Machine learning prediction of the case-fatality of COVID-19 and risk factors for adverse outcomes in patients with non-small cell lung cancer
Highlight box
Key findings
• The case-fatality rate and risk factors for coronavirus disease 2019 (COVID-19) with machine learning have utility in predicting of the effect of COVID-19 on the systemic treatment of patients with advanced-stage non-small cell lung cancer (NSCLC).
What is known and what is new?
• NSCLC has a relatively high risk among solid tumors, with a case-fatality rate of 0.2–0.8%.
• The case fatality rates were 0.86% for patients with early-stage NSCLC, 4.4% for patients with locally advanced NSCLC, and 9.96% for patients with advanced NSCLC.
• According to our validated machine learning model, factors such as palliative chemotherapy (particularly cytotoxic chemotherapy), advanced age, male gender, diabetes, smoking history, history of lung radiotherapy, hypertension, and chronic obstructive pulmonary disease were identified as high-risk factors for case fatality rates. The median area under the curve was 0.84 (95% confidence interval: 0.76–0.92).
What is the implication, and what should change now?
• When advanced NSCLC patients with receive systemic treatment, those with high-risk factors require careful management.
Introduction
The coronavirus disease 2019 (COVID-19) pandemic has resulted in at least 1.5 million deaths worldwide (1). In Korea, the cumulative number of confirmed cases of COVID-19 infection was over 25,000,000 and the case-fatality rate was approximately 0.1% in general population (2). While most patients have only mild symptoms, some experience severe symptoms, including respiratory and multi-organ failure (2,3). The risk factors for severe complications, hospitalization, intensive care unit treatment, and death from COVID-19 include older male patients with chronic diseases (hypertension, diabetes, cardiovascular disease, and respiratory disease) (4,5). A meta-analysis showed that the case fatality rate was significantly higher in males [hazard ratio (HR) 1.67] and in those with cardiovascular disease (HR 6.75), hypertension (HR 4.48), diabetes (HR 4.43), and respiratory disease (HR 3.43) (6). Additionally, the case-fatality rate increased with age, with patients aged >80 years old experiencing a rate of up to 14.8% (6).
Cancer is also a known risk factor for severe complications or death from COVID-19 compared to the general population (6-8). The 30-day fatality rate of patients with cancer is 13–33% (9,10), which is higher than the general population’s 0.1–2% (1-3). However, all cancer patients were not uniformly in the high-risk group of COVID-19 infection. Diverse tumor subtypes, disease status of tumor after anti-cancer treatment might be related with the severity of COVID-19 (5). To be specific, hematological malignancies, such as leukemia, lymphoma, and myeloma, are associated with severe COVID-19 complications compared to solid tumors (HR 1.57) (5,11). The prognosis may differ depending on the cancer stage, treatment type, and treatment response. Therefore, for patients with advanced-stage cancer, maintaining or minimizing the interruption of systemic treatment to control their disease is important for prognosis. A previous study reported that immunotherapy, hormone therapy, targeted therapy, and radiotherapy within the past 4 weeks were not associated with fatality from COVID-19 (12). In patients with cancer, as in the general population, age, male sex, and comorbidities, such as hypertension and cardiovascular disease, were associated with fatality. After adjusting for age, sex, and comorbidities, chemotherapy in the past 4 weeks had no significant effect on fatality from COVID-19 (12). Non-small cell lung cancer (NSCLC) has a relatively high risk among solid tumors, with a case-fatality rate of 0.2–0.8% (5). Other studies have also reported that patients with NSCLC showed high rates of severe or critical COVID-19 events (11,13). Patients with NSCLC share fragility related to defective pulmonary and alveolar architecture because of previous surgery or radiotherapy and underlying lung disease. The goal of treating patients with NSCLC during the COVID-19 pandemic is to minimize the risk of patient exposure and complications should they contract COVID-19 while managing NSCLC, which has a high fatality risk. This study investigated the case fatality rate in patients with NSCLC and identified risk factors which are related with fatality of COVID-19. We present this article in accordance with the TRIPOD reporting checklist (available at https://tcr.amegroups.com/article/view/10.21037/tcr-23-2188/rc).
Methods
Study subjects and data collection
We retrospectively reviewed the medical records of patients with NSCLC who diagnosed with COVID-19 at the Samsung Medical Center in Seoul, Republic of Korea, between January 2020 and April 2022. The patients’ demographics, such as age, sex, and smoking history; disease characteristics, such as stage, type of anti-cancer treatment (chemotherapy, radiotherapy, or surgery), and survival data were collected. We analyzed case fatality rates and risk factors for fatality. Additionally, we investigated the effects of COVID-19 on the systemic treatment in patients receiving palliative systemic treatment.
Ethics statement
This study was conducted in accordance with the Declaration of Helsinki (as revised in 2013). This study was approved by the Institutional Review Board of Samsung Medical Center (approval No. 2022-10-061). The IRB waived the need for informed consent because of the retrospective nature of this study.
Statistical analysis
The study’s primary endpoint was the case fatality rate, defined as the death rate because of COVID-19 complications. The cut-off date for analyzing all the data was June 30, 2022.
Continuous variables were presented as mean and standard deviation, while categorical variables were expressed as frequency and ratio. Chi-square test or Two-Sample t-test was conducted to compare the case and control groups.
To identify predictive factors for COVID-19 mortality, we explored a range of statistical and machine learning methods. The dataset was randomly partitioned into a training set and a test set, with a 7:3 ratio. Subsequently, we conducted analyses using logistic regression models assuming linear correlation in the training set, an elastic net model [a hybrid of least absolute shrinkage and selection operator (LASSO) and Ridge regression], and Tree-based models employing RandomForest (14) and eXtreme Gradient Boosting (XGB) methods (15), which fall under bagging or boosting techniques. These models were analyzed to assess their predictive capabilities on the test set. To develop the logistic model, we assessed the influence of each variable via simple logistic analysis. Variables were selected according to P value and area under the curve (AUC). The final step involved multiple logistic regression analysis.
For LASSO, RandomForest, and glmnet models (16), shrinkage coefficients were determined during training. In the case of tree-based models, variables were selected based on impurity, and the model was rebuilt using the final selected variables to evaluate prediction ability on the test set. AUC was used as a metric for predictive ability to gauge model performance.
To mitigate the influence of chance in data division into training/test sets, the random seed was systematically altered, and the data were repeatedly partitioned. This iterative process allowed us to estimate the model’s performance stability and assess the robustness of predictive abilities.
All statistical analyses were performed using R Statistical Software (version 3.6.1; Foundation for Statistical Computing, Vienna, Austria).
Results
Patient characteristics
Between January 2020 and April 2022, 1,127 patients with NSCLC were diagnosed with COVID-19. Among these patients, 584 were cured after surgery or definitive radiotherapy (RT), 91 had stable disease after concurrent chemoradiotherapy, and 452 had recurrent or metastatic NSCLC. The median follow-up period was 93 days (range, 65–825 days) after COVID-19. Table 1 shows the clinical characteristics of the patients with NSCLC. The median age was 65.5 years (range, 24.0–93.5 years), and 10.3% of patients were older than 75 years. However, 57.2% of patients were male, and 51.8% were ex- or current smokers. Hypertension was the most common underlying comorbidity (39.3%), followed by diabetes (22.2%), chronic obstructive pulmonary disease (COPD) (16.5%), cardiovascular disease (12.0%), and interstitial lung disease (ILD) (8.3%). Regarding vaccination history, 585 patients (51.9%) were vaccinated (one dose: 14 patients, two doses: 121 patients, three doses: 450 patients).
Table 1
Baseline characteristics | No. of patients (n=1,127) | No death event due to COVID-19 infection (n=1,073) | Death event due to COVID-19 infection (n=54) | P value |
---|---|---|---|---|
Age (years) | <0.001 | |||
<75 | 1,011 | 976 (91.0%) | 35 (64.8%) | |
≥75 | 116 | 97 (9.0%) | 19 (35.2%) | |
Sex | 0.003 | |||
Male | 645 | 603 (56.2%) | 42 (77.8%) | |
Female | 482 | 470 (43.8%) | 12 (22.2%) | |
ECOG performance status | <0.001 | |||
0–1 | 1,004 | 955 (89.0) | 49 (90.7%) | |
2 | 8 | 5 (0.5%) | 3 (5.6%) | |
Unknown | 115 | 113 (10.5%) | 2 (3.7%) | |
Vaccination history | 0.37 | |||
No | 17 | 15 (1.4%) | 2 (3.7%) | |
Yes | 585 | 559 (52.1%) | 26 (48.1%) | |
Unknown | 525 | 499 (46.5%) | 26 (48.1%) | |
Smoking history | <0.001 | |||
Never smoker | 528 | 515 (48.0%) | 13 (24.1%) | |
Ex-/current smoker | 584 | 543 (50.6%) | 41 (75.9%) | |
Missing | 15 | 15 (1.4%) | 0 | |
Underlying disease | ||||
Hypertension | 443 | 418 (39.0%) | 25 (46.3%) | 0.32 |
Diabetes | 251 | 225 (21.0%) | 26 (48.1%) | <0.001 |
Cardiovascular disease | 135 | 125 (11.6%) | 10 (18.5%) | 0.13 |
COPD | 186 | 176 (16.4%) | 10 (18.5%) | 0.83 |
ILD | 94 | 87 (8.1%) | 7 (13.0%) | 0.31 |
Viral hepatitis | 36 | 36 (3.4%) | 0 | 0.33 |
History of operation | 669 | 658 (61.3%) | 11 (20.4%) | <0.001 |
History of lung radiotherapy | 235 | 211 (19.7%) | 24 (44.4%) | <0.001 |
History of palliative chemotherapy | 452 | 420 (39.1%) | 32 (59.3%) | 0.004 |
COVID-19 vaccination | 0.69 | |||
No | 542 | 514 (47.9%) | 28 (51.9%) | |
1 | 14 | 13 (1.2%) | 1 (1.9%) | |
2 | 121 | 115 (10.7%) | 6 (11.1%) | |
3 | 450 | 431 (40.2%) | 19 (35.2%) | |
Disease status | <0.001 | |||
NED after surgery or RT | 584 | 578 (53.9%) | 6 (11.1%) | |
SD after CCRT | 91 | 75 (7.0%) | 16 (29.6%) | |
Recurrent or metastatic disease | 452 | 420 (39.1%) | 32 (59.3%) |
COVID-19, coronavirus disease 2019; ECOG, European Cooperative Oncology Group; COPD, chronic obstructive pulmonary disease; ILD, interstitial lung disease; NED, no evidence of disease; RT, radiotherapy; SD, stable disease; CCRT, concurrent chemoradiotherapy.
Among the 452 patients with recurrent or metastatic NSCLC, 387 received chemotherapy during their COVID-19 infection. Furthermore, among these patients, 188 received targeted therapy, 111 received cytotoxic chemotherapy, 62 received immunotherapy +/− chemotherapy, and 26 received other agents, including investigational drug of clinical trials.
Case fatality rate of COVID-19 infection in patients with NSCLC
The following table shows the fatality cases of COVID-19 in 54 patients with NSCLC (Table S1), out of which 21 patients were aged 75 years or older. A total of 17 patients had lung disease, 8 had COPD, and 10 had ILD (one had COPD and ILD). According to disease status, there were 5 patients with no evidence of disease (NED) after surgery or definitive RT for early-stage NSCLC, 4 patients who received concurrent chemoradiotherapy (CCRT) for locally advanced NSCLC, and 45 patients with advanced-stage disease.
The case-fatality rate was 4.8% of the total population. According to the stage, 0.86% (5/584) of patients had early-stage NSCLC, 4.40% (4 of 91) of patients had locally advanced NSCLC, and 9.96% (45 of 452) of patients had recurrent or metastatic NSCLC. Figure 1 shows the analysis flow for developing and evaluating the prediction model for the COVID-19 fatality rate in patients with NSCLC. Among the four prediction models (logistic regression, XGB, random forest, and elastic net), the two best models were chosen after performance and stability assessment.
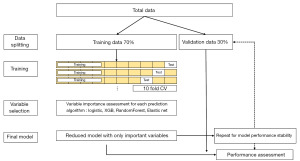
Risk model for a case-fatality rate of COVID-19 infection in patients with NSCLC by logistic regression
The factors related to the case-fatality of COVID-19 included old age (≥75 years) [odds ratio (OR) =2.99; 95% confidence interval (CI): 1.58–5.65], European Cooperative Oncology Group (ECOG) performance status (≥2) (OR =3.27; 95% CI: 1.87–5.71), smoking history (OR =11.69; 95% CI: 2.72–50.35), diabetes (OR =3.50; 95% CI: 2.01–6.09), history of lung radiotherapy (OR =2.26; 95% CI: 1.30–3.95), and type of palliative chemotherapy (Table 2). In multivariate analysis, age (≥75 years) (OR =4.17; 95% CI: 1.85–9.09), ECOG performance status (≥2) (OR =10.6; 95% CI: 2.65–42.23), smoking history (OR =2.29; 95% CI: 1.05–5.00), and type of palliative chemotherapy (OR =2.86; 95% CI: 1.20–6.67) were associated with fatality from COVID-19. Figure 2A shows that the median AUC for the risk model for the case-fatality rate was 0.84 (95% CI: 0.76–0.93).
Table 2
Baseline characteristics | Univariate analysis | Multivariate analysis | |||
---|---|---|---|---|---|
OR (95% CI) | P value | OR (95% CI) | P value | ||
Age (≥75 vs. <75 years) | 2.99 (1.58–5.65) | <0.001 | 4.17 (1.85–9.09) | 0.001 | |
Sex (female vs. male) | 0.37 (0.19–0.70) | <0.001 | – | 0.04 | |
ECOG performance status (0–1 vs. 2) | 3.27 (1.87–5.71) | <0.001 | 10.6 (2.65–42.23) | 0.001 | |
Vaccination (yes vs. no) | 0.35 (0.08–1.61) | 0.18 | – | – | |
Smoking history (ex-/current vs. never smoker) | 11.69 (2.72–50.35) | <0.001 | 2.29 (1.05–5.00) | – | |
Hypertension | 1.35 (0.78–2.34) | 0.28 | – | – | |
Diabetes | 3.50 (2.01–6.09) | <0.001 | – | – | |
Cardiovascular disease | 1.72 (0.85–3.51) | 0.13 | – | – | |
COPD | 1.16 (0.57–2.35) | 0.68 | – | – | |
ILD | 1.69 (0.74–3.85) | 0.21 | – | – | |
History of lung radiotherapy | 2.26 (1.30–3.95) | <0.001 | – | – | |
History of palliative chemotherapy | 2.51 (0.76–8.30) | 0.13 | 2.86 (1.20–6.67) | 0.02 | |
Type of systemic chemotherapy | 0.002 | ||||
IO or others plus chemotherapy | 13.71 (3.67–51.18) | <0.001 | 34.2 (7.6–154.09) | ||
IO | 10.28 (3.22–32.88) | <0.001 | 24.5 (5.36–111.91) | ||
Chemotherapy | 19.80 (8.12–48.27) | <0.001 | 48.35 (14.76–158.32) |
COVID-19, coronavirus disease 2019; OR, odds ratio; CI, confidence interval; ECOG, European Cooperative Oncology Group; COPD, chronic obstructive pulmonary disease; ILD, interstitial lung disease; IO, immunotherapy.
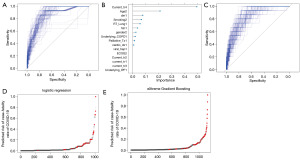
Risk model for a case-fatality rate of COVID-19 infection in patients with NSCLC by XGB
As shown in Figure 2B, the type of palliative chemotherapy (non-targeted vs. targeted therapy), age, diabetes, smoking history, history of lung radiotherapy, hypertension, sex, and COPD disease had high importance scores. Figure 2C shows that the median AUC for the risk model predicting the case-fatality rate was 0.84 (95% CI: 0.76–0.92). Figures 2D,2E show the predicted distributions of COVID-19 fatalities using the prediction model.
Effect of COVID-19 infection on systemic treatment
Among 387 patients who received palliative systemic treatment at the time of COVID-19 infection, 95.3% of patients (369 of 387) continued their systemic treatment within 1 month after the COVID-19 infection. Only one patient discontinued treatment because of complications from the COVID-19 infection, and 18 patients changed their systemic treatment because of disease progression.
Among the total number of patients, 63 were infected with COVID-19 during treatment with a programmed cell death 1/programmed cell death 1 ligand (PD-1/PD-L1) inhibitor alone (n=37) or a PD-1/PD-L1 inhibitor with chemotherapy (n=25). Three patients discontinued treatment because of the disease progression; however, 60 patients continued treatment after the COVID-19 infection. There were no cases of treatment discontinuation among the 188 patients who received targeted therapy, such as epidermal growth factor receptor (EGFR)-tyrosine kinase inhibitor (TKI) and anaplastic lymphoma kinase-TKI (ALK-TKI). However, of the 111 patients who received cytotoxic chemotherapy, 99 continued receiving it, 11 discontinued it because of disease progression, and one discontinued it because of COVID-19 infection.
Discussion
This study demonstrates the case fatality rate of COVID-19 and its risk factors in patients with NSCLC. Our machine learning model validated that palliative chemotherapy (especially cytotoxic chemotherapy), old age, male, diabetes, smoking history, history of lung radiotherapy, hypertension, and COPD were high-risk factors for case fatality rates. In a previous study, smoking history and COPD were associated with severe COVID-19 infection; however, prior thoracic surgery, radiation, and systemic therapies did not affect severity in patients with lung cancer (5,17-20). It was controversial that the effect of type of systemic therapy on the severity of COVID-19. In the previous study, immunotherapy did not increase the severity of COVID-19 (21).
At the beginning of COVID-19 outbreak, the treatment guideline for systemic treatment was not announced. The interruption of systemic therapy in advanced-stage cancer might lead to disease progression and cancer-related fatality. In our study, COVID-19 did not affect systemic treatment, as most patients who received cytotoxic chemotherapy, targeted therapy, or immunotherapy continued their treatment within 1 month after recovery from COVID-19 infection.
The severity of COVID-19 is also affected by the vaccination rate and COVID-19 variants. In our study, 51.9% of patients received COVID-19 vaccination, although the recent vaccination rate has increased to 88.7% (2). The variants of COVID-19 have different clinical features. Delta variant B.1.617.2 (Delta variant) is a variant of lineage B.1.617 of severe acute respiratory syndrome coronavirus 2 (SARS-CoV-2), which is the reason for India’s second wave during the COVID-19 pandemic. On May 31, 2021, the World Health Organization (WHO) named this variant the “Delta variant”. The Omicron (C.1.2) variant was detected in Botswana in November, and subsequently, many nations, particularly South Africa, discovered this novel strain of COVID-19. One of the limitations of our study is that vaccination history could not be confirmed in 46.6% of patients. In addition, we need to be careful in interpreting our result considering that Omicron was the dominant variant of COVID-19 not other fatal variant such as Delta variants during our study period in Korea.
Understanding patient- and cancer-specific features that affect the severity of COVID-19 may help in optimal management during this pandemic. According to our findings, patients with risk factors, such as palliative chemotherapy, type of palliative chemotherapy, age, diabetes, smoking history, history of lung radiotherapy, hypertension, sex, and COPD are risk factors for COVID-19 case fatality rate. This finding highlights the importance of maintaining systemic treatment, including targeted therapy or immunotherapy, and regular follow-up, considering the low case fatality in patients with NSCLC. However, patients with risk factors, such as palliative chemotherapy, type of palliative chemotherapy, age, diabetes, smoking history, history of lung radiotherapy, hypertension, sex, and COPD, require cautious follow-up and management. The strength of this study lies in its utilization of machine learning statistical analysis. Machine learning statistical analysis is focused on maximizing prediction accuracy, whereas traditional statistical models aim to infer relationships between variables. Machine learning offers significant flexibility and is devoid of a priori assumptions, whereas traditional statistical methods rely on strong assumptions, such as the error distribution type, additivity of parameters within the linear predictor, and proportional hazards. Machine learning holds the advantage of incorporating all available information within a specific field. Traditional statistical approaches, even those considered at the apex of the evidence hierarchy, frequently falter due to their a priori selection of variables for consideration (22).
Conclusions
This study showed most patients with advanced NSCLC continued systemic treatment and had a lower-case fatality rate. Patients who received PD-1/PD-L1 with or without chemotherapy or targeted therapy could continue treatment after the COVID-19 infection. However, patients with risk factors, such as old age (≥75 years), a history of previous lung radiotherapy, current cytotoxic chemotherapy, and underlying lung disease, require careful management.
Acknowledgments
The abstract in this article has been previously published at ESMO Congress 2023.
Funding: This study was supported by grants from
Footnote
Reporting Checklist: The authors have completed the TRIPOD reporting checklist. Available at https://tcr.amegroups.com/article/view/10.21037/tcr-23-2188/rc
Data Sharing Statement: Available at https://tcr.amegroups.com/article/view/10.21037/tcr-23-2188/dss
Peer Review File: Available at https://tcr.amegroups.com/article/view/10.21037/tcr-23-2188/prf
Conflicts of Interest: All authors have completed the ICMJE uniform disclosure form (available at https://tcr.amegroups.com/article/view/10.21037/tcr-23-2188/coif). The authors have no conflicts of interest to declare.
Ethical Statement: The authors are accountable for all aspects of the work in ensuring that questions related to the accuracy or integrity of any part of the work are appropriately investigated and resolved. The study was conducted in accordance with the Declaration of Helsinki (as revised in 2013). The study was approved by the Institutional Review Board of Samsung Medical Center (approval No. 2022-10-061). The IRB waived the need for informed consent because of the retrospective nature of this study.
Open Access Statement: This is an Open Access article distributed in accordance with the Creative Commons Attribution-NonCommercial-NoDerivs 4.0 International License (CC BY-NC-ND 4.0), which permits the non-commercial replication and distribution of the article with the strict proviso that no changes or edits are made and the original work is properly cited (including links to both the formal publication through the relevant DOI and the license). See: https://creativecommons.org/licenses/by-nc-nd/4.0/.
References
- World Health Organization (WHO). WHO Coronavirus Disease (COVID-19) Dashboard. Accessed Feb 1, 2023. Available online: https://covid19.who.int/table
- Coronavirus (COVID-19), Republic of Korea. Accessed Feb 1, 2023. Available online: https://ncov.kdca.go.kr/en/
- World Health Organization (WHO). Estimating mortality from COVID-19. Accessed Feb 1, 2023. Available online: https://www.who.int/news-room/commentaries/detail/estimating-mortality-from-covid-19
- Grasselli G, Zangrillo A, Zanella A, et al. Baseline Characteristics and Outcomes of 1591 Patients Infected With SARS-CoV-2 Admitted to ICUs of the Lombardy Region, Italy. JAMA 2020;323:1574-81. [Crossref] [PubMed]
- Lee LYW, Cazier JB, Starkey T, et al. COVID-19 prevalence and mortality in patients with cancer and the effect of primary tumour subtype and patient demographics: a prospective cohort study. Lancet Oncol 2020;21:1309-16. [Crossref] [PubMed]
- Deng G, Yin M, Chen X, et al. Clinical determinants for fatality of 44,672 patients with COVID-19. Crit Care 2020;24:179. [Crossref] [PubMed]
- Wang Q, Berger NA, Xu R. Analyses of Risk, Racial Disparity, and Outcomes Among US Patients With Cancer and COVID-19 Infection. JAMA Oncol 2021;7:220-7. [Crossref] [PubMed]
- Al-Quteimat OM, Amer AM. The Impact of the COVID-19 Pandemic on Cancer Patients. Am J Clin Oncol 2020;43:452-5. [Crossref] [PubMed]
- Kuderer NM, Choueiri TK, Shah DP, et al. Clinical impact of COVID-19 on patients with cancer (CCC19): a cohort study. Lancet 2020;395:1907-18. [Crossref] [PubMed]
- Garassino MC, Whisenant JG, Huang LC, et al. COVID-19 in patients with thoracic malignancies (TERAVOLT): first results of an international, registry-based, cohort study. Lancet Oncol 2020;21:914-22. [Crossref] [PubMed]
- Jee J, Foote MB, Lumish M, et al. Chemotherapy and COVID-19 Outcomes in Patients With Cancer. J Clin Oncol 2020;38:3538-46. [Crossref] [PubMed]
- Lee LY, Cazier JB, Angelis V, et al. COVID-19 mortality in patients with cancer on chemotherapy or other anticancer treatments: a prospective cohort study. Lancet 2020;395:1919-26. [Crossref] [PubMed]
- Richardson S, Hirsch JS, Narasimhan M, et al. Presenting Characteristics, Comorbidities, and Outcomes Among 5700 Patients Hospitalized With COVID-19 in the New York City Area. JAMA 2020;323:2052-9. [Crossref] [PubMed]
- Liaw A, Wiener M. Classification and Regression by randomForest. R News 2002;2:18-22.
- Chen T, He T, Benesty M, et al. xgboost: Extreme Gradient Boosting. R package version 1.6.0.1. 2022. Available online: https://CRAN.R-project.org/package=xgboost
- Friedman J, Hastie T, Tibshirani R. Regularization Paths for Generalized Linear Models via Coordinate Descent. J Stat Softw 2010;33:1-22. [Crossref] [PubMed]
- Luo J, Rizvi H, Preeshagul IR, et al. COVID-19 in patients with lung cancer. Ann Oncol 2020;31:1386-96. [Crossref] [PubMed]
- Passaro A, Bestvina C, Velez Velez M, et al. Severity of COVID-19 in patients with lung cancer: evidence and challenges. J Immunother Cancer 2021;9:e002266. [Crossref] [PubMed]
- Liang W, Guan W, Chen R, et al. Cancer patients in SARS-CoV-2 infection: a nationwide analysis in China. Lancet Oncol 2020;21:335-7. [Crossref] [PubMed]
- Bakouny Z, Hawley JE, Choueiri TK, et al. COVID-19 and Cancer: Current Challenges and Perspectives. Cancer Cell 2020;38:629-46. [Crossref] [PubMed]
- Luo J, Rizvi H, Egger JV, et al. Impact of PD-1 Blockade on Severity of COVID-19 in Patients with Lung Cancers. Cancer Discov 2020;10:1121-8. [Crossref] [PubMed]
- Rajula HSR, Verlato G, Manchia M, et al. Comparison of Conventional Statistical Methods with Machine Learning in Medicine: Diagnosis, Drug Development, and Treatment. Medicina (Kaunas) 2020;56:455. [Crossref] [PubMed]