Bioinformatics prediction and experimental verification identify a cuproptosis-related gene signature as prognosis biomarkers of hepatocellular carcinoma
Highlight box
Key findings
• The present study explored the relationship between cuproptosis-related genes and hepatocellular carcinoma.
What is known and what is new?
• Cuproptosis-related genes are related to the occurrence and development of cancer.
• Through the analysis of the dataset, we explored the relationship between the key genes of copper death and the occurrence of liver cancer, and constructed a prediction model of liver cancer, which provided a new perspective for the diagnosis and evaluation of liver cancer.
What is the implication, and what should change now?
• In terms of the fundamental aspects, further investigation is required to elucidate the underlying mechanisms of cuproptosis-related gene functions. In the clinical domain, exploring whether cuproptosis-related genes can serve as therapeutic targets would provide novel insights for treatment strategies.
Introduction
Hepatocellular carcinoma (HCC) is the fourth leading cause of death related to cancer, and it is the sixth most common malignancy (1). Besides, the most common type of primary liver cancer is HCC which is associated with several etiologies, such as alcohol abuse, chronic hepatitis B virus (HBV) or hepatitis C virus (HCV) infection and so forth (2). World Health Organization (WHO) estimates that, based on annual projections, liver cancer will be the cause of death for more than one million patients by 2030 (3). The death rate of HCC increased by 43% in the US during 2000 to 2016 (4). In addition, HCC, which 5-year survival rate is 18%, is the second most deadly tumor only after pancreatic cancer (5). Complex etiological factors make prognostic prediction challenging.
In addition, on account of the limitation of therapeutic strategies for HCC, new prognostic indictors are in urgent need.
In the past decade, it has been an emerging theme of investigation on killing cells by different metals out of the apoptotic pathways. For example, excessive zinc can induce non-apoptotic cell death by inhibiting adenosine triphosphate (ATP) synthesis (6). The major feature of iron death is that iron catalyzes the generation of toxic membrane lipid peroxides, which is a unique non-apoptotic form of cell death (7). Recently, cuproptosis, a type of cell death mediated by protein lipoylation, has been reported (8). This mode of cell death does not rely on apoptosis (9,10), caspase cell death (11,12), reactive oxygen species (ROS)-induced cell death (13-15), nor inhibition of the ubiquitin-proteasome system (16,17). A phenomenon that toxicity of copper involves the destruction of specific mitochondrial metabolic enzymes has been revealed by Tsvetkov et al. Hence, copper toxicity could be a new approach to treat cancer (8).
To test our hypothesis, we downloaded the expression profiles of mRNA and corresponding clinical data of patients with HCC from public databases. After that, we used functional richness analysis to figure out the underlying mechanisms. Cox regression analysis was performed for the prognostic model construction with differentially expressed genes which were associated with cuproptosis in The Cancer Genome Atlas (TCGA) cohort. We present this article in accordance with the TRIPOD reporting checklist (available at https://tcr.amegroups.com/article/view/10.21037/tcr-23-1561/rc).
Methods
Acquisition of a dataset of cuproptosis-related genes
We obtained the set of genes associated with cuproptosis from the essay of Tsvetkov et al. (8). The genes in the gene set involved: ATP7A, DBT, ATP7B, DLST, DLAT, FDX1, DLD, GCSH, LIPT1, PDHA1, PDHB, SLC31A1, LIAS.
Acquisition and processing of HCC data
RNAseq data of HCC project were downloaded from TCGA database. Fragments per kilobase of transcript per million mapped reads (FPKM) data was converted to transcripts per million (TPM) data and log2 conversion was performed.
Expression of cuproptosis-related genes
The expression of genes which is related to cuproptosis was analyzed in normal and cancer tissues of liver cancer project. The ggplot2 was used for data visualization. UALCAN is a user-friendly, interactive and comprehensive resource for researchers to analyze cancer omics data. It provides easy access to publicly available cancer-omics data (TCGA, MET500 CPTAC and CBTTC) and provides clinical proteomic data analysis (18). Proteomic analysis of genes related to cuproptosis was performed with CPTAC database (19).
Human protein mapping (HPA) is a proteomics database, with information on about 26,000 kinds of tissues and cells of human protein distribution. In this study, database from HPA was utilized to display the protein expression of related genes (20).
The database from cBioPortal was utilized to analyze the mutations of TCGA genes and their relationship with mRNA (21,22).
Screening of cuproptosis-related genes and their effect on prognosis
Univariate Cox regression analysis was performed for screening the genes correlated with cuproptosis. The survival package was utilized for performing the survival statistical data analysis. To visualize analysis results, we utilized the survminer package. Overall survival (OS) was the focus of our study of HCC prognosis here.
Cell lines and cell culture
THLE-2, HuH-7, HepG2 were from General Surgery Laboratory of Tianjin Medical University General Hospital, China. All cells were appropriately cultured in DMEM medium and kept in an incubator at 37 ℃ and 5% CO2.
Quantitative real-time polymerase chain reaction (qRT-PCR)
TRIzol reagent (Solarbio, Beijing, China) was a tool to purify Total RNA from adipose cells or mouse adipose tissue. Meanwhile, CDNA was secured by RNA reverse transcription.
Besides, we used qRT-PCR system (Bio-RAD, California, USA) to analyze expression levels of specific genes. Primers for the four genes were displayed as follows.
LIPT1: F 5'-GTTGCTCGGGGTCGTATG-3';
R 5'-TTCTTCTCCGAGCCAGTTTT-3'.
DLAT: F 5'-CCGCCGCTATTACAGTCTTCC-3';
R 5'-CTCTGCAATTAGGTCACCTTCAT-3'.
PDHA1: F 5'-GAGCTGAGCAGCTGTGTAAC-3';
R 5'-TGCCAATCGTTACAGGTATTACAG-3'.
ATP7A: F 5'-TGACCCTAAACTACAGACTCCAA-3';
R 5'-CGCCGTAACAGTCAGAAACAA-3'.
Cuproptosis-related genes and HCC related factors analysis
The correlation between clinical factors and risk score was analyzed. The statistical method used was Kruskal-Wallis test. Ggplot2 package was utilized for data visualization. Besides, we analysed the correlation between risk score and immune infiltrating cells. The algorithm parameter was single sample gene set enrichment analysis (ssGSEA). Spearman was used as the statistical method. Gene set variation analysis (GSVA) pack was used for the analysis of immunoinfiltrated cells (23). The twenty-four kinds of immune cells were: B cells; cytotoxic cells; CD8 T cells; eosinophils; activated DC (aDC); immature DC (iDC); plasmacytoid DC (pDC); DC; macrophages; natural killer (NK) cells; neutrophils; T cells; NK CD56bright, dim cells; mast cells; T effector memory (Tem); T central memory (Tcm); T gamma delta (Tgd); Treg; T helper cells; T follicular helper (Tfh); Th1, 2, 17cells (24).
Functional enrichment of genes related to cuproptosis
Gene Ontology (GO) and Kyoto Encyclopedia of Genes and Genomes (KEGG) analysis were utilized to screen the cuproptosis-related genes differentially expressed in HCC (25). Ggplot2 package was used to visualize results while clusterProfiler package was utilized to analyze selected data.
Construction and evaluation of nomogram
Univariate Cox regression analysis was performed by using the risk score as an indicator along with clinical factors. With P<0.1 as the standard, significant factors were selected to construct the nomogram (26). Subsequent analysis was performed by rms package and survival package. Calibration diagram and decision curve analysis (DCA) diagram were used to detect the nomogram.
Ethical statement
The study was conducted in accordance with the Declaration of Helsinki (as revised in 2013).
Results
Expression of genes related to cuproptosis in HCC patients from TCGA database
First of all, we performed the analysis of the cuproptosis-related genes expression in normal tissues and cancer tissues. It could be seen that LIAS, PDHA1, DLST, PDHB, DLAT, ATP7A, LIPT1 and DLD were highly expressed in cancer tissues (Figure 1A). Subsequently, we detected the expression of these 13 cuproptosis-related genes in unmatched samples. The expression of FDX1, DBT, GCSH, SLC31A1 were relatively high in normal tissues and the expression of PDHA1, PDHB, LIPT1, LIAS, ATP7A, ATP7B, DLD, DLST, DLAT were relatively high in cancer tissues (Figure 1B). Except for FDX1 and ATP7B, the expression of other genes in TCGA cohort was meaningful (P<0.05). Then, the protein expression of genes aforementioned were analyzed (Figure 1C-1O). The mRNA expression of some genes and their protein expression were different, which might be caused by the inconsistent sample size.
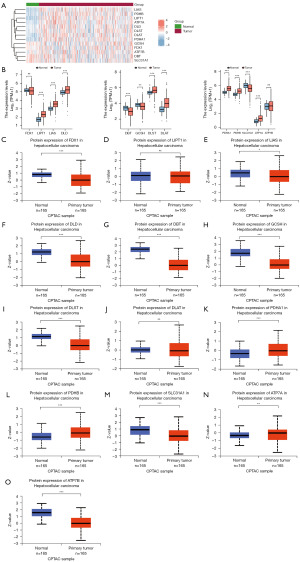
Screening of cuproptosis-related genes associated with prognosis in a TCGA cohort
Univariate Cox regression analysis was performed on 13 genes correlated with cuproptosis (Figure 2A). We identified four meaningful genes. They were LIPT1 [hazard ratio (HR) =1.853; P<0.001], DLAT (HR =1.497; P<0.001), PDHA1 (HR =1.350; P<0.05) and ATP7A (HR =1.446; P<0.05). These four genes were then chosen to calculate risk score. The final risk scores are as follows: e (0.457 × expression level of LIPT1 + 0.323 × expression level of DLAT + 0.024 × expression level of PDHA1 + 0.012 × expression level of ATP7A). Patients were divided into high-risk group and low-risk group by using the median cutoff value of risk scores. We used risk score as an individual indicator to perform univariate Cox regression analysis together with clinical information (Figure 2B). Meanwhile, patients were divided into two groups of low-risk group and high-risk group based on risk score, and the baseline data table was constructed based on it (Table 1). Taking all together, risk factors which were statistically significant (P<0.05) are as follows: T stage (T3&T4 vs. T1) (HR =2.949), M stage (M1 vs. M0) (HR =4.077), Pathologic stage (III&IV vs. I) (HR =2.823), tumor status (with tumor vs. tumor free) (HR =2.317), risk groups (high vs. low) (HR =1.472). After that, we analyzed the OS of these four genes. We found that the OS of the four genes in the low expression group was higher than that in the high expression group, and the results of analysis were statistically significant (Figure 2C-2F). High-level and low-level groups were separated in accordance with the risk score. They were analyzed for OS and disease-specific survival (DSS). We found that the group with low-risk score gained an advantage over the group with high-risk scores in both OS and DSS and had statistical significance (P<0.001) (Figure 2G,2H).
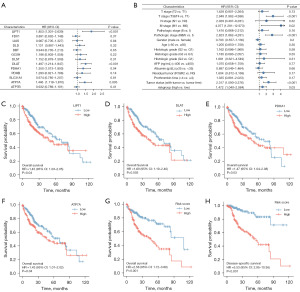
Table 1
Characteristic | Low-risk group | High-risk group | P |
---|---|---|---|
T stage, n (%) | 0.02 | ||
T1 | 105 (28.4) | 78 (21.1) | |
T2 | 41 (11.1) | 53 (14.3) | |
T3 | 31 (8.4) | 49 (13.2) | |
T4 | 7 (1.9) | 6 (1.6) | |
N stage, n (%) | 0.62 | ||
N0 | 126 (48.8) | 128 (49.6) | |
N1 | 1 (0.4) | 3 (1.2) | |
M stage, n (%) | >0.99 | ||
M0 | 133 (48.9) | 135 (49.6) | |
M1 | 2 (0.7) | 2 (0.7) | |
Pathologic stage, n (%) | 0.01 | ||
Stage I | 99 (28.4) | 74 (21.2) | |
Stage II | 40 (11.5) | 46 (13.2) | |
Stage III | 31 (8.9) | 54 (15.5) | |
Stage IV | 3 (0.9) | 2 (0.6) | |
Tumor status, n (%) | 0.08 | ||
Tumor free | 109 (30.8) | 93 (26.3) | |
With tumor | 67 (18.9) | 85 (24.0) | |
Histologic grade, n (%) | 0.02 | ||
G1 | 35 (9.5) | 20 (5.4) | |
G2 | 94 (25.5) | 84 (22.8) | |
G3 | 51 (13.9) | 72 (19.6) | |
G4 | 4 (1.1) | 8 (2.2) | |
AFP (ng/mL), n (%) | 0.47 | ||
≤400 | 114 (40.9) | 101 (36.2) | |
>400 | 30 (10.8) | 34 (12.2) | |
Albumin (g/dL), n (%) | 0.84 | ||
<3.5 | 35 (11.7) | 34 (11.4) | |
≥3.5 | 122 (40.8) | 108 (36.1) | |
Prothrombin time, n (%) | 0.78 | ||
≤4 | 110 (37.2) | 97 (32.8) | |
>4 | 45 (15.2) | 44 (14.9) | |
OS event, n (%) | 0.35 | ||
Alive | 126 (33.8) | 117 (31.4) | |
Dead | 60 (16.1) | 70 (18.8) | |
Residual tumor, n (%) | 0.18 | ||
R0 | 168 (48.8) | 158 (45.9) | |
R1 | 6 (1.7) | 11 (3.2) | |
R2 | 0 | 1 (0.3) |
T, tumor; N, node; M, metastasis; AFP, alpha-fetoprotein; OS, overall survival.
Expression and mutation of genes related to cuproptosis
Four genes related to cuproptosis were validated in liver cell line and liver cancer cell lines, and the expression of these genes was significantly increased and statistically significant in liver cancer cell lines (Figure 3A-3D) (P<0.05). Using the cBioportal database to analyze the mutations of these four genes, we found that the probability of mutation of these four genes was lower in patients with liver cancer (Figure 3E). After that, further analysis of the mutation and the expression of mRNA showed that diploid and gain accounted for a large proportion in the type of mutation (Figure 3F-3I). The expression level of LIPT1 was slightly higher in cancer tissues, according to the Analytical results of the HPA database, than that in normal tissues, while the expression level of DLAT, PDHA1and ATP7A in cancer tissues were significantly higher than the normal range (Figure 4A-4D).
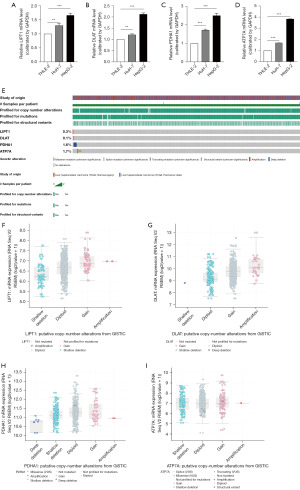
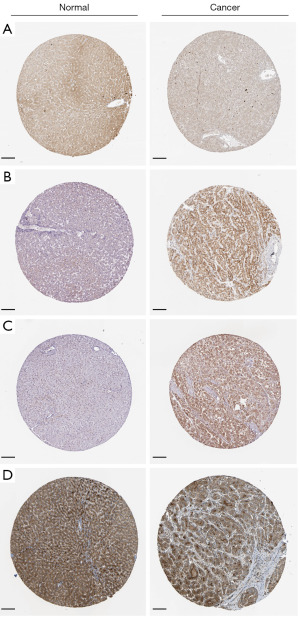
The correlation between the risk groups and clinical factors in TCGA cohort
In accordance with the risk score, we divided groups into high-risk one and low-risk one, and then the risk factor graph was constructed. The high-risk group had less survival time than the low-risk group. The expression of four cuproptosis-related genes was higher in high-risk group (Figure 5A). The time receiver operating characteristic (ROC) curve was analyzed with the risk score as the index (Figure 5B). The risk score was more precise in predicting the survival rate in the first year, and the area under curve (AUC) was 0.726. Next, the relationship analysis between the risk score and clinical factors was performed. In T stage, T3&T4 vs. T1 was statistically significant (P<0.01) (Figure 5C). N1 vs. N0 was statistically significant (P<0.05) (Figure 5D). So as in histologic grade (G3&G4 vs. G1), pathologic stage (Stage III&Stage IV vs. Stage I) and OS event (dead vs. alive) (P<0.05) (Figure 5E-5G). M stage (M1 vs. M0) was not statistically significant (Figure 5H).
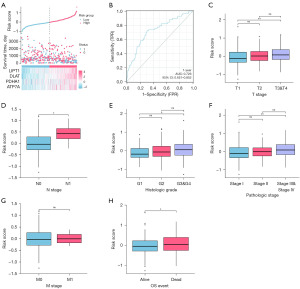
Analyzing functional enrichment of differentially expressed copper death-related genes
According to the analysis in Figure 1B, 11 genes related to cuproptosis were selected for functional enrichment analysis. In biological process (BP), we found that genes were mainly enriched in processes related to metabolism, including tricarboxylic acid cycle, citrate metabolic process, tricarboxylic acid metabolic process, acetyl-coenzyme A (CoA) biosynthetic process from pyruvate and so on (Figure 6A). In cellular component (CC), genes were mainly enriched in tricarboxylic acid cycle enzyme complex, mitochondrial matrix, oxidoreductase complex and dihydrolipoyl dehydrogenase complex (Figure 6B). Next, in molecular function (MF), genes were mainly related to transferase activity, oxidoreductase activity, acting on the aldehyde or oxo group of donors, transferring acyl groups, acting on the aldehyde or oxo group of donors, NAD or NADP as acceptor, oxidoreductase activity, transferring acyl groups and transferase activity (Figure 6C). In KEGG, citrate cycle [tricarboxylic acid (TCA) cycle], pyruvate metabolism, carbon metabolism and glycolysis/gluconeogenesis were enriched (Figure 6D).
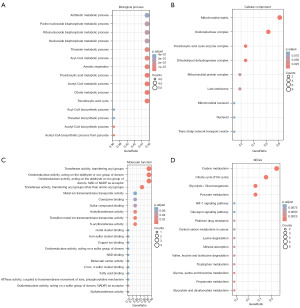
Correlation between the risk score and immune cell infiltration in HCC
We analyzed the relationship between risk score and immune cells. T helper cells, Tcm, Th2 cells, eosinophils, macrophages, aDC, Tfh were positively associated with the risk score and was statistically significant (P<0.05). Treg, DC, pDC, cytotoxic cells were negatively associated with the risk score and had statistical significance (P<0.05) (Figure 7A). Then we further analyzed and proved the relationship between DC, Macrophages, T cells and the risk score (Figure 7B-7D). Risk scores were separated in two groups, high risk and low risk, based on the level of risk. We found a phenomenon that T cells expression was lower in the high-risk group (Figure 7E). Cytotoxic cells and Treg were relatively lower in the high-risk group, whereas T helper cells were higher among high-risk group, and the results were statistically significant (P<0.05) (Figure 7F-7H). The expression of B cell between two groups had no significant difference (Figure 7I). DC and pDC were relatively low in high-risk group, whereas aDC was relatively low in low-risk group (Figure 7J-7L).
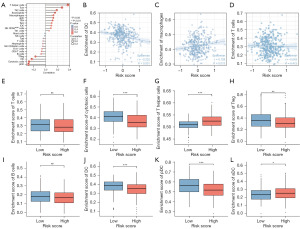
Construction and verification of nomogram diagram
For purpose of providing a quantitative method to predict the prognosis of patients with HCC, we built a nomogram diagram using risk groups and independent clinical risk factors (Figure 8A). These variables were scored using a point scale in the nomogram diagram based on cox analysis. After that, we analyzed the accuracy and applicability of the model we established. The deviation correction line in the calibration diagram was of close proximity to the ideal curve (45 points), and the predicted values were compatible with the observed values (Figure 8B). The nomogram diagram was a good model on predicting short-term or long-term survival of liver cancer patients. In the DCA diagram, the net return of this model was higher when the threshold is about 0.2 to 0.45 (Figure 8C).
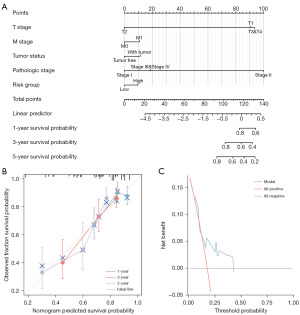
Discussion
Copper is an important trace element that plays an indispensable role in every living thing. In the 1960s, people investigated the anti-tumor activity of thiosemicarbazone complexes, which ushered in a new epoch of treating cancer with copper (27,28). In the twenty-first century, copper-based Fenton reaction has gradually become a hot topic, and inspired by iron-induced Fenton reaction, the study of oxidative stress mediated by ROS has been further enriched and the status of copper in cancer treatment has been improved (29). We should pay more attention on the key role of copper ion in tumor cells. A study has shown that copper in serum and tumor tissues of cancer patients are in higher level than that of healthy people (30). Recently, researchers included Tsvetkov et al. have shown that cells with an active TCA cycle increase the level of lipoylated TCA enzymes and that the lipoylated portion acts as a direct copper binder, leading to the aggregation of lipoylated proteins, which in turn leads to cell death (8). We systematically analyzed the expression of 13 cuproptosis-related genes in HCC tissues and their relationship with prognosis in this context. For the first time, a risk score based on four copper death-related genes was calculated, and the risk score was used as an indicator to combine clinical factors to construct a nomogram. At the same time, functional enrichment analysis and immune correlation analysis were carried out with the risk score as an indicator. LIPT1, DLAT, PDHA1 and ATP7A were screened in our study by differential analysis and univariate Cox analysis, and we verified the expression of these four genes in the liver cell line and HCC cell lines, and proved that the expression of these four genes was raised and being statistically significant in cancer cell lines. This was consistent with the analysis of the TCGA dataset. After that, we used these four genes to construct risk scores and investigated the relationship between these four genes and clinical factors. One study showed that fibroblasts obtained from patients with LIPT2 mutations showed significantly reduced lipoylation of the 2-oxydehydrogenase complex (31). LIPT1 could affect mitochondrial lipoic acid metabolism and thus lipoylation (32). A study has also shown that LIPT1 is closely related to the occurrence and prognosis of liver cancer (33). The study has found that DLAT is related to mitochondrial stress management and ROS stress in B cells (34). DLAT is involved in the proliferation and carbohydrate metabolism of gastric cancer cells and might be one of the potential drug targets in mitochondria (35). MELK promotes the development of HCC by increasing the expression level of DLAT (36). One study showed that mitochondria and PDHA1 contributed to the development of prostate tumors by controlling lipid biosynthesis (37). PDHA1 is associated with poor prognosis in patients with liver cancer (38). Copper ions from intestinal cells are pumped into the blood via ATP7A and into liver cells via CTR1 (39). ATP7A is widely expressed and is mostly located on the trans-Golgi network membrane (40). The absence of Atox1/ATP7Apathway will lead to abnormal distribution of copper and pathological changes of organs (41-45). A study has shown that liver cancer patients with high expression of ATP7A have poor prognosis, and ATP7A is positively correlated with programmed death-ligand 1 (PD-L1) level (46).
Afterwards, GO and KEGG analyses were performed for the differentially expressed copper death genes. It could be seen that these genes were closely related to the tricarboxylic acid cycle, glycolysis, and mitochondrial metabolism, which is consistent with the content of the literature. It is suggested that cuproptosis may affect cell survival through the TCA cycle and glycolysis.
In terms of immune infiltration, we found a close relationship between risk fraction and T cell subsets. T cell penetration into the tumor microenvironment is the key to successful T cell immunotherapy, including immune checkpoint inhibitors (47). In general, copper deficiency reduces the effectiveness of acquired responses, and antibodies produced by splenic cells are significantly reduced in copper-deficient animals (48-50). In the case of severe copper deficiency, neutrophils in human peripheral blood are not only decreased in number, but their ability to produce superoxide anions and kill ingested microorganisms is also decreased in the case of significant and marginal copper deficiency (51). In the human Jurkat T cell line with copper deficiency, interleukin (IL)-2 synthesis was reduced by 75% after mitogen stimulation and IL-2 mRNA was reduced by 50%, suggesting that copper plays a specific role in IL-2 gene transcription (52). Lukasewycz and Prohaska observed a significant upregulate in IL-1 and a significant down regulate in IL-2 in copper deficient animals (49). A study has shown that copper-based nanoscale coordination polymers can induce the production of more ROS, thus combined with radiotherapy to improve the infiltration rate of CD8+T cells and improve complete regression rate of tumor (53).
Cuproptosis has been a hot research field in the past few years, but the potential regulatory mechanism between tumor and copper death has not been fully elucidated. Through the functional enrichment analysis of cuproptosis related genes in HCC, we explored the related BPs and pathways of copper death. We screened four genes that can construct risk scores and analyzed the relationship between risk scores and clinical factors. Finally, we constructed and verified the predictive model by constructing the nomogram map of risk scores and clinical factors.
This study has several limitations. First, our prognostic model uses data from public databases. More forward-looking real data are needed to verify its clinical use. Second, in order to explicate the specific role of copper-related genes in the occurrence and development of HCC, some clinical parameters, such as the details of the treatment patients receive, need to be considered. However, there is lack of information in public databases. Third, with the aim of ensuring the robustness of the prognostic model, the prognostic indicator of our established model is required to be further confirmed in other independent cohorts to make sure its robustness. Fourth, in the current study, there are some differences between the number of cancer patients and the number of healthy subjects as a control; as a consequence, further research is needed to maintain the balance of the sample size.
Conclusions
Our study constructed a risk score associated with four cuproptosis-related genes, and verified its correlation with clinical factors and prognosis. Finally, we constructed the nomogram map using risk scores and clinical factors. It contributes a new perspective for predicting the prognosis of liver cancer. The internal mechanism between cuproptosis-related genes and liver cancer is still little known, which is worthy of further study.
Acknowledgments
Funding: This work was supported by
Footnote
Reporting Checklist: The authors have completed the TRIPOD reporting checklist. Available at https://tcr.amegroups.com/article/view/10.21037/tcr-23-1561/rc
Data Sharing Statement: Available at https://tcr.amegroups.com/article/view/10.21037/tcr-23-1561/dss
Peer Review File: Available at https://tcr.amegroups.com/article/view/10.21037/tcr-23-1561/prf
Conflicts of Interest: All authors have completed the ICMJE uniform disclosure form (available at https://tcr.amegroups.com/article/view/10.21037/tcr-23-1561/coif). The authors have no conflicts of interest to declare.
Ethical Statement: The authors are accountable for all aspects of the work in ensuring that questions related to the accuracy or integrity of any part of the work are appropriately investigated and resolved. The study was conducted in accordance with the Declaration of Helsinki (as revised in 2013).
Open Access Statement: This is an Open Access article distributed in accordance with the Creative Commons Attribution-NonCommercial-NoDerivs 4.0 International License (CC BY-NC-ND 4.0), which permits the non-commercial replication and distribution of the article with the strict proviso that no changes or edits are made and the original work is properly cited (including links to both the formal publication through the relevant DOI and the license). See: https://creativecommons.org/licenses/by-nc-nd/4.0/.
References
- Villanueva A. Hepatocellular Carcinoma. N Engl J Med 2019;380:1450-62. [Crossref] [PubMed]
- Yang JD, Hainaut P, Gores GJ, et al. A global view of hepatocellular carcinoma: trends, risk, prevention and management. Nat Rev Gastroenterol Hepatol 2019;16:589-604. [Crossref] [PubMed]
- World Health Organization. Projections of mortality and causes of death, 2016 to 2060. Available online: https://platform.who.int/mortality
- Centers for Disease Control and Prevention, National Center for Health Statistics. Trends in liver cancer mortality among adults aged 25 and over in the United States, 2000–2016. Available online: https://www.cdc.gov/nchs/products/databriefs/db314.htm
- Jemal A, Ward EM, Johnson CJ, et al. Annual Report to the Nation on the Status of Cancer, 1975-2014, Featuring Survival. J Natl Cancer Inst 2017;109:djx030. [Crossref] [PubMed]
- Dineley KE, Votyakova TV, Reynolds IJ. Zinc inhibition of cellular energy production: implications for mitochondria and neurodegeneration. J Neurochem 2003;85:563-70. [Crossref] [PubMed]
- Kagan VE, Mao G, Qu F, et al. Oxidized arachidonic and adrenic PEs navigate cells to ferroptosis. Nat Chem Biol 2017;13:81-90. [Crossref] [PubMed]
- Tsvetkov P, Coy S, Petrova B, et al. Copper induces cell death by targeting lipoylated TCA cycle proteins. Science 2022;375:1254-61. [Crossref] [PubMed]
- Cen D, Brayton D, Shahandeh B, et al. Disulfiram facilitates intracellular Cu uptake and induces apoptosis in human melanoma cells. J Med Chem 2004;47:6914-20. [Crossref] [PubMed]
- Kirshner JR, He S, Balasubramanyam V, et al. Elesclomol induces cancer cell apoptosis through oxidative stress. Mol Cancer Ther 2008;7:2319-27. [Crossref] [PubMed]
- Tsvetkov P, Detappe A, Cai K, et al. Mitochondrial metabolism promotes adaptation to proteotoxic stress. Nat Chem Biol 2019;15:681-9. [Crossref] [PubMed]
- Tardito S, Bassanetti I, Bignardi C, et al. Copper binding agents acting as copper ionophores lead to caspase inhibition and paraptotic cell death in human cancer cells. J Am Chem Soc 2011;133:6235-42. [Crossref] [PubMed]
- Nagai M, Vo NH, Shin Ogawa L, et al. The oncology drug elesclomol selectively transports copper to the mitochondria to induce oxidative stress in cancer cells. Free Radic Biol Med 2012;52:2142-50. [Crossref] [PubMed]
- Shimada K, Reznik E, Stokes ME, et al. Copper-Binding Small Molecule Induces Oxidative Stress and Cell-Cycle Arrest in Glioblastoma-Patient-Derived Cells. Cell Chem Biol 2018;25:585-594.e7. [Crossref] [PubMed]
- Yip NC, Fombon IS, Liu P, et al. Disulfiram modulated ROS-MAPK and NFκB pathways and targeted breast cancer cells with cancer stem cell-like properties. Br J Cancer 2011;104:1564-74. [Crossref] [PubMed]
- Chen D, Cui QC, Yang H, et al. Disulfiram, a clinically used anti-alcoholism drug and copper-binding agent, induces apoptotic cell death in breast cancer cultures and xenografts via inhibition of the proteasome activity. Cancer Res 2006;66:10425-33. [Crossref] [PubMed]
- Skrott Z, Mistrik M, Andersen KK, et al. Alcohol-abuse drug disulfiram targets cancer via p97 segregase adaptor NPL4. Nature 2017;552:194-9. [Crossref] [PubMed]
- Chandrashekar DS, Bashel B, Balasubramanya SAH, et al. UALCAN: A Portal for Facilitating Tumor Subgroup Gene Expression and Survival Analyses. Neoplasia 2017;19:649-58. [Crossref] [PubMed]
- Chen F, Chandrashekar DS, Varambally S, et al. Pan-cancer molecular subtypes revealed by mass-spectrometry-based proteomic characterization of more than 500 human cancers. Nat Commun 2019;10:5679. [Crossref] [PubMed]
- Uhlen M, Zhang C, Lee S, et al. A pathology atlas of the human cancer transcriptome. Science 2017;357:eaan2507. [Crossref] [PubMed]
- Cerami E, Gao J, Dogrusoz U, et al. The cBio cancer genomics portal: an open platform for exploring multidimensional cancer genomics data. Cancer Discov 2012;2:401-4. [Crossref] [PubMed]
- Gao J, Aksoy BA, Dogrusoz U, et al. Integrative analysis of complex cancer genomics and clinical profiles using the cBioPortal. Sci Signal 2013;6:pl1. [Crossref] [PubMed]
- Hänzelmann S, Castelo R, Guinney J. GSVA: gene set variation analysis for microarray and RNA-seq data. BMC Bioinformatics 2013;14:7. [Crossref] [PubMed]
- Bindea G, Mlecnik B, Tosolini M, et al. Spatiotemporal dynamics of intratumoral immune cells reveal the immune landscape in human cancer. Immunity 2013;39:782-95. [Crossref] [PubMed]
- Yu G, Wang LG, Han Y, et al. clusterProfiler: an R package for comparing biological themes among gene clusters. OMICS 2012;16:284-7. [Crossref] [PubMed]
- Liu J, Lichtenberg T, Hoadley KA, et al. An Integrated TCGA Pan-Cancer Clinical Data Resource to Drive High-Quality Survival Outcome Analytics. Cell 2018;173:400-416.e11. [Crossref] [PubMed]
- Booth BA, Sartorelli AC. Synergistic interaction of kethoxal bis(thiosemicarbazone) and cupric ions in sarcoma 180. Nature 1966;210:104-5. [Crossref] [PubMed]
- Cappuccino JG, Banks S, Brown G, et al. The effect of copper and other metal ions on the antitumor activity of pyruvaldehyde bis(thiosemicarbazone). Cancer Res 1967;27:968-73. [PubMed]
- Singh P, Youden B, Yang Y, et al. Synergistic Multimodal Cancer Therapy Using Glucose Oxidase@CuS Nanocomposites. ACS Appl Mater Interfaces 2021;13:41464-72. [Crossref] [PubMed]
- Lelièvre P, Sancey L, Coll JL, et al. The Multifaceted Roles of Copper in Cancer: A Trace Metal Element with Dysregulated Metabolism, but Also a Target or a Bullet for Therapy. Cancers (Basel) 2020;12:3594. [Crossref] [PubMed]
- Habarou F, Hamel Y, Haack TB, et al. Biallelic Mutations in LIPT2 Cause a Mitochondrial Lipoylation Defect Associated with Severe Neonatal Encephalopathy. Am J Hum Genet 2017;101:283-90. [Crossref] [PubMed]
- Cronan JE. Progress in the Enzymology of the Mitochondrial Diseases of Lipoic Acid Requiring Enzymes. Front Genet 2020;11:510. [Crossref] [PubMed]
- Li Y, Zeng X. A novel cuproptosis-related prognostic gene signature and validation of differential expression in hepatocellular carcinoma. Front Pharmacol 2023;13:1081952. [Crossref] [PubMed]
- Mayer RL, Schwarzmeier JD, Gerner MC, et al. Proteomics and metabolomics identify molecular mechanisms of aging potentially predisposing for chronic lymphocytic leukemia. Mol Cell Proteomics 2018;17:290-303. [Crossref] [PubMed]
- Goh WQ, Ow GS, Kuznetsov VA, et al. DLAT subunit of the pyruvate dehydrogenase complex is upregulated in gastric cancer-implications in cancer therapy. Am J Transl Res 2015;7:1140-51. [PubMed]
- Li Z, Zhou H, Zhai X, et al. MELK promotes HCC carcinogenesis through modulating cuproptosis-related gene DLAT-mediated mitochondrial function. Cell Death Dis 2023;14:733. [Crossref] [PubMed]
- Chen J, Guccini I, Di Mitri D, et al. Compartmentalized activities of the pyruvate dehydrogenase complex sustain lipogenesis in prostate cancer. Nat Genet 2018;50:219-28. [Crossref] [PubMed]
- Li X, Jiang P, Li R, et al. Analysis of cuproptosis in hepatocellular carcinoma using multi-omics reveals a comprehensive HCC landscape and the immune patterns of cuproptosis. Front Oncol 2022;12:1009036. [Crossref] [PubMed]
- Chen J, Jiang Y, Shi H, et al. The molecular mechanisms of copper metabolism and its roles in human diseases. Pflugers Arch 2020;472:1415-29. [Crossref] [PubMed]
- Cox DW, Moore SD. Copper transporting P-type ATPases and human disease. J Bioenerg Biomembr 2002;34:333-8. [Crossref] [PubMed]
- Grimes A, Hearn CJ, Lockhart P, et al. Molecular basis of the brindled mouse mutant (Mo(br)): a murine model of Menkes disease. Hum Mol Genet 1997;6:1037-42. [Crossref] [PubMed]
- Haddad MR, Patel KD, Sullivan PH, et al. Molecular and biochemical characterization of Mottled-dappled, an embryonic lethal Menkes disease mouse model. Mol Genet Metab 2014;113:294-300. [Crossref] [PubMed]
- Hodgkinson VL, Dale JM, Garcia ML, et al. X-linked spinal muscular atrophy in mice caused by autonomous loss of ATP7A in the motor neuron. J Pathol 2015;236:241-50. [Crossref] [PubMed]
- Levinson B, Packman S, Gitschier J. Deletion of the promoter region in the Atp7a gene of the mottled dappled mouse. Nat Genet 1997;16:224-5. [Crossref] [PubMed]
- Reed V, Boyd Y. Mutation analysis provides additional proof that mottled is the mouse homologue of Menkes' disease. Hum Mol Genet 1997;6:417-23. [Crossref] [PubMed]
- Shao K, Shen H, Chen X, et al. Copper transporter gene ATP7A: A predictive biomarker for immunotherapy and targeted therapy in hepatocellular carcinoma. Int Immunopharmacol 2023;114:109518. [Crossref] [PubMed]
- Duan Q, Zhang H, Zheng J, et al. Turning Cold into Hot: Firing up the Tumor Microenvironment. Trends Cancer 2020;6:605-18. [Crossref] [PubMed]
- Prohaska JR, Downing SW, Lukasewycz OA. Chronic dietary copper deficiency alters biochemical and morphological properties of mouse lymphoid tissues. J Nutr 1983;113:1583-90. [Crossref] [PubMed]
- Lukasewycz OA, Prohaska JR. The immune response in copper deficiency. Ann N Y Acad Sci 1990;587:147-59. [Crossref] [PubMed]
- Koller LD, Mulhern SA, Frankel NC, et al. Immune dysfunction in rats fed a diet deficient in copper. Am J Clin Nutr 1987;45:997-1006. [Crossref] [PubMed]
- Percival SS. Copper and immunity. Am J Clin Nutr 1998;67:1064S-8S. [Crossref] [PubMed]
- Hopkins RG, Failla ML. Transcriptional regulation of interleukin-2 gene expression is impaired by copper deficiency in Jurkat human T lymphocytes. J Nutr 1999;129:596-601. [Crossref] [PubMed]
- Wang Y, Ding Y, Yao D, et al. Copper-Based Nanoscale Coordination Polymers Augmented Tumor Radioimmunotherapy for Immunogenic Cell Death Induction and T-Cell Infiltration. Small 2021;17:e2006231. [Crossref] [PubMed]