Development of a novel colon adenocarcinoma m6A-related lncRNA pair prognostic model
Highlight box
Key findings
• Our study identified a brand-new N6-methyladenosine (m6A)-related long noncoding ribonucleic acid (lncRNA) pair predictive model for colon adenocarcinoma (COAD).
What is known and what is new?
• The involvement of lncRNA and m6A modification in cancer are significant, they exhibit a close association.
• This is the first study of COAD the m6A-related lncRNA pair prognostic model.
What is the implication, and what should change now?
• Since the data used in this study came from The Cancer Genome Atlas, more research and access to other publicly accessible databases are necessary. Additional experimental confirmation is needed.
Introduction
Colorectal cancer (CRC) ranks as the third most prevalent cancer and is responsible for the second highest number of cancer-related fatalities globally (1). CRC consists of two primary types: rectal adenocarcinoma (READ) and colon adenocarcinoma (COAD). The majority of CRC cases are COADs, accounting for 80–90% of all pathological subtypes (2,3). Despite the fact that screening techniques are meant to increase early CRC identification, 25% of patients are already in an advanced state at the initial diagnosis (4). A higher cancer stage is connected with a higher risk of death in colon cancer, compared to Stage I patient, Stage IV patient has an 8.86 times greater risk of death (5).
Long non-coding RNAs (lncRNAs) are a class of RNA molecules that are longer than 200 nucleotides in length, influence a number of biological functions, including tumor development and immune cell infiltration (6,7). More and more research shows that the dysregulation of lncRNAs is important in many malignancies, including CRC (8-11). For instance, SP1 transcriptionally activates the cancer-causing lncRNA THAP7-AS1 while METTL3-mediated m6A modification stabilizes it post-transcriptionally (9). Through integrin-mediated focal adhesion signaling, the lncRNA ITGB8-AS1 encourages CRC development and migration (11).
However, the mechanisms regulating lncRNA expression are yet unclear. Numerous studies have demonstrated that changes to m6A influence lncRNAs (12,13). The most frequent epigenetic methylation of mRNAs and non-coding RNAs (ncRNAs), N6-methyladenosine (m6A), has a significant effect on RNA translation, splicing, transportation, and stability (14,15). M6A regulators, which are made up of methyltransferases (writers), RNA-binding proteins (readers), and demethylases (erasers), control invertible and dynamic RNA epigenetic modification (16). Writers are made up of METTL3, METTL14, KIAA1429, RBM15, WTAP, and ZC3H13. Readers are made up of the proteins YTHDF1/2/3, YTHDC1/2, IGF2BP1/2/3, HNRNPC, and HNRNPA2B1. Erasers are made composed of ALKBH3, ALKBH5, and FTO to perform demethylation activity (17).
M6A enzymes and lncRNAs are both excellent prognostic and diagnostic biomarkers. In previous studies, m6A-related mRNAs and lncRNAs were found to be useful in predicting the prognosis for multiple cancers (18-20). For instance, Wang et al. created a signature to predict the prognosis of gastric cancer (GC) that contained 11 m6A-related lncRNAs with variably elevated levels (21). The area under curve (AUC) for 5-year overall survival (OS) was 0.94, indicating that the lncRNA signature has good predictive accuracy for GC. Additionally, Zhang et al. created the m6A-related lncRNA prognostic score (m6A-LRS), which predicted a poor prognosis for bladder cancer patients (22). The receiver operating characteristic (ROC) curves of m6A-LRS for 5-year OS prediction was 0.67, showing that the risk model was effective. LncRNA signature showed promising prognostic potential, but it was not perfect. To lessen batch effects between various testing platforms, specific levels of lncRNAs should be standardized prior to clinical use of the signature. Additionally, by pairing, iterating, and employing a novel modeling approach, Tang et al. discovered a ferroptosis-related lncRNA pair predictive signature in pancreatic ductal carcinoma (8).
In the current research, we created a brand-new m6A-related lncRNA pair predictive model for COAD. The model’s relationships with clinicopathological traits, immune-related variables, and medication sensitivity analysis were exhibited. We present this article in accordance with the TRIPOD reporting checklist (available at https://tcr.amegroups.com/article/view/10.21037/tcr-23-1883/rc).
Methods
Data collection
Data for the COAD cohort, including mRNA sequencing data, lncRNA sequencing data, and clinical features of patients, were downloaded from the TCGA website (version 13-03-2022) (https://portal.gdc.cancer.gov). Patients with complete survival data in TCGA website were included. The training cohort and validation cohort were attained with a 8:2 ratio based on cases having survival data. Using information from a recent study, we were able to derive the expression matrix for 23 m6A RNA methylation regulators, including writers (METTL3, METTL14, METTL16, WTAP, VIRMA, RBM15, RBM15B, and ZC3H13), erasers (FTO and ALKBH5), and readers (YTHDC1, YTHDC2, IGF2BP1, IGF2BP2, IGF2BP3, YTHDF1, YTHDF2, YTHDF3, HNRNPC, LRPPRC, HNRNPA2B1, FMR1, and RBMX) (16). Annotation of lncRNAs was acquired from GENCODE (https://www.gencodegenes.org/). The study was conducted in accordance with the Declaration of Helsinki (as revised in 2013).
Investigation of m6A-related lncRNAs and matching of m6A-related lncRNAs with differential expression
Pearson correlation was used to determine the lncRNAs linked to m6A from the connection between m6A-related genes and long non-coding RNAs. The absolute value of the correlation coefficient greater than 0.4, and P less than 0.001 was considered statistically significant. |log2FC| more than 1.0 and false discovery rate (FDR) less than 0.05 were selected as significant criteria for differentially expressed m6A-related lncRNAs. After that, based on these differentially expressed m6A-related lncRNAs, we constructed m6A-related lncRNA pairings, as previously described (8). All m6A-related lncRNAs that were differently expressed were paired cyclically and assigned a value based on pairwise comparisons: assuming that lncRNA A and lncRNA B were coupled to form lncRNA pair C, which was assigned a value of 1 if lncRNA A’s expression level was higher than lncRNA B’s, and a value of 0 otherwise. An lncRNA pair was filtered if it had a 0 or 1 ratio of less than 20% or more than 80% across all samples. Cox regression analysis was used to determine the prognostic importance of m6A-related lncRNA pairs (P<0.01).
Building and evaluating a predictive signature for m6A-related lncRNA pairs
In the training cohort, prognostically correlated m6A-related lncRNA pairings were utilized to build the least absolute shrinkage and selection operator (LASSO) regression model. Then, for these m6A-related lncRNA pairings, we built a risk score model and computed the risk score for each patient as follows: Risk score = coefficient lncRNA pair1 × expression lncRNA pair1 + coefficient lncRNA pair2 × expression lncRNA pair2 + coefficient lncRNA pair3 × expression lncRNA pair3 +……+ coefficient lncRNA pairn × expression lncRNA pairn. The risk score model was utilized to generate the 1-year ROC curve for predicting OS. Based on the median risk assessments, patients in the training and validation cohorts were separated into high- and low-risk groups. Survival curves were computed using the Kaplan-Meier method. In order to evaluate the stability of this model, a cross test was conducted using the validation cohort. We built the final model using data from the full cohort in order to get an accurate model with a bigger sample size. The ROC curves for 1, 3, and 5 years were calculated. As the ideal cut-off point for classifying various risk categories, the 1-year ROC curve’s greatest inflection point was picked. Survival results and risk scores for each patient were anticipated using a risk assessment model of prognosis prediction. Furthermore, univariate and multivariate regression analyses were performed to determine if the model was an independent predictor of OS in COAD patients.
The risk score model’s clinical correlation analysis and nomogram creation
The Chi-squared test was used to analyze the associations between the risk score model and conventional clinicopathological parameters, and the results were shown as a heatmap. The Wilcoxon signed-rank test was used to determine the risk score differences between several groups of these clinicopathological traits, and box diagrams were used to illustrate the results. Following are the labels for the P values: ***P<0.001, **P<0.01, and *P<0.05. The risk score model and clinicopathological variables were incorporated in the multivariate logistic model, which was used to produce a nomogram to estimate the survival rate of COAD patients.
Risk score model and immune-related component correlations
The Wilcoxon signed-rank test was used to determine whether there was difference in gene expression between the two risk score groups. To assess the relationships between the risk score and tumor-infiltrating immune cells (TIICs), we used a few widely used algorithms, including XCELL, TIMER, QUANTISEQ, MCPCOUNTER, EPIC, CIBERSORTABS, and CIBERSORT. The statistical significance was set at P<0.05.
Chemosensitivity prediction
Genomics of Drug Sensitivity in Cancer (GDSC) database (https://cancerrxgene.org) can be utilized to do large-scale drug screening. Combining genetic analysis with chemotherapy, medication responses can be systematically discovered. Based on the GDSC database, the half-maximum inhibitory concentration (IC50) of 30 commonly used chemotherapy drugs for gastrointestinal tumors was calculated, and an evaluation of this model’s clinical use in the treatment of COAD was conducted. In the above steps, the R package “pRRophetic” was utilized. The Wilcoxon signed-rank test was then used to assess the difference in IC50 between the high-risk group and low-risk group. To illustrate the data, box drawings were generated in R using “pRRophetic” and “ggplot2” (23).
Statistical analysis
The HTSeq FPKM and simple nucleotide variation data were extracted and structured using Perl software (version 5.32). The differentially expressed lncRNAs were identified using the Benjamini-Hochberg technique based on the log fold change and FDR. The Kaplan-Meier method was used to evaluate the survival analyses of COAD patients based on the risk score model. The Cox regression model was used for multivariate analysis. The studies were carried out using R software 4.0.5 and Bioconductor packages.
Results
Data characteristics
The current study included 473 COAD and 41 adjacent healthy tissues with expression data. Table 1 shows the clinical information for the patients (n=452), including age, gender, stage, T status, N status, and M status. Twenty-three m6A-related genes in total were obtained from one earlier article (16). There were 579 lncRNAs identified as m6A-related lncRNAs in total. After that, 243 of them were found to have differently expressed m6A-related lncRNAs (232 of which were upregulated and 11 of which were downregulated), which were displayed using a heatmap (Figure 1A) and a volcano plot (Figure 1B).
Table 1
Variables | Values (N=452) |
---|---|
Age (years) | |
<65 | 169 |
≥65 | 283 |
Gender | |
Male | 214 |
Female | 238 |
Stage | |
I | 76 |
II | 178 |
III | 125 |
IV | 62 |
NA | 11 |
T | |
T0 | 0 |
Tis | 1 |
T1 | 10 |
T2 | 77 |
T3 | 308 |
T4 | 56 |
N | |
N0 | 269 |
N1 | 103 |
N2 | 80 |
M | |
M0 | 334 |
M1 | 62 |
NA | 56 |
COAD, colon adenocarcinoma; TCGA, The Cancer Genome Atlas; NA, not applicable; T, tumor; N, node; M, metastasis.
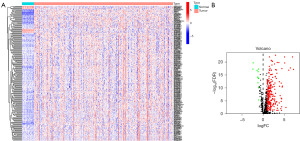
Creation of a predictive model with m6A-related lncRNA pair risk scores
A 0-or-1 matrix of 14,980 m6A-related lncRNA pairs was built for a more objective prognostic evaluation model that did not require normalization of individual expression values. Univariate Cox proportional hazards regression analyses indicated that 318 m6A-related lncRNA pairs were prognostic-associated lncRNA pairs. After performing LASSO regression analysis on the training cohort, a predictive signature containing 26 m6A-related lncRNA pairs was established. The risk score model’s AUC for the 1-year survival rate in the training and validation cohort were 0.926 and 0.979, correspondingly (Figure S1A,S1B). In both the training cohort and the validation cohort, survival analyses revealed a substantial difference between the high- and low-risk groups (Figure S1C,S1D). Following that, we built a risk score model using data from the complete cohort. A prognostic signature with 35 m6A-related lncRNA pairs was created after LASSO regression analysis (Figure 2A-2C). Table 2 lists the 35 m6A-related pairs along with the appropriate calculation coefficients. LncRNAs sequences were acquired from GENCODE. For the 1-, 3-, and 5-year survival rates, the AUCs were 0.938, 0.933, and 0.930 (Figure 3A). Additionally, we determined that the ideal cut-off point on the 1-year ROC curve was the greatest inflection point of 20.296 (Figure 3B). Furthermore, the risk score model outperformed standard clinicopathological factors like age, gender, and stage in predicting the OS of COAD patients, according to our findings (Figure 3C).
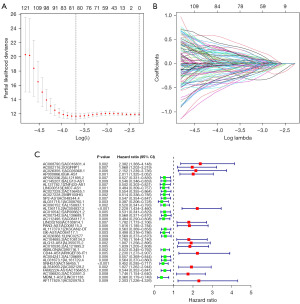
Table 2
lncRNA pair | Coefficient |
---|---|
AC068790.5|AC016831.4 | 0.858997115 |
AC002116.2|OGFRP1 | 0.742623573 |
AC026356.1|AC026368.1 | 0.903499701 |
AP000866.6|GK-AS1 | 1.418833278 |
AP002336.2|AL121895.2 | −0.742888613 |
AC145207.8|ALG13-AS1 | −0.607202023 |
AL137782.1|ZNF433-AS1 | −1.564661653 |
LINC00513|LMO7-AS1 | −1.460502637 |
AC027228.2|AC156455.1 | −0.654081795 |
AC027228.2|MIR100HG | −0.775889553 |
SP2-AS1|AC048344.4 | −0.833135322 |
AL031716.1|AC008760.1 | −0.896116458 |
AC090152.1|AL158837.1 | −0.913222126 |
AL136115.2|AC004837.2 | 1.150803418 |
AC010542.5|AP006621.2 | −0.866521051 |
AC007342.5|AL138689.1 | −0.477772369 |
AC112496.1|AC084117.1 | −0.826878942 |
LINC02163|AC100814.1 | 0.812451829 |
PAN3-AS1|AC008115.3 | 0.737068795 |
AL117379.1|ZKSCAN2-DT | −1.251469056 |
GK-AS1|AC084117.1 | 0.699519969 |
AC026368.1|LINC02577 | −0.876128589 |
AC104695.3|AC108134.3 | 0.528219693 |
ALG13-AS1|AL355075.2 | 1.055026661 |
AC010536.2|AL121895.2 | 0.923678667 |
ABALON|AC090116.1 | −0.728559966 |
CD44-AS1|ARHGEF38-IT1 | 1.422617605 |
AC004241.3|AL138689.1 | −0.670518358 |
AL031673.1|AL161729.4 | −1.015071998 |
SNHG10|AC156455.1 | −0.681577301 |
AL354993.2|AC002128.2 | 0.453187294 |
FAM222A-AS1|AC156455.1 | −0.529227502 |
AC106820.3|AC103591.3 | 1.098883888 |
MBNL1-AS1|LINC01138 | −1.673238208 |
AF117829.1|AC020978.3 | 0.62944427 |
lncRNA, long non-coding RNA.
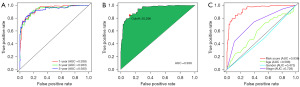
Clinical connection of the prognostic model and prediction evaluation
Using the previously established cut-off point, 344 patients were assigned to the low-risk group and 82 to the high-risk group. According to the risk assessment model for prognosis prediction, there were more deaths as the risk score rose (Figure 4A,4B). Analysis of survival data showed that the high-risk group had significantly lower OS than the low-risk group (Figure 4C). In the univariate analysis, age (P=0.09), T status, N status, M status, and risk score model were found to be significant risk factors (all P<0.001) (Figure 4D). Multivariate analysis supported the risk score model (P<0.001), T status (P<0.001), and M status (P=0.03) as independent predictive variables (Figure 4E). Additionally, the risk score model was substantially correlated with the T status, N status, M status, and stage, according to our findings (Figure 5). Furthermore, a precise predictive nomogram using the risk score model and typical clinicopathological traits was developed for predicting 1-, 3-, and 5-year OS probabilities. This nomogram could be useful in the clinical assessment of COAD patients (Figure 6).
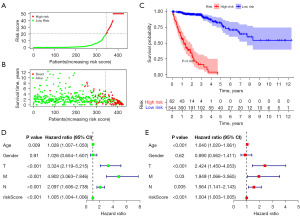
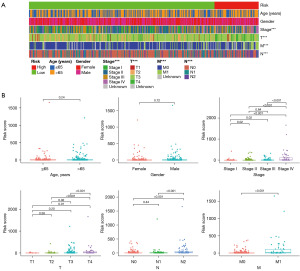
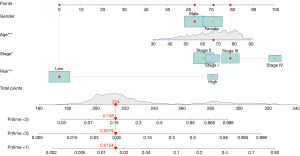
Correlations between the risk score model and immune-related factors
In consideration of the increasing evidence on the correlation between immunological features and survival in malignant tumors, the correlation between risk score model and TIICs was investigated. The results showed that the high-risk group was associated with CD8+ T cells, CD4+ T cells, and macrophage, whereas the low-risk group was associated with neutrophils, B cells, and NK cells (Figure 7).
Correlations between the risk score model and sensitivity to anticancer drugs
In order to determine potential treatment modalities for COAD, the sensitivity to 30 common anticancer drugs between the high- and low-risk groups were compared (Figure 8A). According to the findings, patients in high-risk groups had lower IC50 values for the Food and Drug Administration (FDA)-approved antitumor medicines rapamycin, lenalidomide, embelin, and dimethyloxallyl glycine (DMOG) (Figure 8B). In this context, these drugs have the potential to be applied in the treatment for COAD patients in the high-risk group in the future.
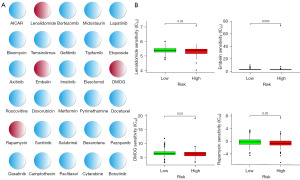
Discussion
m6A is an RNA modification that interacts with mRNAs and lncRNAs, and affects almost all biological functions of tumor cells. For example, METTL14, an m6A writer, is relevant to CRC progression by modulating SOX4 expression (24). Recent study found that LINC00460 improved HMGA1 mRNA stability and protein expression by directly interacting with IGF2BP2 and DHX9 to bind the 3’ untranslated region (UTR) of HMGA1 mRNA. M6A modification of HMGA1 mRNA by METTL3 enhanced HMGA1 expression in CRC (25). Lu and his coworkers revealed that the m6A reader IMP2 worked ZFAS1, and that these two factors work together to induce CRC by boosting mitochondrial energy metabolism (26). It was reported that YTHDF1, an m6A reader, effectively synergizes with cisplatin by inhibiting protein synthesis of GLS1 to induce colon cancer cell death (27). In addition, Zhang et al. summarized the mutual regulation mechanism of m6A modification and lncRNA in tumors, further indicating that not only m6A can regulate the level of lncRNA, but also lncRNA can manipulate the level of m6A modification and biological effects by regulating demethylase and methyl-binding protein (28). These investigations showed that m6A is essential for regulating the development and division of colon cancer cells and that it works in synergy with drugs to cause colon cancer cell death. Additionally, researches have showed that m6A-related lncRNA signatures can be utilized to anticipate prognosis, improve risk analysis for survival, and enable tailored treatment in CRC (29-31). Xue et al. established a prognostic model of disulfidptosis-associated lncRNA in COAD, and the AUCs of 1-, 3-, and 5-year survival rates were 0.679, 0.703, and 0.744, respectively (32). A study has shown that lncRNAs such as ESRG, LINC00518 and PWRN1 can be used as diagnostic and prognostic biomarkers in COAD (33). Compared with the above prognostic models, our model has better predictive value and we identified a brand-new m6A-related lncRNA pair predictive model for COAD. There are certain practical issues with these models. These forecasting algorithms were developed using the particular expression levels of the discovered lncRNAs. Before applying the measurements in a clinical setting, to remove batch effects between different testing platforms, the measured results must be standardized. We created the brand-new m6A-related lncRNA pair predictive model for COAD. The area under the curve (AUC) for predicting 1-, 3-, and 5-year survival rates demonstrated outstanding predictive accuracy, with values of 0.938, 0.930, and 0.916, respectively. Our prognostic signature, which included 35 m6A-related lncRNA pairs, was verified by Cox regression analysis to be an independent predictive factor. Notably, it was better at predicting the OS for COAD than frequent clinicopathological factors. More crucially, the signature was created by pairing, iteration, and a novel modeling technique; as a result, it may be used more effectively in clinical settings.
The immune microenvironment is crucial in carcinogenesis. Infiltrating immune cells may work to antagonize tumors or to promote tumors (34,35). Cancer cells have evolved multiple mechanisms to escape immune surveillance, resulting in cancer development (36). In recent years, immunotherapy has been innovated in cancer treatment and has demonstrated remarkable success (37,38). Furthermore, m6A and immunity are intimately related (39). M6A can regulate a wide range of immune cells and has a number of regulatory modes and processes, including influencing T cell development, regulatory T cell status, and dendritic cell maturation (40). A recent study has shown that m6A methylation is a key epigenetic modification in the differentiation of tumor-associated macrophages in CRC, and METTL3 knockdown can promote the invasiveness of CRC by down-regulating miR-146b (41). In this work, we examined the correlation between the risk score model and variables related to the immune system. The findings demonstrated that the high-risk group was connected to more TIICs, such as CD4+ T cells, CD8+ T cells, and macrophages, in contrast, the low-risk group exhibited a higher association with TIICs, such as neutrophils, B cells, and NK cells. The discovery that m6A-related lncRNAs were connected to immune cell infiltration in COAD may help us identify new treatment targets. Despite recent advances in tumor immunotherapy, the overall therapeutic impact of COAD is unsatisfactory. Therefore, creating multimode therapy and biointegration targets is required. Further investigation is needed to explore the association between COAD and lncRNAs related to m6A modification.
Typically, patients with high-risk COAD undergo a combination of chemotherapy and surgery as part of their treatment regimen. By utilizing the GDSC database, we found that high-risk individuals demonstrated greater sensitivity to commonly prescribed chemotherapeutic drugs (such as lenalidomide, embelin, DMOG, and rapamycin) compared to low-risk patients. This discovery has the potential to unveil novel treatment possibilities for patients with COAD. Although lenalidomide’s anticancer mechanism is still not fully understood, it appears to cause angiogenesis inhibition and immunomodulation (42). In the tumor environment, embelin boosted the infiltration of CD8+ T cells, NK cells, and mature dendritic cells while decreasing the number of regulatory T cells (43). Rapamycin, the first naturally occurring mammalian target of rapamycin (mTOR) inhibitor, prevented the growth of CRC cells that were susceptible to rapamycin (44). Further clinical trials are necessary to assess the effectiveness of these chemotherapeutic drugs in patients with COAD.
There are some limitations in this study. First, additional experimental confirmation is needed as we can only draw inferences from bioinformatics research. Second, more samples should be included in the future.
Conclusions
In conclusion, the m6A-related lncRNA pairs in COAD were systematically identified and analyzed for the first time in the research. We have identified m6A-related lncRNA pairs that hold prognostic value and have developed a novel risk model that demonstrates excellent predictive ability for prognosis and survival status. The risk score is a new and potential biomarker since it has a strong correlation with the malignant clinicopathological characteristics of COAD. Moreover, our findings offer crucial support for additional research on the role of m6A-related lncRNA pairs in COAD, which may offer fresh perspectives on how to direct an efficient immunotherapy regimen for COAD.
Acknowledgments
Funding: This work was supported by
Footnote
Reporting Checklist: The authors have completed the TRIPOD reporting checklist. Available at https://tcr.amegroups.com/article/view/10.21037/tcr-23-1883/rc
Peer Review File: Available at https://tcr.amegroups.com/article/view/10.21037/tcr-23-1883/prf
Conflicts of Interest: All authors have completed the ICMJE uniform disclosure form (available at https://tcr.amegroups.com/article/view/10.21037/tcr-23-1883/coif). The authors have no conflicts of interest to declare.
Ethical Statement: The authors are accountable for all aspects of the work in ensuring that questions related to the accuracy or integrity of any part of the work are appropriately investigated and resolved. The study was conducted in accordance with the Declaration of Helsinki (as revised in 2013).
Open Access Statement: This is an Open Access article distributed in accordance with the Creative Commons Attribution-NonCommercial-NoDerivs 4.0 International License (CC BY-NC-ND 4.0), which permits the non-commercial replication and distribution of the article with the strict proviso that no changes or edits are made and the original work is properly cited (including links to both the formal publication through the relevant DOI and the license). See: https://creativecommons.org/licenses/by-nc-nd/4.0/.
References
- Bardou M, Rouland A, Martel M, et al. Review article: obesity and colorectal cancer. Aliment Pharmacol Ther 2022;56:407-18. [Crossref] [PubMed]
- Barresi V, Reggiani Bonetti L, Ieni A, et al. Histological grading in colorectal cancer: new insights and perspectives. Histol Histopathol 2015;30:1059-67. [PubMed]
- Rawla P, Sunkara T, Barsouk A. Epidemiology of colorectal cancer: incidence, mortality, survival, and risk factors. Prz Gastroenterol 2019;14:89-103. [Crossref] [PubMed]
- Lee RM, Cardona K, Russell MC. Historical perspective: Two decades of progress in treating metastatic colorectal cancer. J Surg Oncol 2019;119:549-63. [Crossref] [PubMed]
- Lee YH, Kung PT, Wang YH, et al. Effect of length of time from diagnosis to treatment on colorectal cancer survival: A population-based study. PLoS One 2019;14:e0210465. [Crossref] [PubMed]
- Mercer TR, Dinger ME, Mattick JS. Long non-coding RNAs: insights into functions. Nat Rev Genet 2009;10:155-9. [Crossref] [PubMed]
- Yao RW, Wang Y, Chen LL. Cellular functions of long noncoding RNAs. Nat Cell Biol 2019;21:542-51. [Crossref] [PubMed]
- Tang R, Wu Z, Rong Z, et al. Ferroptosis-related lncRNA pairs to predict the clinical outcome and molecular characteristics of pancreatic ductal adenocarcinoma. Brief Bioinform 2022;23:bbab388. [Crossref] [PubMed]
- Liu HT, Zou YX, Zhu WJ, et al. lncRNA THAP7-AS1, transcriptionally activated by SP1 and post-transcriptionally stabilized by METTL3-mediated m6A modification, exerts oncogenic properties by improving CUL4B entry into the nucleus. Cell Death Differ 2022;29:627-41. [Crossref] [PubMed]
- Alkhathami AG, Hadi A, Alfaifi M, et al. Serum-Based lncRNA ANRIL, TUG1, UCA1, and HIT Expressions in Breast Cancer Patients. Dis Markers 2022;2022:9997212. [Crossref] [PubMed]
- Lin X, Zhuang S, Chen X, et al. lncRNA ITGB8-AS1 functions as a ceRNA to promote colorectal cancer growth and migration through integrin-mediated focal adhesion signaling. Mol Ther 2022;30:688-702. [Crossref] [PubMed]
- He RZ, Jiang J, Luo DX. The functions of N6-methyladenosine modification in lncRNAs. Genes Dis 2020;7:598-605. [Crossref] [PubMed]
- Huang H, Weng H, Chen J. m(6)A Modification in Coding and Non-coding RNAs: Roles and Therapeutic Implications in Cancer. Cancer Cell 2020;37:270-88. [Crossref] [PubMed]
- Wang T, Kong S, Tao M, et al. The potential role of RNA N6-methyladenosine in Cancer progression. Mol Cancer 2020;19:88. [Crossref] [PubMed]
- Zhao BS, Roundtree IA, He C. Post-transcriptional gene regulation by mRNA modifications. Nat Rev Mol Cell Biol 2017;18:31-42. [Crossref] [PubMed]
- Zaccara S, Ries RJ, Jaffrey SR. Reading, writing and erasing mRNA methylation. Nat Rev Mol Cell Biol 2019;20:608-24. [Crossref] [PubMed]
- Meyer KD, Jaffrey SR. Rethinking m(6)A Readers, Writers, and Erasers. Annu Rev Cell Dev Biol 2017;33:319-42. [Crossref] [PubMed]
- Niu Y, Lin Z, Wan A, et al. RNA N6-methyladenosine demethylase FTO promotes breast tumor progression through inhibiting BNIP3. Mol Cancer 2019;18:46. [Crossref] [PubMed]
- Li Z, Li F, Peng Y, et al. Identification of three m6A-related mRNAs signature and risk score for the prognostication of hepatocellular carcinoma. Cancer Med 2020;9:1877-89. [Crossref] [PubMed]
- Jin Y, Wang Z, He D, et al. Analysis of m6A-Related Signatures in the Tumor Immune Microenvironment and Identification of Clinical Prognostic Regulators in Adrenocortical Carcinoma. Front Immunol 2021;12:637933. [Crossref] [PubMed]
- Wang H, Meng Q, Ma B. Characterization of the Prognostic m6A-Related lncRNA Signature in Gastric Cancer. Front Oncol 2021;11:630260. [Crossref] [PubMed]
- Zhang Y, Zhu B, He M, et al. N6-Methylandenosine-Related lncRNAs Predict Prognosis and Immunotherapy Response in Bladder Cancer. Front Oncol 2021;11:710767. [Crossref] [PubMed]
- Slavin ML. Metastatic malignant meningioma. J Clin Neuroophthalmol 1989;9:55-9. [PubMed]
- Chen X, Xu M, Xu X, et al. METTL14-mediated N6-methyladenosine modification of SOX4 mRNA inhibits tumor metastasis in colorectal cancer. Mol Cancer 2020;19:106. [Crossref] [PubMed]
- Hou P, Meng S, Li M, et al. LINC00460/DHX9/IGF2BP2 complex promotes colorectal cancer proliferation and metastasis by mediating HMGA1 mRNA stability depending on m6A modification. J Exp Clin Cancer Res 2021;40:52. [Crossref] [PubMed]
- Lu S, Han L, Hu X, et al. N6-methyladenosine reader IMP2 stabilizes the ZFAS1/OLA1 axis and activates the Warburg effect: implication in colorectal cancer. J Hematol Oncol 2021;14:188. [Crossref] [PubMed]
- Chen P, Liu XQ, Lin X, et al. Targeting YTHDF1 effectively re-sensitizes cisplatin-resistant colon cancer cells by modulating GLS-mediated glutamine metabolism. Mol Ther Oncolytics 2021;20:228-39. [Crossref] [PubMed]
- Zhang N, Sun Y, Mei Z, et al. Novel insights into mutual regulation between N(6)-methyladenosine modification and LncRNAs in tumors. Cancer Cell Int 2023;23:127. [Crossref] [PubMed]
- Zhang P, Liu G, Lu L. N6-methylandenosine-related lncRNA signature is a novel biomarkers of prognosis and immune response in colon adenocarcinoma patients. Front Cell Dev Biol 2021;9:703629. [Crossref] [PubMed]
- Zeng H, Xu Y, Xu S, et al. Construction and Analysis of a Colorectal Cancer Prognostic Model Based on N6-Methyladenosine-Related lncRNAs. Front Cell Dev Biol 2021;9:698388. [Crossref] [PubMed]
- Song W, Ren J, Yuan W, et al. N6-Methyladenosine-Related lncRNA Signature Predicts the Overall Survival of Colorectal Cancer Patients. Genes (Basel) 2021;12:1375. [Crossref] [PubMed]
- Xue W, Qiu K, Dong B, et al. Disulfidptosis-associated long non-coding RNA signature predicts the prognosis, tumor microenvironment, and immunotherapy and chemotherapy options in colon adenocarcinoma. Cancer Cell Int 2023;23:218. [Crossref] [PubMed]
- Jafari N, Nasiran Najafabadi A, Hamzei B, et al. ESRG, LINC00518 and PWRN1 are newly-identified deregulated lncRNAs in colorectal cancer. Exp Mol Pathol 2022;124:104732. [Crossref] [PubMed]
- Lei X, Lei Y, Li JK, et al. Immune cells within the tumor microenvironment: Biological functions and roles in cancer immunotherapy. Cancer Lett 2020;470:126-33. [Crossref] [PubMed]
- Pauken KE, Wherry EJ. SnapShot: T Cell Exhaustion. Cell 2015;163:1038-1038.e1. [Crossref] [PubMed]
- Zhang Y, Zhang Z. The history and advances in cancer immunotherapy: understanding the characteristics of tumor-infiltrating immune cells and their therapeutic implications. Cell Mol Immunol 2020;17:807-21. [Crossref] [PubMed]
- Hinshaw DC, Shevde LA. The Tumor Microenvironment Innately Modulates Cancer Progression. Cancer Res 2019;79:4557-66. [Crossref] [PubMed]
- Mantovani A, Ponzetta A, Inforzato A, et al. Innate immunity, inflammation and tumour progression: double-edged swords. J Intern Med 2019;285:524-32. [Crossref] [PubMed]
- Dong L, Cao Y, Hou Y, et al. N(6) -methyladenosine RNA methylation: A novel regulator of the development and function of immune cells. J Cell Physiol 2022;237:329-45. [Crossref] [PubMed]
- Zhang M, Song J, Yuan W, et al. Roles of RNA Methylation on Tumor Immunity and Clinical Implications. Front Immunol 2021;12:641507. [Crossref] [PubMed]
- He S, Song W, Cui S, et al. Modulation of miR-146b by N6-methyladenosine modification remodels tumor-associated macrophages and enhances anti-PD-1 therapy in colorectal cancer. Cell Oncol (Dordr) 2023;46:1731-46. [Crossref] [PubMed]
- Leuci V, Maione F, Rotolo R, et al. Lenalidomide normalizes tumor vessels in colorectal cancer improving chemotherapy activity. J Transl Med 2016;14:119. [Crossref] [PubMed]
- Wu T, Wang C, Wang W, et al. Embelin impairs the accumulation and activation of MDSCs in colitis-associated tumorigenesis. Oncoimmunology 2018;7:e1498437. [Crossref] [PubMed]
- Sun L, Yan Y, Lv H, et al. Rapamycin targets STAT3 and impacts c-Myc to suppress tumor growth. Cell Chem Biol 2022;29:373-385.e6. [Crossref] [PubMed]