Signature identification based on immunogenic cell death-related lncRNAs to predict the prognosis and immune activity of patients with endometrial carcinoma
Highlight box
Key findings
• A risk model based on six immunogenic cell death (ICD) related-long non-coding RNAs (lncRNAs) for endometrial carcinoma (EC) was established to predict the prognosis of patients with EC.
What is known and what is new?
• Inducing ICD in tumor cells is a promising new direction for the treatment of cancer.
• This manuscript explored the role of ICD-related lncRNAs in EC and constructed a prognostic model based on the identified ICD-related lncRNAs, which was validated through bioinformatics experiments, cell experiments, and animal experiments.
What is the implication, and what should change now?
• This study provides a potentially useful method of determining prognosis, as well as identifying high-risk groups. This model could also help determine the utility of starting specific treatment regimens in patients, namely, individualized treatment plans.
Introduction
An estimated 65,950 cases of and 12,550 deaths from endometrial carcinoma (EC) were expected to occur in the United States by 2022 (1), making it one of the most prevalent gynecologic malignancies. Over the 21st century, the death rate from EC increased from 0.3% in 1997–2008 to 1.9% in 2008–2018 (2). Despite the favorable prognosis of patients with early-stage EC, the median overall survival (OS) is shorter among patients with relapsed or metastatic disease (3,4) due to a lack of major therapeutic advances. Combination therapy with paclitaxel plus carboplatin is recommended as the first-line treatment for EC. Recent evidence suggests that a novel therapeutic option for EC has emerged in the form of immunotherapy, with promising effects (5). However, the poor reproducibility of the diagnosis of high-grade EC can easily lead to delayed treatment or overtreatment (6). It is therefore essential to have molecular tools to identify high-risk patients early and accurately and select the appropriate treatment options.
Immunogenic cell death (ICD) is a type of regulated cell death (RCD) that drives antigen-specific immune responses. This culminates in immunological memory, mediated by damage-associated molecular patterns (DAMPs), including surface-exposed calreticulin (CRT), secreted adenosine triphosphate (ATP), and the release of high mobility group protein B1 (HMGB1) (7,8). In addition to being the primary initiator of adaptive immunity in the context of infectious and malignant diseases (9), ICD-induced changes in the immune microenvironment may also trigger autoimmune diseases [e.g., some myocarditis (10)] in non-infectious and non-malignant diseases (8), suggesting that ICD has a wide range of immunology applications. Accompanied by the exposure and release of numerous DAMPs, ICD facilitates the recruitment and activation of antigen-presenting cells, contributing to immune infiltration in the tumor microenvironment (TME) (11). The TME includes cancer cells and stromal cells. Infiltrating immune cells including macrophages, natural killer cells, dendritic cells and lymphocytes in stromal cells regulate the progression and invasion of endometrial cancer by releasing growth factors, cytokines, and chemokines (12). Currently, the induction of ICD in tumor cells represents a novel approach to cancer treatment (13-15).
Long non-coding RNA (lncRNA) is a conserved non-protein-coding RNA of more than 200 nucleotides. The abnormal expression or function of these RNAs is closely related to human diseases. Recent studies have found that lncRNAs play an important role in the occurrence and development of EC. For instance, the upregulation of the lncRNA, PCAT1 is associated with E-cadherin down-regulation in EC, which causes invasion and migration of cancer cells (16). LncRNA-ZXF1 regulate the migration, invasion, and proliferation of endometrioid endometrial cancer cells by altering the expression of P21 through two mechanisms (17). LncRNA HOXB-AS3 can affect the lipid metabolism process of endometrial cancer cells by promoting SREBP1 translocation into the nucleus and activating the transcription of downstream lipid metabolism genes (18). In addition, lncRNAs can also modulate the TME to influence tumor progression. In mammary gland tumors, treatment with long intergenic non-coding RNA for kinase activation (LINK-A) locked nucleic acid stabilizes phospholipase C (PLC) components, Rb and p53, making it more susceptible to immune checkpoint inhibitors (ICIs) (19). LncRNA can be isolated from tumor cells or circulating blood to provide pre-clinical easily available, inexpensive, and stable diagnostics that can be used to detect cancer and cancer subtypes (20). However, a considerable amount of information is unknown regarding ICD related-lncRNAs in EC.
Least absolute shrinkage and selection operator (LASSO) regression is a statistical method used for regression analysis, with the goal of obtaining a model with strong interpretability and good predictability (21). Current studies used bioinformatics tools to screen prognostic biomarkers using LASSO regression and constructs a model to predict clinical prognosis outcomes. For example, Li’s team (22) and Huang’s team (23) used the LASSO model to elucidate the roles of copper death related genes in the liver cancer cohort and autophagy related genes in lung cancer patients, respectively, and validated them through in vitro and in vivo models. Model scores can serve as new prognostic factors for cancer patients.
This study aimed to explore the role of ICD related-lncRNAs in EC via bioinformatics and establish a prognostic risk model based on the ICD related-lncRNAs. We also explored immune infiltration and immune cell function across prognostic groups and made treatment recommendations. Meanwhile, this paper adopts the method of consensus clustering to aggregate the existing EC data into subsets of different categories and analyze the difference in survival probability in all the subsets. Immune checkpoint expression and immune scores [including Estimation of STromal and Immune cells in MAlignant Tumor tissues using Expression data (ESTIMATE) score, stromal score, and immune score] of different subsets were investigated. We present this article in accordance with the TRIPOD reporting checklist (available at https://tcr.amegroups.com/article/view/10.21037/tcr-23-2243/rc).
Methods
Transcriptome data and clinical data collection
The transcriptome RNA sequencings were obtained from The Cancer Genome Atlas (TCGA), including 23 normal datasets and 552 tumor datasets. The RNA-seq data were presented as fragments per kilobase of exon model per million mapped reads (FPKM), normalized. Simultaneously, the clinical datasets were retrieved from UCSC Xena, excluding the patients missing OS or with poor OS (less than 30 days).
EC and control samples
This study was conducted in accordance with the Declaration of Helsinki (as revised in 2013). Twenty pairs of EC patients’ tumor tissues and adjacent tissues were collected from the Union Hospital, Tongji Medical College, Huazhong University of Science and Technology, approved by the Institutional Review Board of Tongji Medical College, Huazhong University of Science and Technology (2021-S046) and patients enrolled in the study had provided written informed consent.
Identification of ICD-related lncRNAs
There were 38 ICD-related genes obtained from previous reports about ICD (Table 1). Pearson correlation analysis was performed on 38 ICD-related genes to examine the relationship between genes and lncRNA data expressed in all EC cases. Then, based on Pearson’s correlation analysis (R>0.3, P<0.05), a total of 398 ICD-related lncRNAs were identified. The “igraph” package was used to draw network graphs. The “limma” and “pheatmap” packages were used to draw heatmaps and volcano maps, respectively.
Table 1
Gene | Functions |
---|---|
HMGB1 | Activates tumor antigen-specific T-cell immunity (24) |
CALR | Acts as ‘eat me’ signals and promotes the phagocytosis of tumor cells by DC (25) |
NT5E | Catalyses the hydrolysis of AMP to adenosine (26) |
ANXA-1 | Directs APCs to dying cells (27) |
CXCR3 | Promotes the recruitment of T cells (28) |
IFNA1 | Enhances APC maturation and recruitment of T cells (28) |
IFNB1 | Enhances APC maturation and recruitment of T cells (29) |
ENTPD1 | Expresses on the surface of cells and degrades extracellular ATP to generate ADP and AMP (30) |
CASP8 | Promotes exposure to CRT (31) |
PDIA3 | Forms a complex with CRT as a “eat me” signal (31) |
STING | Increases expression of interferon-stimulated genes and pro-inflammatory cytokines (32-35) |
PIK3CA | Induces ecto-CRT and secretion of ATP (36,37) |
TLR4 | Produces pro-inflammatory factors, such as type I interferons (38) |
BAX | Promotes exposure to CRT (39) |
MER6 | Promotes exposure to CRT (40) |
EIF2AK3 | Promotes exposure to CRT/ERp57 (41) |
LAMP1 | Promotes the release of ATP (42) |
ATG5 | Promotes the release of ATP by dying cells and activates DC cells (43) |
HSP90AA1 | Stimulates the uptake of dead cell-associated antigens (44) |
IL6 | Stimulates T cell proliferation (44) |
IL10 | Inhibits immune cell recruitment (45) |
TNF | Promotes T cells and other killer cells (46) |
CASP1 | Proteolytically cleave and activate the inactive precursor of interleukin-1, a cytokine involved in the processes such as inflammation, septic shock, and wound healing (47) |
IL1R1 | A receptor for interleukin-1 alpha, interleukin-1 beta, and interleukin-1 receptor antagonist (48) |
IL1B | An important mediator of the inflammatory response, and is involved in a variety of cellular activities, including cell proliferation, differentiation, and apoptosis (49) |
NLRP3 | Encode the protein interacting with protein PYCARD/ASC, which contains a caspase recruitment domain, and is a member of the NLRP3 inflammasome complex (50) |
P2RX7 | A ligand-gated ion channel which is responsible for ATP-dependent lysis of macrophages through the formation of membrane pores permeable to large molecules (51) |
LY96 | Associate with toll-like receptor 4 on the cell surface and confers responsiveness to LPS, thus providing a link between the receptor and LPS signaling (52) |
MYD88 | Encode a cytosolic adapter protein that functions as an essential signal transducer in the interleukin-1 and Toll-like receptor signaling pathways (53,54) |
CD4+ | Encode the CD4 membrane glycoprotein of T lymphocytes. initiate or augment the early phase of T-cell activation, and may function as an important mediator of indirect neuronal damage in infectious and immune-mediated diseases of the central nervous system (55-57) |
CD8+A | A cell surface glycoprotein found on most cytotoxic T lymphocytes that mediates efficient cell-cell interactions within the immune system (58) |
CD8+B | A cell surface glycoprotein found on most cytotoxic T lymphocytes that mediates efficient cell-cell interactions within the immune system (59) |
FOXP3 | A member of the forkhead/winged-helix family of transcriptional regulators (60) |
IFNG | Encode a soluble cytokine that is a member of the type II interferon class (61) |
IFNGR1 | Encode the ligand-binding chain (alpha) of the gamma interferon receptor (62) |
IL17A | A proinflammatory cytokine produced by activated T cells (63) |
IL17RA | A proinflammatory cytokine secreted by activated T-lymphocytes (64) |
PRF1 | Form membrane pores that allow the release of granzymes and subsequent cytolysis of target cells (65) |
DC, dendritic cells; AMP, adenosine monophosphate; APCs, antigen-presenting cells; ATP, adenosine triphosphate; ADP, adenosine diphosphate; CRT, calreticulin; NLRP3, NOD-like receptor protein 3 inflammasome; LPS, lipopolysaccyaride.
Identification of prognostic ICD-related lncRNAs
A total of 475 patients were randomly assigned at a 1:1 ratio to the training and testing sets using the “caret” package. Univariate Cox regression analysis identified 16 ICD-related lncRNAs that were significantly associated with prognosis in training set (Table S1). The “limma”, “dplyr”, “ggalluvial”, and “ggplot2” packages were used to draw Sankey diagrams of ICD genes and ICD-related lncRNAs. The “limma”, “pheatmap”, “reshape2” and “ggpubr” packages were used to draw heatmaps and boxplots of differential expression of ICD-related lncRNAs explored by univariate Cox regression analysis.
Construction of the risk model
The LASSO (66) was used to create a predictive signature for ICD-related lncRNAs. A 10-fold cross-validation procedure was used to determine the parameter λ in order to prevent the overfitting effect of the model. Based on the optimal λ value, six ICD-related lncRNAs and the corresponding coefficients were selected to establish risk model. The risk model was as follows:
Gene expression is represented by exp, whereas coefficient value is represented by coef.
Validation of the risk model
Patients were divided into high-risk and low-risk groups based on the median of the risk score. The risk model was used to score the individuals within the set, with the median as the cutoff standard. Individuals who scored higher than the median were assigned to a high-risk group, while those who scored below the median were assigned to a low-risk group. The “pheatmap” package was used to produce the risk curve, scatter plot, and heatmap depicting the expression of the six lncRNAs in training, testing, and full sets. For the evaluation of the signature’s predictive accuracy, the time-dependent receiver operating characteristic (ROC) curve was drawn using the “timeROC” R package and the Kaplan-Meier (K-M) survival curves were drawn using the “survival” package. Multivariate and univariate analyses were also conducted to determine whether risk scores and other possible characteristics could be used as independent variables for predicting prognosis; as a result, prognostic indicators were identified.
Construction and verification of nomogram
“Survival” and “regplot” packages were used to establish and verify a prognostic nomogram based on ICD-related lncRNAs and other clinicopathologic features such as age and stage. “rms” R package was used to draw the calibration plots.
Enrichment analyses of the Kyoto Encyclopedia of Genes and Genomes (KEGG) and gene set enrichment analysis (GSEA)
The KEGG assays were used to analyze mainly cell signal pathways regulated by these lncRNAs. A GSEA (http://www.broadinstitute.org/gsea) (67) with a P value <0.05 and a false discovery rate (FDR) value <0.25 was performed on the genomic expression profiles of EC patients to identify genes that displayed differential expression between high-risk and low-risk individuals by non-parametric and unsupervised.
Tumor immune microenvironment analysis
The Tumor IMmune Estimation Resource (TIMER) (68), CIBERSORT (69), QUANTISEQ (70), Microenvironment Cell Populations-counter (MCP-counter) (71), XCELL, and Estimating the Proportion of Immune and Cancer cells (EPIC) algorithms were used to estimate the abundances of immune cells between the high-risk and low-risk groups based on the signature. “scales”, “ggplot2”, “ggtext”, “tidyverse”, “ggpubr” and “reshape2” R packages were used to draw a bubble gram of immune cells in low- and high-risk groups. The “gsva” package was used to quantify the immune cells and pathways between the two groups via single sample GSEA (ssGSEA) (72). “estimate” R package was employed to show stromal score and immune score in low- and high-risk groups. The difference in immune checkpoints between the low- and high-risk groups was determined via the R package above. The Tumor Immune Dysfunction and Exclusion (TIDE) algorithm was used to evaluate the potential response to ICIs therapy using the online tool (www.tide.dfci.harvard.edu/).
Consensus clustering
Consensus clustering (73) was adopted to categorize existing EC data using the “ConsensusClusterPlus” R package. In addition, using the “Rtsne” package, t-Distributed Stochastic Neighbor Embedding (t-SNE) can be employed to examine the cluster distribution of patients by visual inspection.
Drug sensitivity analysis
“limma”, “ggpubr”, “pRRophetic”, and “ggplot2” R packages were used to compare the half inhibitory centration (IC50) of typical chemotherapy drugs between low- and high-risk groups. A Wilcoxon signed-rank test was then used to detect heterogeneous IC50 between the low-risk and high-risk groups.
Isolation and culture of endometrial epithelial cells (ECCs)
A collection of human endometrial tissues was obtained from women who underwent hysterectomy surgery at the Union Hospital, Tongji Medical College, Huazhong University of Science and Technology. A single-cell suspension was prepared by digesting tissue fragments with collagenase type II (100 mg/mL; Sigma, Missouri, USA) and mechanically dissolving them at 37 ℃ for 50–60 min. Separately, cells were dispersed through 400- and 200-mesh sieves (BD Biosciences, New Jersey, USA). DMEM/F12 (Gibco, Massachusetts, USA) was used to rinse and backwash the filter in order to recover epithelial sheets and glands from the surface of the 200-mesh sieve. The cells were seeded in DMEM/F-12 containing antibiotics (100 mg/mL penicillin G sodium, 100 mg/mL streptomycin), and 20% fetal bovine serum (FBS) and incubated in 5% CO2 at 37 ℃.
Cell lines and cell culture
The cells Ishikawa, KLE, RL95-2, and HEC-1B were purchased from Zhong Qiao Xin Zhou Biotechnology (Shanghai, China) and cultured in DMEM/F12 (Gibco) supplemented with 10% FBS (HyClone, Utah, USA). In an incubator containing 5% CO2, cells were maintained at 37 ℃. The experiments were conducted only on cells under the age of P20. The cells were negative for mycoplasma at the beginning and at the end of the experiment.
Quantitative real-time polymerase chain reaction (qRT-PCR)
Total RNA was extracted using Trizol reagent (Invitrogen, California, USA). cDNA was reverse transcribed by HiScript II qRT SuperMix II (Vazyme, Nanjing, China). qPCR was performed by Universal SYBR Green Fast qPCR MIX (ABclonal, Wuhan, China). The RNA expression levels were calculated using β-actin expression as internal references by the 2−ΔΔCT method. The differences in SCARNA9, FAM198B-AS1, FKBP14-AS1, FBXO30-DT, LINC01943, and AL161431.1 expression were tested by t-tests. Graph-Pad Prism (version 8.0.2) was used to create the graphs (* if P<0.05, ** if P<0.01, and *** if P<0.001). The primers are shown in Table S2.
Cell transfection
Ishikawa cells were transfected with sh-FKBP14-AS1, sh-FBXO30-DT, sh-AL161431.1 (Table S3), LINC01943, FAM198B-AS1 and SCARNA9 plasmids (Miaolingbio, Wuhan, China) using Lipofectamine 2000 (Invitrogen) according to manufacturer’s instructions.
Cell Counting Kit-8 (CCK-8) assay
A CCK-8 kit (Bimake, Texas, USA) was used to measure the proliferation of KLE cells. Twelve hours prior to treatment, cells were seeded in 96 well plates at a density of 5,000 cells per well. Doxorubicin (DOX) was incubated at increasing doses for 48 h (range, 100 nM–500 µM). A working solution was then generated by adding 10 µL of CCK-8 reagent to 90 µL of DMEM. 450 nm absorbance was detected using an automatic microplate reader (BioTek, Vermont, USA). A IC50 is defined as the concentration that results in a 50% reduction in proliferation. An analysis of the mean IC50 values was conducted for all experiments in triplicate.
Chemotherapeutics treatments
FKBP14-AS1 siRNA, FBXO30-DT siRNA, AL161431.1 siRNA, LINC01943 plasmids, FAM198B-AS1 plasmids and SCARNA9 plasmids were transfected into KLE cells. After 48 h of incubation, cells were treated for 24 h with DOX (5 µM; Sigma).
ATP release assay
The supernatants with different treatment were examined using an ATP assay kit (Beyotime, Shanghai, China) to quantify the extracellular ATP level following the manufacturer’s instructions. These experiments were performed in triplicate.
CRT externalization measurement—Western blot analysis
The isolation of plasma membrane proteins was performed using the Plasma Membrane Protein Isolation Kit (Invent, Minnesota, USA) according to the manufacturer’s instructions. The BCA protein assay kit (Servicebio, Wuhan, China) was used for the quantitative measurement of the proteins. Protein samples (30 µg) were pipetted into a 10% sodium dodecyl-sulfate polyacrylamide gel electrophoresis (SDS-PAGE) gel for gel electrophoresis and then transferred to polyvinylidene difluoride membranes for analysis. The membranes were incubated with primary antibodies [CRT (1:1,000, ABclonal), Na+/K+ ATPase 1 (1:8,000, Proteintech, Wuhan, China), β-actin (1:3,000, ABclonal)] overnight at 4 ℃. Then, the membranes were incubated with goat anti-rabbit secondary antibodies [HRP Goat Anti-Rabbit IgG (1:8,000, ABclonal)] for 1 h at room temperature. The ChemiDoc MP (Bio-Rad, California, USA) was used to conduct imaging. These experiments were performed in triplicate.
Immunofluorescence
Immunofluorescence was performed by staining EC cells with 30-min fixation in 4% paraformaldehyde. A blocking solution of 5% bovine serum albumin (BSA) was added to the cells for 1 h, followed by overnight incubation with primary antibodies [CRT (1:1,000, ABclonal)] in 5% BSA at 4 ℃. Fluorescein isothiocyanate (FITC)-conjugated AffiniPure goat anti-rabbit IgG (H + L) (BOSTER, California, USA, BA1105, 1:200) was used as the secondary antibody. Nuclei were stained with DAPI (4’,6-diamidino-2-phenylindole) (C1002, Beyotime).
HMGB1 secretion measurement—enzyme linked immunosorbent assay
The supernatants with different treatment were examined using the HMGB1 enzyme-linked immunosorbent assay (Elabscience, Wuhan, China) according to the manufacturer’s instructions. These experiments were performed in triplicate.
Colony formation assay
KLE cells were seeded at a density of 500 cells and then allowed to grow for 2 weeks. 4% methanol were used to fix cells (Servicebio) for 15 min. Then 0.1% violet crystal were used to stain cells (Servicebio) for 30 min.
Tumor xenograft model
A specific pathogen-free (SPF) facility was used to house 48 female 5-week-old BALB/c-nu nude mice purchased from Vital River (Beijing, China). Animal care, feeding and housing were managed uniformly in SPF level facilities. Every 6 mice were divided into 8 groups randomly and injected subcutaneously with normal saline (100 µL), DOX (3 mg/kg/2 days), DOX + FKBP14-AS1 knocked-down EC cells, DOX + FBXO30-DT knocked-down EC cells, DOX + AL161431.1 knocked-down EC cells, DOX + LINC01943 overexpression EC cells, DOX + FAM198B-AS1 overexpression EC cells, DOX + SCARNA9 overexpression EC cells (5×106 cells/site). The tumors were measured every week with calipers for four weeks. After day 28 of cell implantation, the mice were sacrificed, and the tumors were excised and weighed. The mice were euthanized by cervical dislocation. Experiments were performed under a project license (No. 3357) granted by the Tongji Medical College Animal Care Committee, in compliance with guidelines of Huazhong University of Science and Technology for the care and use of animals.
Immunohistochemistry
Dewaxing, dehydration, rehydration, and repair with citric acid were performed on paraffin sections of subcutaneous transplanted tumor tissue in mice. At 4 ℃ overnight, the tissue sections were incubated with the primary antibody [CRT (1:1,000, ABclonal)] after peroxidase blocking. Secondary antibodies were incubated for 1 h at 37 ℃ followed by washing with phosphate buffered saline (PBS). The sections were imaged under microscope.
Statistical analysis
All the data were analyzed in R Studio software (version 2022.02.0+443), strawberry-Perl-5.32.1.1, and GraphPad Prism (version 7). To determine the statistical significance, the K-W test and Wilcoxon test were used based on the threshold of P<0.05. For evaluating the differences between risk groups in terms of OS, the log-rank test was applied. Spearman’s correlation analysis was used to examine the correlations between the two based on P<0.05.
Results
Identification of prognostic ICD-related lncRNAs in EC
Figure 1 illustrates the flowchart of the study design. We conducted a comprehensive literature review (by searching through PubMed, Scopus, and Google Scholar for relevant studies until 2021) to re-collect validated and putative ICD-related genes. ICD-related genes were accepted in the study if all of the following criteria were met: (I) the studies explored the mechanism in ICD and immunological processes and carried out experimental interventions, (II) the studies carried out vaccination assays with immunocompetent, syngeneic mice or other rodents (74), (III) the studies carried out co-culturing of cancer cells and immune cells, (IV) the studies carried out experiments involving ICD biomarkers validations, such as cell surface exposure of CRT, ATP secretion validation and extracellular release of HMGB-1 (75,76). Thirty-eight ICD-related genes were obtained from previous reports. A total of 23 normal samples and 552 tumor samples were obtained from TCGA. There were 153 ICD-related lncRNAs differently expressed between tumor and normal samples were determined through Pearson’s correlation analysis (R>0.3, P<0.05) (Figure 2A); 55 genes were downregulated, 98 genes were upregulated (Figure 2B, Figure S1).
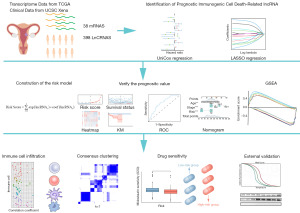
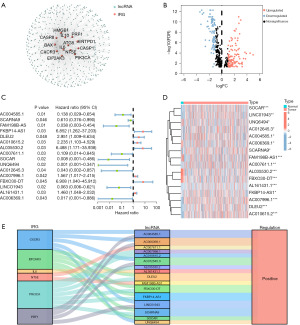
A univariate Cox regression analysis (P<0.05) was performed to identify 16 prognostic ICD-related lncRNAs. Among them, 7 lncRNAs were poor prognostic factors for EC, while the remaining 9 lncRNAs appeared to be protective (Figure 2C, Table S1). The expression of the prognostic lncRNAs in all samples was represented in a heatmap (Figure 2D). Based on the Sankey diagram (Figure 2E), we were able to determine the correlation between these 16 lncRNAs and their corresponding genes. All of the lncRNAs were positively correlated with the gene (Table 2).
Table 2
Gene | LncRNA | cor | P value |
---|---|---|---|
CXCR3 | AC004585.1 | 0.651091206498471 | <0.001 |
CXCR3 | UNQ6494 | 0.461838782808825 | <0.001 |
CXCR3 | AC012645.3 | 0.532188563441362 | <0.001 |
CXCR3 | LINC01943 | 0.597983497534661 | <0.001 |
CXCR3 | AC006369.1 | 0.590073357733589 | <0.001 |
EIF2AK3 | SCARNA9 | 0.427551010434767 | <0.001 |
EIF2AK3 | FKBP14-AS1 | 0.471064518151103 | <0.001 |
EIF2AK3 | DLEU2 | 0.572720703065882 | <0.001 |
EIF2AK3 | SOCAR | 0.488485413188215 | <0.001 |
EIF2AK3 | FBXO30-DT | 0.402592597988471 | <0.001 |
IL6 | AL161431.1 | 0.437236843212603 | <0.001 |
NT5E | FAM198B-AS1 | 0.487772456631103 | <0.001 |
NT5E | AC007611.1 | 0.451563733789954 | <0.001 |
PIK3CA | SCARNA9 | 0.426493491962078 | <0.001 |
PIK3CA | FKBP14-AS1 | 0.471861758198812 | <0.001 |
PIK3CA | DLEU2 | 0.52656059384847 | <0.001 |
PIK3CA | AC010615.2 | 0.46700914056783 | <0.001 |
PIK3CA | AL035530.2 | 0.403690868250551 | <0.001 |
PIK3CA | AC007996.1 | 0.402630249999208 | <0.001 |
PIK3CA | FBXO30-DT | 0.410318377151942 | <0.001 |
PRF1 | AC004585.1 | 0.734166381452014 | <0.001 |
PRF1 | AC012645.3 | 0.450474907446685 | <0.001 |
PRF1 | LINC01943 | 0.690221110880813 | <0.001 |
PRF1 | AC006369.1 | 0.52002327964519 | <0.001 |
ICD, immunogenic cell death; lncRNAs, long non-coding RNAs; cor, correlation.
Establishment and validation of risk model
An arbitrary ratio of 1:1 was applied to all samples to divide them into a training set and testing set, ensuring that the clinical characteristics of the samples from the two subsets were not statistically different (Table 3).
Table 3
Covariates | Type | Total, n (%) | Test, n (%) | Train, n (%) | P value |
---|---|---|---|---|---|
Age | ≤65 years | 274 (57.68) | 134 (56.54) | 140 (58.82) | 0.68 |
>65 years | 199 (41.89) | 102 (43.04) | 97 (40.76) | ||
Unknown | 2 (0.42) | 1 (0.42) | 1 (0.42) | ||
Stage | Stage I | 310 (65.26) | 154 (64.98) | 156 (65.55) | 0.18 |
Stage II | 47 (9.89) | 18 (7.59) | 29 (12.18) | ||
Stage III | 100 (21.05) | 53 (22.36) | 47 (19.75) | ||
Stage IV | 18 (3.79) | 12 (5.06) | 6 (2.52) | ||
Histology | Endometrioid | 277 (58.32) | 138 (58.23) | 139 (58.4) | 0.68 |
Mixed | 11 (2.32) | 6 (2.53) | 5 (2.10) | ||
Serous | 46 (9.68) | 26 (10.97) | 20 (8.40) | ||
Unknown | 141 (29.68) | 67 (28.27) | 74 (31.09) | ||
Grade | Grade 1 | 87 (18.32) | 39 (16.46) | 48 (20.17) | 0.32 |
Grade 2 | 94 (19.79) | 47 (19.83) | 47 (19.75) | ||
Grade 3 | 153 (32.21) | 84 (35.44) | 69 (28.99) | ||
Unknown | 141 (29.68) | 67 (28.27) | 74 (31.09) |
Specifically, we analyzed the training set data and performed LASSO regression to obtain hub genes to construct risk models. Based on the expression of the six lncRNAs in the training set and LASSO regression analysis (Figure 3A,3B, Table S4), the following equation was developed:
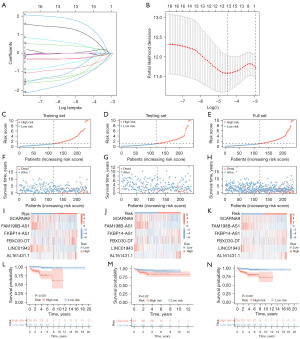
Gene expression is represented by exp. The coefficients were obtained from LASSO regression.
We calculated the risk score for each EC patient by combining the coefficients and expression of the lncRNAs discussed above (Figure 3). Patients in the testing set were assigned to high- and low-risk groups based on the risk model. We compared, in the training and testing set, the distribution of risk score (Figure 3C,3D), survival status (Figure 3F,3G), relevant expressions of the six lncRNAs (Figure 3I,3J), and survival time between low and high-risk group (Figure 3L,3M). The risk score distribution and survival status in the training and testing sets remained relatively consistent, indicating that the risk model was well fitted. The lncRNA expression heatmap showed that FKBP14-AS1, FBXO30-DT and AL161431.1 were increased in cancer tissues whereas LINC01943, FAM198B-AS1 and SCARNA9 were decreased in cancer tissues in all sets, demonstrating the consistency of the sample data in all sets. Most importantly, in the survival curves of the test set and full set, the OS of the low-risk group was higher than that of the high-risk group, indicating that the model predicted the risk well. In conclusion, the above results indicated that the training set and testing set are both consistent and that the model is able to predict the prognosis accurately. The analysis of the full set was used to visualize the performance of the model in the TCGA database (Figure 3E,3H,3K,3N).
Independent prognostic analysis and stratified prognostic analysis
Univariate Cox regression revealed a hazard ratio (HR) in the risk score of 2.014 and a 95% confidence interval (CI) of 1.534–2.644 (P<0.001) (Figure 4A), while multivariate Cox regression results showed a HR in the risk score of 1.976 and a CI of 1.516–2.574 (P<0.001) (Figure 4B). Further, two additional independent prognostic variables were found, age (1.051 and 1.021–1.082; P<0.001) and stage (2.581 and 2.251–2.942; P=0.009) (Figure 4B). In addition, the area under the curve (AUC) values obtained from the ROC curve analysis were: 0.729 for 1-year survival, 0.748 for 3-year survival, and 0.742 for 5-year survival (Figure S2, Figure S2A). We performed the K-M analysis by log-rank test (Figure 4C-4F) on EC patients after stratifying them into subgroups based on their stage and age to determine the predictive power of multiple clinical characteristics. The risk model was found to be effective in distinguishing between groups at high and low risk, as the OS rate of the high-risk groups was lower than the low-risk groups in the subgroups “patients aged 30–60”, “patients aged 61–90”, “patients with stage I–II”, and “patients with stage III–IV”. The accuracy of the risk model was also determined by the AUC values of the ROC curve (Figure 4G). As the AUC value of the risk score (0.729) was significantly higher than that of other clinical parameters, it further demonstrated that the risk model is an extremely accurate predictor of patient outcome.
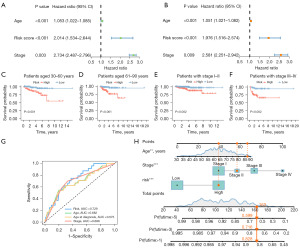
Establishment of a prognostic nomogram
An age, stage, and risk score integrated nomogram was developed to further enhance prognostic accuracy (Figure 4H). To assess the predictive accuracy and clinical applicability of the nomogram, we constructed calibration curves, of which the y- and x-axes represent the actual and the predicted survival rates. There was a satisfactory consistency between the actual and the predicted survival probabilities in the calibration plot for OS at 1, 3, and 5 years (Figure S2B).
KEGG enrichment analysis
According to KEGG analysis (Figure 5A), ICD-related signaling pathways were implicated in the risk model. The data from GSEA revealed that axon guidance, cell cycle, RNA degradation, spliceosome, and ubiquitin-mediated proteolysis were enriched in the high-risk group, whereas other pathways were enriched in the low-risk group, including allograft rejection, asthma, autoimmune thyroid disease, graft versus host disease and the type I diabetes mellitus (P<0.05; FDR <0.25; |NES| >1.5, NES, normalized enrichment score). Collectively, this all suggested that there might be differences in immunobiology and immune infiltration in the two subgroups. In light of this, we attempted to analyze immunity in the model.
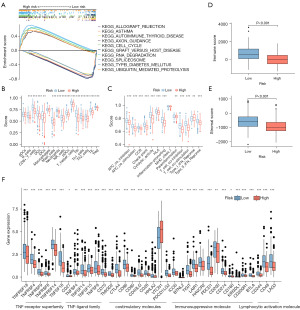
Analysis of the risk score in relation to immune factors and TME
The relative abundance of immune cells in the microenvironment was analyzed by R package “immunedeconv”, demonstrating that various immune cells negatively correlated with risk scores, which suggested that the low-risk group possessed greater immune infiltration (Figure S3). We performed ssGSEA to obtain immune cell and immune function scores in different risk categories. Compared to the high-risk group, most immune cells had higher levels of infiltration in the low-risk group. Only activated dendritic cells and macrophages did not have significantly different levels of infiltration between the risk groups (Figure 5B). In addition, 12 immune pathways showed a greater level of activity in the low-risk group, while the remaining three did not differ significantly in the two groups (Figure 5C). The immune and stromal scores of patients in the low-risk group were higher (Figure 5D,5E). A further analysis was conducted to determine whether immune checkpoint expression differed between the high- and low-risk groups, where most immune checkpoints were more positive in the low-risk group (Figure 5F). Accordingly, immunotherapy may be more beneficial to patients in the low-risk group. TIDE analysis revealed differences in immune evasion and response to immunotherapy between the high- and low-risk groups. The low-risk group was more likely to respond to immunotherapy and less likely to escape immune response (Figure S2F,S2G).
Categorization of EC subtype by consensus clustering
To investigate the association between the expression levels of the above six ICD-related lncRNAs and EC subtypes, we performed consensus clustering analysis on all 552 EC samples in the TCGA cohort. By increasing the clustering variable (k) from 2 to 9, we found that when k =4, the intra-cluster similarity and inter-cluster dissimilarity of the groupings were at their highest (Figure 6A,6B, Figure S2D,S2E). Survival analysis showed that there were significant differences in survival among the four subtypes, and clusters 1 and 2 had higher survival probabilities over the five-year period than the other clusters (Figure S2H). A heat map of immune cell infiltration was constructed (Figure S4), which indicated that cluster 4 exhibited strong immune cell infiltration. The expression differences of the four clusters at each immune checkpoint were discussed. The results showed that the expression levels of the immune checkpoints in cluster 4 were significantly higher than those in other clusters (Figure 6C). Subsequent studies could target the aforementioned immune checkpoints to treat patients in the corresponding clusters. Cluster 4 had higher ESTIMATE scores, stromal scores, and immune scores in the immune microenvironment, indicating that patients in cluster 4 had certain immunotherapy prospects (Figure 6D-6F).
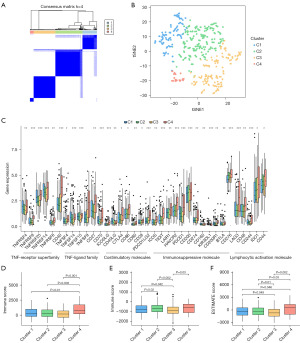
Prediction of drug sensitivity
An analysis of the IC50 values of several chemotherapeutic agents was conducted to assess the chemotherapeutic response of EC patients. Compared to patients with low risk, patients with high risk of developing cancer had significantly lower IC50 values for Shikonin, Midostaunin, MAPK inhibitors, etc. A total of 18 chemotherapeutic or targeted agents used for EC treatment were found to show lower IC50s in the high-risk group, while 18 drugs in the low-risk group showed lower IC50 (Figures S5,S6). The findings indicated that the model may predict patients’ tumor chemosensitivity.
Analysis of lncRNAs expression in clinical samples and EC cell lines
Among the above six ICD-related lncRNAs, we observed a differential expression in normal tissues and tumor tissues (Figure 7A-7F). FKBP14-AS1, FBXO30-DT, and AL161431.1 was upregulated while SCARNA9, FAM198B-AS1, and LINC01943 were downregulated in tumor tissues, further supporting our previous conclusions. Then, we examined the expression of these lncRNAs in ECCs and 4 EC cell lines (Ishikawa, KLE, RL95-2, and HEC-1B), which provided consistent results (Figure 7G-7L).
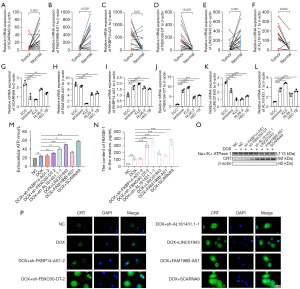
LncRNAs involvement in the regulation of ICD in vitro and in vivo
To confirm the function of the six prognostic ICD-related lncRNAs in the risk model, we examined ICD biomarkers including ATP secretion, Ecto-CRT and HMGB1 release (75,76). Short hairpin RNAs (shRNAs) and overexpression plasmid were used to manipulate the levels of the six lncRNAs in KLE cells (Figure S7A-S7F). Based on risk models, FKBP14-AS1, FBXO30-DT and AL161431.1 were risk factors for endometrial cancer and were increased in clinical samples and EC cell lines. To verify whether FKBP14-AS1, FBXO30-DT and AL161431.1 are ICD suppressors, we knocked them down independently in KLE cells. In contrast, LINC01943, FAM198B-AS1 and SCARNA9 were predicted to be protective factors for endometrial cancer and were decreased in clinical samples and EC cell lines. To verify whether they are ICD promoters, we overexpressed them independently in KLE cells. The efficiency of knocked down and overexpression is shown in Figure S7A,S7B. DOX was applicated as an inducer of ICD (77). The concentration of DOX was selected based on IC50 (Figure S7G) and was considered to be within its pharmacological range. We induced ICD by DOX chemotherapy after knockdown of the corresponding genes using sh-FKBP14-AS1, sh-FBXO30-DT, sh-AL161431.1, and overexpression of the corresponding genes using LINC01943, FAM198B-AS1, SCARNA9 plasmids in KLE cells, respectively. The supernatants of the cell cultures were collected for the measurement of ATP and HMGB-1. To measure the level of CRT exposure on cell surfaces, membrane proteins were extracted from isolated cell membranes for Western blot assay. In our study, cells transfected with sh-FKBP14-AS1, sh-FBXO30-DT, sh-AL161431.1, LINC01943 plasmid, FAM198B-AS1 plasmid, SCARNA9 plasmid demonstrated an increased release of ATP (Figure 7M), increased release of HMGB1 (Figure 7N), and increased exposure of CRT on the cell membrane (Figure 7O,7P) when compared to negative control (NC) and chemotherapy only groups, which indicated that cancer cells were induced to undergo ICD. The colony formation assay also revealed that the numbers of colonies were significantly reduced in cells transfected with sh-FKBP14-AS1, sh-FBXO30-DT, sh-AL161431.1, LINC01943 plasmid, FAM198B-AS1 plasmid, SCARNA9 plasmid compared with control cells (Figure 8A). An in vivo xenograft tumor model in female BALB/c nude mice was established to further investigate the role of lncRNAs mentioned above. As shown by tumor volume, weight, and ki67 expression levels, knocking down FKBP14-AS1, FBXO30-DT, AL161431.1, or overexpressing LINC01943, FAM198B-AS1, SCARNA9 significantly enhanced the inhibitory effect of DOX on tumor growth in vivo (Figure 8B-8E, Figure S7H-S7K). Collectively, these data pointed to an essential role for FKBP14-AS1, FBXO30-DT, AL161431.1, LINC01943, FAM198B-AS1 and SCARNA9 in ICD.
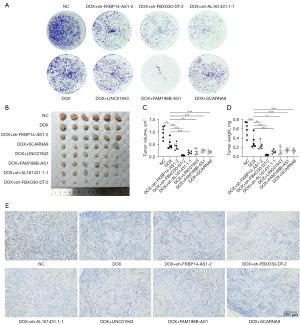
Discussion
As one of the most common gynecological malignancies, EC has increased in incidence among young women in recent years (78). The prognosis remains poor for patients with advanced stages of EC, recurrence of the disease, or distant metastases despite surgical treatment being effective for many patients with early-stage EC (3).
Encouragingly, immunotherapy is emerging as a next-generation anti-tumor regimen. A recent clinical trial proved that the programmed death-1 (PD-1) monoclonal antibody dostarlimab was associated with clinically meaningful and durable antitumor activity with an acceptable safety profile for patients (79). While the activation of ICD can directly induce tumor cell death, it is also capable of reshaping immunosuppressive TME to an immunoactivated one, initiating antitumor immunity and improving the response to immunotherapy (80). In the progression of EC, however, the role of ICD is relatively unknown.
Based on genomic characterization, the European Society of Gynaecological Oncology (ESGO) categorizes EC patients into four categories, but this classification does not adequately explain patients’ varying responses to systemic therapy (81). A novel molecular signature is required to grade EC patients for better descriptions of TME and a more reliable prognosis. LncRNAs are involved in tumorigenesis, tumor cell proliferation, invasion, migration, apoptosis, and angiogenesis (82,83). Furthermore, extracellular lncRNAs can circulate in body fluids; they are detectable and highly resistant to ribonucleases (84,85). Growing evidence suggests that lncRNAs may make effective biomarkers for tumors (86).
This study obtained 38 ICD-related genes from previous reports. Then, 398 ICD-related lncRNAs were identified. Among them, a total of 16 prognostic ICD-related lncRNAs were determined via univariate Cox regression. A risk model was constructed via LASSO regression. K-M survival analysis, univariate Cox regression, multivariate Cox regression, and the AUCs were determined to verify the accuracy and repeatability of our model. We then established a nomogram based on age, tumor stage, and risk score to estimate individualized survival. The C-index for the risk model (0.729) in our study demonstrated the good reliability of this model. Compared with the latest studies on endometrial cancer prognosis models with C indices of 0.651, 0.617, and 0.711 (87-89), our model was more competitive.
The proposed prognostic gene expression profile contains six ICD-related lncRNAs, which are SCARNA9, FAM198B-AS1, FKBP14-AS1, and FBXO30-DT, LINC01943, AL161431.1. Among the six lncRNAs, FAM198B-AS1, FKBP14-AS1, FBXO30-DT, and LINC01943 have not been reported in tumorigenesis and development, and still, need to be further elucidated. Our previous study demonstrated that SCARNA9, an immune- and autophagy-related lncRNA, may be a positive prognostic factor in EC (90,91). In this study, we found that SCARNA9 also acts as a potential positive prognostic biomarker for EC. However, the exact mechanism of SCARNA9 in EC has not yet been revealed. Future studies can explore this in more detail. AL161431.1 functions as a molecular sponge for miR-1252-5p, resulting in the de-repression of MAPK signaling to facilitate the growth and metastasis of EC cells (92). The expression of AL161431.1 has also been shown to promote cancer growth in other forms of cancer, including lung adenocarcinoma, pancreatic cancer, non-small cell lung cancer, etc., and thus suggesting a poor prognosis in these cases (93-95).
Evidence is mounting that TME interactions, especially the immune microenvironment, influence the occurrence and progression of cancer (96-98). ICD releases DAMPs into the tumor environment as immune adjuvants and recruits various types of immune cells. This increases the level of immune cell infiltration in the TME (80). Therefore, a signature is required to predict changes in the immune microenvironment caused by ICD. This study examined immune cell infiltration, immune cell function, and immune checkpoint expression in high- and low-risk model groups. The results showed that the majority of them were upregulated in patients with low-risk scores, providing evidence that the risk model may play a role in predicting the efficacy of immune treatment in EC patients. Patients with low-risk scores may benefit from receiving immune therapy in EC. Moreover, we predicted potential therapeutic compounds for different prognostic risk groups. It was found that EC drug sensitivity was strongly correlated with ICD-related lncRNAs. This provides a novel approach to treating patients with EC on an individual basis.
Our study has inherent limitations that cannot be ignored. On one hand, our risk model was developed based on public transcriptome data from the TCGA and clinical data. In addition, the internal test set was used to assess its accuracy, and more multi-center clinical samples are needed for validation. On the other hand, our experiment preliminarily analyzed the immune environment through bioinformatics methods and validated immunogenic death using three testing standards. Unfortunately, we have not yet used flow cytometry to further confirm the activation of immune cells. The mechanism underlying the involvement of ICD-related lncRNAs in occurrence and development of EC, as well as the regulation of TME still require further detailed studies.
As far as we are aware, this study is the first one using ICD-related lncRNAs to predict prognosis in EC. It analyzes ICD-related lncRNAs to establish a prognostic risk model for EC and a novel subtype classification using consensus clustering analysis. It has been shown that this model can be used to predict the prognosis of EC patients. Furthermore, we describe the characteristics of TME cell infiltration and the sensitivity of the patient to chemotherapy drugs in different subgroups. Subsequent studies could combine these drugs with first-line chemotherapy drugs to improve precision therapy in EC. The risk model based on ICD-related lncRNAs may therefore be an effective tool for predicting chemotherapy outcomes. Our findings reveal the clinical significance of ICD which provides a new direction for the study of EC biomarker detection and individualized precision therapy.
Conclusions
In our study, a prognostic signature composed of six ICD related-lncRNAs in EC was established, and the risk model based on this signature can be used to predict the prognosis of patients with EC.
Acknowledgments
All the authors acknowledge and appreciate the data provided from TCGA and GSEA databases. And we are grateful to Shijun Chen for his assistance with part of the coding.
Funding: This work was supported by grants from the
Footnote
Reporting Checklist: The authors have completed the TRIPOD reporting checklist. Available at https://tcr.amegroups.com/article/view/10.21037/tcr-23-2243/rc
Data Sharing Statement: Available at https://tcr.amegroups.com/article/view/10.21037/tcr-23-2243/dss
Peer Review File: Available at https://tcr.amegroups.com/article/view/10.21037/tcr-23-2243/prf
Conflicts of Interest: All authors have completed the ICMJE uniform disclosure form (available at https://tcr.amegroups.com/article/view/10.21037/tcr-23-2243/coif). The authors have no conflicts of interest to declare.
Ethical Statement: The authors are accountable for all aspects of the work in ensuring that questions related to the accuracy or integrity of any part of the work are appropriately investigated and resolved. This study was carried out in accordance with Declaration of Helsinki (as revised 2013). The study involving human participants was reviewed and approved by the Ethics Committee (2021-S046) of Tongji Medical College of Huazhong University of Science and Technology. The patients/participants provided their written informed consent to participate in this study. Animal experiments were performed under a project license (No. 3357) granted by the Tongji Medical College Animal Care Committee, in compliance with guidelines of Huazhong University of Science and Technology for the care and use of animals.
Open Access Statement: This is an Open Access article distributed in accordance with the Creative Commons Attribution-NonCommercial-NoDerivs 4.0 International License (CC BY-NC-ND 4.0), which permits the non-commercial replication and distribution of the article with the strict proviso that no changes or edits are made and the original work is properly cited (including links to both the formal publication through the relevant DOI and the license). See: https://creativecommons.org/licenses/by-nc-nd/4.0/.
References
- Abu-Rustum N, Yashar C, Arend R, et al. Uterine Neoplasms, Version 1.2023, NCCN Clinical Practice Guidelines in Oncology. J Natl Compr Canc Netw 2023;21:181-209. [Crossref] [PubMed]
- Siegel RL, Miller KD, Fuchs HE, et al. Cancer Statistics, 2021. CA Cancer J Clin 2021;71:7-33. [Crossref] [PubMed]
- McAlpine JN, Temkin SM, Mackay HJ. Endometrial cancer: Not your grandmother's cancer. Cancer 2016;122:2787-98. [Crossref] [PubMed]
- Jemal A, Ward EM, Johnson CJ, et al. Annual Report to the Nation on the Status of Cancer, 1975-2014, Featuring Survival. J Natl Cancer Inst 2017;109:djx030. [Crossref] [PubMed]
- Makker V, Taylor MH, Aghajanian C, et al. Lenvatinib Plus Pembrolizumab in Patients With Advanced Endometrial Cancer. J Clin Oncol 2020;38:2981-92. [Crossref] [PubMed]
- Gilks CB, Oliva E, Soslow RA. Poor interobserver reproducibility in the diagnosis of high-grade endometrial carcinoma. Am J Surg Pathol 2013;37:874-81. [Crossref] [PubMed]
- Krysko DV, Garg AD, Kaczmarek A, et al. Immunogenic cell death and DAMPs in cancer therapy. Nat Rev Cancer 2012;12:860-75. [Crossref] [PubMed]
- Kroemer G, Galassi C, Zitvogel L, et al. Immunogenic cell stress and death. Nat Immunol 2022;23:487-500. [Crossref] [PubMed]
- Galluzzi L, Buqué A, Kepp O, et al. Immunogenic cell death in cancer and infectious disease. Nat Rev Immunol 2017;17:97-111. [Crossref] [PubMed]
- Del Re DP, Amgalan D, Linkermann A, et al. Fundamental Mechanisms of Regulated Cell Death and Implications for Heart Disease. Physiol Rev 2019;99:1765-817. [Crossref] [PubMed]
- Fucikova J, Kepp O, Kasikova L, et al. Detection of immunogenic cell death and its relevance for cancer therapy. Cell Death Dis 2020;11:1013. [Crossref] [PubMed]
- Casas-Arozamena C, Abal M. Endometrial Tumour Microenvironment. Adv Exp Med Biol 2020;1296:215-25. [Crossref] [PubMed]
- Hu M, Zhang J, Kong L, et al. Immunogenic Hybrid Nanovesicles of Liposomes and Tumor-Derived Nanovesicles for Cancer Immunochemotherapy. ACS Nano 2021;15:3123-38. [Crossref] [PubMed]
- Minnie SA, Hill GR. Immunotherapy of multiple myeloma. J Clin Invest 2020;130:1565-75. [Crossref] [PubMed]
- Duan X, Chan C, Lin W. Nanoparticle-Mediated Immunogenic Cell Death Enables and Potentiates Cancer Immunotherapy. Angew Chem Int Ed Engl 2019;58:670-80. [Crossref] [PubMed]
- Zhang C, Shao S, Zhang Y, et al. LncRNA PCAT1 promotes metastasis of endometrial carcinoma through epigenetical downregulation of E-cadherin associated with methyltransferase EZH2. Life Sci 2020;243:117295. [Crossref] [PubMed]
- Kong D, Hou Y, Li W, et al. LncRNA-ZXF1 stabilizes P21 expression in endometrioid endometrial carcinoma by inhibiting ubiquitination-mediated degradation and regulating the miR-378a-3p/PCDHA3 axis. Mol Oncol 2022;16:813-29. [Crossref] [PubMed]
- Zhou Q, Kong D, Li W, et al. LncRNA HOXB-AS3 binding to PTBP1 protein regulates lipid metabolism by targeting SREBP1 in endometrioid carcinoma. Life Sci 2023;320:121512. [Crossref] [PubMed]
- Hu Q, Ye Y, Chan LC, et al. Oncogenic lncRNA downregulates cancer cell antigen presentation and intrinsic tumor suppression. Nat Immunol 2019;20:835-51. [Crossref] [PubMed]
- Wu T, Du Y. LncRNAs: From Basic Research to Medical Application. Int J Biol Sci 2017;13:295-307. [Crossref] [PubMed]
- Tibshirani R. Regression Shrinkage and Selection via The Lasso: A Retrospective. Journal of the Royal Statistical Society Series B: Statistical Methodology 2011;73:273-82. [Crossref]
- Li Y, Zeng X. A novel cuproptosis-related prognostic gene signature and validation of differential expression in hepatocellular carcinoma. Front Pharmacol 2023;13:1081952. [Crossref] [PubMed]
- Huang G, Xu X, Ju C, et al. Identification and validation of autophagy-related gene expression for predicting prognosis in patients with idiopathic pulmonary fibrosis. Front Immunol 2022;13:997138. [Crossref] [PubMed]
- Apetoh L, Ghiringhelli F, Tesniere A, et al. Toll-like receptor 4-dependent contribution of the immune system to anticancer chemotherapy and radiotherapy. Nat Med 2007;13:1050-9. [Crossref] [PubMed]
- Obeid M, Tesniere A, Ghiringhelli F, et al. Calreticulin exposure dictates the immunogenicity of cancer cell death. Nat Med 2007;13:54-61. [Crossref] [PubMed]
- Loi S, Pommey S, Haibe-Kains B, et al. CD73 promotes anthracycline resistance and poor prognosis in triple negative breast cancer. Proc Natl Acad Sci U S A 2013;110:11091-6. [Crossref] [PubMed]
- Baracco EE, Stoll G, Van Endert P, et al. Contribution of annexin A1 to anticancer immunosurveillance. Oncoimmunology 2019;8:e1647760. [Crossref] [PubMed]
- Sistigu A, Yamazaki T, Vacchelli E, et al. Cancer cell-autonomous contribution of type I interferon signaling to the efficacy of chemotherapy. Nat Med 2014;20:1301-9. [Crossref] [PubMed]
- Schiavoni G, Sistigu A, Valentini M, et al. Cyclophosphamide synergizes with type I interferons through systemic dendritic cell reactivation and induction of immunogenic tumor apoptosis. Cancer Res 2011;71:768-78. [Crossref] [PubMed]
- Michaud M, Sukkurwala AQ, Martins I, et al. Subversion of the chemotherapy-induced anticancer immune response by the ecto-ATPase CD39. Oncoimmunology 2012;1:393-5. [Crossref] [PubMed]
- Panaretakis T, Kepp O, Brockmeier U, et al. Mechanisms of pre-apoptotic calreticulin exposure in immunogenic cell death. EMBO J 2009;28:578-90. [Crossref] [PubMed]
- Gulla A, Morelli E, Samur MK, et al. Bortezomib induces anti-multiple myeloma immune response mediated by cGAS/STING pathway activation. Blood Cancer Discov 2021;2:468-83. [Crossref] [PubMed]
- Proctor M, Gonzalez Cruz JL, Daignault-Mill SM, et al. Targeting Replication Stress Using CHK1 Inhibitor Promotes Innate and NKT Cell Immune Responses and Tumour Regression. Cancers (Basel) 2021;13:3733. [Crossref] [PubMed]
- Go EJ, Yang H, Chon HJ, et al. Combination of Irreversible Electroporation and STING Agonist for Effective Cancer Immunotherapy. Cancers (Basel) 2020;12:3123. [Crossref] [PubMed]
- Wang-Bishop L, Wehbe M, Shae D, et al. Potent STING activation stimulates immunogenic cell death to enhance antitumor immunity in neuroblastoma. J Immunother Cancer 2020;8:e000282. [Crossref] [PubMed]
- Garg AD, Krysko DV, Verfaillie T, et al. A novel pathway combining calreticulin exposure and ATP secretion in immunogenic cancer cell death. EMBO J 2012;31:1062-79. [Crossref] [PubMed]
- Teo ZL, Versaci S, Dushyanthen S, et al. Combined CDK4/6 and PI3Kα Inhibition Is Synergistic and Immunogenic in Triple-Negative Breast Cancer. Cancer Res 2017;77:6340-52. [Crossref] [PubMed]
- Gay NJ, Symmons MF, Gangloff M, et al. Assembly and localization of Toll-like receptor signalling complexes. Nat Rev Immunol 2014;14:546-58. [Crossref] [PubMed]
- Riley JS, Quarato G, Cloix C, et al. Mitochondrial inner membrane permeabilisation enables mtDNA release during apoptosis. EMBO J 2018;37:e99238. [Crossref] [PubMed]
- Uscanga-Palomeque AC, Calvillo-Rodríguez KM, Gómez-Morales L, et al. CD47 agonist peptide PKHB1 induces immunogenic cell death in T-cell acute lymphoblastic leukemia cells. Cancer Sci 2019;110:256-68. [Crossref] [PubMed]
- Panaretakis T, Joza N, Modjtahedi N, et al. The co-translocation of ERp57 and calreticulin determines the immunogenicity of cell death. Cell Death Differ 2008;15:1499-509. [Crossref] [PubMed]
- Martins I, Wang Y, Michaud M, et al. Molecular mechanisms of ATP secretion during immunogenic cell death. Cell Death Differ 2014;21:79-91. [Crossref] [PubMed]
- Michaud M, Martins I, Sukkurwala AQ, et al. Autophagy-dependent anticancer immune responses induced by chemotherapeutic agents in mice. Science 2011;334:1573-7. [Crossref] [PubMed]
- Fucikova J, Kralikova P, Fialova A, et al. Human tumor cells killed by anthracyclines induce a tumor-specific immune response. Cancer Res 2011;71:4821-33. [Crossref] [PubMed]
- Manukian G, Kivolowitz C, DeAngelis T, et al. Caloric Restriction Impairs Regulatory T cells Within the Tumor Microenvironment After Radiation and Primes Effector T cells. Int J Radiat Oncol Biol Phys 2021;110:1341-9. [Crossref] [PubMed]
- Cerqueira OLD, Clavijo-Salomon MA, Cardoso EC, et al. Combined p14ARF and Interferon-β Gene Transfer to the Human Melanoma Cell Line SK-MEL-147 Promotes Oncolysis and Immune Activation. Front Immunol 2020;11:576658. [Crossref] [PubMed]
- Mónaco A, Chilibroste S, Yim L, et al. Inflammasome activation, NLRP3 engagement and macrophage recruitment to tumor microenvironment are all required for Salmonella antitumor effect. Cancer Immunol Immunother 2022;71:2141-50. [Crossref] [PubMed]
- Tian T, Lofftus S, Pan Y, et al. IL1α Antagonizes IL1β and Promotes Adaptive Immune Rejection of Malignant Tumors. Cancer Immunol Res 2020;8:660-71. [Crossref] [PubMed]
- Dudek-Perić AM, Ferreira GB, Muchowicz A, et al. Antitumor immunity triggered by melphalan is potentiated by melanoma cell surface-associated calreticulin. Cancer Res 2015;75:1603-14. [Crossref] [PubMed]
- Oresta B, Pozzi C, Braga D, et al. Mitochondrial metabolic reprogramming controls the induction of immunogenic cell death and efficacy of chemotherapy in bladder cancer. Sci Transl Med 2021;13:eaba6110. [Crossref] [PubMed]
- Tappe KA, Budida R, Stankov MV, et al. Immunogenic cell death of dendritic cells following modified vaccinia virus Ankara infection enhances CD8(+) T cell proliferation. Eur J Immunol 2018;48:2042-54. [Crossref] [PubMed]
- Tandon A, Harioudh MK, Ishrat N, et al. An MD2-derived peptide promotes LPS aggregation, facilitates its internalization in THP-1 cells, and inhibits LPS-induced pro-inflammatory responses. Cell Mol Life Sci 2018;75:2431-46. [Crossref] [PubMed]
- Chen R, Huang M, Yang X, et al. CALR-TLR4 Complex Inhibits Non-Small Cell Lung Cancer Progression by Regulating the Migration and Maturation of Dendritic Cells. Front Oncol 2021;11:743050. [Crossref] [PubMed]
- Garg AD, Vandenberk L, Fang S, et al. Pathogen response-like recruitment and activation of neutrophils by sterile immunogenic dying cells drives neutrophil-mediated residual cell killing. Cell Death Differ 2017;24:832-43. [Crossref] [PubMed]
- Reyes-Ruiz A, Calvillo-Rodriguez KM, Martínez-Torres AC, et al. The bovine dialysable leukocyte extract IMMUNEPOTENT CRP induces immunogenic cell death in breast cancer cells leading to long-term antitumour memory. Br J Cancer 2021;124:1398-410. [Crossref] [PubMed]
- Zheng J, Sun J, Chen J, et al. Oxygen and oxaliplatin-loaded nanoparticles combined with photo-sonodynamic inducing enhanced immunogenic cell death in syngeneic mouse models of ovarian cancer. J Control Release 2021;332:448-59. [Crossref] [PubMed]
- Si Y, Yue J, Liu Z, et al. Phase-Transformation Nanoparticle-Mediated Sonodynamic Therapy: An Effective Modality to Enhance Anti-Tumor Immune Response by Inducing Immunogenic Cell Death in Breast Cancer. Int J Nanomedicine 2021;16:1913-26. [Crossref] [PubMed]
- Wang Y, Wang Z, Chen B, et al. Cooperative Self-Assembled Nanoparticle Induces Sequential Immunogenic Cell Death and Toll-Like Receptor Activation for Synergistic Chemo-immunotherapy. Nano Lett 2021;21:4371-80. [Crossref] [PubMed]
- Ma Y, Aymeric L, Locher C, et al. Contribution of IL-17-producing gamma delta T cells to the efficacy of anticancer chemotherapy. J Exp Med 2011;208:491-503. [Crossref] [PubMed]
- Wang L, Guan R, Xie L, et al. An ER-Targeting Iridium(III) Complex That Induces Immunogenic Cell Death in Non-Small-Cell Lung Cancer. Angew Chem Int Ed Engl 2021;60:4657-65. [Crossref] [PubMed]
- Huang J, Chen P, Liu K, et al. CDK1/2/5 inhibition overcomes IFNG-mediated adaptive immune resistance in pancreatic cancer. Gut 2021;70:890-9. [Crossref] [PubMed]
- El Darzi E, Bazzi S, Daoud S, et al. Differential regulation of surface receptor expression, proliferation, and apoptosis in HaCaT cells stimulated with interferon-γ, interleukin-4, tumor necrosis factor-α, or muramyl dipeptide. Int J Immunopathol Pharmacol 2017;30:130-45. [Crossref] [PubMed]
- Paroli M, Bellati F, Videtta M, et al. Discovery of chemotherapy-associated ovarian cancer antigens by interrogating memory T cells. Int J Cancer 2014;134:1823-34. [Crossref] [PubMed]
- Zhao C, Li Y, Zhang W, et al. IL-17 induces NSCLC A549 cell proliferation via the upregulation of HMGA1, resulting in an increased cyclin D1 expression. Int J Oncol 2018;52:1579-92. [Crossref] [PubMed]
- Fan C, Hu H, Shen Y, et al. PRF1 is a prognostic marker and correlated with immune infiltration in head and neck squamous cell carcinoma. Transl Oncol 2021;14:101042. [Crossref] [PubMed]
- Friedman J, Hastie T, Tibshirani R. Regularization Paths for Generalized Linear Models via Coordinate Descent. J Stat Softw 2010;33:1-22. [Crossref] [PubMed]
- Hänzelmann S, Castelo R, Guinney J. GSVA: gene set variation analysis for microarray and RNA-seq data. BMC Bioinformatics 2013;14:7. [Crossref] [PubMed]
- Li T, Fu J, Zeng Z, et al. TIMER2.0 for analysis of tumor-infiltrating immune cells. Nucleic Acids Res 2020;48:W509-14. [Crossref] [PubMed]
- Chen B, Khodadoust MS, Liu CL, et al. Profiling Tumor Infiltrating Immune Cells with CIBERSORT. Methods Mol Biol 2018;1711:243-59. [Crossref] [PubMed]
- Finotello F, Mayer C, Plattner C, et al. Molecular and pharmacological modulators of the tumor immune contexture revealed by deconvolution of RNA-seq data. Genome Med 2019;11:34. [Crossref] [PubMed]
- Becht E, Giraldo NA, Lacroix L, et al. Estimating the population abundance of tissue-infiltrating immune and stromal cell populations using gene expression. Genome Biol 2016;17:218. [Crossref] [PubMed]
- Barbie DA, Tamayo P, Boehm JS, et al. Systematic RNA interference reveals that oncogenic KRAS-driven cancers require TBK1. Nature 2009;462:108-12. [Crossref] [PubMed]
- Wilkerson MD, Hayes DN. ConsensusClusterPlus: a class discovery tool with confidence assessments and item tracking. Bioinformatics 2010;26:1572-3. [Crossref] [PubMed]
- Kepp O, Senovilla L, Vitale I, et al. Consensus guidelines for the detection of immunogenic cell death. Oncoimmunology 2014;3:e955691. [Crossref] [PubMed]
- Kroemer G, Galluzzi L, Kepp O, et al. Immunogenic cell death in cancer therapy. Annu Rev Immunol 2013;31:51-72. [Crossref] [PubMed]
- Ghiringhelli F, Apetoh L, Tesniere A, et al. Activation of the NLRP3 inflammasome in dendritic cells induces IL-1beta-dependent adaptive immunity against tumors. Nat Med 2009;15:1170-8. [Crossref] [PubMed]
- Casares N, Pequignot MO, Tesniere A, et al. Caspase-dependent immunogenicity of doxorubicin-induced tumor cell death. J Exp Med 2005;202:1691-701. [Crossref] [PubMed]
- Sung H, Ferlay J, Siegel RL, et al. Global Cancer Statistics 2020: GLOBOCAN Estimates of Incidence and Mortality Worldwide for 36 Cancers in 185 Countries. CA Cancer J Clin 2021;71:209-49. [Crossref] [PubMed]
- Oaknin A, Tinker AV, Gilbert L, et al. Clinical Activity and Safety of the Anti-Programmed Death 1 Monoclonal Antibody Dostarlimab for Patients With Recurrent or Advanced Mismatch Repair-Deficient Endometrial Cancer: A Nonrandomized Phase 1 Clinical Trial. JAMA Oncol 2020;6:1766-72. [Crossref] [PubMed]
- Jin MZ, Wang XP. Immunogenic Cell Death-Based Cancer Vaccines. Front Immunol 2021;12:697964. [Crossref] [PubMed]
- Concin N, Matias-Guiu X, Vergote I, et al. ESGO/ESTRO/ESP guidelines for the management of patients with endometrial carcinoma. Radiother Oncol 2021;154:327-53. [Crossref] [PubMed]
- Schmidt LH, Spieker T, Koschmieder S, et al. The long noncoding MALAT-1 RNA indicates a poor prognosis in non-small cell lung cancer and induces migration and tumor growth. J Thorac Oncol 2011;6:1984-92. [Crossref] [PubMed]
- Kogo R, Shimamura T, Mimori K, et al. Long noncoding RNA HOTAIR regulates polycomb-dependent chromatin modification and is associated with poor prognosis in colorectal cancers. Cancer Res 2011;71:6320-6. [Crossref] [PubMed]
- Zhou X, Yin C, Dang Y, et al. Identification of the long non-coding RNA H19 in plasma as a novel biomarker for diagnosis of gastric cancer. Sci Rep 2015;5:11516. [Crossref] [PubMed]
- Li Q, Shao Y, Zhang X, et al. Plasma long noncoding RNA protected by exosomes as a potential stable biomarker for gastric cancer. Tumour Biol 2015;36:2007-12. [Crossref] [PubMed]
- Shi T, Gao G, Cao Y. Long Noncoding RNAs as Novel Biomarkers Have a Promising Future in Cancer Diagnostics. Dis Markers 2016;2016:9085195. [Crossref] [PubMed]
- Yu Y, Zhang Y, Li Z, et al. An EMT-related genes signature as a prognostic biomarker for patients with endometrial cancer. BMC Cancer 2023;23:879. [Crossref] [PubMed]
- Jin W, Zhuang X, Lin Y, et al. Integrating ferroptosis-related genes (FRGs) and prognostic models to enhance UCEC outcome prediction and therapeutic insights. J Appl Genet 2023;64:723-35. [Crossref] [PubMed]
- Zhai L, Yang X, Cheng Y, et al. Glutamine and amino acid metabolism as a prognostic signature and therapeutic target in endometrial cancer. Cancer Med 2023;12:16337-58. [Crossref] [PubMed]
- Wang Z, Liu Y, Zhang J, et al. An Immune-Related Long Noncoding RNA Signature as a Prognostic Biomarker for Human Endometrial Cancer. J Oncol 2021;2021:9972454. [Crossref] [PubMed]
- Wang Z, Zhang J, Liu Y, et al. An Integrated Autophagy-Related Long Noncoding RNA Signature as a Prognostic Biomarker for Human Endometrial Cancer: A Bioinformatics-Based Approach. Biomed Res Int 2020;2020:5717498. [Crossref] [PubMed]
- Gu ZR, Liu W. The LncRNA AL161431.1 targets miR-1252-5p and facilitates cellular proliferation and migration via MAPK signaling in endometrial carcinoma. Eur Rev Med Pharmacol Sci 2020;24:2294-302. [PubMed]
- Shao J, Zhang B, Kuai L, et al. Integrated analysis of hypoxia-associated lncRNA signature to predict prognosis and immune microenvironment of lung adenocarcinoma patients. Bioengineered 2021;12:6186-200. [Crossref] [PubMed]
- Ke M. Identification and Validation of Apparent Imbalanced Epi-lncRNAs Prognostic Model Based on Multi-Omics Data in Pancreatic Cancer. Front Mol Biosci 2022;9:860323. [Crossref] [PubMed]
- Jiang H, Xu A, Li M, et al. Seven autophagy-related lncRNAs are associated with the tumor immune microenvironment in predicting survival risk of nonsmall cell lung cancer. Brief Funct Genomics 2022;21:177-87. [Crossref] [PubMed]
- Schreiber RD, Old LJ, Smyth MJ. Cancer immunoediting: integrating immunity's roles in cancer suppression and promotion. Science 2011;331:1565-70. [Crossref] [PubMed]
- Salmon H, Remark R, Gnjatic S, et al. Host tissue determinants of tumour immunity. Nat Rev Cancer 2019;19:215-27. [PubMed]
- Galon J, Angell HK, Bedognetti D, et al. The continuum of cancer immunosurveillance: prognostic, predictive, and mechanistic signatures. Immunity 2013;39:11-26. [Crossref] [PubMed]