Identification of a novel cellular senescence-related lncRNA signature for prognosis and immune response in osteosarcoma
Highlight box
Key findings
• This study established a prognostic model consisting of six cellular senescence-related long non-coding RNAs (CSR-lncRNAs) and uncovered potential markers associated with immune infiltration in osteosarcoma.
What is known and what is new?
• While recent research has leveraged lncRNAs to develop diagnostic and prognostic models for numerous cancers, the field of osteosarcoma remains relatively unexplored.
• In this study the prediction model of six-CSR-lncRNA is important for accurately identifying independent prognostic factors in patients with osteosarcoma and for individualized treatment.
What is the implication, and what should change now?
• The identified CSR-lncRNA signature may be prognostic biomarkers for osteosarcoma. In addition, it could be employed by clinicians to estimate prognosis and immune therapeutic response.
Introduction
Background
Osteosarcoma is one of the most prevalent primary malignant bone tumors, characterized by its malignancy and invasiveness with an incidence rate of 2–4 cases/million, accounting for 20% of primary malignant bone tumors in both younger and older individuals (1). Younger patients, specifically those aged between 10 and 14 years, within Asian and African populations, as well as adults exceeding 65 years of age in European and American populations, are particularly susceptible to severe health risks associated with osteosarcoma (2). Although treatment modalities, such as surgical intervention (3,4), chemotherapy, radiotherapy, and immunotherapy (5), have shown promise clinically, their efficacy remains uncertain. Over time, relentless research efforts have managed to boost the 5-year survival rates of patients affected by osteosarcoma to approximately 60–65% (6). However, a quarter to a third of these patients experience recurrence or metastasis, emphasizing the pressing need for innovative treatments (7). Therefore, understanding the intricate mechanisms underlying osteosarcoma and identifying novel biomarkers and therapeutic options are essential for refining osteosarcoma management.
The concept of cellular senescence was first proposed in the 1960s by Hayflick and Moorhead who described this phenomenon within the context of restricted replication of primary human fibroblasts under laboratory conditions (8). Today, this irreversible halt in the cell cycle is recognized as a hallmark of aging, with potential implications for accelerating the aging process in organisms (9). Notably, although this cellular arrest could inhibit the growth of potentially cancerous cells, it could simultaneously activate immune mechanisms to thwart tumor development through a process known as the senescence-associated secretory phenotype, which involves the release of several immune-modulating substances (10). However, owing to the dual nature of senescent cells, they can also create an immunosuppressive environment, inadvertently promoting cancer growth (11). Given the prevalence of cell senescence in the context of osteosarcoma, it is pivotal to identify senescence-related signatures with prognostic value for patients with osteosarcoma (12,13).
Long non-coding RNAs (lncRNAs), RNA sequences exceeding 200 nucleotides without protein-coding attributes, have attracted attention for their multifaceted biological roles (14). LncRNAs, particularly those exceeding 1,000 nucleotides, exhibit evolutionary conservation, highlighting their importance in diverse cellular processes. These activities range from regulating the cell cycle to immune responses and maintaining embryonic stem cell properties (15). Aberrant lncRNA expression is linked to a spectrum of diseases, including cancer (16,17). For instance, certain lncRNAs influence the dynamics of liver and breast cancers (18,19).
Rationale and knowledge gap
Despite recent scientific endeavors utilizing lncRNAs to establish diagnostic and prognostic models for numerous cancers, osteosarcoma remains relatively uncharted (20).
Objective
We aimed to address this issue by harnessing the potential of these unique RNA molecules to construct a predictive model, offering insights into clinical outcomes, navigating the genetic complexity of such patients, and contributing to the discovery of markers associated with prognosis, particularly those related to survival, in osteosarcoma.
In this study, we collected data from The Cancer Genome Atlas (TCGA) and Gene Expression Omnibus (GEO) databases, identified key cellular senescence-related long non-coding RNAs (CSR-lncRNAs) relevant for diagnosis, and constructed a CSR-lncRNA-risk signature that accurately discriminated patients with osteosarcoma from controls. Subsequently, we used CIBERSORT for the first time to analyze the differences in the immune microenvironment between patients with osteosarcoma and controls. Finally, we examined the correlation between the diagnostic markers and infiltrating immune cells to explore the molecular mechanisms involved in osteosarcoma development. We present this article in accordance with the TRIPOD reporting checklist (available at https://tcr.amegroups.com/article/view/10.21037/tcr-24-163/rc).
Methods
Data acquisition and extraction
From the UCSC Xena database (https://xena.ucsc.edu/), we acquired the expression profile data of the osteosarcoma GDC TARGET-osteosarcoma dataset, including HTSeq-Counts (n=88) and HTSeq-FPKM formats (n=88). All the samples in the TARGET-osteosarcoma dataset were derived from Homo sapiens. Subsequently, we collected the clinical details of patients with osteosarcoma. After filtering out incomplete clinical records, we included 84 patients with osteosarcoma, including 63 without metastasis and 21 with metastasis. We also assessed another gene dataset, GSE21257, from the GEO database (https://www.ncbi.nlm.nih.gov/geo/) and included all expression profile data samples from the dataset GSE21257 in subsequent analyses. This dataset included the records of 19 patients without metastases and 34 patients with metastases. We compiled a list of 3,597 CSR genes (CSRGs) from the GeneCards Database (https://www.genecards.org/) and articles from the Pubmed Database (https://pubmed.ncbi.nlm.nih.gov/). The study was conducted in accordance with the Declaration of Helsinki (as revised in 2013).
Identification of CSR-lncRNAs in osteosarcoma
Using the limma package, we identified genes with differential expression between metastatic and non-metastatic samples based on specific criteria [P<0.05, false discovery rate (FDR) <0.05, and |log2FC| >0]. By cross-referencing these genes to CSRGs, we identified the CSR differentially expressed genes (CSRDEGs).
We then applied Pearson’s correlation analysis using the Hmisc R package (21) to detect CSR-lncRNAs from CSRDEGs and lncRNAs with a cutoff value of |Coef| >0.3 and P<0.05.
Construction and validation of the prognostic signature for CSR-lncRNAs
We performed univariate Cox regression analysis to obtain potential prognostic CSR-lncRNAs with a threshold of R<0.05 in the R package survival. Furthermore, to screen promising osteosarcoma-associated lncRNAs, we used the randomForestSRC R package (22) to filter these CSR-lncRNAs with ntree =1,500 and nsplit =10 and obtained the important lncRNAs (relative importance >0.3 or importance >0.015). We then used multivariate Cox analysis (stepwise model) to determine which CSR-lncRNAs would be included in the model (P<0.05). The risk score was calculated as follows:
where coef (lncRNAn) represents the coefficient of the lncRNAs that correlated with survival and expr (lncRNAn) denotes lncRNA expression. Based on median scores, 84 patients in the TARGET-osteosarcoma dataset were divided into two groups, namely low- or high-risk.
To compare the overall survival between the high- and low-risk groups in the training, validation, and the entire sets, Kaplan-Meier survival analysis was performed using the R packages survminer and survival (23).
Receiver operating characteristic (ROC) curves were constructed and the area under the curve (AUC) was calculated. Additionally, we categorized the clinical data of patients with osteosarcoma to refine our lncRNA prognostic model.
Establishment and validation of the nomogram
We performed univariate and multivariate Cox regression analyses to determine the independence of risk score as a prognostic factor for osteosarcoma. We analyzed factors including age, sex, primary site, distant metastasis, and risk scores. To predict the 1-, 3-, and 5-year survival probabilities, we established a nomogram integrating the risk score and clinicopathological factors using the R package rms (24) and the nomogram formula (25). We then evaluated the nomogram model using ROC, calibration curve, and decision curve analysis (26).
Principal component and related functional enrichment analyses
We performed principal component analysis (PCA) (27) to assess the distribution of high- and low-risk patients based on the different datasets. To discern biological annotations and pathway differences between these risk groups, we conducted Geno Ontology (GO) and Kyoto Encyclopedia of Genes and Genome (KEGG) analyses of the differentially expressed genes (DEGs) using the clusterProfiler, enrichplot, and GOplot R packages (P<0.05).
Immune infiltration analysis
We employed the ESTIMATE method to gauge the tumor microenvironment characteristics of patients with osteosarcoma using the R package estimate (28). CIBERSORT algorithm provided insights into the immune cell composition of each patient with osteosarcoma. The sum of fractions of the 22 immune cell types in each sample was 1 (29). The single-sample gene set enrichment analysis (ssGSEA) method offers a perspective on the infiltration levels of 28 immune cell types using the R package gene set variation analysis (GSVA) (30,31). We also assessed the variations in immune checkpoint-related gene expression between the groups using R studio software.
Statistical analysis
Data manipulations and statistical evaluations were conducted using R software (version 4.3.0). We examined the mRNA-lncRNA relationships linked to cell senescence via Pearson’s correlation. We used Kaplan-Meier curves to visualize survival trends, as well as ROC analysis to evaluate the reliability of our prognostic risk scores. Immune-related evaluations were performed using the Wilcoxon test. All P values presented in this study were two-sided, and statistical significance was set at P≤0.05.
Results
Identification of CSR-lncRNAs
The methodology is illustrated in Figure 1. We sourced 88 osteosarcoma samples from the UCSC Xena database and 53 from the GEO database. From the GeneCards Database and previous publications in the PubMed Database, we identified 3,597 CSRGs (available online: https://cdn.amegroups.cn/static/public/tcr-24-163-1.pdf). Using the R package limma, we identified 738 and 4,387 DEGs by comparing non-metastatic and metastatic osteosarcoma samples (Figure 2A,2B) based on specific criteria (|log2FC| >0, FDR <0.05, and P<0.05). We then identified 94 common DEGs (Figure 2C) and 11 CSRGs (Figure 2D). Moreover, using heatmaps, we highlighted the differential expression of these 11 genes in the TARGET-osteosarcoma and GSE21257 datasets (Figure 2E,2F). We derived 1,262 CSR-lncRNAs through co-expression analysis using the R package Hmisc (available online: https://cdn.amegroups.cn/static/public/tcr-24-163-2.pdf). The results were visualized using a Sankey diagram (Figure 3).
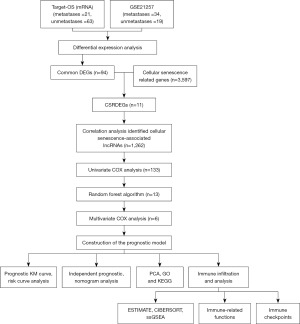
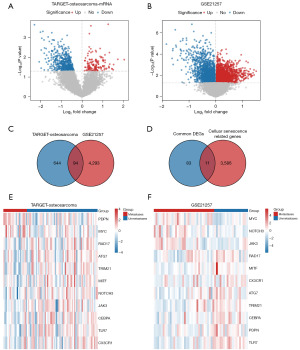
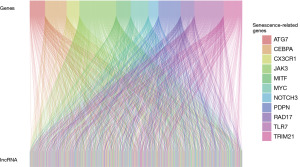
Construction of the CSR-lncRNA prognostic model
From the UCSC Xena database, we utilized 84 osteosarcoma samples (out of 88) with complete clinical data. Univariate Cox regression analysis revealed 131 lncRNAs significantly linked to osteosarcoma (available online: https://cdn.amegroups.cn/static/public/tcr-24-163-3.pdf); these lncRNAs were visualized using Cytoscape software (Figure 4A). In the interactive protein-protein interaction network, the node size corresponds to its degree, with larger and deeper colored nodes indicating higher values. To minimize overfitting, we employed the “randomForestSRC” package. As shown in Figure 4B, the out-of-bag (OOB) error rate stabilized with trees >500. We used the variable importance (VIMP) algorithm to determine the significance of variables (Figure 4C). Based on specific criteria (relative importance >0.3 or importance >0.015), we selected pivotal genes for the multivariate Cox regression model (Table S1). The optimal prognostic model comprised six lncRNAs, namely ELFN1-AS1, CYP2U1-AS1, AL162274.1, LINC02328, AP000785.1, and VPS9D1-AS1, which were deemed the best candidates for constructing a prognostic model (Table 1). Among the six CSR-lncRNAs, the risk factors of LINC02328 and VPS9D1-AS1 exhibited a hazard ratio (HR) less than 1, whereas the remaining lncRNAs had an HR exceeding 1. The risk scores were calculated using the following formula:
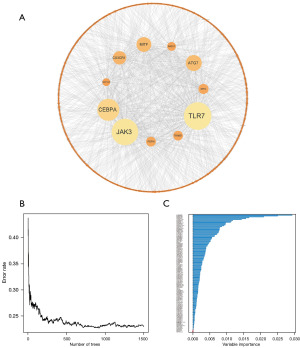
Table 1
CSR-lncRNA | coef | HR | HR.95L | HR.95H | P value |
---|---|---|---|---|---|
ELFN1-AS1 | 0.7672 | 2.153824 | 1.15378 | 4.020662 | 0.02 |
CYP2U1-AS1 | 3.0007 | 20.10054 | 1.30035 | 310.7099 | 0.03 |
AL162274.1 | 1.5786 | 4.848352 | 1.782752 | 13.18552 | 0.002 |
LINC02328 | −1.7503 | 0.173719 | 0.076516 | 0.394404 | <0.001 |
AP000785.1 | 3.4881 | 32.72345 | 5.1949 | 206.1298 | <0.001 |
VPS9D1-AS1 | −0.6993 | 0.496927 | 0.279817 | 0.882495 | 0.02 |
CSR-lncRNA, cellular senescence-related long non-coding RNA; coef, correlation coefficients; HR, hazard ratio; 95L, lower limit of 95% confidence interval; 95H, upper limit of 95% confidence interval.
Verification of the prognostic model
We divided the entire cohort into training and validation subsets for subgroup analysis. Across the three distinct cohorts, the low-risk group displayed superior survival outcomes (Figure S1A-S1C). Heatmaps depicted the expression of model CSR-lncRNAs for both risk groups (Figure S1D). Cross-validation confirmed the independence of the cohorts (Table 2).
Table 2
Variable | Type | Entire set | Training set | Validation set | χ2 | P value |
---|---|---|---|---|---|---|
Age (years) | >15 | 35 | 15 | 20 | 1.224 | 0.54 |
≤15 | 49 | 27 | 22 | |||
Sex | Female | 37 | 18 | 19 | 0.024 | 0.99 |
Male | 47 | 24 | 23 | |||
Translation | Metastatic | 21 | 11 | 10 | 0.063 | 0.97 |
Un-metastatic | 63 | 31 | 32 | |||
Primary site | Lower limb | 76 | 40 | 36 | 2.211 | 0.35 |
Upper limb | 8 | 2 | 6 |
We further stratified the clinical data to evaluate the prognostic model. Kaplan-Meier survival curves based on various factors, such as sex (male: P<0.001; female: P<0.001), age (age ≥15: P<0.001; age <15: P<0.001), metastasis (metastasis: P=0.005; non-metastasis: P=0.001), and tumor primary sites (legs: P<0.001; other: P=0.29), are presented in Figure S2.
Independent prognostic and nomogram analysis
We employed Cox regressions to assess the clinical characteristics of the entire cohort. Both uni-Cox (Figure 5A) and multi-Cox regressions (Figure 5B) revealed risk scores with hazard ratios (HRs) of 1.045 and 1.056, respectively. These significant findings (P<0.001) confirmed the accuracy and validity of the CSR-lncRNA prognostic model in predicting overall survival. Figure 5C further demonstrates that the risk score for the CSR-lncRNA prognostic signature had an area under the curve (AUC) of 0.893, surpassing that for age (AUC =0.456), sex (AUC =0.467), and primary site (AUC =0.462), and was similar to that metastasis (AUC =0.905). Subsequently, we generated a nomogram for the 1-, 3-, and 5-year overall survival based on this model (Figure 5D). Calibration curves (Figure 5E) indicated that the nomogram exhibited a reliable predictive performance. Using decision curve analysis, we compared the prognostic signatures of the CSR-lncRNAs to those of other clinicopathological features, revealing that this nomogram exhibited the highest risk threshold (Figure 5F).
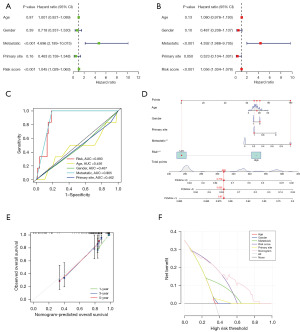
Principal component and enrichment analyses of risk subgroups
We performed three-dimensional (3D) PCA visualization based on the six lncRNAs in CSR-lncRNAs prognostic signatures (CSLPS) to highlight distinct distributions between the high- and low-risk groups (Figure 6A). The Scree Plot revealed six favorable factors in our risk signature, corresponding to the six CSR-lncRNAs used to construct the model (Figure 6B). Moreover, we employed GO and KEGG to investigate the potential biological functions in both risk groups. Figure 6C shows the top 10 enriched terms for biological processes (BPs), cellular components (CCs), and molecular functions (MFs) in the GO enrichment analysis. Notably, the primary enriched terms in BP were activation and regulation of the immune response, whereas vacuolar and lysosomal membranes were enriched in CC. Additionally, actin binding and actin filament binding were the enriched terms in MF. Figure 6D emphasizes the significance of specific pathways, such as the regulation of the actin cytoskeleton and PI3k-Akt signaling pathway, in both risk groups.
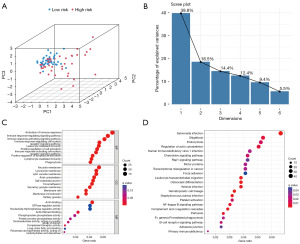
Immune-related multiomics analysis
To explore the correlation between our established CSR-lncRNA prognostic signature and the immune landscape, we first explored the differences in the tumor microenvironment between the high- and low-risk groups. ESTIMATE analysis indicated that the high-risk group exhibited lower stromal, immune, and overall ESTIMATE scores (Figure S3A-S3C), whereas the low-risk group exhibited higher tumor purity (Figure S3D). Correlation analysis conducted between various scores and the CSRDEG prognostic model risk score yielded significant outcomes, exhibiting that all correlation coefficients exceeding 0.3 with P<0.005 (Figure S3E-S3H). We then used CIBERSORT analysis to identify 22 types of immune cell infiltrations in the high- and low-risk groups; the results are displayed in a boxplot. As shown in Figure 7A, osteosarcoma samples tended to have a lower proportion of neutrophils, mast cells, naïve B cells, CD8 T cells, and natural killer cells, and a higher proportion of M0 macrophages, M2 macrophages, and naïve CD4 cells. Notably, the high-risk group exhibited a lower proportion of CD8 T (P<0.001) and regulatory T cells (P<0.05; Figure 7B). Additionally, ssGSEA revealed lower expression levels of 12 immune cell subtypes (activated B cells, activated CD8 T cells, natural killer T cells, and memory CD8 T cells) in the high-risk group than in the low-risk group (Figure 7C). These results suggest weaker immune infiltration in the high-risk group than in the low-risk group, especially for CD8 T cells and regulatory T cells.
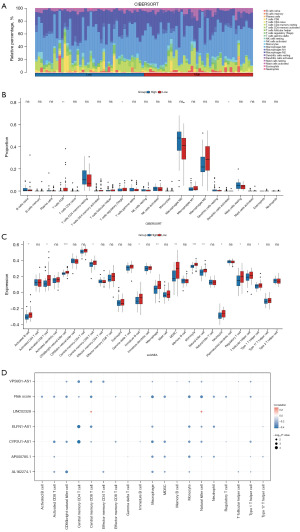
To further analyze the relationship between the model and immune signature, we performed a correlation analysis of the immune components, six modeling lncRNAs, and risk scores. The results (Figure 7D) indicated that most immune cells were negatively correlated with the model indices, especially CD8 T cells, macrophages, monocytes, natural killer cells, and myeloid-derived suppressor cells. Finally, we generated a heatmap, which revealed that among 13 immune-related functions, cytolytic activity, inflammation promotion, and T cell co-stimulation were notably different between the high- and low-risk groups, with the high-risk group displaying weaker activity (Figure 8A). Based on 79 immune checkpoint-related genes (32), we analyzed their expression levels in the two groups and revealed that CD40, D40LG, HAVCR2, HLA-A, HLA-DMA, HLA-DMB, HLA-DPB1, HLA-DQA1, HLA-DQB1, HLA-DRA, HLA-DRB1, HLA-E, LAG3, LGALS9, SIRPA, TDO2, HLA-F, and TNFRSF14 were highly expressed in the low-risk group compared to the high-risk group (Figure 8B). These findings indicate that patients with high-risk present with a inhibitory tumor immune microenvironment compared to patients with low-risk.
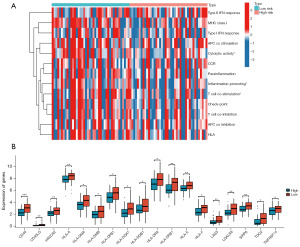
Discussion
Osteosarcoma, a primary malignancy of the bone characterized by its aggressive spread and metastasis (33), primarily affects individuals between the ages of 10–30 years, with a notable surge during the adolescent growth phase. Osteosarcoma frequently occurs in areas with significant bone growth, such as the knee and shoulder (6). Our study included 84 osteosarcoma cases and revealed an average age of 15 years with a significant number of cases localized near the knee. Cellular senescence, now recognized in the realm of cancer, plays a dual role by either inhibiting or promoting tumor growth (34). Recent research has focused on lncRNA-related risk signatures owing to their predictive capabilities (35-37). However, the prognostic signature domains linked to CSR-lncRNAs in osteosarcoma remain unclear. To the best of our knowledge, our study is the first to identify 1,262 differentially expressed CSR-lncRNAs using both differential expression and correlation methodologies, of which 133 were highlighted via univariate Cox regression analysis.
We constructed a prognostic model encompassing the six identified lncRNAs using a combination of the random forest method and multivariate Cox regression analysis. When tested on a training set using survival and ROC analyses, our mode exhibited a robust ability to predict the outcomes of patients with osteosarcoma. Remarkably, consistent outcomes were also observed when applying these same evaluations to the validation and complete datasets. Our subgroup survival analysis revealed shorter survival spans for patients in the high-risk categories across eight distinct clinical subgroups. Further univariate and multivariate analyses confirmed that the risk score derived from the 15 lncRNAs served as a solitary prognostic marker for patients with osteosarcoma. Based on these findings, we devised a nomogram that assigned risk scores to clinicopathological traits. Both the calibration curves and decision curve analysis indicated the elevated risk threshold of the nomogram, highlighting its precision in predicting the 1-, 3-, and 5-year survival probabilities for patients with osteosarcoma.
Our pioneering prognostic model encompassed six lncRNAs linked to cellular senescence, among which, CYP2U1-AS1, LINC02328, and AP000785.1 were highlighted in osteosarcoma for the first time. Our findings are consistent with those of earlier investigations, identifying ELFN1-AS1 (38) and AL162274.1 (39) as potential risk lncRNAs for patients with osteosarcoma. Conversely, our findings regarding the association between VPS9D1-AS1 and a better prognosis diverge from the existing literature (40). VPS9D1-AS1 has shown a promising ability to inhibit lymphoblastic leukemia cell growth, mediated by the miR-491-5p-miR-214-3p/GPX1 pathway, suggesting its potential therapeutic relevance for lymphoblastic leukemia (41). Moreover, the suggested involvement of LINC02328 in immune-centric PANoptosis in renal clear cell carcinoma is supported by RNA transcriptome insights, and single-cell sequencing has revealed its possible role in modulating T cell activity and directing cell death (42). ELFN1-AS1, which is known to inhibit natural killer (NK) cell activity in colorectal cancer cells, has emerged as a prospective therapeutic target for colorectal cancer (43). Notably, a significant proportion of these lncRNAs are associated with immune mechanisms. Therefore, we performed an extensive analysis of the differences in immune infiltration between the high- and low-risk groups and demonstrated that the high-risk group exhibited diminished StromalScore, ImmuneScore, and ESTIMATEScore, suggesting a unique tumor microenvironment compared to the low-risk group.
Macrophages are divided into M1 and M2 types, and the latter creates an environment to suppress the immune response and promote tumor growth (44). Our data indicate the pronounced presence of M2 macrophages, which may explain why patients with elevated risk scores often experience reduced overall survival, potentially due to the prominence of M2 macrophage infiltration (9). Using ssGSEA, we identified several immune cell types, such as activated B, activated CD8+ T, and CD56 bright natural killer cells, in the low-risk group. Our correlation analysis further revealed that most of these immune cells had an inverse relationship with the model lncRNAs and risk score. Specifically, activated CD8+ T cells, CD56 bright natural killer cells, central memory CD8+ T cells, macrophages, monocytes, and NK cells showed a negative association with most of the markers mentioned. These results suggest the tumors in the high-risk group exhibit lower levels of immune cell infiltration than their low-risk counterparts.
CD8+ T lymphocytes, as major effector cells (45), compete with tumor cells for essential nutrients, such as glucose (46), and target tumors by identifying specific antigenic peptides present on malignant cells via MHC-I molecules, subsequently releasing cytolytic granules containing perforin and granzymes (47). The significance of effector T cells in tumor protection is well established given their ability to proliferate clonally, exert cytotoxic effects, and the crucial role of long-lasting memory CD8+ T cells in maintaining long-term tumor immunity (48). NK cells, an innate lymphoid cell subset, are used to identify and neutralize virus-infected and tumorous cells (49). Their role in anticancer defense mechanisms is accentuated by their varied mechanisms of cytotoxicity and influence on the broader immune response via cytokine release. With the current trend, NK cells are emerging as promising candidates for immunotherapy, especially after demonstrating their potential in treating patients with advanced leukemia (50). Therefore, the scarcity of CD8+ T and NK cells in the high-risk group observed in the present study may be pivotal in influencing the osteosarcoma trajectory.
In this study, we focused on a new lncRNA, CYP2U1-AS1, which is linked to 10 immune cell types. Similarly, lncRNA AP000785.1 is associated with eight unique immune cells. The interplay between these two lncRNAs and the tumor immune response is yet to be elucidated, which will help to facilitate new investigative paths for the immunological aspects of osteosarcoma. To further elucidate the inhibitory immune microenvironment in in high-risk patients, we conducted a comprehensive analysis of the disparities in immunological function between high- and low-risk cohorts and demonstrated a noteworthy enhancement in immune cytolytic activity, immune inflammation promotion, and T cell co-stimulation in the low-risk osteosarcoma group. Researchers have unveiled multiple strategies for harnessing the functional capacity of NK cells in patients with leukemia or solid tumors to enhance their cytolytic activity against malignant cells (51). The co-stimulatory factors within T cell biology intricately determine the functional outcomes of T cell receptor signaling, thereby playing a pivotal role in orchestrating immunoreactivity (52). Recently, targeted immune checkpoint therapy has emerged as a novel approach for treating osteosarcoma. However, the overall response rate to immunotherapy within a broader population remains less than optimal (53). Our analysis highlighted increased gene expression of 18 immune checkpoints in patients with low risk, with CD40, CD40LG, and ALG3 being particularly prominent. Jiang et al. (54) reported that the pharmacological suppression of c-Myc in osteosarcoma promotes intricate crosstalk between antigen-presenting dendritic cells and T lymphocytes through the CD40/CD40L co-stimulatory pathway. Moreover, our multi-omics analysis, focused on immune-related factors, revealed the upregulation of diverse immunotherapeutic targets in low-risk osteosarcoma specimens compared to their metastatic counterparts, concurrently identifying hypoimmune infiltration in high-risk disease states. Deficiency in immune cell infiltration and diminished expression of immune checkpoints within high-risk tumor cohorts might highlight their resistance to immunotherapy and immune checkpoint inhibitors (ICIs). Therefore, strategies aimed at ameliorating the osteosarcoma microenvironment by increasing the infiltration of antitumor immune cells hold promise for enhancing the efficacy of these immunotherapeutic modalities (6).
Although various methodologies were used to refine our model, it was not without limitations. First, while internal validation encompassed the entire cohort, external validation was omitted. However, upon subsequent validation of the model via a third-party platform, statistically significant disparities emerged between the low- and high-risk factions. To a certain extent, this may be considered an external validation of the model. Moreover, pertinent empirical data substantiating the correlation between the six lncRNAs and osteosarcoma prognosis were absent. Nevertheless, half of the lncRNAs included in this study have been definitively implicated in osteosarcoma genesis. Hence, our prognostic model possesses moderate reliability. Thirdly, patients with low risk exhibit elevated gene expression of 18 immune checkpoints, with the majority of these genes being associated with MHC-II and only three pertaining to MHC-I (HLA-A, HLA-E and HLA-F). Given the critical influence of MHC class expression on NK and CD8+ T cell responses, careful consideration is necessary when assessing immune responses. Future studies must prioritize functional exploration of MHC class I and II genes to further understand immune system interactions. Ultimately, we acknowledge the need for clinical sample experiments to validate the reliability of the risk model and plan to incorporate these experiments in future research.
Conclusions
In this study, we developed a novel CSR-lncRNA prognostic signature that can accurately predict the prognosis of patients with osteosarcoma. The high-risk groups displayed lower immune infiltration, immune score estimates, and proportion of CD8+ T cells, implying less sensitivity to immunotherapy and ICIs. Our investigation has the following merits: first, we identified differentially expressed and prognosis-associated CSR-lncRNAs in osteosarcoma, providing important insights into subsequent exploration of the intricate role of lncRNAs in senescence. Second, we identified pronounced variations in the tumor immune microenvironment between the high- and low-risk cohorts. Notably, individuals in the high-risk group exhibit an environment characterized by hypoimmune infiltration, thus providing insights into the factors contributing to lower overall survival in this patient population. Further investigation into the mechanisms and pathways of CSR-lncRNAs in the current model is warranted to enhance understanding of their role in osteosarcoma.
Acknowledgments
We acknowledge The Cancer Genome Atlas (TCGA) and GEO databases for providing a platform and contributors for uploading their meaningful datasets. We would like to thank Editage (www.editage.cn) for English language editing.
Funding: This work was supported by
Footnote
Reporting Checklist: The authors have completed the TRIPOD reporting checklist. Available at https://tcr.amegroups.com/article/view/10.21037/tcr-24-163/rc
Peer Review File: Available at https://tcr.amegroups.com/article/view/10.21037/tcr-24-163/prf
Conflicts of Interest: All authors have completed the ICMJE uniform disclosure form (available at https://tcr.amegroups.com/article/view/10.21037/tcr-24-163/coif). The authors have no conflicts of interest to declare.
Ethical Statement: The authors are accountable for all aspects of the work in ensuring that questions related to the accuracy or integrity of any part of the work are appropriately investigated and resolved. The study was conducted in accordance with the Declaration of Helsinki (as revised in 2013).
Open Access Statement: This is an Open Access article distributed in accordance with the Creative Commons Attribution-NonCommercial-NoDerivs 4.0 International License (CC BY-NC-ND 4.0), which permits the non-commercial replication and distribution of the article with the strict proviso that no changes or edits are made and the original work is properly cited (including links to both the formal publication through the relevant DOI and the license). See: https://creativecommons.org/licenses/by-nc-nd/4.0/.
References
- Smeland S, Bielack SS, Whelan J, et al. Survival and prognosis with osteosarcoma: outcomes in more than 2000 patients in the EURAMOS-1 (European and American Osteosarcoma Study) cohort. Eur J Cancer 2019;109:36-50. [Crossref] [PubMed]
- Sadykova LR, Ntekim AI, Muyangwa-Semenova M, et al. Epidemiology and Risk Factors of Osteosarcoma. Cancer Invest 2020;38:259-69. [Crossref] [PubMed]
- Hashimoto K, Nishimura S, Hara Y, et al. Clinical outcomes of patients with primary malignant bone and soft tissue tumor aged 65 years or older. Exp Ther Med 2019;17:888-94. [PubMed]
- Hashimoto K, Nishimura S, Oka N, et al. Surgical management of sarcoma in adolescent and young adult patients. BMC Res Notes 2020;13:257. [Crossref] [PubMed]
- Cheng S, Wang H, Kang X, et al. Immunotherapy Innovations in the Fight against Osteosarcoma: Emerging Strategies and Promising Progress. Pharmaceutics 2024;16:251. [Crossref] [PubMed]
- Meltzer PS, Helman LJ. New Horizons in the Treatment of Osteosarcoma. N Engl J Med 2021;385:2066-76. [Crossref] [PubMed]
- Smrke A, Anderson PM, Gulia A, et al. Future Directions in the Treatment of Osteosarcoma. Cells 2021;10:172. [Crossref] [PubMed]
- Hayflick L, Moorhead PS. The serial cultivation of human diploid cell strains. Exp Cell Res 1961;25:585-621. [Crossref] [PubMed]
- Zeng C, Liu Y, He R, et al. Identification and validation of a novel cellular senescence-related lncRNA prognostic signature for predicting immunotherapy response in stomach adenocarcinoma. Front Genet 2022;13:935056. [Crossref] [PubMed]
- Reynolds LE, Maallin S, Haston S, et al. Effects of senescence on the tumour microenvironment and response to therapy. FEBS J 2024;291:2306-19. [Crossref] [PubMed]
- Campisi J. Aging, cellular senescence, and cancer. Annu Rev Physiol 2013;75:685-705. [Crossref] [PubMed]
- Lv Y, Wu L, Jian H, et al. Identification and characterization of aging/senescence-induced genes in osteosarcoma and predicting clinical prognosis. Front Immunol 2022;13:997765. [Crossref] [PubMed]
- Wang H, Liu H, Wang L, et al. Subtype Classification and Prognosis Signature Construction of Osteosarcoma Based on Cellular Senescence-Related Genes. J Oncol 2022;2022:4421952. [Crossref] [PubMed]
- Han S, Chen X, Huang L. The tumor therapeutic potential of long non-coding RNA delivery and targeting. Acta Pharm Sin B 2023;13:1371-82. [Crossref] [PubMed]
- Xu J, Bai J, Zhang X, et al. A comprehensive overview of lncRNA annotation resources. Brief Bioinform 2017;18:236-49. [PubMed]
- Coellar JD, Long J, Danesh FR. Long Noncoding RNAs and Their Therapeutic Promise in Diabetic Nephropathy. Nephron 2021;145:404-14. [Crossref] [PubMed]
- Ghafouri-Fard S, Hussen BM, Gharebaghi A, et al. LncRNA signature in colorectal cancer. Pathol Res Pract 2021;222:153432. [Crossref] [PubMed]
- Pei X, Wang X, Li H. LncRNA SNHG1 regulates the differentiation of Treg cells and affects the immune escape of breast cancer via regulating miR-448/IDO. Int J Biol Macromol 2018;118:24-30. [Crossref] [PubMed]
- Liang L, Huan L, Wang J, et al. LncRNA RP11-295G20.2 regulates hepatocellular carcinoma cell growth and autophagy by targeting PTEN to lysosomal degradation. Cell Discov 2021;7:118. [Crossref] [PubMed]
- Liao X, Wei R, Zhou J, et al. Emerging roles of long non-coding RNAs in osteosarcoma. Front Mol Biosci 2024;11:1327459. [Crossref] [PubMed]
- Luan F, Chen W, Chen M, et al. An autophagy-related long non-coding RNA signature for glioma. FEBS Open Bio 2019;9:653-67. [Crossref] [PubMed]
- Zhao P, Zhen H, Zhao H, et al. Identification of hub genes and potential molecular mechanisms related to radiotherapy sensitivity in rectal cancer based on multiple datasets. J Transl Med 2023;21:176. [Crossref] [PubMed]
- Zhao W, Liu M, Zhang M, et al. Effects of Inflammation on the Immune Microenvironment in Gastric Cancer. Front Oncol 2021;11:690298. [Crossref] [PubMed]
- Xu D, Wang Y, Liu X, et al. Development and clinical validation of a novel 9-gene prognostic model based on multi-omics in pancreatic adenocarcinoma. Pharmacol Res 2021;164:105370. [Crossref] [PubMed]
- Bi G, Li R, Liang J, et al. A nomogram with enhanced function facilitated by nomogramEx and nomogramFormula. Ann Transl Med 2020;8:78. [Crossref] [PubMed]
- Dilixiati N, Lian M, Hou Z, et al. Development of a Diagnostic Nomogram to Predict CAP in Hospitalized Patients with AECOPD. COPD 2023;20:224-32. [Crossref] [PubMed]
- Ringnér M. What is principal component analysis? Nat Biotechnol 2008;26:303-4. [Crossref] [PubMed]
- Yoshihara K, Shahmoradgoli M, Martínez E, et al. Inferring tumour purity and stromal and immune cell admixture from expression data. Nat Commun 2013;4:2612. [Crossref] [PubMed]
- Newman AM, Liu CL, Green MR, et al. Robust enumeration of cell subsets from tissue expression profiles. Nat Methods 2015;12:453-7. [Crossref] [PubMed]
- Bindea G, Mlecnik B, Tosolini M, et al. Spatiotemporal dynamics of intratumoral immune cells reveal the immune landscape in human cancer. Immunity 2013;39:782-95. [Crossref] [PubMed]
- Hänzelmann S, Castelo R, Guinney J. GSVA: gene set variation analysis for microarray and RNA-seq data. BMC Bioinformatics 2013;14:7. [Crossref] [PubMed]
- Sibilio P, Belardinilli F, Licursi V, et al. An integrative in-silico analysis discloses a novel molecular subset of colorectal cancer possibly eligible for immune checkpoint immunotherapy. Biol Direct 2022;17:10. [Crossref] [PubMed]
- Chen C, Xie L, Ren T, et al. Immunotherapy for osteosarcoma: Fundamental mechanism, rationale, and recent breakthroughs. Cancer Lett 2021;500:1-10. [Crossref] [PubMed]
- Hanahan D. Hallmarks of Cancer: New Dimensions. Cancer Discov 2022;12:31-46. [Crossref] [PubMed]
- Wang Q. Five cuprotosis-related lncRNA signatures for prognosis prediction in acute myeloid leukaemia. Hematology 2023;28:2231737. [Crossref] [PubMed]
- Thandar M, Zhu Y, Zhang X, et al. Construction and validation of stemness-related lncRNA pair signature for predicting prognosis in colorectal cancer. J Cancer Res Clin Oncol 2023;149:11815-28. [Crossref] [PubMed]
- Ma Y, Sun Y, Zhao X, et al. Identification of m(5)C-related lncRNAs signature to predict prognosis and therapeutic responses in esophageal squamous cell carcinoma patients. Sci Rep 2023;13:14499. [Crossref] [PubMed]
- He Y, Zhou H, Xu H, et al. Construction of an Immune-Related lncRNA Signature That Predicts Prognosis and Immune Microenvironment in Osteosarcoma Patients. Front Oncol 2022;12:769202. [Crossref] [PubMed]
- Zhang Y, He R, Lei X, et al. Comprehensive Analysis of a Ferroptosis-Related lncRNA Signature for Predicting Prognosis and Immune Landscape in Osteosarcoma. Front Oncol 2022;12:880459. [Crossref] [PubMed]
- Wei J, Fang DL, Huang CK, et al. Screening a novel signature and predicting the immune landscape of metastatic osteosarcoma in children via immune-related lncRNAs. Transl Pediatr 2021;10:1851-66. [Crossref] [PubMed]
- Xiao S, Xu N, Ding Q, et al. LncRNA VPS9D1-AS1 promotes cell proliferation in acute lymphoblastic leukemia through modulating GPX1 expression by miR-491-5p and miR-214-3p evasion. Biosci Rep 2020;40:BSR20193461. [Crossref] [PubMed]
- Liu W, Qu C, Wang X. Comprehensive analysis of the role of immune-related PANoptosis lncRNA model in renal clear cell carcinoma based on RNA transcriptome and single-cell sequencing. Oncol Res 2023;31:543-67. [Crossref] [PubMed]
- Han B, He J, Chen Q, et al. ELFN1-AS1 promotes GDF15-mediated immune escape of colorectal cancer from NK cells by facilitating GCN5 and SND1 association. Discov Oncol 2023;14:56. [Crossref] [PubMed]
- Pan Y, Yu Y, Wang X, et al. Tumor-Associated Macrophages in Tumor Immunity. Front Immunol 2020;11:583084. [Crossref] [PubMed]
- Mami-Chouaib F, Blanc C, Corgnac S, et al. Resident memory T cells, critical components in tumor immunology. J Immunother Cancer 2018;6:87. [Crossref] [PubMed]
- Chang CH, Qiu J, O'Sullivan D, et al. Metabolic Competition in the Tumor Microenvironment Is a Driver of Cancer Progression. Cell 2015;162:1229-41. [Crossref] [PubMed]
- Corgnac S, Boutet M, Kfoury M, et al. The Emerging Role of CD8(+) Tissue Resident Memory T (T(RM)) Cells in Antitumor Immunity: A Unique Functional Contribution of the CD103 Integrin. Front Immunol 2018;9:1904. [Crossref] [PubMed]
- Han J, Khatwani N, Searles TG, et al. Memory CD8(+) T cell responses to cancer. Semin Immunol 2020;49:101435. [Crossref] [PubMed]
- Laskowski TJ, Biederstädt A, Rezvani K. Natural killer cells in antitumour adoptive cell immunotherapy. Nat Rev Cancer 2022;22:557-75. [Crossref] [PubMed]
- Bakhtiyaridovvombaygi M, Yazdanparast S, Mikanik F, et al. Cytokine-Induced Memory-Like NK Cells: Emerging strategy for AML immunotherapy. Biomed Pharmacother 2023;168:115718. [Crossref] [PubMed]
- Sivori S, Pende D, Quatrini L, et al. NK cells and ILCs in tumor immunotherapy. Mol Aspects Med 2021;80:100870. [Crossref] [PubMed]
- Chen L, Flies DB. Molecular mechanisms of T cell co-stimulation and co-inhibition. Nat Rev Immunol 2013;13:227-42. [Crossref] [PubMed]
- Panez-Toro I, Muñoz-García J, Vargas-Franco JW, et al. Advances in Osteosarcoma. Curr Osteoporos Rep 2023;21:330-43. [Crossref] [PubMed]
- Jiang K, Zhang Q, Fan Y, et al. MYC inhibition reprograms tumor immune microenvironment by recruiting T lymphocytes and activating the CD40/CD40L system in osteosarcoma. Cell Death Discov 2022;8:117. [Crossref] [PubMed]