Eleven inflammation-related genes risk signature model predicts prognosis of patients with breast cancer
Highlight box
Key findings
• The 11-inflammation-related genes (IRGs) risk signature model can predict the survival of breast cancer patients. The proportion of T cells showed a decreased trend according to the increase of risk score, whereas macrophages M2 were more highly distributed in the high-risk group. The expressions of IL12B and SCARF1 may serve as potential targets for therapy of breast cancer.
What is known and what is new?
• Changes in gene expression and inflammation are associated with the occurrence and development of tumors.
• Our study was the first to involve a more comprehensive predictive model for breast cancer of genetic variation, genetic mutation, inflammatory cell infiltration, and drug sensitivity analysis.
What is the implication, and what should change now?
• IL12B and SCARF1 may serve as potential markers to predict therapeutic efficacy in breast cancer and the mechanisms associated with them need to be further explored. The nomogram we established can guide clinical evaluation of survival time and treatment response of breast cancer patients.
Introduction
Breast cancer has replaced lung cancer as the most commonly diagnosed cancer (1). With over 2.3 million new cases and 685,000 deaths occurring in 2020, by 2040, it is predicted that there will be over 3 million new cases and 1 million deaths due to breast cancer every year (1,2). Despite the availability of multiple therapies, including surgery, radiotherapy, chemotherapy, endocrine therapy, and immunotherapy, the median survival of patients with locally advanced or metastatic breast cancer is approximately 2–4 years. Currently, about 30–50% of patients who are diagnosed at earlier stages will progress to metastatic breast cancer (3,4). Therefore, breast cancer, as a genetic and molecular heterogeneous disease, is a serious global threat to women’s health. One of the hallmarks of malignant tumors is the change of gene expression (4,5). Genetic, epigenetic, or transcriptomic alterations are associated with the genesis and progression of breast cancer (6). Gene expression data has been analyzed to reveal distinct cancer subtypes, critical molecular drivers, and prognostic signatures (7), and to predict cancer vulnerability, therapy response, and mortality (8,9).
In 1863, the connections between inflammation and cancer were firstly recognized (10,11). On the one hand, inflammation causes DNA damage that leads to mutations, and in course of time accumulated mutations result in the occurrence of cancers (12). On the other hand, DNA damage contributes to inflammation, where tumors create their own local inflammation microenvironment to recruit various immune cells such as T lymphocytes, B cells, dendritic cells, macrophages, neutrophils, monocytes, and natural killer (NK) cells, thus promoting the growth of the tumors (10,13,14). The effects of cancer-associated inflammation not only comprise beneficial effects but also detrimental systemic effects (15). T-cell-mediated cytotoxicity leads to tumor suppression (16). The so-called B symptoms such as fevers, sweats, and weight loss as well as cachexia and a series of other paraneoplastic symptoms constitute the negative systemic reaction to malignancy (15,17,18).
As previously mentioned, the tumor microenvironment infiltrated by inflammatory cells is an indispensable participant in breast cancer development, progression, survival, and migration (13,19). Interleukin (IL)-6, as a pro-inflammatory cytokine, facilitates the survival of cancer cells through promoting endothelial cell migration and proliferation (20,21). Furthermore, the over-expression of the IL-6 family cytokines combining with vascular endothelial growth factor (VEGF) A leads to significantly worse survival in human epidermal growth factor receptor 2 (HER2)-negative breast cancer (22). The over-expression of IL-1β causes higher breast cancer stage and poorer prognosis (23,24). In triple-negative breast cancer (TNBC), A20/TNFAIP3 is up-regulated and its expression level is highly linked to a poor prognosis of metastasis-free patients (25,26). Recently, several predictive models based on inflammation-related genes (IRGs) have been developed and validated to evaluate prognosis as well as predicting survival probability in breast cancer patients. In 2019, Zhao et al. developed a 3-messenger RNA (mRNA) (TBX21, TGIF2, and CYCS) model of breast cancer by collecting clinical materials and mRNA data from The Cancer Genome Atlas (TCGA), a research team (Cat. #BR1504a, Alenabio Company, Shanxi, China), and Gene Expression Omnibus (GEO) (27). Zang et al. developed an inflammatory risk model to indicate prognosis and reflect the immune microenvironment in breast cancer using the databases from TCGA, GEO, and Molecular Signatures Database (MSigDB) (28). In 2022, a tumor immune-inflammation signature for breast cancer was explored by Liu et al. by extracting the datasets from The Cancer Genome Atlas-Breast Invasive Carcinoma (TCGA-BRCA), Molecular Taxonomy of Breast Cancer International Consortium (METABRIC), and MSigDB (29). Although the models in the first and second studies lacked validations in vitro cell experiments, the second study identified expression levels of the 5-gene signature by quantitative real time polymerase chain reaction (qRT-PCR) in breast tissue samples. In present study, we systematically and comprehensively analyzed the expression levels of IRGs in breast cancer patients through the datasets from TCGA and GEO. We explored the links between expression of genes and sensitivity of drugs to screen possible potential biomarkers guiding the clinical application of targeted therapy for breast cancer. We constructed an IRG risk signature for patients with breast cancer by conducting least absolute shrinkage and selection operator (LASSO). We present this article in accordance with the TRIPOD reporting checklist (available at https://tcr.amegroups.com/article/view/10.21037/tcr-24-215/rc).
Methods
Data acquisition
We downloaded a list of 200 IRGs from the MSigDB database (http://www.gsea-msigdb.org/gsea/msigdb/index.jsp). RNA-sequence (RNA-seq) data, the simple nucleotide variation data from the “Masked Somatic Mutation” category, and the corresponding clinical information of 1,092 female breast cancer patients were obtained from TCGA (https://portal.gdc.cancer.gov). The dataset of GSE96058 was downloaded from GEO (https://www.ncbi.nlm.nih.gov/geo/).
Identification of differentially expressed IRGs (DEIRGs)
DESeq2 (R package version 1.34) in the R software (version 4.1.1; R Foundation for Statistical Computing, Vienna, Austria) was used to normalize raw read count of RNA-seq from TCGA and calculate differentially expressed genes (DEGs). DEGs were identified as genes with log2(fold change) ≥1.0 and adjusted P value <0.05 (tumor tissues vs. normal tissues). Subsequently, the DEIRGs were identified from the DEGs. The heatmap was drawn by pheatmap package (R package version 1.0.12).
Functional enrichment analysis of DEIRGs
Gene Ontology (GO) and Kyoto Encyclopedia of Genes and Genomes (KEGG) were carried out to annotate the functions of the DEIRGs with clusterProfiler (R package version 4.0.5). A P value adjusted with Benjamini and Hochberg method less than 0.05 was considered statistically significant. To clarify the connections between genes and enriched GO terms, a network was constructed with Cytoscape (version 3.9.0; https://cytoscape.org/).
Construction and validation of multiple-IRG risk signature
We used LASSO regression analysis to construct the prognostic model while using the TCGA cohort as training dataset with “glmnet” package (R package version 4.1–7). The RNA-seq data was standardized by log2(normalized read counts +1) transformation. With the addition of λ, LASSO tends to shrink the regression coefficients to zero. For 10-fold cross-validation, we chose a λ value that produced the minimum cross-validation error as our optimal λ value. We calculated a risk score of each sample as follows: risk score = sum of coefficients from the LASSO regression × normalized read counts. The median value of the risk score was used to divide the patients into high-risk or low-risk groups. We used the log-rank test with survival package (R package version 3.5-5) to analyze the overall survival (OS) of the patients. We drew time-dependent receiver operating characteristic (ROC) curves to calculate the area under the ROC curves (AUCs) to measure the predictive accuracy of the prognostic model using ROCR package (R package version 1.0-11). GSE96058 was used as test dataset to validate the multiple-IRG risk signature.
Construction and validation of nomogram
The univariate and multivariate Cox proportional hazards regression analyses were used to identify key factors associated with OS of breast cancer patients from risk score and other clinical factors. We used rms package (R package version 6.7-1) to draw a nomogram with the key factors as well as calibration curves. The calibration curves were used to assess whether the actual outcomes of 3-, 5-, and 10-year OS were similar to the predicted outcomes (30).
Gene set variation analysis (GSVA)
We obtained 50 hallmark pathways with msigdbr package (R package version 7.5.1) and removed the genes involved in two or more pathways (31). Then, GSVA was performed to assign pathway activity estimates to individual patients using GSVA package (R package version 1.40.1). Finally, we compared the pathway activity estimates between high- and low-risk patients with limma package (R package version 3.50.3).
Mutation analysis
We used maftools package (R package version 2.8.05) to compare mutation load between high- and low-risk patients.
Cell type estimation
Cell type estimation was performed using Estimation of Stromal and Immune cells in Malignant Tumor tissues (ESTIMATE) with TCGA dataset. We compared cell enrichments between high- and low-risk groups. The correlations between risk score and the enrichments of immune cells were analyzed by using Spearman’s correlation.
Drug response analysis
We used Cancer Therapeutics Response Portal (CTRP) (32) as a training dataset to predict imputed sensitivity score for TCGA dataset with oncoPredict (33) (R package version 0.2). The difference in drug response between high and low risk groups were assessed using Wilcoxon test. The correlations between expression levels of genes and imputed sensitivity scores were assessed using Spearman’s correlation. In the drug response analysis, we only included the approved drugs.
The study was conducted in accordance with the Declaration of Helsinki (as revised in 2013).
Results
DEGs associated with inflammation
We obtained 65 DEIRGs after comparing 1,092 breast cancer tissues with 113 paracancerous tissues in TCGA (Figure 1A). Figure 1B displays the dissimilarity of expression levels of the 65 IRGs in breast cancer tissues and paracancerous tissues.
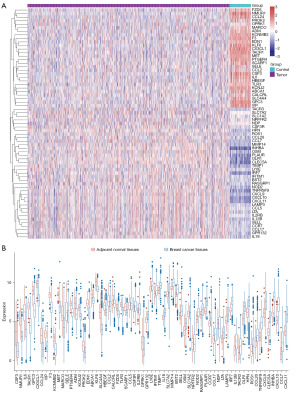
Enrichment analysis of the DEIRGs
We applied GO and KEGG enrichment analysis to annotate the functions in all DEIRGs. The genes were annotated into three ontologies in GO annotation, including cellular component, molecular function, and biological process. As shown in Figure 2A, the DEIRGs were mainly distributed in endocytic vesicle, external side of plasma membrane, and endoplasmic reticulum lumen. The top 3 terms enriched molecular function were the activity of signaling receptor activator and cytokine as well as cytokine receptor binding. Cell chemotaxis, response to molecule of bacterial origin, and myeloid leukocyte migration were the top 3 terms enriched in biological process.
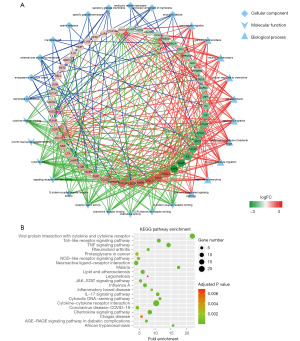
In the KEGG pathway analysis, the DEIRGs were mainly enriched in viral protein interaction with cytokine and cytokine receptor, cytokine-cytokine receptor interaction, tumor necrosis factor (TNF) signaling pathway, and chemokine signaling pathway (Figure 2B).
Construction and validation of the 11-IRGs risk signature
LASSO Cox regression analysis was performed to screen crucial IRGs from the 65 DEIRGs. Ultimately, 11 IRGs were obtained to construct the prognostic model (Figure 3A,3B). The 11 IRGs and their corresponding regression coefficients are shown in Figure 3C. We calculated risk scores for all patients, and separated the patients into low- or high-risk score groups. Survival analysis indicated that the low-risk group had fewer deaths and longer survival time (Figure 3D-3G). The median survival days of the low- and high-risk groups were 6,593 and 3,462, respectively [Figure 3G, hazard ratio (HR) =1.926, 95% confidence interval (CI): 1.382–2.684, P<0.001]. ROC curve analysis indicated that the AUCs for 3-, 5-, and 10-year survival were 0.638, 0.633, and 0.617, respectively (Figure 3H). These results manifested that the model could distinguish high-risk patients from low-risk patients.
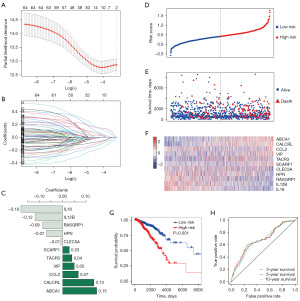
The GSE96058 cohort was used to evaluate the performance of the 11-IRGs risk signature. We calculated risk score for each patient and then stratified them into high- and low-risk groups. Patients with low-risk scores had longer survival time and less deaths (Figure 4A-4C). The low-risk group had longer survival time than high-risk group (Figure 4D; HR =1.253, 95% CI: 1.016–1.545, P=0.03). In ROC curve analysis, the AUCs were 0.563, 0.530, and 0.528 for 3-, 5-, and 10-year survival (Figure 4E). We also investigated the basal characteristics of patients in the different risk groups. In the TCGA cohort, only estrogen receptor (ER) showed a significant difference between the low- and high-risk group (Table 1). In the GSE96058 cohort, the difference existed in age and grade (Table 1).
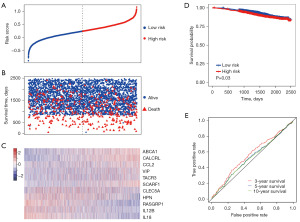
Table 1
Characteristics | TCGA cohort | GSE96058 cohort | |||||
---|---|---|---|---|---|---|---|
High risk | Low risk | P value | High risk | Low risk | P value | ||
Age | 0.71 | 0.006 | |||||
<60 years | 282 | 288 | 603 | 681 | |||
≥60 years | 246 | 240 | 1,101 | 1,024 | |||
Stage | 0.11 | – | |||||
I | 86 | 95 | – | – | |||
II | 288 | 309 | – | – | |||
III | 130 | 107 | – | – | |||
IV | 13 | 6 | – | – | |||
Grade | |||||||
G1 | – | – | 317 | 188 | <0.001 | ||
G2 | – | – | 822 | 771 | |||
G3 | – | – | 535 | 711 | |||
ER | <0.001 | 0.36 | |||||
Positive | 418 | 356 | 1,474 | 1,461 | |||
Negative | 89 | 146 | 120 | 134 | |||
PR | 0.058 | 0.47 | |||||
Positive | 352 | 321 | 1,307 | 1,337 | |||
Negative | 153 | 180 | 209 | 198 | |||
HER2 | 0.30 | 0.90 | |||||
Positive | 87 | 69 | 219 | 219 | |||
Negative | 278 | 266 | 1,413 | 1,430 |
All patients from the two datasets were included, including those with triple-negative breast cancer. TCGA, The Cancer Genome Atlas; GSE, National Center of Biotechnology Information Gene Expression Omnibus series; ER, estrogen receptor; PR, progesterone receptor; HER2, human epidermal growth receptor 2.
Construction and validation of nomogram
In the univariate Cox analysis, six risk factors were significantly relevant with survival of breast cancer patients, including risk score, age, stage, tumor (T), node (N), and metastasis (M) (Figure 5A). Then, we carried out multivariate Cox analysis with the 6 factors, and found that risk score, age, and stage were the three independent prognostic factors (Figure 5B). Thereby, we used the three factors to develop a nomogram to predict the survival time of breast cancer patients (Figure 5C). As shown in Figure 5D-5F, calibration curves revealed that the nomogram model owned high accuracy in predicting 3-, 5-, and 10-year OS.
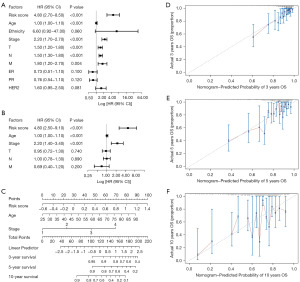
GSVA between high- and low-risk groups
Figure S1 shows the pathways significantly enriched in the high- and low-risk groups. Among the 50 pathways, 12 pathways had higher activities and 24 pathways had lower activities in the high-risk group compared with the low-risk group.
Correlation between gene mutation and risk score
Mutations in genes have been shown to play key roles in both the development of tumors and their response to therapy (34), thus we compared mutation load between high- and low-risk patients. However, no difference was observed between the two groups (Figure S2).
Status of immune infiltration
We predicted the composition of infiltrating immune cells in breast cancer tissues with TCGA cohort. We noted that the proportion of T cells showed a decreased trend according to the increase of risk score (Figure 6A). The differences between low- and high-risk groups in cell composition were observed in 15 types of immune cells (Figure 6B). Most of the immune cells in Figure 6B were enriched in the low-risk group, including T cells CD4 memory activated, T cells CD8, T cells follicular helper, and T cells regulatory Treg, and several cells showed an opposite trend, such as macrophages M2, which were also confirmed in the following Pearson correlation analysis (Figure 6C-6G).
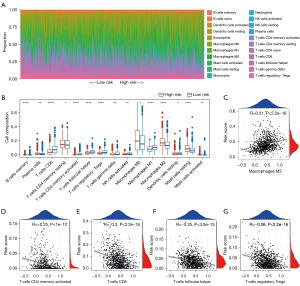
The difference in drug response between high- and low-risk patients
We identified 67 approved drugs that showed a different effect between high- and low-risk patients (Figure 7A). Then, the correlations between expression levels of the 11 genes in the signature and imputed sensitivity scores were assessed using Spearman’s correlation. Totally, 72 gene-drug pairs with P<0.05 and absolute value of Pearson correlation coefficient >0.25 were identified (Figure 7B). The top 2 gene-drug pairs are shown in Figure 7C,7D.
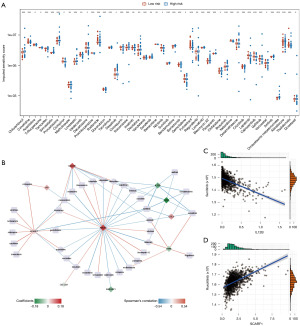
Discussion
Inflammation occurs when the body’s immune system responds to injury or infection to maintain homeostasis (35). Studies have shown that inflammation is involved in the initiation, growth, progression, and metastasis of tumors (36). Perhaps the most striking case is that ulcerative colitis, a type of inflammatory disease, increases the risk of bowel cancer (37). Analogously, hepatitis B and chronic gastritis with Helicobacter pylori increase the risks of liver and stomach cancer (38). It is noteworthy that chronic inflammation is remarkably associated with breast cancer recurrence (39) and uncontrolled inflammation kills more than a third of breast cancer patients (40).
In our study, we firstly obtained 65 DEIRGs from TCGA dataset. Then an 11-IRGs risk signature model was established to calculate risk score of breast cancer patients. We further constructed a nomogram to predict 3-, 5-, or 10-year survival of breast cancer patients. By now, several models of inflammation-related breast cancer prognosis have been published. In the 3-mRNA model constructed by Zhao et al., only 5-year survival was predicted for breast cancer patients (27). In the 5-inflammatory-gene signature developed by Zang et al., two validation sets were used to test the predictive feasibility of the model (28). They only analyzed risk score for differences in gene variation and signaling pathways. In our study, we explored the differences between high- and low-risk groups in genetic variation, genetic mutation, inflammatory cell infiltration, and drug sensitivity analysis.
Tumor microenvironment is a complex cellular ecosystem, in which inflammatory cells infiltration could interact with tumor cells, thus affecting tumor progression and therapeutic efficacy (41). The inflammatory cells recruited to the tumor site exhibited dual natures: some inhibited tumor growth, such as T cells and NK cells, whereas some promoted tumor progression (42). As a key immune cell in the tumor microenvironment, the abundance of macrophages correlates with adverse clinical outcomes, especially type M2 macrophages. Macrophage M2 have been shown to promote angiogenesis, invasion, movement, and infiltration at site of metastasis to stimulate the extravasation and continuous growth of tumor cells (43). Increased macrophage M2 infiltration has been associated with metastasis and poor prognosis in osteosarcoma (44). Macrophage M2 also accelerated lymphoma progression and deteriorates the patient’s prognosis (45). In addition, CD8+ T and CD4+ T cells have been shown to have possible anti-tumor effects on breast cancer (42). Herein, more macrophage cells infiltrated in the high-risk group indicated a worse prognosis. Our analysis of the correlation between risk scores and immune-infiltrating cells confirmed the above findings.
In drug sensitivity analysis between high- and low-risk patients, IL12B, of which high expression was negatively correlated with risk core, was associated with increased sensitivity to sunitinib. High expression of SCARF1 was negatively correlated with sensitivity of ruxolitinib. IL-12 is a multipotent cytokine encoded by IL12B that has been shown to be effective in anti-tumor therapy (46). Previous studies have shown that anti-tumor therapies combined with IL-12 gene therapy potentiated the efficacy of experimental cancer therapy. Co-injection of IL-12 in adenovirus vector and paclitaxel enhanced the therapeutic effect of melanoma (47). In mammary tumors, animals injected intratumorally with IL-2 and IL-12 had a higher rate of tumor regression (48). Sunitinib is a tyrosine kinase inhibitor (49) which has become the optimum first-line treatment for advanced renal cell carcinoma when united with immune checkpoint inhibitors (50). Together, they have the ability to antagonize vascular endothelial growth factor receptors (50). Another study elucidated that immune checkpoint inhibition in combination significantly prolonged OS compared with sunitinib alone in metastatic renal cell carcinoma (51). SCARF1 expresses in several cells, such as dendritic cells, epithelial cells, and macrophages (51). As a member of the scavenger receptor protein superfamily (52), it not only promotes the specific recruitment of pro-inflammatory CD4+ T cells to hepatocarcinoma tissues (53), but also binds and clears apoptotic cells (52). Moreover, high expression of SCARF1 was found to be associated with lung adenocarcinoma (54). Li et al. validated that ERβ2 promoted TNBC metastasis by binding SCARF1 (55). Ruxolitinib is a JAK inhibitor that has been shown to work by inhibiting Janus kinase, which in turn inhibits cytokine receptor signaling pathways (56). It has anti-inflammatory as well as immunosuppressive effects (57). During the treatment of myelofibrosis and high-risk polycythemia vera, ruxolitinib has been shown to affect immune function and increase the risk of infection (58). Therefore, the expressions of IL12B and SCARF1 may serve as potential targets for therapy of breast cancer, but the specific mechanism needs further investigation.
In this study, we provided a comprehensive tool to predict the prognosis of breast cancer. However, the research involved deficiencies, as follows. First, the prediction model was based on public databases and was not evaluated in a prospective cohort. Second, the model was only tested in one validation set, and the sample size was not large enough to validate the feasibility of the model. Third, the molecular mechanisms underlying IL12B and SCARF1 as prognostic biomarkers in breast cancer were unclear. Taken together, the roles of the IL12B and SCARF1 genes in breast cancer warrant further investigation.
Conclusions
We have provided a comprehensive prognostic tool for predicting breast cancer survival. Drug sensitivity analyses further hinted at the possibility that we use IL12B and SCARF1 as potential markers to predict therapeutic efficacy in breast cancer, although the mechanisms associated with them need to be further explored.
Acknowledgments
Funding: This work was supported by
Footnote
Reporting Checklist: The authors have completed the TRIPOD reporting checklist. Available at https://tcr.amegroups.com/article/view/10.21037/tcr-24-215/rc
Peer Review File: Available at https://tcr.amegroups.com/article/view/10.21037/tcr-24-215/prf
Conflicts of Interest: All authors have completed the ICMJE uniform disclosure form (available at https://tcr.amegroups.com/article/view/10.21037/tcr-24-215/coif). The authors have no conflicts of interest to declare.
Ethical Statement: The authors are accountable for all aspects of the work in ensuring that questions related to the accuracy or integrity of any part of the work are appropriately investigated and resolved. The study was conducted in accordance with the Declaration of Helsinki (as revised in 2013).
Open Access Statement: This is an Open Access article distributed in accordance with the Creative Commons Attribution-NonCommercial-NoDerivs 4.0 International License (CC BY-NC-ND 4.0), which permits the non-commercial replication and distribution of the article with the strict proviso that no changes or edits are made and the original work is properly cited (including links to both the formal publication through the relevant DOI and the license). See: https://creativecommons.org/licenses/by-nc-nd/4.0/.
References
- Arnold M, Morgan E, Rumgay H, et al. Current and future burden of breast cancer: Global statistics for 2020 and 2040. Breast 2022;66:15-23. [Crossref] [PubMed]
- Sung H, Ferlay J, Siegel RL, et al. Global Cancer Statistics 2020: GLOBOCAN Estimates of Incidence and Mortality Worldwide for 36 Cancers in 185 Countries. CA Cancer J Clin 2021;71:209-49. [Crossref] [PubMed]
- Cazzaniga ME, Biganzoli L, Cortesi L, et al. Treating advanced breast cancer with metronomic chemotherapy: what is known, what is new and what is the future? Onco Targets Ther 2019;12:2989-97. [Crossref] [PubMed]
- Kashyap D, Pal D, Sharma R, et al. Global Increase in Breast Cancer Incidence: Risk Factors and Preventive Measures. Biomed Res Int 2022;2022:9605439. [Crossref] [PubMed]
- Pranav P, Palaniyandi T, Baskar G, et al. Gene expressions and their significance in organoid cultures obtained from breast cancer patient-derived biopsies. Acta Histochem 2022;124:151910. [Crossref] [PubMed]
- Abd-Elnaby M, Alfonse M, Roushdy M. Classification of breast cancer using microarray gene expression data: A survey. J Biomed Inform 2021;117:103764. [Crossref] [PubMed]
- Nolan E, Lindeman GJ, Visvader JE. Deciphering breast cancer: from biology to the clinic. Cell 2023;186:1708-28. [Crossref] [PubMed]
- Kourou K, Exarchos TP, Exarchos KP, et al. Machine learning applications in cancer prognosis and prediction. Comput Struct Biotechnol J 2014;13:8-17. [Crossref] [PubMed]
- Yu Z, Chen H, You J, et al. Double Selection Based Semi-Supervised Clustering Ensemble for Tumor Clustering from Gene Expression Profiles. IEEE/ACM Trans Comput Biol Bioinform 2014;11:727-40. [Crossref] [PubMed]
- Balkwill F, Mantovani A. Inflammation and cancer: back to Virchow? Lancet 2001;357:539-45. [Crossref] [PubMed]
- Mantovani A, Allavena P, Sica A, et al. Cancer-related inflammation. Nature 2008;454:436-44. [Crossref] [PubMed]
- Kay J, Thadhani E, Samson L, et al. Inflammation-induced DNA damage, mutations and cancer. DNA Repair (Amst) 2019;83:102673. [Crossref] [PubMed]
- Coussens LM, Werb Z. Inflammation and cancer. Nature 2002;420:860-7. [Crossref] [PubMed]
- Khandia R, Munjal A. Interplay between inflammation and cancer. Adv Protein Chem Struct Biol 2020;119:199-245. [Crossref] [PubMed]
- Diakos CI, Charles KA, McMillan DC, et al. Cancer-related inflammation and treatment effectiveness. Lancet Oncol 2014;15:e493-503. [Crossref] [PubMed]
- Roxburgh CS, McMillan DC. The role of the in situ local inflammatory response in predicting recurrence and survival in patients with primary operable colorectal cancer. Cancer Treat Rev 2012;38:451-66. [Crossref] [PubMed]
- Guthrie GJ, Charles KA, Roxburgh CS, et al. The systemic inflammation-based neutrophil-lymphocyte ratio: experience in patients with cancer. Crit Rev Oncol Hematol 2013;88:218-30. [Crossref] [PubMed]
- McMillan DC. The systemic inflammation-based Glasgow Prognostic Score: a decade of experience in patients with cancer. Cancer Treat Rev 2013;39:534-40. [Crossref] [PubMed]
- Mittal S, Brown NJ, Holen I. The breast tumor microenvironment: role in cancer development, progression and response to therapy. Expert Rev Mol Diagn 2018;18:227-43. [Crossref] [PubMed]
- De Palma M, Biziato D, Petrova TV. Microenvironmental regulation of tumour angiogenesis. Nat Rev Cancer 2017;17:457-74. [Crossref] [PubMed]
- Marech I, Leporini C, Ammendola M, et al. Classical and non-classical proangiogenic factors as a target of antiangiogenic therapy in tumor microenvironment. Cancer Lett 2016;380:216-26. [Crossref] [PubMed]
- Tawara K, Scott H, Emathinger J, et al. Co-Expression of VEGF and IL-6 Family Cytokines is Associated with Decreased Survival in HER2 Negative Breast Cancer Patients: Subtype-Specific IL-6 Family Cytokine-Mediated VEGF Secretion. Transl Oncol 2019;12:245-55. [Crossref] [PubMed]
- Korobeinikova E, Ugenskiene R, Insodaite R, et al. Association of angiogenesis and inflammation-related gene functional polymorphisms with early-stage breast cancer prognosis. Oncol Lett 2020;19:3687-700. [Crossref] [PubMed]
- Litmanovich A, Khazim K, Cohen I. The Role of Interleukin-1 in the Pathogenesis of Cancer and its Potential as a Therapeutic Target in Clinical Practice. Oncol Ther 2018;6:109-27. [Crossref] [PubMed]
- Lee JH, Jung SM, Yang KM, et al. A20 promotes metastasis of aggressive basal-like breast cancers through multi-monoubiquitylation of Snail1. Nat Cell Biol 2017;19:1260-73. [Crossref] [PubMed]
- Song C, Kendi AT, Lowe VJ, et al. The A20/TNFAIP3-CDC20-CASP1 Axis Promotes Inflammation-mediated Metastatic Disease in Triple-negative Breast Cancer. Anticancer Res 2022;42:681-95. [Crossref] [PubMed]
- Zhao S, Shen W, Du R, et al. Three inflammation-related genes could predict risk in prognosis and metastasis of patients with breast cancer. Cancer Med 2019;8:593-605. [Crossref] [PubMed]
- Zang H, Ni G, Gong L. Characterization of 5-inflammatory-gene signature to affect the immune status and predict prognosis in breast cancer. Cent Eur J Immunol 2022;47:218-33. [Crossref] [PubMed]
- Liu Y, Ouyang W, Huang H, et al. Identification of a tumor immune-inflammation signature predicting prognosis and immune status in breast cancer. Front Oncol 2023;12:960579. [Crossref] [PubMed]
- Zhou ZR, Wang WW, Li Y, et al. In-depth mining of clinical data: the construction of clinical prediction model with R. Ann Transl Med 2019;7:796. [Crossref] [PubMed]
- Yu X, Xie L, Ge J, et al. Integrating single-cell RNA-seq and spatial transcriptomics reveals MDK-NCL dependent immunosuppressive environment in endometrial carcinoma. Front Immunol 2023;14:1145300. [Crossref] [PubMed]
- Rees MG, Seashore-Ludlow B, Cheah JH, et al. Correlating chemical sensitivity and basal gene expression reveals mechanism of action. Nat Chem Biol 2016;12:109-16. [Crossref] [PubMed]
- Maeser D, Gruener RF, Huang RS. oncoPredict: an R package for predicting in vivo or cancer patient drug response and biomarkers from cell line screening data. Brief Bioinform 2021;22:bbab260. [Crossref] [PubMed]
- Jacqueline C, Biro PA, Beckmann C, et al. Cancer: A disease at the crossroads of trade-offs. Evol Appl 2017;10:215-25. [Crossref] [PubMed]
- Chen T, Liu J, Li S, et al. The role of protein arginine N-methyltransferases in inflammation. Semin Cell Dev Biol 2024;154:208-14. [Crossref] [PubMed]
- Greten FR, Grivennikov SI. Inflammation and Cancer: Triggers, Mechanisms, and Consequences. Immunity 2019;51:27-41. [Crossref] [PubMed]
- Tang X, Cui Y, Feng B. The chemical constituents and metabolite profiles of Huangqin decoction in normal and ulcerative colitis rats by UHPLC-Q-TOF/MS analysis. J Pharm Biomed Anal 2024;237:115763. [Crossref] [PubMed]
- Trinchieri G. Cancer and inflammation: an old intuition with rapidly evolving new concepts. Annu Rev Immunol 2012;30:677-706. [Crossref] [PubMed]
- Pierce BL, Ballard-Barbash R, Bernstein L, et al. Elevated biomarkers of inflammation are associated with reduced survival among breast cancer patients. J Clin Oncol 2009;27:3437-44. [Crossref] [PubMed]
- Wang D, Yin GH. Non-coding RNAs mediated inflammation in breast cancers. Semin Cell Dev Biol 2024;154:215-20. [Crossref] [PubMed]
- Casey SC, Amedei A, Aquilano K, et al. Cancer prevention and therapy through the modulation of the tumor microenvironment. Semin Cancer Biol 2015;35:S199-223. [Crossref] [PubMed]
- Li W, Xu M, Li Y, et al. Comprehensive analysis of the association between tumor glycolysis and immune/inflammation function in breast cancer. J Transl Med 2020;18:92. [Crossref] [PubMed]
- Qian BZ, Pollard JW. Macrophage diversity enhances tumor progression and metastasis. Cell 2010;141:39-51. [Crossref] [PubMed]
- Cersosimo F, Lonardi S, Bernardini G, et al. Tumor-Associated Macrophages in Osteosarcoma: From Mechanisms to Therapy. Int J Mol Sci 2020;21:5207. [Crossref] [PubMed]
- Xiong X, Xie X, Wang Z, et al. Tumor-associated macrophages in lymphoma: From mechanisms to therapy. Int Immunopharmacol 2022;112:109235. [Crossref] [PubMed]
- Lasek W, Zagożdżon R, Jakobisiak M. Interleukin 12: still a promising candidate for tumor immunotherapy? Cancer Immunol Immunother 2014;63:419-35. [Crossref] [PubMed]
- Cao L, Zeng Q, Xu C, et al. Enhanced antitumor response mediated by the codelivery of paclitaxel and adenoviral vector expressing IL-12. Mol Pharm 2013;10:1804-14. [Crossref] [PubMed]
- Addison CL, Bramson JL, Hitt MM, et al. Intratumoral coinjection of adenoviral vectors expressing IL-2 and IL-12 results in enhanced frequency of regression of injected and untreated distal tumors. Gene Ther 1998;5:1400-9. [Crossref] [PubMed]
- Buart S, Diop MK, Damei I, et al. Sunitinib Treatment of VHL C162F Cells Slows Down Proliferation and Healing Ability via Downregulation of ZHX2 and Confers a Mesenchymal Phenotype. Cancers (Basel) 2023;16:34. [Crossref] [PubMed]
- Sweeney PL, Suri Y, Basu A, et al. Mechanisms of tyrosine kinase inhibitor resistance in renal cell carcinoma. Cancer Drug Resist 2023;6:858-73. [Crossref] [PubMed]
- Patten DA. SCARF1: a multifaceted, yet largely understudied, scavenger receptor. Inflamm Res 2018;67:627-32. [Crossref] [PubMed]
- Jorge AM, Lao T, Kim R, et al. SCARF1-Induced Efferocytosis Plays an Immunomodulatory Role in Humans, and Autoantibodies Targeting SCARF1 Are Produced in Patients with Systemic Lupus Erythematosus. J Immunol 2022;208:955-67. [Crossref] [PubMed]
- Patten DA, Wilkinson AL, O'Rourke JM, et al. Prognostic Value and Potential Immunoregulatory Role of SCARF1 in Hepatocellular Carcinoma. Front Oncol 2020;10:565950. [Crossref] [PubMed]
- Feng N, Wang Y, Zheng M, et al. Genome-wide analysis of DNA methylation and their associations with long noncoding RNA/mRNA expression in non-small-cell lung cancer. Epigenomics 2017; Epub ahead of print. [Crossref] [PubMed]
- Chen D, Wang M, Zhang H, et al. Estrogen receptor β2 (ERβ2)-mediated upregulation of hsa_circ_0000732 promotes tumor progression via sponging microRNA-1184 in triple-negative breast cancer (TNBC). Inflamm Res 2022;71:255-66. [Crossref] [PubMed]
- Adesola AA, Cozma MA, Chen YF, et al. Risk of hepatitis B reactivation in patients with myeloproliferative neoplasms treated with ruxolitinib. World J Hepatol 2023;15:1188-95. [Crossref] [PubMed]
- Elli EM, Baratè C, Mendicino F, et al. Mechanisms Underlying the Anti-inflammatory and Immunosuppressive Activity of Ruxolitinib. Front Oncol 2019;9:1186. [Crossref] [PubMed]
- Lussana F, Cattaneo M, Rambaldi A, et al. Ruxolitinib-associated infections: A systematic review and meta-analysis. Am J Hematol 2018;93:339-47. [Crossref] [PubMed]