Combining non-invasive liquid biopsy and a methylation analysis to assess surgical risk for early esophageal cancer
Highlight box
Key findings
• Non-invasive liquid biopsy techniques and a methylation analysis can be combined to assess the surgical risk of early esophageal cancer (ESCA) patients.
What is known and what is new?
• Circulating-free DNA (cfDNA) methylation has been widely used in early lung, colorectal and breast cancer screening.
• The model established in this study was able to differentiate between early ESCA and esophageal intraepithelial neoplasia with versatility and accuracy.
What is the implication, and what should change now?
• cfDNA methylation detection has been widely used in modern tumor screening. Meanwhile, a methylation analysis provides key insights into tumor status and progression. In this study, we combined these two approaches to assess the risk of patients with early-stage ESCA prior to endoscopic submucosal dissection and found the model to be effective and promising. The model may be refined and extended in the future.
Introduction
Esophageal cancer (ESCA) is a widespread malignant neoplasm worldwide, with a particularly high prevalence in East Asia, notably in regions such as Henan and Fujian in China, where esophageal squamous cell carcinoma is common (1-3). Despite notable advancements in human epidermal growth factor receptor 2-targeted therapy and immune checkpoint inhibitors, which have substantially extended the lifespan of certain esophageal squamous cell carcinoma patients, whereas ESCA patients continue to have poorer overall survival (OS) due to the fact that the majority of patients are invariably diagnosed at an advanced stage (4,5).
In China, a comprehensive strategy of early screening for ESCA, coupled with the widespread adoption of endoscopic submucosal dissection (ESD), has yielded a significant reduction in the incidence of ESCA (6,7). However, ESD has limited ability to strip deeply infiltrating esophageal lesions, which leads to a considerable risk of intraoperative perforation. Consequently, there is an urgent need to establish a new method to assess the surgical risk of ESD in patients before surgery (8,9).
Circulating-free DNA (cfDNA), originating from apoptotic cells, includes circulating tumor DNA released by tumor cells. cfDNA is extensively used in liquid biopsies, and plays a pivotal role in the screening, drug selection, and prognosis prediction of patients with various cancers, particularly lung and colorectal cancer. DNA methylation, a fundamental mechanism in gene expression regulation and genomic stability maintenance, also plays a critical role in gene expression regulation and genomic stability maintenance (10-12). Research suggests that alterations in genomic methylation patterns, including the increased methylation of tumor suppressor gene expression and the decreased methylation of oncogenes, manifest early in the carcinogenic process. Alterations in genomic methylation patterns promotes the expression of oncogenes, leading to genomic instability (13,14).
Circulation-free DNA (cfDNA) methylation testing has been widely used in the early screening of cancers such as lung, colorectal, and breast cancers, and has been proven to be reliable and effective (15-17). The aim of this study was to assess the extent of tumor infiltration by identifying specific cfDNA methylation markers and thus to establish a systematic framework for assessing the risk of preoperative perforation in ESD. These markers could provide valuable insights into the risks associated with ESD surgery. Any such approach would significantly enhance the assessment of patient risks before they undergo ESD procedures. We present this article in accordance with the TRIPOD reporting checklist (available at https://tcr.amegroups.com/article/view/10.21037/tcr-24-314/rc).
Methods
Analyzing genes for progression-free survival (PFS) in ESCA using The Cancer Genome Atlas (TCGA) database
To investigate the genes related to PFS in ESCA, we obtained RNA-sequencing data (level 3) for 42 stage I to IIA tumors from TCGA database (available at https://portal.gdc.cancer.gov/). The data set included corresponding clinical information. We conducted an extensive analysis of messenger RNA (mRNA) differential expression using the Limma package (version: 3.40.2, Lucent Technologies, USA) in R software. During the analysis, we made adjustments to TCGA or Genotype-Tissue Expression (GTEx) data sets and applied adjusted P values to correct for potential false-positive results. Specifically, we selected genes using the following criteria: adjusted P<0.05, and log2 (fold change) >1 or log2 (fold change) <−1.
Gene Ontology (GO) and Kyoto Encyclopedia of Genes and Genomes (KEGG) enrichment analysis of the potential target genes
To gain further insights into the biological functions of the potential target genes, we performed a functional enrichment analysis. We used the GO tool to annotate the functional attributes of genes, including their molecular functions, biological processes (BPs), and cellular components. Additionally, we conducted a KEGG enrichment analysis to elucidate the functions of the genes and obtain advanced genomic functional information. When exploring the oncogenic roles of these target genes, we employed the ClusterProfiler package in R (Lucent Technologies) to perform the GO functional and KEGG pathways enrichment analysis of potential mRNAs (18-20).
For the Kaplan-Meier survival analysis of 182 ESCA patients from TCGA data set, we conducted log-rank tests and a univariate Cox proportional hazards regression analysis. This analysis provided the hazard ratios along with the P values and 95% confidence intervals (CIs). These analytical methods were executed using R software version 4.0.3 (R Foundation for Statistical Computing, 2020). A significance threshold of P<0.05 was considered statistically significant (18).
Analyzing methylation site differences in ESCA genes using Shiny Methylation Analysis Resource Tool (SMART)
To analyze the differences in the methylation sites, we conducted an analysis to identify differentially expressed genes (DEGs) by intersecting those significantly associated with PFS and those displaying either up-regulation or down-regulation compared to normal genes (sub G1 phase/normal). Subsequently, we employed the SMART to examine these genes, examining the interactions among cytosine-phosphate-guanine (CpG) island methylation levels, transcript expression, and survival duration. This investigative effort improved our understanding of the mechanisms underlying ESCA development. It may also lead to the development of tailored therapeutic approaches and more precise survival predictions (21-23).
Sample collection and ethical considerations
This study was approved by the Ethics Committee of Henan Province Hospital of Traditional Chinese Medicine, which is the Second Affiliated Hospital of Henan University of Chinese Medicine (ethics number: HNSZYY20210108). A total of 73 formalin-fixed paraffin-embedded (FFPE) samples used for validation were provided by the Pathology Department and Clinical Sample Repository of Henan Province Hospital of Traditional Chinese Medicine. These samples were collected post-ESD surgery and informed consent was obtained from the patients prior to surgery. All the patients who participated in the study signed informed consent forms. Blood samples were obtained from all patients undergoing ESD before the procedure, and plasma was separated and stored. The study was conducted in accordance with the Declaration of Helsinki (as revised in 2013).
The study collected a total of 169 plasma samples, comprising 163 blood samples from ESD patients and six control samples from asymptomatic individuals. Blood (3 mL) was collected 12 hours before surgery and stored at room temperature in cfDNA blood collection tubes (BCTs) (Cat#218962, Streck, USA). Plasma (with no apparent hemolysis) was separated from the blood within 48 hours of collection and stored at −80 ℃ until DNA isolation. For asymptomatic normal participants, 3–5 mL of blood was drawn using BD Vacutainer ethylenediaminetetraacetic acid (EDTA) tubes (SKU/REF368661, Becton Dickinson, USA). Plasma was immediately separated within 2 hours of blood collection and stored at −80 ℃.
Methylation-specific quantitative polymerase chain reaction (MS-qPCR)
DNA extraction and bisulfite treatment: DNA from the FFPE samples was extracted using the TianGen FFPE sample DNA extraction kit (DP340, TianGen, China), while cfDNA from the plasma was isolated using the Qiagen Circulating Nucleic Acid Kit (Cat#55114, Qiagen, Germany). The bisulfite conversion was performed using the TianGen bisulfite conversion kit (DP215-02, TianGen).
Around 130 µL of the lightning conversion reagent was added to 20 µL of the DNA sample and incubated in a thermal cycler as follows: incubation at 98 ℃ for 8 minutes, followed by incubation for 60 minutes at 54 ℃, and a final incubation at 4 ℃ for 20 hours. Subsequently, the bisulfite-converted DNA was mixed with M-binding buffer, and desulfurization, washing, and elution were carried out using the Zymo-Spin column (Cat#C1003-250, Zymo Research, USA) with 17 µL of M-elution buffer (24,25).
Fluorescence quantitative PCR detection: for cfDNA samples ranging from 10 to 20 ng, an amplification phase was carried out using a primer pool, starting with 3-minute incubation at 95 ℃, followed by 8 cycles of 30 seconds at 95 ℃ and 60 seconds at 56 ℃. The ProFlexTM PCR System from Thermo Fisher (ProFlexTM 2 × 96 well, Thermo Fisher, USA) was employed for this process. The amplified products were then divided for quantitative PCR using the standard procedure with Luna® Universal Probe qPCR Master Mix (M3004L, NEB, USA) in an ABI 7500 Real-Time PCR thermal cycler (Thermo Fisher, USA). The reference gene ACTB was used as the experimental quality control gene (26,27). The primers used in the study are listed in Table 1.
Table 1
Oligo name | Oligo sequence (5'–3') |
---|---|
cg01691856 | |
Fwd primer | GCCAGAGGTTGGAGACTC |
Rev primer | CTGCCCTTAGTGACAAAGC |
Probe | FAM-AGGACACTTCCCTGGGCTCCGGAT-BHQ1 |
cg01725199 | |
Fwd primer | GATGCAAGTCTCAGCCAGC |
Rev primer | ACTGCAGCCAGCGATCTG |
Probe | FAM-TGAAGACCCTGCCGAAATGAGCCAC-BHQ1 |
cg08104146 | |
Fwd primer | CCACGGCTATGAGAAATG |
Rev primer | GCTCCAGAATTGCTATCC |
Probe | FAM-CCACAGATTGTTAATGTTTACAGGA-BHQ1 |
cg11871280 | |
Fwd primer | GAAGCACATAGCTGGAATG |
Rev primer | GCCATACACTTCCTACCA |
Probe | FAM-CAAGTGACCATGAGGAAAGCC-BHQ1 |
cg25742394 | |
Fwd primer | CAGGGTTTCACCATGTTG |
Rev primer | GTGCTAATTTGTTGTCATTCAA |
Probe | FAM-AACTCGTGACCTCAAGTGATCCA-BHQ1 |
cg26090940 | |
Fwd primer | CCACTTTAGGACCCTATC |
Rev primer | GCTAGAAAGAGATGAAGAAC |
Probe | FAM-TGACCAGCCTTGTTGGAACT-BHQ1 |
Fwd, forward; Rev, reverse.
Development of the LunaCAM classifier
To construct the LunaCAM classifier, a stepwise approach was employed to test and rank markers using the area under the curve (AUC) as the criterion. This process was conducted using the logistic regression method in Python (version 3.8.5, open-source machine) and the scikit-learn package (version 0.23.2, open-source machine). The marker with the highest AUC was selected as the anchor marker, and combinations with the remaining markers were assessed using 10×4-fold cross-validations for each addition. The final model with the most optimized combination was determined based on the highest AUC observed during the cross-validation process (27).
Statistical analysis
The survival analysis was performed using the Kaplan-Meier method, including the log-rank test, with the aid of GraphPad Prism (version 9.0, GraphPad Software, USA). Fisher’s least significant difference procedure was used to compare methylation levels across the various sample groups. Fisher’s exact test was then employed to compare the sensitivity between the MS-qPCR and Single-Entity Profiling by Transcriptional (SEPT) assays. Additionally, a Spearman’s rank correlation analysis was conducted to compare the median variant allele frequency as quantified using TCGA clinical data and MS-qPCR values. All the analysis methods and R packages were implemented by R (version 4.0.3, Foundation for Statistical Computing 2020, Lucent Technologies). A P value <0.05 (two-sided) was considered statistically significant (28,29).
Results
DEGs and PFS-related genes in early stage ESCA
We conducted a comprehensive analysis of TCGA data from 42 patients diagnosed with early stage ESCA (stage I–IIA) and 17 control samples (Figure 1). The primary objective of the study was to identify the molecular mechanisms and potential therapeutic targets associated with early stage ESCA. The analysis of the expression profiles of these squamous ESCA patients revealed that 1,263 genes were up-regulated, while 460 genes were down-regulated. This differential genomic landscape provided valuable insights into the molecular characteristics of early stage ESCA (Figure 2A,2B).
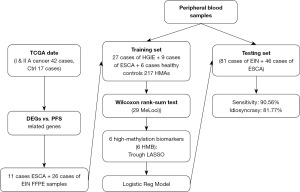
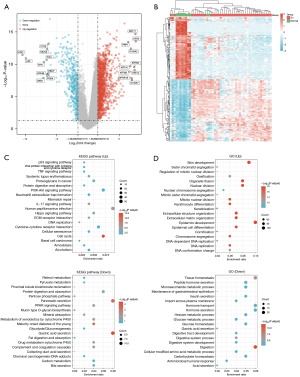
To obtain a deeper understanding of the functional roles of these DEGs, we conducted GO and KEGG pathway analyses (Figure 2C,2D). The results of the GO analysis highlighted that the up-regulated genes primarily participated in crucial BPs, such as cell cycle regulation, DNA repair, and apoptosis, all of which play pivotal roles in tumor development and progression. Conversely, the down-regulated genes were significantly enriched in metabolic pathways, immune responses, and signaling pathways. Additionally, the results of the KEGG pathway analysis indicated that up-regulated genes were predominantly associated with the pathways related to tumor genesis, including the p53 signaling pathway, cell cycle pathway, and cancer pathway, which highlights their central role in the pathophysiological process of early ESCA. Conversely, the down-regulated genes were implicated in various immune and metabolic pathways, revealing that they may play a role in tumor immune evasion and metabolic abnormalities.
Our study also explored the genes associated with PFS among ESCA patients in TCGA database. The preliminary findings indicated that a total of 216 genes were correlated with PFS (Figure 3). Subsequently, we cross-referenced these genes with the previously identified up-regulated and down-regulated genes, and ultimately identified 38 DEGs linked to PFS (Figure 4A).
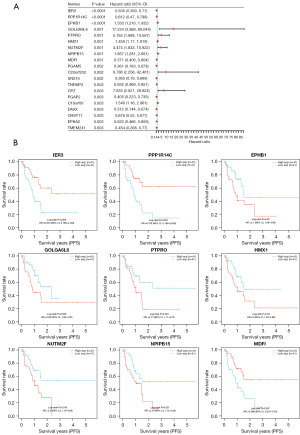
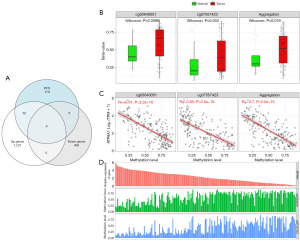
Screening early methylation markers in ESCA
The identification of these 38 DEGs provided valuable insights into the disease progression of ESCA patients. To gain a more comprehensive understanding of the characteristics of these DEGs, we used the SMART to conduct an in-depth investigation with a primary focus on the differential levels of the methylation sites in these genes. Encouragingly, our SMART analysis results revealed that the levels of hyper-methylated blocks (HMBs) were inversely correlated with the expression levels of both the genes themselves and the associated transcripts (Figure 4B-4D). This discovery underscores the pivotal role of methylation in gene expression regulation, particularly in the context of PFS and OS among ESCA patients. These differentially methylated sites may ultimately affect patient survival by suppressing or silencing the expression of these genes.
Using the SMART analysis method, we successfully identified 217 HMB regions in 38 genes. These regions can serve as potential early markers for ESCA occurrence. To validate the reliability of these potential markers, we conducted validation experiments using 73 FFPE tissue samples that had undergone prior validation. The results indicated that only 29 HMBs were detected in accordance with our predictions (for further details, see Table 2). This suggests a correlation between the methylation levels of these 29 loci and the early occurrence of ESCA in these samples. Further, to assess the potential utility of these markers in diverse clinical scenarios, we performed validation tests on cfDNA from 42 serum samples. Due to considerations related to sensitivity and the sample characteristics, only six of the HMBs passed the validation test (Table 3).
Table 2
HMBs locus | FFPE | cfDNA | |||
---|---|---|---|---|---|
SA (%) | Δct (mean ± SEM) | SA (%) | Δct (mean ± SEM) | ||
cg00153693 | 100.00 | 9.59±4.31 | 76 | 15.52±3.44 | |
cg00178984 | 100.00 | 12.11±3.17 | 62 | 10.05±4.15 | |
cg00260201 | 100.00 | 6.58±3.84 | 55 | 15.05±3.78 | |
cg00293940 | 100.00 | 8.46±3.55 | 79 | 14.74±5.02 | |
cg00424800 | 100.00 | 6.94±2.69 | 60 | 13.94±3.19 | |
cg01691856 | 100.00 | 8.16±2.83 | 100.00 | 15.84±4.23 | |
cg01725199 | 100.00 | 7.15±3.04 | 100.00 | 11.11±3.72 | |
cg04468551 | 100.00 | 7.3±2.98 | 64 | 10.41±2.46 | |
cg04511702 | 100.00 | 7.53±4.08 | 83 | 14.15±2.89 | |
cg04747517 | 100.00 | 7.07±2.36 | 71 | 12.84±3.79 | |
cg05099221 | 100.00 | 8.07±2.97 | 60 | 13.33±2.65 | |
cg06233073 | 100.00 | 12.67±3.13 | 76 | 12.45±3.81 | |
cg08104146 | 100.00 | 9.9±4.12 | 100.00 | 15.51±4.58 | |
cg08936516 | 100.00 | 6.83±3.29 | 74 | 11.91±4.17 | |
cg10812247 | 100.00 | 11.68±3.43 | 67 | 12.23±4.26 | |
cg11871280 | 100.00 | 10.71±2.91 | 98 | 10.34±3.93 | |
cg13480157 | 100.00 | 9.16±3.76 | 60 | 12.79±4.35 | |
cg14168790 | 100.00 | 11.75±3.62 | 57 | 13.33±5.17 | |
cg14352586 | 100.00 | 8.97±3.41 | 50 | 15.11±3.82 | |
cg14372792 | 100.00 | 7.69±4.13 | 74 | 12.17±3.98 | |
cg14400354 | 100.00 | 8.3±2.09 | 62 | 15.22±5.42 | |
cg16101148 | 100.00 | 9.33±2.85 | 81 | 13.76±3.31 | |
cg16532606 | 100.00 | 12.27±3.57 | 62 | 11.13±4.42 | |
cg21826946 | 100.00 | 10.74±4.43 | 88 | 12.45±4.74 | |
cg22447839 | 100.00 | 6.69±3.05 | 83 | 11.24±3.54 | |
cg22680075 | 100.00 | 8.44±2.49 | 62 | 12.36±2.99 | |
cg22684443 | 100.00 | 7.01±2.57 | 69 | 13.15±5.34 | |
cg25742394 | 100.00 | 12.57±3.93 | 100.00 | 14.56±4.92 | |
cg26090940 | 100.00 | 11.11±4.18 | 98 | 15.32±4.33 |
HMBs, hyper-methylated blocks; FFPE, formalin-fixed paraffin-embedded; SA, successful amplification; Δct, Δcycle threshold; SEM, standard error of the mean; cfDNA, circulating-free DNA.
Table 3
HMBs locus | Pathology | cfDNA | |||||
---|---|---|---|---|---|---|---|
EIN | ESCA | EIN | ESCA | False positives | False negatives | ||
cg01691856 | 81 | 46 | 57 | 38 | 9 | 23 | |
cg01725199 | 81 | 46 | 71 | 29 | 13 | 14 | |
cg08104146 | 81 | 46 | 65 | 46 | 15 | 1 | |
cg11871280 | 81 | 46 | 58 | 38 | 11 | 20 | |
cg25742394 | 81 | 46 | 69 | 41 | 8 | 9 | |
cg26090940 | 81 | 46 | 73 | 38 | 3 | 13 | |
6 in 1 | 81 | 46 | 78 | 44 | 3 | 2 |
HMBs, hyper-methylated blocks; EIN, esophageal intraepithelial neoplasia; ESCA, early esophageal cancer; cfDNA, circulating-free DNA.
Prediction using cfDNA for early ESCA detection
Given that different clinical applications have distinct criteria for sensitivity and specificity, we selected a population in which LunaCAM was used for the early detection of ESCA lesions. Our study encompassed several critical phases, including model construction, robustness assessment, and estimation. These steps were executed with the help of a highly reliable 10-fold, four-fold cross-validation methodology, and we meticulously recorded and calculated the average scores for each cross-validation. The outcomes were indeed promising, providing support for our study hypothesis. Specifically, the LunaCAM model exhibited outstanding performance in discriminating between early ESCA and early intra-esophageal intraepithelial neoplasia (EIN). According to our data, the model achieved a notably high AUC of 0.89, which indicates its exceptional diagnostic potential and accuracy.
To further substantiate our findings, we applied these classifiers to an additional, independent validation set (Figure 5). We performed this validation to confirm the robustness and reproducibility of the LunaCAM model across various data sets and cases. This meticulous validation process was pivotal in ensuring the reliability of the study results and offers robust support for future clinical applications.
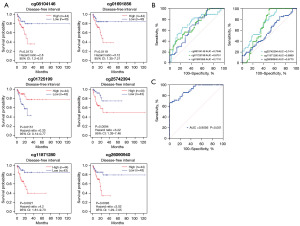
Using the SMART analysis method, we conducted a comprehensive investigation into the relationship between these six HMBs and their prognostic relevance in ESCA (Figure 5A). Subsequently, we used a validation set that included 127 serum samples to validate the accuracy of the evaluation system. The evaluation method for the validation set included preoperative blood collection for the initial assessment, with pathology reports from postoperative samples obtained from ESD serving as the gold standard for determination.
Comprehensive assessment and performance improvement of HMBs in early ESCA detection
Initially, we assessed the performance of each HMB individually to investigate the independent contribution of each highly methylated site to early cancer detection. However, the results revealed that the independent predictive ability of individual HMBs while valuable, did not have a high level of accuracy (Figure 5B).
To address this issue, we conducted another integrated assessment of these six HMBs, considering them as a collective rating system. This integrated assessment method was designed to ascertain whether their combination could complement each other, thereby enhancing their overall performance. Within this rating system, we obtained encouraging results. This combined strategy significantly improved the predictive performance of the model, positioning it as a key component in the diagnosis and prediction of early cancerous lesions. Notably, using a combination of these six HMBs for prediction, we achieved excellent sensitivity of 90.56% (Figure 5C). This discovery highlights the significance of a combinatorial assessment, particularly when multiple biomarkers are involved, as their synergy can significantly enhance the overall performance.
Discussion
In modern tumor screening, non-invasive liquid biopsy techniques, particularly cfDNA non-invasive testing, have gained widespread application (30). Additionally, a methylation analysis is a unique approach that provides critical insights into tumor status and progression through the examination of DNA methylation status (31). The primary objective of this study was to explore the potential of combining these two methodologies to assess the risk of patients with early stage ESCA prior to ESD (32).
This study used data from TCGA database to analyze gene expression disparities between stage I squamous ESCA samples and healthy samples to identify the genes associated with ESCA. Additionally, the genes linked with PFS in ESCA patients were identified, which laid the foundation for the subsequent methylation analysis. A methylation analysis of the methylation sites of these genes was also conducted, employing sophisticated analytical tools like the SMART. The inverse correlation between the HMBs and both gene expression levels and associated transcripts highlights the potential effect of methylation on PFS and OS as shown in other studies (33,34). The identification of 217 HMB regions associated with 38 genes offers a promising set of early markers for ESCA occurrence, DNA methylation potentially influencing patient survival by modulating gene expression. The results of the validation experiments, particularly those that used FFPE tissue samples and cfDNA from serum samples, support the reliability of these methylation markers as reported in another study (35). It is essential, however, to underscore the importance of scrutinizing sensitivity and sample characteristics during the validation process to ensure the practical applicability of these findings in a clinical setting.
The stability of these methylation sites under different lesion conditions was confirmed through MS-qPCR, which ensured the reliability of the methylation analysis results (36). Subsequently, the study also examined peripheral blood samples from 42 additional cases to identify gene combinations characterized by heightened sensitivity. Subsequently, the investigation expanded to include an analysis of 137 more peripheral blood samples, and the obtained results underwent validation against postoperative pathology results. This comprehensive validation process substantiated the practicality of methylation analysis in evaluating surgical risk for early ESCA (37). The exceptional performance of the LunaCAM model, which had a high AUC of 0.89, highlights its potential as a diagnostic tool (27). The ability of the model to discriminate between early ESCA and intra-EIN demonstrates its versatility and accuracy. The validation of the LunaCAM model across diverse data sets further supports its robustness and reliability. The investigation into the prognostic relevance of the identified HMBs adds an additional layer of understanding, strengthening the diagnostic potential of the LunaCAM model in clinical applications.
This study introduced a novel approach that combines non-invasive liquid biopsy techniques with a methylation analysis to assess the risk of early stage ESCA patients before ESD. The correlation observed between changes in the methylation sites and lesion status provides crucial insights for risk assessment prior to surgical intervention (38-40). Further extensive large-scale studies and clinical validation studies need to be conducted to ascertain the effectiveness of this method in clinical practice. This approach holds particular significance in regions such as China, where ESCA is prevalent and early screening and ESD are pivotal tools in reducing the risk of ESCA development (41).
The integration of a differential gene expression analysis, methylation patterns, and the LunaCAM model offers a comprehensive approach for assessing surgical risk for early ESCA (42). These findings have significant clinical implications, and could be used to inform personalized treatment strategies and facilitate proactive risk assessments before surgery. The identified HMBs stand out as promising markers, warranting further investigation in larger cohorts and diverse clinical scenarios. The practical utility of the LunaCAM model in discriminating between early ESCA and intra-esophageal intraepithelial lesions positions it as a valuable tool for clinicians seeking accurate and non-invasive diagnostic options (27).
The assessment of individual HMBs for early cancer detection highlights the significance of a combinatorial assessment, particularly when dealing with multiple biomarkers, and showcases the potential clinical utility of our findings in enhancing the accuracy of early cancer detection. Acknowledging the limitations of our study is crucial for a balanced interpretation of the results. Factors such as the sample size, heterogeneity, and retrospective nature of the analysis might affect the generalizability of our findings and represent limitation. Additionally, the sensitivity considerations in the validation of the methylation markers emphasize the need for cautious interpretation, especially in diverse clinical scenarios. The insufficient consideration of potentially important methylation markers and the necessity of ongoing improvement in the analysis to reduce the false-positive rate is another limitation. In short, this study presents a valuable exploration of the application of non-invasive liquid biopsy techniques combined with a methylation analysis for early ESCA risk assessment. However, further in-depth research and refinements are essential to facilitate the translation of this method into clinical practice and provide more precise and personalized diagnostic and treatment guidelines for ESCA patients.
Future research should seek to refine and expand on our findings by considering factors such as sample heterogeneity and integrating multi-omics approaches. Additionally, prospective clinical studies should be conducted to validate the utility of the identified markers and the LunaCAM model in real-world scenarios, bringing us closer to practical and impactful applications in the field of ESCA management.
Conclusions
This study emphasized the potential of combining non-invasive liquid biopsy techniques and a methylation analysis for early ESCA risk assessment. By analyzing gene expression disparities, identifying PFS-related genes and examining methylation sites, our research has laid a foundation for robust risk assessment. The strong correlation between methylation changes and lesion status provides key insights that the synergistic analysis of methylation loci addressed in this paper enables a highly sensitive diagnosis of early ESCA. While further research and clinical validation are essential, this approach may offer the potential for more accurate and personalized diagnosis and treatment, enhancing early detection and interventions for ESCA patients.
Acknowledgments
The authors would like to thank all the patients who participated in this article and the various analytic software companies used in the article.
Funding: None.
Footnote
Reporting Checklist: The authors have completed the TRIPOD reporting checklist. Available at https://tcr.amegroups.com/article/view/10.21037/tcr-24-314/rc
Data Sharing Statement: Available at https://tcr.amegroups.com/article/view/10.21037/tcr-24-314/dss
Peer Review File: Available at https://tcr.amegroups.com/article/view/10.21037/tcr-24-314/prf
Conflicts of Interest: All authors have completed the ICMJE uniform disclosure form (available at https://tcr.amegroups.com/article/view/10.21037/tcr-24-314/coif). The authors have no conflicts of interest to declare.
Ethical Statement: The authors are accountable for all aspects of the work in ensuring that questions related to the accuracy or integrity of any part of the work are appropriately investigated and resolved. The study was conducted in accordance with the Declaration of Helsinki (as revised in 2013). The study was approved by the Ethics Committee of Henan Province Hospital of Traditional Chinese Medicine, which is the Second Affiliated Hospital of Henan University of Chinese Medicine (ethics number: HNSZYY20210108) and informed consent was taken from all the patients.
Open Access Statement: This is an Open Access article distributed in accordance with the Creative Commons Attribution-NonCommercial-NoDerivs 4.0 International License (CC BY-NC-ND 4.0), which permits the non-commercial replication and distribution of the article with the strict proviso that no changes or edits are made and the original work is properly cited (including links to both the formal publication through the relevant DOI and the license). See: https://creativecommons.org/licenses/by-nc-nd/4.0/.
References
- Li J, Xu J, Zheng Y, et al. Esophageal cancer: Epidemiology, risk factors and screening. Chin J Cancer Res 2021;33:535-47. [Crossref] [PubMed]
- Yang S, Lin S, Li N, et al. Burden, trends, and risk factors of esophageal cancer in China from 1990 to 2017: an up-to-date overview and comparison with those in Japan and South Korea. J Hematol Oncol 2020;13:146. [Crossref] [PubMed]
- Liang H, Fan JH, Qiao YL. Epidemiology, etiology, and prevention of esophageal squamous cell carcinoma in China. Cancer Biol Med 2017;14:33-41. [Crossref] [PubMed]
- Valkema MJ, Mostert B, Lagarde SM, et al. The effectivity of targeted therapy and immunotherapy in patients with advanced metastatic and non-metastatic cancer of the esophagus and esophago-gastric junction. Updates Surg 2023;75:313-23. [Crossref] [PubMed]
- Acharya R, Mahapatra A, Verma HK, et al. Unveiling Therapeutic Targets for Esophageal Cancer: A Comprehensive Review. Curr Oncol 2023;30:9542-68. [Crossref] [PubMed]
- Chinese expert consensus on early diagnosis and treatment of esophageal cancer. Zhonghua Zhong Liu Za Zhi 2022;44:1066-75. [PubMed]
- Wu Y, Zhang H, Zhou B, et al. Clinical efficacy of endoscopic submucosal dissection in the treatment of early esophageal cancer and precancerous lesions. J Cancer Res Ther 2018;14:52-6. [Crossref] [PubMed]
- Zhou GY, Hu JL, Wang S, et al. Delayed perforation after endoscopic resection of a colonic laterally spreading tumor: A case report and literature review. World J Clin Cases 2020;8:3608-15. [Crossref] [PubMed]
- Noguchi M, Yano T, Kato T, et al. Risk factors for intraoperative perforation during endoscopic submucosal dissection of superficial esophageal squamous cell carcinoma. World J Gastroenterol 2017;23:478-85. [Crossref] [PubMed]
- Wu Z, Yu L, Hou J, et al. Plasma cfDNA for the Diagnosis and Prognosis of Colorectal Cancer. J Oncol 2022;2022:9538384. [Crossref] [PubMed]
- Gedvilaitė V, Schveigert D, Cicėnas S. Cell-free DNA in non-small cell lung cancer. Acta Med Litu 2017;24:138-44. [Crossref] [PubMed]
- Markou A, Londra D, Tserpeli V, et al. DNA methylation analysis of tumor suppressor genes in liquid biopsy components of early stage NSCLC: a promising tool for early detection. Clin Epigenetics 2022;14:61. [Crossref] [PubMed]
- Liu C, Tang H, Hu N, et al. Methylomics and cancer: the current state of methylation profiling and marker development for clinical care. Cancer Cell Int 2023;23:242. [Crossref] [PubMed]
- Chen C, Wang Z, Ding Y, et al. DNA Methylation: From Cancer Biology to Clinical Perspectives. Front Biosci (Landmark Ed) 2022;27:326. [Crossref] [PubMed]
- Luo H, Zhao Q, Wei W, et al. Circulating tumor DNA methylation profiles enable early diagnosis, prognosis prediction, and screening for colorectal cancer. Sci Transl Med 2020;12:eaax7533. [Crossref] [PubMed]
- Leal A, Sidransky D, Brait M. Tissue and Cell-Free DNA-Based Epigenomic Approaches for Cancer Detection. Clin Chem 2020;66:105-16. [Crossref] [PubMed]
- Constantin N, Sina AA, Korbie D, et al. Opportunities for Early Cancer Detection: The Rise of ctDNA Methylation-Based Pan-Cancer Screening Technologies. Epigenomes 2022;6:6. [Crossref] [PubMed]
- Zhang Q, Huang R, Hu H, et al. Integrative Analysis of Hypoxia-Associated Signature in Pan-Cancer. iScience 2020;23:101460. [Crossref] [PubMed]
- Lin W, Wu S, Chen X, et al. Characterization of Hypoxia Signature to Evaluate the Tumor Immune Microenvironment and Predict Prognosis in Glioma Groups. Front Oncol 2020;10:796. [Crossref] [PubMed]
- Zhang Z, Lin E, Zhuang H, et al. Construction of a novel gene-based model for prognosis prediction of clear cell renal cell carcinoma. Cancer Cell Int 2020;20:27. [Crossref] [PubMed]
- Li Y, Ge D, Lu C. The SMART App: an interactive web application for comprehensive DNA methylation analysis and visualization. Epigenetics Chromatin 2019;12:71. [Crossref] [PubMed]
- Wan B, Zhang W, Deng X, et al. Molecular Expression and Prognostic Implications of Krüppel-Like Factor 3 (KLF3) in Clear Cell Renal Cell Carcinoma. Crit Rev Eukaryot Gene Expr 2024;34:45-59. [Crossref] [PubMed]
- Xi Y, Lin Y, Guo W, et al. Multi-omic characterization of genome-wide abnormal DNA methylation reveals diagnostic and prognostic markers for esophageal squamous-cell carcinoma. Signal Transduct Target Ther 2022;7:53. [Crossref] [PubMed]
- Liang W, Zhao Y, Huang W, et al. Non-invasive diagnosis of early-stage lung cancer using high-throughput targeted DNA methylation sequencing of circulating tumor DNA (ctDNA). Theranostics 2019;9:2056-70. [Crossref] [PubMed]
- Minskaia E, Lacerda JF. Analysis of FOXP3 DNA Methylation Patterns to Identify Functional FOXP3+ T-Cell Subpopulations. Methods Mol Biol 2023;2559:115-36. [Crossref] [PubMed]
- Ahmed FE, Gouda MM, Ahmed NC, et al. Quantification of Micrornas by Absolute Dpcr for the Diagnostic Screening of Colon Cancer. Journal of Colon and Rectal Cancer 2019;1:10-37. [Crossref]
- Wang Z, Xie K, Zhu G, et al. Early detection and stratification of lung cancer aided by a cost-effective assay targeting circulating tumor DNA (ctDNA) methylation. Respir Res 2023;24:163. [Crossref] [PubMed]
- Jin S, Zhu D, Shao F, et al. Efficient detection and post-surgical monitoring of colon cancer with a multi-marker DNA methylation liquid biopsy. Proc Natl Acad Sci U S A 2021;118:e2017421118. [Crossref] [PubMed]
- Yu G, Wang LG, Han Y, et al. clusterProfiler: an R package for comparing biological themes among gene clusters. OMICS 2012;16:284-7. [Crossref] [PubMed]
- van der Pol Y, Mouliere F. Toward the Early Detection of Cancer by Decoding the Epigenetic and Environmental Fingerprints of Cell-Free DNA. Cancer Cell 2019;36:350-68. [Crossref] [PubMed]
- Roy D, Tiirikainen M. Diagnostic Power of DNA Methylation Classifiers for Early Detection of Cancer. Trends Cancer 2020;6:78-81. [Crossref] [PubMed]
- Doumbe-Mandengue P, Beuvon F, Belle A, et al. Outcomes of endoscopic submucosal dissection for early esophageal and gastric cardia adenocarcinomas. Clin Res Hepatol Gastroenterol 2021;45:101700. [Crossref] [PubMed]
- Burkitt K. Role of DNA Methylation Profiles as Potential Biomarkers and Novel Therapeutic Targets in Head and Neck Cancer. Cancers (Basel) 2023;15:4685. [Crossref] [PubMed]
- Taryma-Leśniak O, Sokolowska KE, Wojdacz TK. Current status of development of methylation biomarkers for in vitro diagnostic IVD applications. Clin Epigenetics 2020;12:100. [Crossref] [PubMed]
- Qiao G, Zhuang W, Dong B, et al. Discovery and validation of methylation signatures in circulating cell-free DNA for early detection of esophageal cancer: a case-control study. BMC Med 2021;19:243. [Crossref] [PubMed]
- Aleotti V, Catoni C, Poggiana C, et al. Methylation Markers in Cutaneous Melanoma: Unravelling the Potential Utility of Their Tracking by Liquid Biopsy. Cancers (Basel) 2021;13:6217. [Crossref] [PubMed]
- David P, Mittelstädt A, Kouhestani D, et al. Current Applications of Liquid Biopsy in Gastrointestinal Cancer Disease-From Early Cancer Detection to Individualized Cancer Treatment. Cancers (Basel) 2023;15:1924. [Crossref] [PubMed]
- Gissi DB, Fabbri VP, Gabusi A, et al. Pre-Operative Evaluation of DNA Methylation Profile in Oral Squamous Cell Carcinoma Can Predict Tumor Aggressive Potential. Int J Mol Sci 2020;21:6691. [Crossref] [PubMed]
- Meng H, Li G, Wei W, et al. Epigenome-wide DNA methylation signature of benzo[a]pyrene exposure and their mediation roles in benzo[a]pyrene-associated lung cancer development. Journal of Hazardous Materials 2021;416:125839. [Crossref] [PubMed]
- Nikanjam M, Kato S, Kurzrock R. Liquid biopsy: current technology and clinical applications. J Hematol Oncol 2022;15:131. [Crossref] [PubMed]
- Qiu ML, Lin JB, Li X, et al. Current state of esophageal cancer surgery in China: a national database analysis. BMC Cancer 2019;19:1064. [Crossref] [PubMed]
- Spainhour JC, Lim HS, Yi SV, et al. Correlation Patterns Between DNA Methylation and Gene Expression in The Cancer Genome Atlas. Cancer Inform 2019;18:1176935119828776. [Crossref] [PubMed]