Construction of m7G RNA modification-related prognostic model and prediction of immune therapy response in hepatocellular carcinoma
Highlight box
Key findings
• We identified a subtype related with m7G RNA modification and construct a risk model in hepatocellular carcinoma (HCC).
What is known and what is new?
• m7G RNA modification could involve in the progression of HCC. m7G RNA modification could guide the treatment of HCC.
• m7G RNA modification could predict the prognosis in HCC. Risk model and nomogram could predict the survival rate in HCC patients. m7G could predict the immune response in HCC.
What is the implication, and what should change now?
• Our study could help the patients in HCC to predict the prognosis and immune response.
Introduction
The study of RNA modifications has shown that such modifications potentially influence all RNA mechanisms, such as localization, stability, and splicing (1). The methylation of guanosine on internal RNAs at position N7 (also known as m7G) has been discovered in all domains of life, and it may play a role in human disease (2). Several research reports have illustrated that abnormal m7G RNA modification performs a critical function in tumor progression, therefore, it is of great significance to screen the genes related with m7G RNA modification that play a role in tumor prognosis.
Hepatocellular carcinoma (HCC) is the most frequent kind of tumor originating in the liver. In recent years, there has been a continued elevation in both the morbidity and death rates associated with HCC (3). Hepatitis virus infection, alcohol, and improper use of drugs are major risk factors for HCC (4). Surgery is still the primary treatment in HCC patients. With the improvement of imaging technology and the increased attention given to HCC, the detection rate of HCC is gradually increasing (5). However, it is still a challenge to give a prognostic prediction for the HCC patients today. Therefore, it is of great necessity to discover new subtypes and biological markers to better treat HCC.
Our study builds on existing research to identify different subtypes related with key regulators of m7G RNA modification and construct a prognostic model to investigate their potential role in HCC. We generated a prognostic risk signature prediction model that divided HCC patients into two categories depending on the optimal cutoff value. According to validation set, our model demonstrated a positive predictive performance for HCC patients. We also performed the functional annotation of these genes and their related genes using bioinformatics methods. Finally, we investigated the relationship between the gene expression and the response of immune therapy. We present this article in accordance with the TRIPOD reporting checklist (available at https://tcr.amegroups.com/article/view/10.21037/tcr-24-22/rc).
Methods
Consensus clustering and differential expression analysis
This study was conducted in accordance with the Declaration of Helsinki (as revised in 2013). Genes currently considered to be regulators of m7G RNA modification were acquired from the literature retrieval and gene set enrichment analysis (GSEA) database (http://software.broadinstitute.org/gsea/index.jsp) (6). The Cancer Genome Atlas (TCGA) database (7) was searched for data on gene expression as well as clinical data of patients with HCC. Cluster analysis was performed using ConsensusClusterPlus and the optimal number of cluster was determined using the empirical cumulative distribution function plot. The score of m7G RNA modification in different subtypes were performed by single sample GSEA (ssGSEA) method. Differential analysis was conducted with R program, and the differentially expressed genes (DEGs) were identified under the condition of the adjusted P value (Padj) <0.05 coupled with |log2FC| >1.
Cell culture
We selected HCC cell line, Huh-7, from BeNa Culture collection company. And human normal liver tissue cell line THLE-2 and L02 were purchased from American type culture collection (ATCC). Cells were cultured in Dulbecco’s modified eagle medium (DMEM) medium supplemented with 10% fetal bovine serum (FBS). They all cultured in an incubator with the condition of 5% carbon and 37 ℃.
Western blot
Total protein of Huh-7, THLE-2 and L02 cells were selected with Radio Immunoprecipitation Assay (RIPA) buffer, and then performed electrophoresis in 10% agarose and transferred onto polyvinylidene fluoride (PVDF) membrane. We used 5% skimmed milk for blocking. The primary antibody was incubated with the membrane overnight at a temperature of 4 ℃. The secondary antibody was incubated for 2 hours at normal temperature and centrifuged at 4 ℃. The results of western blot were analyzed by ImageJ, photoshop and Graphpad prism9.
Prognostic model construction and validation
The least absolute shrinkage and selection operator (LASSO) Cox regression method was used to select the prognostic related key genes. We calculated risk score from LASSO model and constructed a prognostic model with high risk and low risk two groups. Receiver operating characteristic (ROC) curves were utilized to examine the effectiveness of the model as a tool for prognosis prediction. Validation set GSE14520 (8) was selected from GEO database (https://www.ncbi.nlm.nih.gov/geo/) (9).
Protein-protein interactions (PPI) network construction and enrichment analysis
The PPI network was constructed using the GeneMANIA database (https://genemania.org/) (10) and DAVID database (https://david.ncifcrf.gov/) (11) was utilized for enrichment analysis of key genes and their related genes. R software was used to complete this visualization. To determine the possible molecular processes or functional pathways, GSEA (http://software.broadinstitute.org/gsea/index.jsp) was applied (12).
Immune infiltration analysis
Cibersort method (13) was used to describe the immune infiltration between two different subtypes. Tumor Immune Dysfunction and Exclusion database (TIDE) method was performed to compare the immunotherapy response (14). The immune therapy response cohort GSE126044 (15) was used to show the relationship between the gene expression and the response result. We performed visualization by R software.
Statistical analysis
R software and its resource packages were used for statistical analysis and the creation of the related visualizations. The differential expression was calculated using a Wilcoxon Rank Sum Test or Student’s t-test. In this investigation, Kaplan-Meier plots were created, and log-rank tests were carried out. For all statistical tests in this analysis, P<0.05 was established as the criterion for determining statistically significant differences.
Results
Identification of m7G RNA modification-related subtypes by consensus cluster
By consensus cluster method, we first divided the TCGA-LIHC database into two groups (Figure 1A,1B). In this cohort, there were 378 samples of HCC patients with clinical information. We calculated the enrichment score of m7G RNA modification related genes in the two groups and found that one group (C1) with low score and one group (C2) with high score (Figure 1C). Next, we performed the survival curve between the two groups and we found that high score group was related with unfavorable prognosis (Figure 1D). The different expression genes were selected by limma package and we made an intersection with m7G RNA modification related genes (Figure 1E). We finally found four genes (WDR4, EIF3D, NCBP2 and AGO2) as our key genes. We screened different function by gene ontology (GO) and Kyoto Encyclopedia of Genes and Genomes (KEGG) analyses between two groups (Figure 1F,1G).
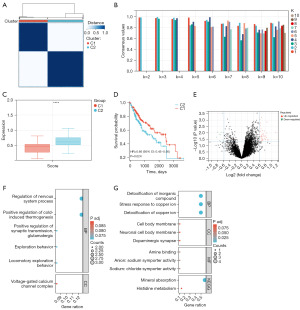
Different genes expression and validation by experimental method
We used key genes to perform differential analysis on the TCGA-LIHC datasets. The expression levels of WDR4, EIF3D, NCBP2, and AGO2 were considerably elevated in HCC tumor specimens in contrast with those in normal specimens. These four genes satisfied the requirements for assessment (Padj <0.05, |log2FC|>1.0) and were identified as key m7G-related genes in HCC. The unpaired box plots were used to show their differential expression in HCC (Figure 2A-2D). By using western blot, the levels of WDR4, NCBP2, EIF3D, and AGO2 in HCC cell lines were elevated compared with those in normal liver cell lines. We found the different expression of the four genes by western blot (Figure 2E-2H). By using image, the different expression of four genes were visualized (Figure 2I-2L).
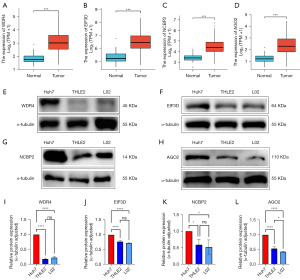
Construction and validation of a genetic risk score model for HCC patient
We performed univariate analysis and visualization by forest map to find the correlation between the four genes and the prognosis of patients with HCC. We used TCGA-LIHC database to find the relationship between the expression of the four genes and the prognostic value. Depending on the prognostic curves, we found that with the high expression of WDR4 and NCBP2, the prognostic of HCC patients were worse in overall survival (OS) and progress-free survival (PFS) (Figure 3A-3D). However, the expression of AGO2 had no prognostic significance in OS and the expression of EIF3D had no prognostic significance in PFS in TCGA-LIHC database (Figure 3E-3H).
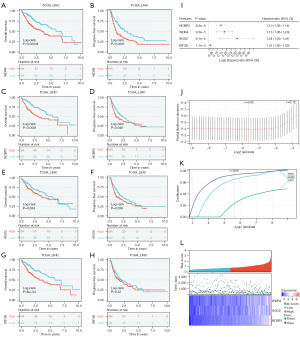
To further exam the prognostic value of these four genes, we selected three genes as our key genes through LASSO Cox regression analysis (Figure 3I-3K). WDR4, AGO2, and NCBP2, were selected to establish the HCC risk model. The risk score was calculated: risk score = (0.066 × expression value of WDR4) + (0.023 × expression value of AGO2) + (0.074 × expression value of NCBP2). Based on the computational process, we divide into high-risk group and low risk group by the optimal cut-off value of approximately 1.017. A dot plot was performed to show the survival rate of each patient and a heatmap was to depict the differential expression of 3 key genes (Figure 3L).
We found a significant prognostic difference and high-risk group had a poor prognosis in K-M curve (P=9.7e−8) (Figure 4A). After that, the ROC curve and AUC was calculated to exam the efficiency of this model (Figure 4B). To further validate this model, we selected another dataset GSE14520 from GEO database and calculate risk score by the same method. Tumor and paired non-tumor samples of 338 patients contained in this dataset. K-M curve and ROC curve show that this model can predict prognosis in another dataset (Figure 4C,4D). To further investigate the prognostic value of our three key genes, we established a nomogram based on the expression of our key genes. We could calculate the score of every patient by this diagram and predict the survival probability (Figure 5).
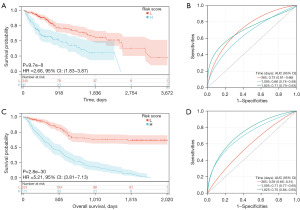
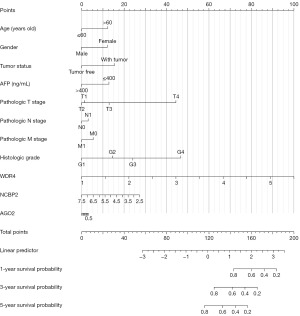
In conclusion, we found that this three-gene signature model has the most accurate capability for predicting the prognosis of patients with HCC.
Establishment of the PPI network and functional annotation of key genes in HCC
After completing the differential analysis, we further identified genes related to the key genes using the GeneMANIA database and finished the PPI network (Figure 6A). In addition, the functions of the three genes and their corresponding genes were evaluated by GO and KEGG in DAVID. As depicted in the map (Figure 6B), a strong enrichment of these genes was found in the biological processes (BP) category, including the development of translation initiation complex in the cytoplasm, assembly of the ribonucleoprotein complex, organization of the subunits of the ribonucleoprotein complex, cytoplasmic translation, and initiation of translation in the cytoplasm. Moreover, these genes played a role in cellular components (CC), such as translation initiation factor 3 complex in eukaryotic cells, 48S pre-initiation complex in eukaryotic cells, 43S pre-initiation complex in eukaryotic cells, translation pre-initiation complex, and cytoplasmic ribonucleoprotein granule. In addition, these genes also prominently affected the activity of molecular functions (MF), including RNA binding, translation factor, tRNA methyltransferase, translation initiation factor, RNA methyltransferase, catalysis, and acting on RNA. In KEGG analysis, we found these genes enriched in some pathways, including RNA transport, Spliceosome, and mRNA surveillance pathway.
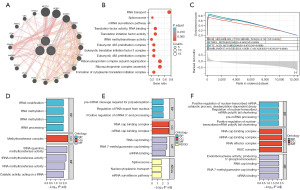
Furthermore, the findings of the GSEA highlighted that the tumor markers could be implicated in the drug-metabolizing cytochrome P450; retinol metabolism; metabolism of fatty acids; complement and coagulation cascades (Figure 6C).
Besides, we investigated the function of the three key genes by GO and KEGG methods. As the bar charts shown, we found that WDR4 mainly involved in the process of RNA methylation and tRNA modification and it mainly joined these processes by activating RNA methyltransferase (Figure 6D). NCBP2 mainly involved in pre-mRNA cleavage required for polyadenylation, regulation of RNA export from nucleus and positive regulation of mRNA 3’-end processing. And the molecular function including the binding of RNA, m7G-cap and snRNA (Figure 6E). We also found that AGO2 could join in the positive regulation of nuclear-transcribed mRNA catabolic processing, deadenylation-dependent decay and pre-miRNA processing. And it played a role in molecular function as endoribonuclease activity, producing 5’-phosphomonoesters, RNA cap binding, m7G cap binding and siRNA binding (Figure 6F).
Immune infiltration analysis of key genes in HCC
By Cibersort method, we performed immune infiltration between C1 and C2, we found that the different expression of Tregs (Figure 7A). We used TIDE method to evaluate the immune response between two groups, finally, the immune response rate of the high expression group was lower than that of the low expression group (Figure 7B).
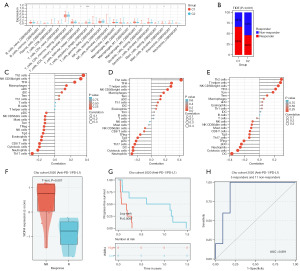
To determine whether the key genes were involved in tumor immunity, we described the immune infiltration of these key genes in HCC by ssGSEA method. We found that the expression of the genes were all positive correlated with the Th2 cells and TFH cells (Figure 7C-7E). This may help them to perform immune escaping in tumor. We further tested the relationship between the expression of the three genes and the result of immune response in immune therapy cohort GSE126044, we found that the high expression of WDR4 related with the non-response to anti PD-1 therapy (Figure 7F-7H).
Discussion
RNA modification may serve as a key point to cure cancer in the future. Some studies have confirmed that m7G is involved in tumor growth and progression (16-18). However, few biomarkers associated with m7G modification have been found to be significant in the cancer field. HCC is a kind of cancer that has a high incidence of morbidity and death all over the globe (19). Despite the multitude of therapeutic methods available for HCC, surgery remains an essential treatment, and the prognosis is poor (20). Therefore, there is an urgent need to find biomarkers that can be used to detect the occurrence of HCC. Recently, some experts and researchers have focused on finding these biomarkers for HCC tumors. Previous studies have identified several prognostic prediction signatures on the basis of mRNA, miRNA, and lncRNA, such as PSMD14, ISG20L2, NRAS, OSGIN1, BRD8, CACYBP, CD320, HSP90AA1, MAPT, FABP6, and NDRG1 (21,22).
We identified m7G RNA modification-related subtypes and key regulators with high expression in HCC tissues and a low expression in normal tissues. Furthermore, to identify genes that are linked to prognosis, we performed LASSO Cox regression analyses. Three genes, including WDR4, AGO2, and NCBP2, were selected and defined as our key genes. Risk score methods that are based on polygenic signatures are being used more often to anticipate the prognosis of patients with malignancies. With the help of three key genes, we built a predictive signature. Surprisingly, we confirmed that this risk score model can effectively anticipate HCC patients’ prognoses. By the validation set, we discovered that the risk signatures constructed in this research have the potential to assist practitioners in producing more accurate personalized survival predictions. Functional annotation and immune analysis were investigated of these key genes to identify their function and their value to immune therapy.
WDR4 has a wide range of effects that prove its critical role in translation and tumor progression. The MYC/WDR4/CCNB1 signaling pathway and its impact on PI3K/AKT and P53 have previously been studied. Furthermore, according to the findings of the research, one of the oncogenic factors in lung cancer is METTL1/WDR4-mediated alteration of m7G tRNA. These findings provide new ideas for studying m7G modification in cancer (23,24). WDR4 may have more complex functions in HCC, which poses a new challenge to the study.
AGO2 is one of the key regulators in tumorigenesis. Numerous research reports have concentrated on the role that AGO2 plays in the onset and progression of tumors (25). Multiple forms of tumors, including colon cancer, HCC, breast cancer, and gastric carcinoma, have been shown to have an upregulated level of AGO2. However, in both lung adenocarcinoma and non-small cell lung cancer, AGO2 has been shown in previous research to inhibit the progression of tumors and/or metastases (26). In recent work, the researchers discovered a new modulatory axis composed of AGO2/miR-185-3p/NRP1 that regulates epithelial-mesenchymal transition (EMT) and the potential for metastasis. These studies proved that AGO2, an important gene, is essential to further investigation (27). Notably, few studies have explored the mechanism of action of NCBP2 in tumors. Therefore, its pivotal role is also worth revealing in future studies in HCC.
There are some limitations of our study. First, due to the limited amount of data, not many groups were assessed. Furthermore, the utility of the key genes identified in this study as drug targets must be investigated further.
Conclusions
In conclusion, the current study suggests that high expression of m7G RNA modification subtype is related with poor prognosis and immune response. WDR4, AGO2, and NCBP2 are key regulators of m7G RNA modification which can be clinically promising biomarkers that can be used to treat HCC. In addition, our risk score model was shown to have a strong link to OS in patients with HCC, which provides a better understanding of the molecular targets of HCC cells so that therapeutic strategies can be improved in the future. Furthermore, we investigated the immune infiltration of key genes in HCC with the hope that they may contribute to future immunotherapy.
Acknowledgments
Funding: This study was supported by
Footnote
Reporting Checklist: The authors have completed the TRIPOD reporting checklist. Available at https://tcr.amegroups.com/article/view/10.21037/tcr-24-22/rc
Data Sharing Statement: Available at https://tcr.amegroups.com/article/view/10.21037/tcr-24-22/dss
Peer Review File: Available at https://tcr.amegroups.com/article/view/10.21037/tcr-24-22/prf
Conflicts of Interest: All authors have completed the ICMJE uniform disclosure form (available at https://tcr.amegroups.com/article/view/10.21037/tcr-24-22/coif). Y.L. reports the National Natural Science Foundation of China (NO.81901576) and the Health Commission of Heilongjiang Province (NO.2018348); S.T. reports the National Natural Science Foundation of China (No.81972724) has been used for this article. The other authors have no conflicts of interest to declare.
Ethical Statement: The authors are accountable for all aspects of the work in ensuring that questions related to the accuracy or integrity of any part of the work are appropriately investigated and resolved. This study was conducted in accordance with the Declaration of Helsinki (as revised in 2013).
Open Access Statement: This is an Open Access article distributed in accordance with the Creative Commons Attribution-NonCommercial-NoDerivs 4.0 International License (CC BY-NC-ND 4.0), which permits the non-commercial replication and distribution of the article with the strict proviso that no changes or edits are made and the original work is properly cited (including links to both the formal publication through the relevant DOI and the license). See: https://creativecommons.org/licenses/by-nc-nd/4.0/.
References
- Zhang X, Zhu WY, Shen SY, et al. Biological roles of RNA m7G modification and its implications in cancer. Biol Direct 2023;18:58. [Crossref] [PubMed]
- Tang Q, Li L, Wang Y, et al. RNA modifications in cancer. Br J Cancer 2023;129:204-21. [Crossref] [PubMed]
- Zou Z, Zhao M, Yang Y, et al. The role of pyroptosis in hepatocellular carcinoma. Cell Oncol (Dordr) 2023;46:811-23. [Crossref] [PubMed]
- Zou YW, Ren ZG, Sun Y, et al. The latest research progress on minimally invasive treatments for hepatocellular carcinoma. Hepatobiliary Pancreat Dis Int 2023;22:54-63. [Crossref] [PubMed]
- Zheng Y, Gao K, Gao Q, et al. Glycoproteomic contributions to hepatocellular carcinoma research: a 2023 update. Expert Rev Proteomics 2023;20:211-20. [Crossref] [PubMed]
- Subramanian A, Tamayo P, Mootha VK, et al. Gene set enrichment analysis: a knowledge-based approach for interpreting genome-wide expression profiles. Proc Natl Acad Sci U S A 2005;102:15545-50. [Crossref] [PubMed]
- Tomczak K, Czerwińska P, Wiznerowicz M. The Cancer Genome Atlas (TCGA): an immeasurable source of knowledge. Contemp Oncol (Pozn) 2015;19:A68-77. [Crossref] [PubMed]
- Li Z, Kwon SM, Li D, et al. Human constitutive androstane receptor represses liver cancer development and hepatoma cell proliferation by inhibiting erythropoietin signaling. J Biol Chem 2022;298:101885. [Crossref] [PubMed]
- Barrett T, Edgar R. Gene expression omnibus: microarray data storage, submission, retrieval, and analysis. Methods Enzymol 2006;411:352-69. [Crossref] [PubMed]
- Warde-Farley D, Donaldson SL, Comes O, et al. The GeneMANIA prediction server: biological network integration for gene prioritization and predicting gene function. Nucleic Acids Res 2010;38:W214-20. [Crossref] [PubMed]
- Huang da W. Sherman BT, Lempicki RA. Systematic and integrative analysis of large gene lists using DAVID bioinformatics resources. Nat Protoc 2009;4:44-57. [Crossref] [PubMed]
- Ru B, Wong CN, Tong Y, et al. TISIDB: an integrated repository portal for tumor-immune system interactions. Bioinformatics 2019;35:4200-2. [Crossref] [PubMed]
- Newman AM, Liu CL, Green MR, et al. Robust enumeration of cell subsets from tissue expression profiles. Nat Methods 2015;12:453-7. [Crossref] [PubMed]
- Gao J, Aksoy BA, Dogrusoz U, et al. Integrative analysis of complex cancer genomics and clinical profiles using the cBioPortal. Sci Signal 2013;6:pl1. [Crossref] [PubMed]
- Cho JW, Hong MH, Ha SJ, et al. Genome-wide identification of differentially methylated promoters and enhancers associated with response to anti-PD-1 therapy in non-small cell lung cancer. Exp Mol Med 2020;52:1550-63. [Crossref] [PubMed]
- Chen W, Feng P, Song X, et al. iRNA-m7G: Identifying N(7)-methylguanosine Sites by Fusing Multiple Features. Mol Ther Nucleic Acids 2019;18:269-74. [Crossref] [PubMed]
- Lin S, Liu Q, Lelyveld VS, et al. Mettl1/Wdr4-Mediated m(7)G tRNA Methylome Is Required for Normal mRNA Translation and Embryonic Stem Cell Self-Renewal and Differentiation. Mol Cell 2018;71:244-255.e5. [Crossref] [PubMed]
- Deng Y, Zhou Z, Ji W, et al. METTL1-mediated m(7)G methylation maintains pluripotency in human stem cells and limits mesoderm differentiation and vascular development. Stem Cell Res Ther 2020;11:306. [Crossref] [PubMed]
- Budny A, Kozłowski P, Kamińska M, et al. Epidemiology and risk factors of hepatocellular carcinoma. Pol Merkur Lekarski 2017;43:133-9. [PubMed]
- Carr BI. Introduction: hepatocellular carcinoma. Semin Oncol 2012;39:367-8. [Crossref] [PubMed]
- Li L, Lei Q, Zhang S, et al. Screening and identification of key biomarkers in hepatocellular carcinoma: Evidence from bioinformatic analysis. Oncol Rep 2017;38:2607-18. [Crossref] [PubMed]
- Berasain C, Castillo J, Prieto J, et al. New molecular targets for hepatocellular carcinoma: the ErbB1 signaling system. Liver Int 2007;27:174-85. [Crossref] [PubMed]
- Xia P, Zhang H, Xu K, et al. MYC-targeted WDR4 promotes proliferation, metastasis, and sorafenib resistance by inducing CCNB1 translation in hepatocellular carcinoma. Cell Death Dis 2021;12:691. [Crossref] [PubMed]
- Ma J, Han H, Huang Y, et al. METTL1/WDR4-mediated m(7)G tRNA modifications and m(7)G codon usage promote mRNA translation and lung cancer progression. Mol Ther 2021;29:3422-35. [Crossref] [PubMed]
- Liu X, Meng X, Peng X, et al. Impaired AGO2/miR-185-3p/NRP1 axis promotes colorectal cancer metastasis. Cell Death Dis 2021;12:390. [Crossref] [PubMed]
- Chen Y, Yang F, Fang E, et al. Circular RNA circAGO2 drives cancer progression through facilitating HuR-repressed functions of AGO2-miRNA complexes. Cell Death Differ 2019;26:1346-64. [Crossref] [PubMed]
- Chen XJ, Zhang CJ, Wang YH, et al. Retinal Degeneration Caused by Ago2 Disruption. Invest Ophthalmol Vis Sci 2021;62:14. [Crossref] [PubMed]