Construction and validation of a prognostic and therapeutic cuproptosis- and immune-related gene signature in hepatocellular carcinoma
Highlight box
Key findings
• This study innovatively developed a hepatocellular carcinoma (HCC) risk signature based on cuproptosis- and immune-related genes (CIRGs).
• The signature performed well in predicting the prognosis of HCC patients in both the training and external validation cohorts.
• Remarkable differences in the immune profiles between the high- and low-risk groups were observed.
• The signature is promising in evaluating tumor mutation burden (TMB) and treatment response to immunotherapy, targeted therapy and chemotherapy.
What is known and what is new?
• Cuproptosis-related genes play an essential role in the development of multiple tumors.
• This study constructed and validated a risk signature in HCC based on CIRGs.
What is the implication, and what should change now?
• This study constructed and validated a risk signature in HCC based on CIRGs and further explored systematically the value of the signature for clinical applications and stratification research, focusing on functional enrichment analysis, tumor mutation burden analysis, immune profiles analysis, and drug sensitivity analysis. We can envision that combined with other accepted clinicopathological features, the signature could significantly help oncologists tailor more efficient treatment strategies for HCC patients.
Introduction
Cell metabolism is crucial in tumor development. Tumor cells differ from normal cells in several ways, including autonomous proliferation. To meet the energy and material basis required for rapid proliferation, tumor metabolism is abnormally active and undergoes metabolic reprogramming in glucose, amino acid, and lipid metabolism, which is considered one of the hallmarks of cancer (1). Copper works as a cofactor for numerous essential enzymes and is engaged in a variety of physiological activities, such as oxidative stress and energy metabolism. Copper ions are present in low concentrations and maintain equilibrium in organisms under normal conditions; however, when abnormal accumulation of copper occurs, cell death and tumor growth can be induced (2). Cuproptosis is a new nonprogrammed cell death mechanism that differentiates from the well-known apoptosis, pyroptosis, necroptosis, and ferroptosis mechanisms. Tsvetkov et al. (3) discovered that copper acts by binding to the lipoylation elements of the tricarboxylic acid (TCA) cycle, causing the aggregation and disorder of these proteins, thereby blocking TCA cycle, triggering proteotoxic stress, and inducing cell death. Notably, this study identified ten cuproptosis-related genes (CRGs) through genome-wide analysis, providing a reference for research on cuproptosis.
Hepatocellular carcinoma (HCC) is the fourth leading cause of cancer deaths worldwide and the second leading cause of cancer deaths in China (4,5). HCC develops insidiously, and most patients are identified at a late stage. Patients with advanced HCC often show terrible survival outlook, with a median survival of only 1–2 years (6), and systemic therapy is a critical treatment alternative for these HCC patients. Plenty of studies, represented by the IMbrave150 phase III clinical trial (7), have confirmed that immunotherapy combined with targeted therapy provides significant benefit to HCC patients and becomes the primary option for HCC patients at an advanced stage. Currently, immunotherapy has become a hot topic in oncology research; however, one major issue confronting the field is the lack of effective biomarkers or risk models to guide medication and optimize individualized chronic management for HCC patients. It has been demonstrated that copper ions within tumors can affect immune escape by regulating programmed cell death ligand 1 (PD-L1) expression (8); nevertheless, the role of cuproptosis- and immune-related genes (CIRGs) in HCC is still unknown. Therefore, we intended to establish and verify a risk signature based on CIRGs through bioinformatics to predict HCC patients’ prognosis, immunotherapy, targeted therapy, and chemotherapy response. We present this article in accordance with the TRIPOD reporting checklist (available at https://tcr.amegroups.com/article/view/10.21037/tcr-23-2182/rc).
Methods
Data processing and candidate CIRGs recognition
The Cancer Genome Atlas (TCGA) (https://portal.gdc.cancer.gov/) was used to access RNA-seq and clinical data of HCC patients. Figure S1 depicts the analysis process. According to a previous study, ten CRGs (FDX1, LIAS, LIPT1, DLD, DLAT, PDHA1, PDHB, MTF1, GLS, and CDKN2A) were gained (3). Data on immune-related genes (IRGs) were obtained from IMMPORT (https://www.immport.org/). To identify CIRGs, we utilized Pearson correlation analysis, with |coefficient| >0.1 and P<0.05 as screening requirements.
Validation data in our study were from the International Cancer Genome Consortium (ICGC) (https://dcc.icgc.org/) and GSE14520 dataset in the Gene Expression Omnibus (GEO) (http://www.ncbi.nlm.nih.gov/geo/). The study was conducted in accordance with the Declaration of Helsinki (as revised in 2013).
Construction of the CIRG signature
The CIRGs associated with HCC patients’ survival were filtered using univariate Cox regression analysis. For systematic analysis, total samples were randomly classified into two categories in a one: one ratio, including training and testing groups. The training set was then applied to build a CIRG signature using least absolute shrinkage and selection operator (LASSO) and multivariate Cox regression analyses. Multivariate Cox relapse coefficients (β) were employed to calculate risk scores, and the formula was as follows:
Each CIRG’s coefficient and expression level are represented by “Coefi” and “Xi”, respectively. Depending on the median risk score, the training group’ samples were classified into two different risk groups. The R package “pheatmap” was employed to evaluate patients’ risk scores and survival time distributions, as well as the expression of seven CIRGs in the risk signature. Kaplan-Meier (K-M) survival curves were plotted utilizing the R package “survival”, and risk scores were included in Cox regression analysis to determine if risk scores could independently affect HCC patients’ survival. Plotting time-dependent receiver operating characteristic (ROC) curves with R package “timeROC” was performed to evaluate the model’s predictive reliability.
Validation of the CIRG signature
The samples’ risk scores were calculated employing the above formula in the testing group, the entire group, the ICGC database, and GSE14520 dataset. In different validation cohorts, the risk signature’s prognostic value was validated comprehensively through a series of survival analyses.
Evaluation of correlation between the signature and HCC clinicopathological characteristics
We investigated the link among the signature and HCC clinicopathological characteristics utilizing the R package “ComplexHeatmap”, and we also mapped the proportion of clinicopathological factors in different risk groups, as well as K-M survival curves in clinical subgroups.
Principal component and differentially expressed genes (DEGs) enrichment analyses
We employed the R package “scatterplot3d” for principal component analysis. Genes that were differentially expressed in various risk groups were screened utilizing R package “limma”, with the screening criteria being |logFC| >1 and false discovery rate (FDR) <0.05. Following that, Gene Ontology (GO) and Kyoto Encyclopedia of Genes and Genomes (KEGG) enrichment studies were carried out by DEGs, and the R package “gplot2” was applied to visualize the results.
This study was conducted using the R package “clusterProfiler” for gene set enrichment analysis (GSEA), and the reference dataset was obtained from the MSigDB database (http://www.gsea-msigdb.org/gsea/msigdb/index.jsp), and FDR <0.25, adjusted P<0.05 and |normalized enrichment score (NES)| >1 were considered as significant enrichment, and R package “ggplot2” was used for visualization.
Assessment of tumor mutation burden (TMB) between different risk groups
The R package “maftools” was employed to draft the waterfall maps of the top 15 mutated genes of HCC patients. We calculated TMB, compared TMB discrepancies between two risk groups, and examined at the impact of TMB on overall survival (OS) utilizing the R packages “limma” and “ggpubr”.
Comparison of immune profiles among different risk groups
The ESTIMATE algorithm was applied to evaluate immune score, stromal score, as well as tumor purity of HCC samples in tumor immune microenvironment (TIME). The ESTIMATE analysis was carried out on cancer samples by making use of transcriptional profiles in estimating the number of tumor cells, immune cells, and stromal cells that had infiltrated tumor. The “estimateScore” function was utilized in order to assess immune score, stromal score and tumor purity (P<0.05). Then, using a single-sample GSEA approach, we assessed various immune cell subgroups, associated functions, and pathways. In addition, we compared a series of immune checkpoint genes’ expression in HCC patients with different risk.
Prediction of treatment benefits between different risk groups
The immunophenoscore (IPS) was employed to forecast immunotherapy sensitivity. The immune status of samples was determined using the IPS function. IPS quantified and visualized four different immunophenotypes in every sample by using immune response or immune tolerance markers (antigen presentation, effector cells, suppressor cells and checkpoint markers), which produced a z score that summarized the above four types. TCIA (https://www.cancerimagingarchive.net/) was applied to obtain IPS data from The Cancer Genome Atlas-liver hepatocellular carcinoma (TCGA-LIHC) samples. The 50% inhibitory concentration (IC50) values of several familiar medicines for HCC patients with different risk were then calculated employing the R packages “pRRophetic” and “ggplot2”.
Statistical analysis
The t test or the Wilcoxon test was employed to compare two groups. For the purpose of comparing proportionate variations, Chi-squared test was utilized. Survival curves for subgroups were generated for per dataset using K-M plotters, and the log-rank test was performed to examine whether the discrepancies were noteworthy. P<0.05 was deemed statistically significance. Utilizing the R 4.1.2 program, all statistical analyses were performed.
Results
Identifying CIRGs in the TCGA database
The TCGA database was exploited to obtain transcriptional and clinical data of 374 HCC samples and 50 normal samples. As shown in Figure S2, ten CRGs had varying levels of expression in HCC and normal tissues, and except for FDX1, nine CRGs differed significantly between HCC and corresponding paracancerous normal tissues, with DLTA, PDHA1, GLS, and CDKN2A thought to have the potential to predict HCC patients’ prognosis (all P<0.05). In addition, a Sankey diagram demonstrated the strong correlation between CRGs and IRGs (Figure 1A), and 939 IRGs were identified as CIRGs.
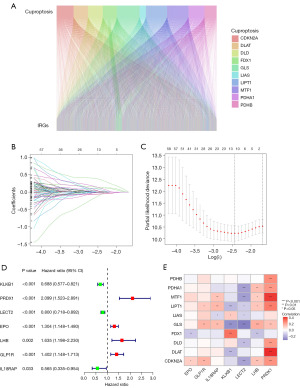
Construction of the CIRG signature
After excluding cases with incomplete data, 370 valid samples were obtained. Using univariate Cox regression analysis, 176 differentially expressed CIRGs associated with prognosis were identified. The HCC samples were allocated at a ratio of one: one to the training and testing groups, and the clinical features of three groups had no obvious difference (Table 1). LASSO regression analysis was done to determine which model performed the best in the training cohort (Figure 1B,1C), and a risk signature based on seven CIRGs was developed using multivariate Cox regression analysis (Figure 1D). Furthermore, Figure 1E demonstrates that the seven CIRGs had high correlations with ten CRGs.
Table 1
Characteristics | Train (n=185) | Test (n=185) | Total (n=370) | P |
---|---|---|---|---|
Age (years) | 0.75 | |||
≤65 | 114 (61.62) | 118 (63.78) | 232 (62.7) | |
>65 | 71 (38.38) | 67 (36.22) | 138 (37.3) | |
Gender | 0.12 | |||
Female | 53 (28.65) | 68 (36.76) | 121 (32.7) | |
Male | 132 (71.35) | 117 (63.24) | 249 (67.3) | |
Histologic grade | 0.67 | |||
G1 | 29 (15.68) | 26 (14.05) | 55 (14.86) | |
G2 | 84 (45.41) | 93 (50.27) | 177 (47.84) | |
G3 | 65 (35.14) | 56 (30.27) | 121 (32.7) | |
G4 | 7 (3.78) | 5 (2.70) | 12 (3.24) | |
Unknown | 0 (0.00) | 5 (2.70) | 5 (1.35) | |
Pathologic stage | 0.89 | |||
I | 81 (43.78) | 90 (48.65) | 171 (46.22) | |
II | 42 (22.7) | 43 (23.24) | 85 (22.97) | |
III | 44 (23.78) | 41 (22.16) | 85 (22.97) | |
IV | 2 (1.08) | 3 (1.62) | 5 (1.35) | |
Unknown | 16 (8.65) | 8 (4.32) | 24 (6.49) | |
T | 0.81 | |||
T1 | 90 (48.65) | 91 (49.19) | 181 (48.92) | |
T2 | 48 (25.95) | 45 (24.32) | 93 (25.14) | |
T3 | 42 (22.70) | 38 (20.54) | 80 (21.62) | |
T4 | 5 (2.70) | 8 (4.32) | 13 (3.51) | |
Unknown | 0 | 3 (1.62) | 3 (0.81) | |
N | 0.11 | |||
N0 | 119 (64.32) | 52 (28.11) | 252 (68.11) | |
N1 | 4 (2.16) | 133 (71.89) | 4 (1.08) | |
Unknown | 62 (33.51) | 0 | 114 (30.81) | |
M | 0.61 | |||
M0 | 134 (72.43) | 132 (71.35) | 266 (71.89) | |
M1 | 1 (0.54) | 3 (1.62) | 4 (1.08) | |
Unknown | 50 (27.03) | 50 (27.03) | 100 (27.03) |
HCC, hepatocellular carcinoma; TCGA, The Cancer Genome Atlas.
HCC patients were split in half depending on the median risk score, and low-risk patients fared superior in terms of survival in the training set. Furthermore, the high-risk group had high expression levels of PRDX1, EPO, LHB, and GLP1R while having low expression levels of KLKB1, LECT2, and IL18RAP (Figure 2A). Compared to high-risk patients, low-risk patients showed better survival outlook (P<0.001, Figure 2B), and according to Cox regression analysis, risk score and pathologic stage could serve as predictors of HCC patients’ OS independently (all P<0.001, Figure 2C,2D). Risk score was found to be the most effective in predicting 1-year OS when compared to clinicopathological characteristics (Figure 2E), with area under the curve (AUC) values of 0.852, 0.858, and 0.820 for 1-, 3-, and 5-year OS, separately (Figure 2F).
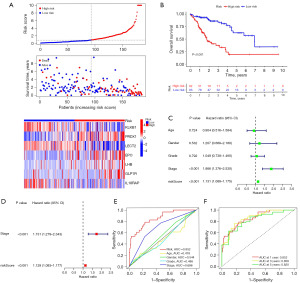
Validation of the CIRG signature
We used the internal and external cohorts to validate the risk signature’s accuracy and reliability. Table 2 shows the clinicopathological features of the ICGC and GEO databases. Different validation cohorts produced results resembled the training dataset. Low-risk patients fared superior in terms of survival, and risk score could independently affect HCC patients’ OS (all P<0.05, Figures 3,4).
Table 2
Characteristics | ICGC (n=231) | GEO (n=221) |
---|---|---|
Age (years) | ||
≤65 | 89 (38.53) | 200 (90.50) |
>65 | 142 (61.47) | 21 (9.50) |
Gender | ||
Female | 61 (26.41) | 30 (13.57) |
Male | 170 (73.59) | 191 (86.43) |
Pathologic stage | ||
I | 36 (15.58) | 95 (42.99) |
II | 105 (45.45) | 77 (34.84) |
III | 71 (30.74) | 49 (22.17) |
IV | 19 (8.23) | 0 |
Cirrhosis | ||
Yes | – | 203 (91.86) |
No | – | 18 (8.14) |
HCC, hepatocellular carcinoma; ICGC, International Cancer Genome Consortium; GEO, Gene Expression Omnibus.
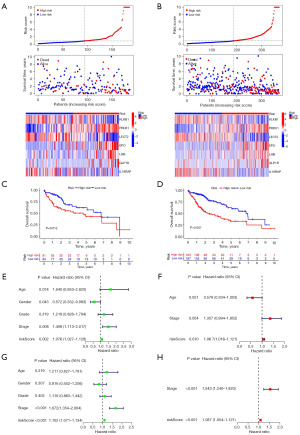
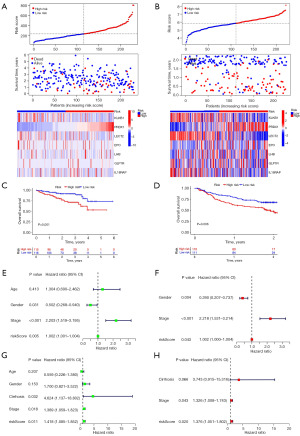
Correlation among the signature with HCC clinical features
Figure 5 shows that histological grade, pathological stage and T stage were relevant to risk score (all P<0.01). Moreover, risk score was still a dependable prognostic marker in multiple clinical subgroups (all P<0.05, Figure S3). In addition, samples in N1 and M1 subgroups were too small to perform survival analysis.
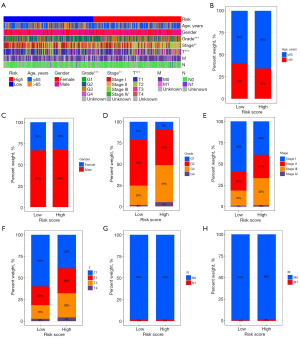
Principal component and DEGs enrichment analyses
According to principal component analysis, the high- and low-risk groups were optimally differentiated in terms of the seven CIRGs (Figure 6A-6D). The GO functional enrichment results discovered that the DEGs were mainly mapped to GO terms related to genetic material synthesis and cell division (Figure 6E,6F). DEGs were also used in KEGG functional enrichment analyses, and we discovered that DEGs were largely enriched pathways associated with cancer, including the cell cycle and P53 signaling pathways (Figure 6G,6H).
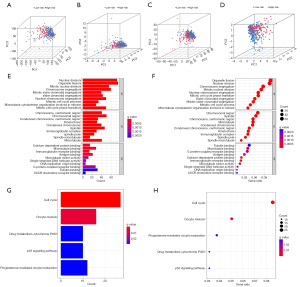
GSEA revealed that the model could possibly engage in HCC metabolism via drug metabolism cytochrome P450, drug metabolism other enzymes, fatty acid metabolism, alanine aspartate and glutamate metabolism, glycine serine and threonine metabolism, histidine metabolism, tryptophan metabolism, tyrosine metabolism, arginine and proline metabolism (all P<0.05, Figure S4).
Correlation among the risk signature with TMB
The mutagenesis outlook of the top 15 mutated genes in two risk groups was depicted using waterfall plots, with TP53 showing notably more mutations in high-risk patients (Figure 7A,7B). Further study discovered that link among TMB with risk score was positive, with TMB markedly lower in the low-risk group (P=0.0061, Figure 7C). Then, low-TMB group had a greater survival, and low-risk combination with the low-TMB group had the best prognosis (all P<0.05, Figure 7D,7E).
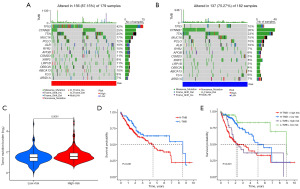
The risk signature’s immune profiles
The assessment of the correlations among the signature with TIME showed that the immune score (P=0.0076, Figure 8A), stromal score (P=6.8e−07, Figure 8B), and ESTIMATE score (P=0.0001, Figure 8C) were markedly higher in low-risk patients, whereas tumor purity (P=0.0001, Figure 8D) demonstrated the opposite results. Figure 8E depicts discrepancies in immune cell infiltration levels from two risk subgroups. It was discovered that the low-risk group had high infiltration levels of B cells, CD8+ T cells, DCs, Mast cells, Neutrophils, NK cells, pDCs, T helper cells, Th1 cells, and TIL, while having low infiltration levels of Macrophages. In addition, we looked at discrepancies in immune function among two risk subgroups and uncovered that low-risk group had high scores of cytolytic activity, HLA, inflammation promoting, T cell co-inhibition, T cell co-stimulation, and type II IFN response (all P<0.05, Figure 8F). Given that immune checkpoint inhibitors are widely used in clinical practice and effectively improve HCC patients’ survival, we found that the low-risk group had high expression levels of IDO2, BTLA, IDO1, CD48, TMIGD2, CD40LG, and KIR3DL1 while having low expression levels of VTCN1, TNFSF9, TNFRSF4, HHLA2, LAIR1, TNFSF15, TNFSF4 CD276, HAVCR2, CD80, LGALS9, TNFRSF18, and TNFRSF14 (all P<0.05, Figure 8G).
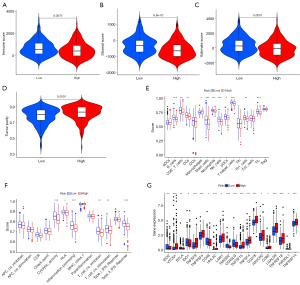
Prediction of sensitivity to different kinds of drugs and potential small-molecule inhibitors
Finally, we evaluated whether the risk signature can predict the treatment benefits among the two risk groups from different kinds of drugs, including immunotherapy, targeted therapy, chemotherapy, and small-molecule inhibitors. According to the CIRG signature, each HCC patient received corresponding risk score and was then classified into high- or low-risk group. HCC individuals treated with cytotoxic T lymphocyte associated antigen-4 (CTLA4) and programmed cell death protein 1 (PD-1) blockers had higher IPS in the low-risk group. The higher an IPS’s z score is, the more immunogenic the sample (9); therefore, a higher IPS often indicates greater odds of being attacked by immune system. Our research showed that immunotherapy is most effective for low-risk patients (all P<0.05, Figure 9A-9D). Next, we evaluated the IC50 values distinctions among the two risk groups for sorafenib, two familiar chemotherapeutic drugs (doxorubicin and gemcitabine), and AKT (also known as protein kinase B) inhibitors. It was found that high-risk patients exhibited low IC50 values for sorafenib (all P<0.05, Figure 9E,9F), doxorubicin (all P<0.05, Figure 9G,9H), gemcitabine (all P<0.05, Figure 9I,9J), and AKT inhibitors (all P<0.05, Figure 9K,9L), indicating higher sensitivities of high-risk patients to these drugs or potential drugs. Having said all of above, immunotherapy proved more effective for low-risk patients than it did for high-risk individuals who responded better to targeted therapy, chemotherapy, and AKT inhibitors.
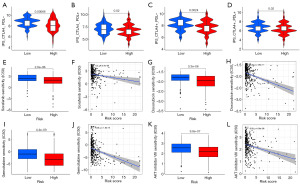
Discussion
Copper is an important micronutrient that is required by the body, but an imbalance in copper can affect the physiological activities of a variety of organs, such as the liver, heart, and central nervous system, and vital biological processes, such as cell metabolism, drug response, and telomere length regulation (10,11). Most importantly, copper metabolism has been demonstrated to be closely related to tumorigenesis (12,13) and to be involved in regulating tumor proliferation and migration and promoting cisplatin resistance (14). Currently, some risk models developed using CRGs data have already shown to be promising as prognostic indicators for patients with a wide range of cancers including HCC (15-17). For example, Zhang et al. (15) established a cuproptosis-related lncRNAs model in HCC that could predict the survival outlook as well as some immune checkpoints expression; however, the study did not deeply analyze the immune profiles and the predictive value of the risk signature for drugs other than immunotherapy. In another study, a signature based on CIRGs showed a high performance in predicting treatment for HCC patients, but this study did not evaluate the correlation between the model and immune landscape, such as TIME, immune checkpoint genes and immune cells, and the prognostic prediction of the model at 1, 3 and 5 years remains to be improved (16). Therefore, we intended to build a risk signature for HCC based on CIRGs and to conduct a comprehensive analysis of immune profiles and the risk signature’s predictive value in prognosis, responses to different kinds of drugs and even potential small-molecule inhibitors.
The risk signature was constructed based on seven CIRGs screened from the TCGA database. Several of these CIRGs have been shown to be linked to the progression and survival of HCC. KLKB1 expression was low in HCC patients, and individuals with lower serum KLKB1 levels showed significantly poor survival than those with higher expression by as demonstrated Che et al. (18). Two studies both found that PRDX1 was highly expressed in HCC samples, which related to tumor angiogenesis and poor prospects for survival (19,20). Several studies have consistently found that LECT2 is expressed at low levels in HCC tissues, which was found to be markedly related to early recurrence and poor prospects for survival in HCC patients (21-23). It has been reported that EPO is abundantly expressed in HCC tissues, and linked to HCC patients’ terrible survival prospects (24), whereas IL18RAP was revealed to be lowly expressed in HCC tissues (25). The risk signature based on seven CIRGs worked excellently in forecasting survival in the training and validation datasets. Besides, the risk score was discovered to be associated with important HCC clinical factors such as tumor histological grade, pathological stage, and T stage. Therefore, we concluded that the novel risk signature proposed in this study exhibited excellent performance in predicting HCC prognosis.
GO analysis found that DEGs were primarily abundant in the synthesis of genetic material and cell division. Considering that exuberant proliferation is an important feature of malignancies, the results supported the value of the risk signature in identifying HCC malignancy. The research conducted by KEGG found that DEGs were largely related to cell cycle and p53 signaling pathways. p53 is a classical anti-oncogene that encodes a transcription factor controlling the initiation of the cell cycle. Abnormalities in p53 are associated with the development of diverse cancers, including HCC (26). Recently, p53 loss or mutation has been reported to have the potential to inhibit immune signaling and allow immune evasion by reducing MHC-I delivery and increasing MDSC and Treg recruitment (27,28). However, copper can alter structure and function of p53 protein, thereby regulating the HCC cell cycle (29,30). Therefore, the link between the CIRGs identified in this study and the p53 signaling pathway deserves further investigation. This study discovered that the model was closely associated with multiple metabolism-related pathways by GSEA, and it has been proven that cytochrome P450 metabolism (31), fatty acid metabolism (32) and amino acid metabolism (33) may engage in HCC development, further demonstrating that metabolism plays an influential role in HCC.
TMB is an indicator to evaluate the frequency of gene mutations, and high TMB results in more antigens on the cell surface, which can be easily attacked by the immune system. Therefore, TMB is considered a predictor of immunotherapy sensitivity (34); however, TMB is not capable of predicting the response to immunotherapy across all cancer types for unidentified reasons (35). This study discovered that high-risk patients harbored higher mutation frequency and TMB, but showed a poor response to immunotherapy, supporting that TMB is an imperfect biomarker for evaluating the treatment outcome of immunotherapy in HCC. Furthermore, we observed that high-TMB patients exhibited worse survival outlook, possibly because patients with high TMB tend to carry more mutated genes and genetically heterogeneous factors, which may mediate drug resistance and tumor evolution (36,37). Current findings have shown that sustaining antigen exposure caused by TMB causes T-cell dysfunction (38), which may lead to a poor survival.
TIME is highly associated with immune cell invasion and immunological escape (39,40). Higher immune, stromal, and ESTIMATE scores indicated higher contents of immune, stromal, and normal cells in the TIME, respectively, whereas lower tumor purity scores indicated fewer tumor cells. Combined with the findings of this study, low-risk patients had fewer tumor cells and stronger immune response than high-risk patients, proving the signature’s value in predicting HCC risk. The low-risk group had high infiltration levels of B cells, CD8+ T cells, DCs, Mast cells, Neutrophils, NK cells, pDCs, T helper cells, Th1 cells, and TIL, while having low infiltration levels of Macrophages. The infiltrated cells in low-risk patients were mainly responsible for phagocytosis or related to reinforcing the immunoreaction of the host. Macrophages are derived mostly from monocytes and can facilitate in the initiation and progression of cancer via several different mechanisms (41,42); thus, it is not difficult to understand that more Macrophages occur in high-risk patients. When comparing discrepancies in immune functions among different risk groups, it was discovered that immune function scores were lower in high-risk patients, which matched poorer immunoreaction and lower immune cell infiltration levels shown in this group, as described above.
As PD-1 and CTLA4 are the dominant marketable targets for immunotherapy, this study classified HCC patients into four categories depending on PD-1 and CTLA4 expression condition, and discovered that IPS was higher in low-risk patients across all four categories, indicating that low-risk patients may profit more by immunotherapy than high-risk patients. These findings corroborated what TMB, TIME, immune cell infiltration, and immune function assessments found. We noticed that low-risk patients had higher IC50 values for sorafenib, doxorubicin, gemcitabine, and AKT inhibitors, indicating that low-risk patients are less responsive to the above drugs or potential drugs. Thus, the risk signature is not only accurate in assessing HCC survival outlook but also benefits in guiding clinical management.
Conclusions
In conclusion, the risk signature based on CIRGs presented in this study performed exceptionally well in forecasting both prognosis and treatment benefits for HCC patients, and it could serve as a good reference and tool in medical management of HCC. Nonetheless, this research contains a few flaws. The conclusions based on public databases have not been confirmed in the cohort of investigator, and its applicability in the real world needs to be further verified.
Acknowledgments
Funding: This work was supported by
Footnote
Reporting Checklist: The authors have completed the TRIPOD reporting checklist. Available at https://tcr.amegroups.com/article/view/10.21037/tcr-23-2182/rc
Peer Review File: Available at https://tcr.amegroups.com/article/view/10.21037/tcr-23-2182/prf
Conflicts of Interest: All authors have completed the ICMJE uniform disclosure form (available at https://tcr.amegroups.com/article/view/10.21037/tcr-23-2182/coif). The authors have no conflicts of interest to declare.
Ethical Statement: The authors are accountable for all aspects of the work in ensuring that questions related to the accuracy or integrity of any part of the work are appropriately investigated and resolved. The study was conducted in accordance with the Declaration of Helsinki (as revised in 2013).
Open Access Statement: This is an Open Access article distributed in accordance with the Creative Commons Attribution-NonCommercial-NoDerivs 4.0 International License (CC BY-NC-ND 4.0), which permits the non-commercial replication and distribution of the article with the strict proviso that no changes or edits are made and the original work is properly cited (including links to both the formal publication through the relevant DOI and the license). See: https://creativecommons.org/licenses/by-nc-nd/4.0/.
References
- Cantor JR, Sabatini DM. Cancer cell metabolism: one hallmark, many faces. Cancer Discov 2012;2:881-98. [Crossref] [PubMed]
- Eagon PK, Teepe AG, Elm MS, et al. Hepatic hyperplasia and cancer in rats: alterations in copper metabolism. Carcinogenesis 1999;20:1091-6. [Crossref] [PubMed]
- Tsvetkov P, Coy S, Petrova B, et al. Copper induces cell death by targeting lipoylated TCA cycle proteins. Science 2022;375:1254-61. [Crossref] [PubMed]
- Yang JD, Hainaut P, Gores GJ, et al. A global view of hepatocellular carcinoma: trends, risk, prevention and management. Nat Rev Gastroenterol Hepatol 2019;16:589-604. [Crossref] [PubMed]
- Xia C, Dong X, Li H, et al. Cancer statistics in China and United States, 2022: profiles, trends, and determinants. Chin Med J (Engl) 2022;135:584-90. [Crossref] [PubMed]
- Marrero JA, Kulik LM, Sirlin CB, et al. Diagnosis, Staging, and Management of Hepatocellular Carcinoma: 2018 Practice Guidance by the American Association for the Study of Liver Diseases. Hepatology 2018;68:723-50. [Crossref] [PubMed]
- Llovet JM, Kelley RK, Villanueva A, et al. Hepatocellular carcinoma. Nat Rev Dis Primers 2021;7:6. [Crossref] [PubMed]
- Voli F, Valli E, Lerra L, et al. Intratumoral Copper Modulates PD-L1 Expression and Influences Tumor Immune Evasion. Cancer Res 2020;80:4129-44. [Crossref] [PubMed]
- Charoentong P, Finotello F, Angelova M, et al. Pan-cancer Immunogenomic Analyses Reveal Genotype-Immunophenotype Relationships and Predictors of Response to Checkpoint Blockade. Cell Rep 2017;18:248-62. [Crossref] [PubMed]
- Lutsenko S. Human copper homeostasis: a network of interconnected pathways. Curr Opin Chem Biol 2010;14:211-7. [Crossref] [PubMed]
- Lin Z, Gao H, Wang B, et al. Dietary Copper Intake and Its Association With Telomere Length: A Population Based Study. Front Endocrinol (Lausanne) 2018;9:404. [Crossref] [PubMed]
- Feng Y, Zeng JW, Ma Q, et al. Serum copper and zinc levels in breast cancer: A meta-analysis. J Trace Elem Med Biol 2020;62:126629. [Crossref] [PubMed]
- Abdullah KM, Kaushal JB, Takkar S, et al. Copper metabolism and cuproptosis in human malignancies: Unraveling the complex interplay for therapeutic insights. Heliyon 2024;10:e27496. [Crossref] [PubMed]
- Shanbhag VC, Gudekar N, Jasmer K, et al. Copper metabolism as a unique vulnerability in cancer. Biochim Biophys Acta Mol Cell Res 2021;1868:118893. [Crossref] [PubMed]
- Zhang G, Sun J, Zhang X. A novel Cuproptosis-related LncRNA signature to predict prognosis in hepatocellular carcinoma. Sci Rep 2022;12:11325. [Crossref] [PubMed]
- Zhang Y, Sui P, Zhong C, et al. Development and Validation of the novel Cuproptosis- and Immune-related Signature for Predicting Prognosis in Hepatocellular Carcinoma. J Cancer 2024;15:2260-75. [Crossref] [PubMed]
- Zhang Y, Li X, Li X, et al. Comprehensive analysis of cuproptosis-related long noncoding RNA immune infiltration and prediction of prognosis in patients with bladder cancer. Front Genet 2022;13:990326. [Crossref] [PubMed]
- Che YQ, Zhang Y, Li HB, et al. Serum KLKB1 as a Potential Prognostic Biomarker for Hepatocellular Carcinoma Based on Data-Independent Acquisition and Parallel Reaction Monitoring. J Hepatocell Carcinoma 2021;8:1241-52. [Crossref] [PubMed]
- Zhang S, Li X, Zheng Y, et al. Single cell and bulk transcriptome analysis identified oxidative stress response-related features of Hepatocellular Carcinoma. Front Cell Dev Biol 2023;11:1191074. [Crossref] [PubMed]
- Sun QK, Zhu JY, Wang W, et al. Diagnostic and prognostic significance of peroxiredoxin 1 expression in human hepatocellular carcinoma. Med Oncol 2014;31:786. [Crossref] [PubMed]
- Qin J, Sun W, Zhang H, et al. Prognostic value of LECT2 and relevance to immune infiltration in hepatocellular carcinoma. Front Genet 2022;13:951077. [Crossref] [PubMed]
- Chu TH, Ko CY, Tai PH, et al. Leukocyte cell-derived chemotaxin 2 regulates epithelial-mesenchymal transition and cancer stemness in hepatocellular carcinoma. J Biol Chem 2022;298:102442. [Crossref] [PubMed]
- L'Hermitte A, Pham S, Cadoux M, et al. Lect2 Controls Inflammatory Monocytes to Constrain the Growth and Progression of Hepatocellular Carcinoma. Hepatology 2019;69:160-78. [Crossref] [PubMed]
- Huang C, Zhang C, Sheng J, et al. Identification and Validation of a Tumor Microenvironment-Related Gene Signature in Hepatocellular Carcinoma Prognosis. Front Genet 2021;12:717319. [Crossref] [PubMed]
- Xu D, Wang Y, Wu J, et al. Systematic Characterization of Novel Immune Gene Signatures Predicts Prognostic Factors in Hepatocellular Carcinoma. Front Cell Dev Biol 2021;9:686664. [Crossref] [PubMed]
- Zhao B, Zhao W, Wang Y, et al. Connexin32 regulates hepatoma cell metastasis and proliferation via the p53 and Akt pathways. Oncotarget 2015;6:10116-33. [Crossref] [PubMed]
- Ghosh M, Saha S, Bettke J, et al. Mutant p53 suppresses innate immune signaling to promote tumorigenesis. Cancer Cell 2021;39:494-508.e5. [Crossref] [PubMed]
- Blagih J, Buck MD, Vousden KH. p53, cancer and the immune response. J Cell Sci 2020;133:jcs237453. [Crossref] [PubMed]
- Tassabehji NM, VanLandingham JW, Levenson CW. Copper alters the conformation and transcriptional activity of the tumor suppressor protein p53 in human Hep G2 cells. Exp Biol Med (Maywood) 2005;230:699-708. [Crossref] [PubMed]
- Narayanan VS, Fitch CA, Levenson CW. Tumor suppressor protein p53 mRNA and subcellular localization are altered by changes in cellular copper in human Hep G2 cells. J Nutr 2001;131:1427-32. [Crossref] [PubMed]
- Wang K, Shi JH, Gao J, et al. Arachidonic acid metabolism CYP450 pathway is deregulated in hepatocellular carcinoma and associated with microvascular invasion. Cell Biol Int 2024;48:31-45. [Crossref] [PubMed]
- Xu K, Xia P, Chen X, et al. ncRNA-mediated fatty acid metabolism reprogramming in HCC. Trends Endocrinol Metab 2023;34:278-91. [Crossref] [PubMed]
- Zhang Q, Wei T, Jin W, et al. Deficiency in SLC25A15, a hypoxia-responsive gene, promotes hepatocellular carcinoma by reprogramming glutamine metabolism. J Hepatol 2024;80:293-308. [Crossref] [PubMed]
- Chan TA, Yarchoan M, Jaffee E, et al. Development of tumor mutation burden as an immunotherapy biomarker: utility for the oncology clinic. Ann Oncol 2019;30:44-56. [Crossref] [PubMed]
- McGrail DJ, Pilié PG, Rashid NU, et al. High tumor mutation burden fails to predict immune checkpoint blockade response across all cancer types. Ann Oncol 2021;32:661-72. [Crossref] [PubMed]
- Bozic I, Reiter JG, Allen B, et al. Evolutionary dynamics of cancer in response to targeted combination therapy. Elife 2013;2:e00747. [Crossref] [PubMed]
- Valero C, Lee M, Hoen D, et al. The association between tumor mutational burden and prognosis is dependent on treatment context. Nat Genet 2021;53:11-5. [Crossref] [PubMed]
- Ghorani E, Reading JL, Henry JY, et al. The T cell differentiation landscape is shaped by tumour mutations in lung cancer. Nat Cancer 2020;1:546-61. [Crossref] [PubMed]
- Zhu KL, Su F, Yang JR, et al. TP53 to mediate immune escape in tumor microenvironment: an overview of the research progress. Mol Biol Rep 2024;51:205. [Crossref] [PubMed]
- Barkley D, Moncada R, Pour M, et al. Cancer cell states recur across tumor types and form specific interactions with the tumor microenvironment. Nat Genet 2022;54:1192-201. [Crossref] [PubMed]
- Cassetta L, Pollard JW. Targeting macrophages: therapeutic approaches in cancer. Nat Rev Drug Discov 2018;17:887-904. [Crossref] [PubMed]
- Wang H, Wang X, Zhang X, et al. The promising role of tumor-associated macrophages in the treatment of cancer. Drug Resist Updat 2024;73:101041. [Crossref] [PubMed]