Expression profiles of serum transfer RNA-derived fragments and their potential roles in non-small cell lung cancer
Highlight box
Key findings
• tRF-31-87R8WP9N1EWJ0 and tRF-31-79MP9P9NH57SD might be the potential biological markers for non-small cell lung cancer (NSCLC).
What is known and what is new?
• Transfer RNA-derived fragments (tRFs) are a new class of small non-coding RNAs. tRFs participate in some pathological processes of cancer.
• This is the first study to identify differentially expressed serum tRFs in NSCLC.
What is the implication, and what should change now?
• The role of tRF-31-87R8WP9N1EWJ0 and tRF-31-79MP9P9NH57SD in NSCLC needs further study.
Introduction
Lung cancer is recognized as a frequent type of cancer in human. The mortality of lung cancer continually occupies first place among all malignancies in China. Non-small cell lung cancer (NSCLC) causes more than 80% of lung cancer incidences (1). Over the past 20 years, a never-ending series of treatments for NSCLC has largely been used to decrease the number of deaths and enhance the well-being of patients. However, the five-year surviving chance of patients remains only 15%, partly because most patients have advanced-stage lung cancer at initial diagnosis (2-4). Therefore, it is critical to explore novel biomarkers and effective molecular targets in NSCLC.
Small RNAs (tsRNAs) formed from transfer RNA (tRNA) is a unique type of small non-coding RNAs (sncRNAs) discovered lately as a consequence of developments in high-throughput sequencing and bioinformatic research (5,6). According to their catalytic sites of tRNA and length, tsRNAs can be divided into two subgroups: tRNA-derived fragments (tRFs) and tRNA halves (tiRNAs) with lengths of 14–36 and 30–40 nt, respectively (7-9). Although the investigation of tsRNA biology is still in its infancy, emerging evidence has revealed the diverse functional roles of tsRNAs, such as regulating post-transcriptional gene silencing (10), promoting translation by accelerating ribosome biogenesis (11), and modulating noncoding RNA biogenesis and global chromatin organization (12).
Growing evidence evinces the participation of tsRNAs in cell proliferation, epigenetic regulation, gene silencing, and various human diseases (13,14). For example, hypoxic microenvironment-induced half-tRNA 5’tiRNA-His-GTG encourages the gene expression that favors the rapid growth of cancerous cells by attacking the large tumor suppressor kinase 2 (LATS2) (15). Han et al. reported that tRF3008A expression was significantly reduced in colorectal cancer (CRC) and tRF3008A could suppress the proliferation and migration of CRC through destabilization of FOXK1 in an AGO-dependent way (16). tRF-Leu-CAG is increased in cell lines and tissues of NSCLC, which promotes cell proliferation and the cell cycle (17). In addition, Huang et al. report the landscape of tRF and tiRNA expression profiles in lung adenocarcinoma (LUAD) tissues and found that downregulated tRF-1s may be involved in the pathogenesis of LUAD (18). The tsRNAs expression in serum from NSCLC patients, on the other hand, is yet to be identified. RNA-sequencing and quantitative reverse transcription polymerase chain reaction (qRT-PCR) verification were utilized for evaluating the expression profiles of tRFs/tiRNAs in NSCLC serum samples in this investigation. We next established a tRF-mRNA network utilizing bioinformatic analysis to locate functioning tRFs. These discoveries could lead to obtaining clues for possible biological markers and therapeutic targets for NSCLC. We present this article in accordance with the REMARK reporting checklist (available at https://tcr.amegroups.com/article/view/10.21037/tcr-23-2364/rc).
Methods
Patients and samples
In the retrospective study, the clinical serum samples for this investigation were gathered from patients at The Affiliated People’s Hospital of Ningbo University between 2020 and 2022. The tRFs/tiRNAs sequencing group included three serum samples of NSCLC and three healthy controls. The validation group included 20 serum samples of NSCLC patients before surgery and serum samples from 20 healthy controls. Inclusion criteria for patients enrolled were as follows: (I) older than 18 years of age, (II) confirmation of disease by histopathology, (III) no receipt of chemotherapy and radiography. The exclusion criteria for patients were: (I) concurrent pregnancy or breast-feeding, (II) diagnosis of other cancers or serious diseases. All serum samples were centrifuged immediately after collection and the supernatant was kept at −80 ℃ before its usage. This study was conducted in accordance with the Declaration of Helsinki (as revised in 2013). This investigation was approved by The Affiliated People’s Hospital of Ningbo University’s Ethical Committee (approval ID. 2017040). All the recruited subjects signed the informed consent voluntarily. Clinical information of the samples is described in Table S1.
RNA preparation and quantitative PCR
Total RNA was separated from serum using the TRIzolTM LS Reagent (Invitrogen, CA, USA) as per the directions provided by the manufacturer. To reverse transcribe total RNA, the ImProm-II Reverse Transcription System (Promega, WI, USA) was used as per the instructions of manufacturer. Based on the reported literatures (19,20), stem-loop quantitative PCR method was used for quantification of mature tRFs, and for the quantification of tRFs, miR-16 was chosen as internal control. The 2−∆∆Ct technique was utilized to determine the relative expression levels. Table S2 lists the primers used for quantitative PCR.
Library preparation and sequencing
Total RNA was first treated with the rtStarTMtRF and tiRNA Pretreatment Kit (KangChen Bio-tech, Shanghai, China) to remove RNA modifications (such as, deacylation, phosphorylation, or demethylation). The entire RNA from all samples was then ligated to 3' and 5' small RNA adapters sequentially. Illumina’s proprietary RT primers and amplification primers were used to synthesize and amplify cDNA. Following that, the PAGE gel was used to extract and purify ~134–160 bp PCR generated fragments. Finally, the Agilent 2100 Bioanalyzer was used to quantify the finished libraries. The abundance of tRF, tiRNA, and miRNA was measured and was normalized as counts per million of total aligned reads (CPM).
Bioinformatic analysis of tRFs/tiRNAs
We used TargetScan and miRanda algorithms to predict gene targets of tRF-31-87R8WP9N1EWJ0 and tRF-31-79MP9P9NH57SD. The Gene Ontology (GO) and Kyoto Encyclopedia of Genes and Genomes (KEGG) pathway enrichment analysis were carried out by utilizing DAVID database (http://david.ncifcrf.gov/). Cytoscape 3.7.2 was used to graph the data.
Statistical analysis
Statistical analysis was performed by availing GraphPad Prism 7.0 (GraphPad, Inc., CA, USA). The two-tail unpaired Student t-test was performed to examine the variations among the groups. The receiver operating characteristic (ROC) curve studies were performed using MedCalc 11.0 (MedCalc, Ostend, Belgium). In these studies, the significance level was set as P<0.05.
Results
General features of tRFs/tiRNAs expression in NSCLC serum
To study the expression of tsRNAs in NSCLC, we conducted tRFs/tiRNAs sequencing on serum samples from three NSCLC patients and three healthy controls. Only high-quality reads with 16–40 nt insertion were recorded after the raw sequencing data were processed by eliminating both 5' and 3' adaptors. As demonstrated in Figure 1A,1B, tRF-5 was the most abundant tRNAs in both NSCLC patients and healthy controls. The majority of tRNAs in NSCLC were 22 and 32 nt in length, according to the length distribution of tsRNAs, while healthy controls were mainly 20 nt in length (Figure 1C,1D).
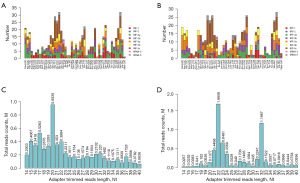
Differentially expressed tRFs/tiRNAs between NSCLC and healthy groups
The commonly and specifically expressed tRFs/tiRNAs are depicted in a Venn diagram (Figure 2A). The CPM values that were higher than 20 in both groups were represented by the commonly expressed tRFs/tiRNAs, and the CPM values that were more than 20 in one group but less than 20 in the other group were represented by the particularly expressed tRFs/tiRNAs. In total, we discovered 197 upregulated tRFs/tiRNAs and 296 downregulated tRFs/tiRNAs. The heatmap depicts hierarchical clustering of tRFs/tiRNAs that are significantly differentially expressed (Figure 2B). In the volcano plots, we show the entire alterations in tRFs/tiRNAs expression among the 2 groups (Figure 2C). There were 11 up-regulated and 18 down-regulated tRFs/tiRNAs after restricting the condition to fold change ≥2.0 and P<0.05 (Table S3).
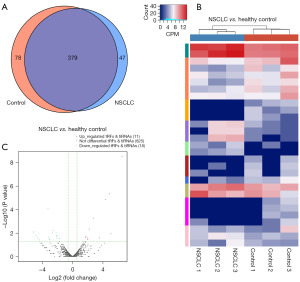
tRF-31-87R8WP9N1EWJ0 and tRF-31-79MP9P9NH57SD were upregulated in NSCLC serum
To determine the tRFs/tiRNAs’ potential for the biological marker, we performed qRT-PCR to detect the top five upregulated tRFs/tiRNAs in 20 paired healthy controls and NSCLC samples. As shown in Figure 3A,3B, the NSCLC patients exhibited significantly higher levels of tRF-31-87R8WP9N1EWJ0 and tRF-31-79MP9P9NH57SD in the serum samples compared with healthy controls. However, no discernible differentiation in tRF-29-1OR6QOH04MI9, tRF-30-1OR6QOH04MR1 and tRF-28-1OR6QOH04MDY expression between NSCLC patients and healthy controls was noted (Figure 3C-3E). Then, the ROC curve was constructed to determine the potential diagnostic power of tRF-31-87R8WP9N1EWJ0 and tRF-31-79MP9P9NH57SD.As shown in Figure 3F, the area under the ROC curve (AUC) of tRF-31-87R8WP9N1EWJ0 was 0.773 (95% confidence interval: 0.613–0.890), with 70% sensitivity and 80% specificity, the AUC of tRF-31-79MP9P9NH57SD was 0.707 (95% confidence interval: 0.543–0.840), with 80% sensitivity and 65% specificity. These results demonstrated that serum tRF-31-87R8WP9N1EWJ0 and tRF-31-79MP9P9NH57SD might be utilized as new biomarkers for diagnosing NSCLC. To further establish the diagnostic utility of tRFs, more individual patient samples are required.
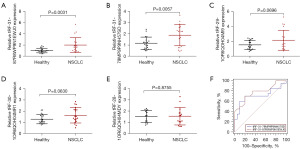
Target gene prediction with bioinformatic tool
TargetScan and miRanda online tools were availed to study the putative target genes of tRF-31-87R8WP9N1EWJ0 and tRF-31-79MP9P9NH57SD. As shown in Figure 4A, we predicted 3218 targets and 1572 targets, respectively. Overlapped between tRF-31-87R8WP9N1EWJ0 and tRF-31-79MP9P9NH57SD were 307 potential target genes. In the network diagrams created by Cytoscape, the target genes were displayed (Figure 4B).
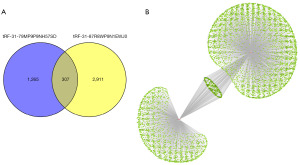
GO/KEGG enrichment analysis
For target genes of tRF-31-87R8WP9N1EWJ0 and tRF-31-79MP9P9NH57SD, we used GO and KEGG enrichment analysis. GO functional enrichment analysis hinted that tRF-31-87R8WP9N1EWJ0 might have a role in regulation of biological quality, organic substance transport, and nervous system development (Figure 5A), Whereas, the most enriched terms of target genes of tRF-31-79MP9P9NH57SD were “Regulation of nucleobase-containing compound metabolic process” (Figure 5B). Next, enrichment analysis of the KEGG signaling pathway indicated that tRF-31-87R8WP9N1EWJ0 might participate in Ras signaling pathway and Endocytosis (Figure 5C). While, the most enriched pathways of tRF-31-79MP9P9NH57SD were Herpes simplex virus 1 infection, Proteoglycans in cancer and mTOR signaling pathway (Figure 5D).
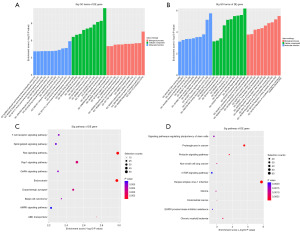
Discussion
Despite the fact that the clinical effect of NSCLC has been significantly enhanced during the previous few decades, its incidence and malignancy remain elevated, and its 5-year estimation of life is lesser than that of other cancerous tumors, owing to the deficiency of a valid and useful diagnostic tool and targeted therapies (3,21). As a result, finding novel and accurate biomarkers for correctly diagnosing NSCLC and clinical decision-making is a top priority.
Recent studies have found that tsRNAs are dysregulated in various types of cancer (22-24). Chen et al. reported that tRF-phe-GAA-031 and tRF-VAL-TCA-002 were upregulated and linked with distant metastasis and in CRC (25). The bi-sesncRNA signature (composing of exosomal tRNA-GlyGCC-5 and sRESE) could be a potential biomarker for esophageal squamous cell carcinoma (26). However, the exploration of the potentials of tsRNAs is at an early stage (13,24,27). tRFs/tiRNAs sequencing was carried out in three pairs of serum samples from NSCLC patients and healthy controls in this investigation. In addition, 11 up-regulated and 18 down-regulated tsRNAs were effectively identified. By increasing the quantity of NSCLC serum samples to carry out the stem-loop qRT-PCR analysis, we found that tRF-31-87R8WP9N1EWJ0 and tRF-31-79MP9P9NH57SD exhibited significantly higher levels in NSCLC serum samples compared with healthy controls. The positive results suggest that tRF-31-87R8WP9N1EWJ0 and tRF-31-79MP9P9NH57SD may be new potential biomarkers for NSCLC. Tissue or blood expression level in larger samples of NSCLC patients with different stages and healthy people could further help determine the diagnostic and prognostic value of the two tRFs.
It has been recognized that by silencing of target genes, controlling transcription and modifying mRNA stability, tsRNAs can influence cancer development (28,29). Xu et al. reported that tRF-Glu-TTC-027 was downregulated and repressed the cancerous progression via MAPK pathway in gastric carcinoma (30). Parallel outcomes were observed that tRF-5026a could be a new tumor-suppressor through the PTEN/PI3K/AKT signaling pathway in gastric cancer (31). Recently, Wang et al. reported that tRF-3024b could hijack miR-192-5p to increase BCL-2-mediated resistance to cytotoxic T lymphocytes in Esophageal Squamous Cell Carcinoma (32). In lung cancer, AS-tDR-007333 was found to promote the malignancy of NSCLC via the HSPB1/MED29 and ELK4/MED29 axes (33). We used KEGG pathway analysis to see if tRF-31-87R8WP9N1EWJ0 and tRF-31-79MP9P9NH57SD are responsible for the manifestation of NSCLC. The Ras signaling route, the Rap1 signaling pathway, the AMPK signaling pathway, and the mTOR signaling pathway are among the top 10 enriched pathways, and have been linked to carcinogenesis in prior studies (34-36). Our findings showed that tRF-31-87R8WP9N1EWJ0 and tRF-31-79MP9P9NH57SD may be implicated in the pathogenesis of NSCLC, but protein functional experiments are needed to confirm these theories.
Conclusions
To conclude, we determined the expression profiles of tRFs/tiRNAs in NSCLC serum samples and found that serum levels of tRF-31-87R8WP9N1EWJ0 and tRF-31-79MP9P9NH57SD were dramatically increased in NSCLC patients when compared to the healthy controls, suggesting that they may be novel diagnostic biomarkers for NSCLC. In addition, bioinformatic analysis found that tRF-31-87R8WP9N1EWJ0 and tRF-31-79MP9P9NH57SD might have a part in the pathological development of NSCLC, which lays a theoretical foundation for our further study.
Acknowledgments
Funding: The current study was carried out with the support of
Footnote
Reporting Checklist: The authors have completed the REMARK reporting checklist. Available at https://tcr.amegroups.com/article/view/10.21037/tcr-23-2364/rc
Data Sharing Statement: Available at https://tcr.amegroups.com/article/view/10.21037/tcr-23-2364/dss
Peer Review File: Available at https://tcr.amegroups.com/article/view/10.21037/tcr-23-2364/prf
Conflicts of Interest: Both authors have completed the ICMJE uniform disclosure form (available at https://tcr.amegroups.com/article/view/10.21037/tcr-23-2364/coif). The authors have no conflicts of interest to declare.
Ethical Statement: The authors are accountable for all aspects of the work in ensuring that questions related to the accuracy or integrity of any part of the work are appropriately investigated and resolved. The study was conducted in accordance with the Declaration of Helsinki (as revised in 2013). The study was approved by the Ethical Committee of The Affiliated People’s Hospital of Ningbo University (No. 2017040). Written informed consent was obtained from all enrolled patients.
Open Access Statement: This is an Open Access article distributed in accordance with the Creative Commons Attribution-NonCommercial-NoDerivs 4.0 International License (CC BY-NC-ND 4.0), which permits the non-commercial replication and distribution of the article with the strict proviso that no changes or edits are made and the original work is properly cited (including links to both the formal publication through the relevant DOI and the license). See: https://creativecommons.org/licenses/by-nc-nd/4.0/.
References
- Siegel RL, Miller KD, Jemal A. Cancer statistics, 2020. CA Cancer J Clin 2020;70:7-30. [Crossref] [PubMed]
- Krzyżanowska N, Wojas-Krawczyk K, Milanowski J, et al. Future Prospects of Immunotherapy in Non-Small-Cell Lung Cancer Patients: Is There Hope in Other Immune Checkpoints Targeting Molecules? Int J Mol Sci 2022;23:3087. [Crossref] [PubMed]
- Mithoowani H, Febbraro M. Non-Small-Cell Lung Cancer in 2022: A Review for General Practitioners in Oncology. Curr Oncol 2022;29:1828-39. [Crossref] [PubMed]
- Ma LR, Li JX, Tang L, et al. Immune checkpoints and immunotherapy in non-small cell lung cancer: Novel study progression, challenges and solutions. Oncol Lett 2021;22:787. [Crossref] [PubMed]
- Yang N, Li R, Liu R, et al. The Emerging Function and Promise of tRNA-Derived Small RNAs in Cancer. J Cancer 2024;15:1642-56. [Crossref] [PubMed]
- Zuo Y, Zhu L, Guo Z, et al. tsRBase: a comprehensive database for expression and function of tsRNAs in multiple species. Nucleic Acids Res 2021;49:D1038-45. [Crossref] [PubMed]
- Salehi M, Kamali MJ, Rajabzadeh A, et al. tRNA-derived fragments: Key determinants of cancer metastasis with emerging therapeutic and diagnostic potentials. Arch Biochem Biophys 2024;753:109930. [Crossref] [PubMed]
- Cabrelle C, Giorgi FM, Mercatelli D. Quantitative and qualitative detection of tRNAs, tRNA halves and tRFs in human cancer samples: Molecular grounds for biomarker development and clinical perspectives. Gene 2024;898:148097. [Crossref] [PubMed]
- Wang JH, Chen WX, Mei SQ, et al. tsRFun: a comprehensive platform for decoding human tsRNA expression, functions and prognostic value by high-throughput small RNA-Seq and CLIP-Seq data. Nucleic Acids Res 2022;50:D421-31. [Crossref] [PubMed]
- Wang JZ, Zhu H, You P, et al. Upregulated YB-1 protein promotes glioblastoma growth through a YB-1/CCT4/mLST8/mTOR pathway. J Clin Invest 2022;132:e146536. [Crossref] [PubMed]
- Liu Z, Kim HK, Xu J, et al. The 3'tsRNAs are aminoacylated: Implications for their biogenesis. PLoS Genet 2021;17:e1009675. [Crossref] [PubMed]
- Boskovic A, Bing XY, Kaymak E, Rando OJ. Control of noncoding RNA production and histone levels by a 5' tRNA fragment. Genes Dev 2020;34:118-31.Correction appears in Genes Dev 2020;34:462.
- Zong T, Yang Y, Zhao H, et al. tsRNAs: Novel small molecules from cell function and regulatory mechanism to therapeutic targets. Cell Prolif 2021;54:e12977. [Crossref] [PubMed]
- Kim HK, Yeom JH, Kay MA. Transfer RNA-Derived Small RNAs: Another Layer of Gene Regulation and Novel Targets for Disease Therapeutics. Mol Ther 2020;28:2340-57. [Crossref] [PubMed]
- Tao EW, Wang HL, Cheng WY, et al. A specific tRNA half, 5'tiRNA-His-GTG, responds to hypoxia via the HIF1α/ANG axis and promotes colorectal cancer progression by regulating LATS2. J Exp Clin Cancer Res 2021;40:67. [Crossref] [PubMed]
- Han Y, Peng Y, Liu S, et al. tRF3008A suppresses the progression and metastasis of colorectal cancer by destabilizing FOXK1 in an AGO-dependent manner. J Exp Clin Cancer Res 2022;41:32. [Crossref] [PubMed]
- Shao Y, Sun Q, Liu X, et al. tRF-Leu-CAG promotes cell proliferation and cell cycle in non-small cell lung cancer. Chem Biol Drug Des 2017;90:730-8. [Crossref] [PubMed]
- Huang LT, Cui M, Silva M, et al. Expression profiles of tRNA-derived fragments and their potential roles in lung adenocarcinoma. Ann Transl Med 2022;10:196. [Crossref] [PubMed]
- Zhu L, Li J, Gong Y, et al. Exosomal tRNA-derived small RNA as a promising biomarker for cancer diagnosis. Mol Cancer 2019;18:74. [Crossref] [PubMed]
- Yang LH, Wang SL, Tang LL, et al. Universal stem-loop primer method for screening and quantification of microRNA. PLoS One 2014;9:e115293. [Crossref] [PubMed]
- Huang Y, Qin S, Gu X, et al. Comprehensive Assessment of Serum hsa_circ_0070354 as a Novel Diagnostic and Predictive Biomarker in Non-small Cell Lung Cancer. Front Genet 2022;12:796776. [Crossref] [PubMed]
- Sun X, Yang J, Yu M, et al. Global identification and characterization of tRNA-derived RNA fragment landscapes across human cancers. NAR Cancer 2020;2:zcaa031. [Crossref] [PubMed]
- Shen Y, Xie Y, Yu X, et al. Clinical diagnostic values of transfer RNA-derived fragment tRF-19-3L7L73JD and its effects on the growth of gastric cancer cells. J Cancer 2021;12:3230-8. [Crossref] [PubMed]
- Jia Y, Tan W, Zhou Y. Transfer RNA-derived small RNAs: potential applications as novel biomarkers for disease diagnosis and prognosis. Ann Transl Med 2020;8:1092. [Crossref] [PubMed]
- Chen H, Xu Z, Cai H, et al. Identifying Differentially Expressed tRNA-Derived Small Fragments as a Biomarker for the Progression and Metastasis of Colorectal Cancer. Dis Markers 2022;2022:2646173. [Crossref] [PubMed]
- Li K, Lin Y, Luo Y, et al. A signature of saliva-derived exosomal small RNAs as predicting biomarker for esophageal carcinoma: a multicenter prospective study. Mol Cancer 2022;21:21. [Crossref] [PubMed]
- Yu M, Lu B, Zhang J, et al. tRNA-derived RNA fragments in cancer: current status and future perspectives. J Hematol Oncol 2020;13:121. [Crossref] [PubMed]
- Fu M, Gu J, Wang M, et al. Emerging roles of tRNA-derived fragments in cancer. Mol Cancer 2023;22:30. [Crossref] [PubMed]
- Gu X, Zhang Y, Qin X, et al. Transfer RNA-derived small RNA: an emerging small non-coding RNA with key roles in cancer. Exp Hematol Oncol 2022;11:35. [Crossref] [PubMed]
- Xu W, Zhou B, Wang J, et al. tRNA-Derived Fragment tRF-Glu-TTC-027 Regulates the Progression of Gastric Carcinoma via MAPK Signaling Pathway. Front Oncol 2021;11:733763. [Crossref] [PubMed]
- Zhu L, Li Z, Yu X, et al. The tRNA-derived fragment 5026a inhibits the proliferation of gastric cancer cells by regulating the PTEN/PI3K/AKT signaling pathway. Stem Cell Res Ther 2021;12:418. [Crossref] [PubMed]
- Wang L, Peng B, Yan Y, et al. The tRF-3024b hijacks miR-192-5p to increase BCL-2-mediated resistance to cytotoxic T lymphocytes in Esophageal Squamous Cell Carcinoma. Int Immunopharmacol 2024;126:111135. [Crossref] [PubMed]
- Yang W, Gao K, Qian Y, et al. A novel tRNA-derived fragment AS-tDR-007333 promotes the malignancy of NSCLC via the HSPB1/MED29 and ELK4/MED29 axes. J Hematol Oncol 2022;15:53. [Crossref] [PubMed]
- S M N Mydin RB. Regulatory role of miRNAs in nasopharyngeal cancer involving PTEN/PI3K/AKT, TGFβ/SMAD, RAS/MAPK, Wnt/β-catenin and pRB-E2F signaling pathways: A review. Cell Biochem Funct 2024;42:e3945. [Crossref] [PubMed]
- Liao H, Wang Y, Zou L, et al. Relationship of mTORC1 and ferroptosis in tumors. Discov Oncol 2024;15:107. [Crossref] [PubMed]
- Khattar E, Tergaonkar V. Role of Rap1 in DNA damage response: implications in stem cell homeostasis and cancer. Exp Hematol 2020;90:12-7. [Crossref] [PubMed]