [Crossref] [PubMed]
- Swann JB, Smyth MJ. Immune surveillance of tumors. J Clin Invest 2007;117:1137-46. [Crossref] [PubMed]
- Shay JW, Roninson IB. Hallmarks of senescence in carcinogenesis and cancer therapy. Oncogene 2004;23:2919-33. [Crossref] [PubMed]
- Kallingal A, Olszewski M, Maciejewska N, et al. Cancer immune escape: the role of antigen presentation machinery. J Cancer Res Clin Oncol 2023;149:8131-41. [Crossref] [PubMed]
- Han Y, Liu D, Li L. PD-1/PD-L1 pathway: current researches in cancer. Am J Cancer Res 2020;10:727-42. [PubMed]
- Fessas P, Lee H, Ikemizu S, et al. A molecular and preclinical comparison of the PD-1-targeted T-cell checkpoint inhibitors nivolumab and pembrolizumab. Semin Oncol 2017;44:136-40. [Crossref] [PubMed]
- Li W, Hao Y, Zhang X, et al. Targeting RNA N(6)-methyladenosine modification: a precise weapon in overcoming tumor immune escape. Mol Cancer 2022;21:176. [Crossref] [PubMed]
- Yin H, Zhang X, Yang P, et al. RNA m6A methylation orchestrates cancer growth and metastasis via macrophage reprogramming. Nat Commun 2021;12:1394. [Crossref] [PubMed]
- Dong L, Chen C, Zhang Y, et al. The loss of RNA N(6)-adenosine methyltransferase Mettl14 in tumor-associated macrophages promotes CD8(+) T cell dysfunction and tumor growth. Cancer Cell 2021;39:945-957.e10. [Crossref] [PubMed]
- Joller N, Kuchroo VK. Tim-3, Lag-3, and TIGIT. Curr Top Microbiol Immunol 2017;410:127-56. [Crossref] [PubMed]
- Lee JB, Ha SJ, Kim HR. Clinical Insights Into Novel Immune Checkpoint Inhibitors. Front Pharmacol 2021;12:681320. [Crossref] [PubMed]
- Fabbro M, Zhou BB, Takahashi M, et al. Cdk1/Erk2- and Plk1-dependent phosphorylation of a centrosome protein, Cep55, is required for its recruitment to midbody and cytokinesis. Dev Cell 2005;9:477-88. [Crossref] [PubMed]
- Li GS, Zhang W, Huang WY, et al. CEP55: an immune-related predictive and prognostic molecular biomarker for multiple cancers. BMC Pulm Med 2023;23:166. [Crossref] [PubMed]
- Luo J, Pan J, Jin Y, et al. MiR-195-5p Inhibits Proliferation and Induces Apoptosis of Non-Small Cell Lung Cancer Cells by Targeting CEP55. Onco Targets Ther 2019;12:11465-74. [Crossref] [PubMed]
- Chen CH, Lu PJ, Chen YC, et al. FLJ10540-elicited cell transformation is through the activation of PI3-kinase/AKT pathway. Oncogene 2007;26:4272-83. [Crossref] [PubMed]
- Chen CH, Lai JM, Chou TY, et al. VEGFA upregulates FLJ10540 and modulates migration and invasion of lung cancer via PI3K/AKT pathway. PLoS One 2009;4:e5052. [Crossref] [PubMed]
- Zhou C, Hancock JL, Khanna KK, et al. First meiotic anaphase requires Cep55-dependent inhibitory cyclin-dependent kinase 1 phosphorylation. J Cell Sci 2019;132:jcs233379. [Crossref] [PubMed]
- Sinha D, Nag P, Nanayakkara D, et al. Cep55 overexpression promotes genomic instability and tumorigenesis in mice. Commun Biol 2020;3:593. [Crossref] [PubMed]
- Li M, Liu Y, Jiang X, et al. Inhibition of miR-144-3p exacerbates non-small cell lung cancer progression by targeting CEP55. Acta Biochim Biophys Sin (Shanghai) 2021;53:1398-407. [Crossref] [PubMed]
- Zhang X, Xu Q, Li E, et al. CEP55 predicts the poor prognosis and promotes tumorigenesis in endometrial cancer by regulating the Foxo1 signaling. Mol Cell Biochem 2023;478:1561-71. [Crossref] [PubMed]
- Li W, Liu J. The Prognostic and Immunotherapeutic Significance of AHSA1 in Pan-Cancer, and Its Relationship With the Proliferation and Metastasis of Hepatocellular Carcinoma. Front Immunol 2022;13:845585. [Crossref] [PubMed]
- Xu W, Beebe K, Chavez JD, et al. Hsp90 middle domain phosphorylation initiates a complex conformational program to recruit the ATPase-stimulating cochaperone Aha1. Nat Commun 2019;10:2574. [Crossref] [PubMed]
- Zhang P, Pei S, Gong Z, et al. The integrated single-cell analysis developed a lactate metabolism-driven signature to improve outcomes and immunotherapy in lung adenocarcinoma. Front Endocrinol (Lausanne) 2023;14:1154410. [Crossref] [PubMed]
- Zhang J, Ren Z, Zheng D, et al. AHSA1 Promotes Proliferation and EMT by Regulating ERK/CALD1 Axis in Hepatocellular Carcinoma. Cancers (Basel) 2022;14:4600. [Crossref] [PubMed]
- Šustić M, Cokarić Brdovčak M, Krmpotić A, et al. CD8 T Cell Vaccines and a Cytomegalovirus-Based Vector Approach. Life (Basel) 2021;11:1097. [Crossref] [PubMed]
- Chen SW, Zhu SQ, Pei X, et al. Cancer cell-derived exosomal circUSP7 induces CD8(+) T cell dysfunction and anti-PD1 resistance by regulating the miR-934/SHP2 axis in NSCLC. Mol Cancer 2021;20:144. [Crossref] [PubMed]
- Huang Y, Jia A, Wang Y, et al. CD8(+) T cell exhaustion in anti-tumour immunity: The new insights for cancer immunotherapy. Immunology 2023;168:30-48. [Crossref] [PubMed]
- He QF, Xu Y, Li J, et al. CD8+ T-cell exhaustion in cancer: mechanisms and new area for cancer immunotherapy. Brief Funct Genomics 2019;18:99-106. [Crossref] [PubMed]