Machine learning-based integration of CD8 T cell-related gene signatures for comprehensive prognostic assessment in lung adenocarcinoma
Highlight box
Key findings
• Employing ten machine-learning algorithms, we generated 101 combinations, selecting the optimal algorithm to construct an artificial intelligence-derived prognostic signature named riskScore. The signature was a strong predictor of lung adenocarcinoma (LUAD).
What is known and what is new?
• CD8 T cells are consistently present throughout all stages of tumor development and play a pivotal role within the tumor microenvironment.
• CD8 T cell-related genes were used to construct a prognostic model, and the new model was found to be associated with the prognosis of LUAD.
What is the implication, and what should change now?
• This study highlights the importance of CD8 T cell-related genes in predicting prognosis in LUAD.
Introduction
Lung cancer continues to pose a significant health challenge, with increasing global incidence and mortality rates. A defining characteristic of this disease is the prevalence of non-small cell lung cancer (NSCLC), with lung adenocarcinoma (LUAD) standing out as the most common subtype (1,2). Despite significant advances in cancer research and the development of various treatments, the prognosis for LUAD patients remains compromised due to late-stage diagnosis, metastasis, and recurrence. Early-stage LUAD is particularly prone to metastasis, resulting in a generally unfavorable prognosis, with a less than 20% average 5-year survival rate (3,4). In clinical practice, decision-making, therapeutic strategies, and follow-up procedures still heavily rely on the conventional anatomy-based tumor-node-metastasis (TNM) staging system for NSCLC. This system serves as both a prognostic tool and a guide for treatment decisions. However, the current system, primarily based on tumor histology and morphology, falls short in comprehensively elucidating the complexity of this disease. Notably, tumors with similar histological characteristics or pathological stages do not consistently exhibit similar clinical behaviors or respond equally to identical treatments. In fact, a significant proportion, ranging from 30% to 55%, of early-stage NSCLC patients experience disease relapse and succumb to the illness despite undergoing complete resection with clear resection margins (5). The pursuit of novel prognostic biomarkers is of paramount importance to improve patient stratification and treatment efficacy. One of the most prominent features of tumors is the imbalance within the tumor microenvironment (TME). Beyond cancer cells and T cells, the TME encompasses a multitude of immune and non-immune components, including stromal cells, blood vessels, neurons, and the extracellular matrix. In the last decade, our understanding of CD8 T cell differentiation within tumors has become increasingly comprehensive and detailed. CD8 T cells serve as the ultimate effectors of cancer immunity, and the effectiveness of most cancer immunotherapies hinges on the effector functions of CD8 T cells (6). Traditional bulk RNA-sequencing methods involve the analysis of a mixture of all cells, which tends to obscure the distinct transcriptomes unique to individual cell types. In contrast, single-cell RNA-sequencing (scRNA-seq) captures and characterizes the gene expression patterns of each individual cell, allowing for the deciphering of their intercellular signaling networks (7). It is now evident that CD8 T cells infiltrating tumor tissue can exhibit a range of states, including a naive-like, effector, resident memory, or exhausted state (8). In recent years, with the advancement of high-throughput sequencing and evidence-based medicine, studies from The Cancer Genome Atlas (TCGA) have provided comprehensive characterizations of the major subtypes in the transcriptome and genome of LUAD (9,10). Many multigene panels have been developed to address the extensive heterogeneity of the disease, showing promising performance in specific cohorts. For example, Jones introduced a genomic-pathologic annotated risk model for predicting recurrence in early-stage LUAD (11). Zhang et al. devised a novel basement membrane-related gene signature to predict prognosis (12). Shi et al. developed a prognostic immune-related gene signature for LUAD with resistance to tyrosine kinase inhibitors (TKIs) (13). However, there were a handful of known studies with CD8 T cells-related signatures (14,15). Therefore, it became imperative to incorporate CD8 T cell-related genes into preclinical models to construct prognostic biomarkers. However, the limitations of current modeling methods and the lack of rigorous validation in large multicenter cohorts have rendered expression-based multigene signatures less applicable in clinical settings.
In this study, which aimed to establish an optimal biomarker centered on CD8 T cell-related genes, we meticulously constructed and subjected the 23 artificial intelligence-derived CD8 T prognostic signatures (riskScore) to multicenter validation. This validation process encompassed a comprehensive analysis using 101 machine-learning algorithm combinations, based on data from four independent public datasets. The riskScore demonstrated remarkable and consistent performance in predicting overall survival (OS), progression-free interval (PFI), disease-free interval (DFI), response to immunotherapy, and drug efficacy. The findings of this research hold the potential to significantly enhance the precision of treatment strategies and subsequently improve the clinical outcomes of patients with LUAD. We present this article in accordance with the TRIPOD reporting checklist (available at https://tcr.amegroups.com/article/view/10.21037/tcr-23-2332/rc).
Methods
Data acquisition and preprocessing
We collected the TCGA-LUAD dataset from TCGA (http://portal.gdc.cancer.gov/), which comprised RNA expression data in transcripts per kilobase million (TPM) format along with corresponding clinical features. Additionally, we obtained datasets GSE31210 (16), GSE3141 (17), GSE135222 (18), and GSE72094 (19) from the Gene Expression Omnibus (GEO) (https://www.ncbi.nlm.nih.gov/geo/), all of which included RNA expression data and associated clinical information for LUAD. For the IMvigor210 cohort, we retrieved both expression data and clinical data from http://research-pub.gene.com/IMvigor210CoreBiologies/ (20). Comprehensive details regarding scRNA-seq data (GSE176021) were sourced from the Tumor Immune Single Cell Hub 2 (TISCH2) (21). This study was conducted in accordance with the Declaration of Helsinki (as revised in 2013).
Signature generated from machine-learning-based integrative approaches
To develop a consensus risk model with high accuracy and stability, we employed a multistep methodology. First, by applying the Wilcoxon rank-sum test to the TCGA-LUAD dataset, we pinpointed genes that showed significant expression differences between tumor and adjacent normal tissues. Following this, we identified genes with significant prognostic value using univariate Cox regression analysis on the same dataset. Additionally, we isolated genes associated with CD8 T cells by comparing them against other cell types in the TISCH2 database. The intersection of these three gene sets yielded a final list genes related to CD8 T cell-related prognosis genes (CD8TRPGs). We then integrated 10 machine-learning algorithms and 101 algorithm combinations. These algorithms included random survival forest (RSF), elastic network (Enet), least absolute shrinkage and selection operator (Lasso), ridge regression (Ridge), stepwise Cox (StepCox), CoxBoost, partial least squares regression for Cox (plsRcox), supervised principal components (SuperPC), generalized boosted regression modeling (GBM), and survival support vector machine (survivalSVM). Among them, RSF, Lasso, CoxBoost, and stepwise Cox possessed dimensionality reduction and variable screening capabilities, and we combined them with other algorithms to create 101 machine-learning algorithm combinations. The detailed process of signature generation unfolded as follows:
- Initially, we conducted differential expression analysis between tumor and normal samples in the TCGA-LUAD dataset using the Wilcoxon rank-sum test. Genes were selected based on the following criteria: P<0.05 and |log2fold change (FC)| >1. Additionally, in the TCGA-LUAD cohort, we carried out univariate Cox regression analysis and selected genes using the following criterion: P<0.05, and TISCH2 served as a valuable resource for scRNA-seq data from both human and mouse tumors, enabling a comprehensive characterization of gene expression within the TME. In this study, we retrieved CD8 T-related genes from TISCH2 using specific criteria: log2FC > log2(1.5) and adjusted P<0.05. Subsequently, an intersection of these three gene sets was performed, yielding a consolidated list of 33 CD8TRPGs.
- Following this, the 101 combinations of algorithms were utilized to independently construct prognostic signatures based on the expression profiles of the 33 CD8TRPGs within the TCGA-LUAD training cohort.
- Based on the above results, we selected the combination of RSF and StepCox[forward], which achieved the highest average C-index (0.707). This combination identified a final model named riskScore consisting of 23 CD8TRPGs.
- We calculated a riskScore for each patient using the expression of 23 CD8TRPGs weighted by their regression coefficients in a Cox model. RiskScores were computed for each validation dataset, namely GSE31210, GSE3141, and GSE72094, were calculated using the signature derived from the training cohort.
- Harrell’s concordance index (C-index) was then computed for each model across all validation datasets, and the model with the highest average C-index was selected as the optimal one.
Comprehensive analysis of single-cell datasets and cell cluster annotation
We conducted an extensive analysis of single-cell datasets and performed cell cluster annotation. The analysis of the scRNA-seq dataset was carried out using the R package Seurat (v4.1.1) (22). Uniform Manifold Approximation and Projection (UMAP) analysis was performed using Seurat’s built-in function RunUMAP and the umap-learn algorithm, in addition to the Leiden algorithm. To visualize the results, we utilized dimplot, featureplot, violin, and dotplot. To further characterize different clusters of cell subtypes, we calculated metabolic scores using the R package scMetabolism with the AUCell method within the reactome pathway (23). The outcomes of the scMetabolism calculations were integrated and visualized using dotplot pheatmap, allowing us to display the metabolism of various cell subtype clusters.
Validating the prognostic value of risk model
To validate the prognostic value of the risk model, patients in the training cohort, three testing cohorts, and the meta-cohort were stratified into high and low-risk score groups based on the optimal cutpoint value. The prognostic significance of the riskScore was assessed using Kaplan-Meier curves and multivariate Cox regression analysis. Additionally, calibration curves and receiver operating characteristic (ROC) curves were generated to evaluate the predictive accuracy of the risk model.
Nomogram and calibration
Multivariate Cox regression analysis incorporating clinical features (age, stage, gender) and the riskScore was conducted to construct the nomogram using the R package “regplot”. Subsequently, calibration curves at 1, 3, and 5 years were generated to validate the accuracy of the nomogram.
Genomic alteration landscape
To explore the genomic alteration landscape in the high- and low-risk subgroups, we conducted a comparative analysis of tumor mutational burden (TMB), neoantigen (NEO), and microsatellite instability (MSI) score between the high- and low-risk subgroups within the TCGA-LUAD dataset.
Cells infiltration estimation
Single-sample gene set enrichment analysis (ssGSEA) was employed using the R package gene set variation analysis (GSVA) to quantify the relative infiltration of immune cells and immune cell functions within the TCGA-LUAD cohort. To validate the stability and robustness of the ssGSEA results, we utilized seven other algorithms, including TIMER (24), CIBERSORT (25), CIBERSORT_ABS, QUANTISEQ (26), MCPCOUNTER (27), XCELL (28), and EPIC algorithms (29). Additionally, the R package “estimate” was utilized to determine immune and estimate scores. Information regarding immune subtypes, derived from a previous study, was compared between the high-risk and low-risk subgroups. To predict the response to checkpoint blockade, the immunophenoscore (IPS) obtained from The Cancer Immunome Atlas (TCIA; https://tcia.at/home) was employed (30,31).
Gene set enrichment analysis (GSEA)
GSEA was utilized to identify specific functional pathways from Gene Ontology (GO), Kyoto Encyclopedia of Genes and Genomes (KEGG), and Hallmark collections in both the high-risk and low-risk subgroups. GSEA v4.3.2 from the MSigDB database (http://software.broadinstitute.org/gsea/msigdb/) was employed for this analysis. The criteria for selection were set at false discovery rate (FDR) <0.25 and nominal P<0.05 to determine significant pathways (32,33).
Prediction of drug sensitivity
The original data regarding chemotherapy response were sourced from the Genomics of Drug Sensitivity in Cancer (GDSC version 2) (https://www.cancerrxgene.org/) (34-36). Curated data were downloaded from https://osf.io/temyk for further analysis. To predict the difference in chemotherapy response between the high-risk and low-risk subgroups, we utilized the R package oncoPredict (37).
Consensus clustering
To discover clusters within the TCGA-LUAD cohort based on the expression of risk model genes, we employed a resampling-based method known as consensus clustering. This procedure was executed using the ConsensusClusterPlus package (38). Following cluster generation, the consensus score matrix was utilized to identify the optimal number of clusters.
Cancer Cell Line Encyclopedia (CCLE) gene expression
We obtained RNA-seq data for all LUAD cell lines from the CCLE (39), which provides gene expression profiles in cancer cells. Subsequently, we generated plots depicting the expression of the risk model genes.
Statistical analysis
All statistical analyses were conducted in R (v4.2.2, https://www.r-project.org/). Comparison between the two groups was conducted utilizing the Wilcoxon rank-sum test, and the Kruskal-Wallis test was carried out for normal multiple groups. The level of statistical significance used in this research was determined to be P<0.05.
Results
Workflow
Our study’s workflow, outlining the sequential steps utilized in our research, is illustrated in Figure 1.
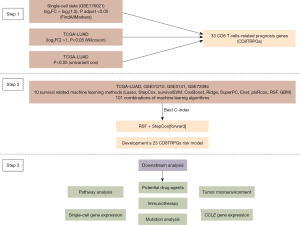
Analysis of LUAD single-cell sequencing data
Using the TISCH2 database, we obtained the scRNA-seq dataset GSE176021. As demonstrated in Figure 2A,2B, we observed that CD8 T cells had the highest proportion in the dataset. Additionally, GSEA analysis of KEGG pathways revealed that CD8 T cells were significantly enriched in pathways related to antigen processing and presentation, MAPK signaling pathway, oxidative phosphorylation, natural killer (NK) cell-mediated cytotoxicity, Regulation of actin cytoskeleton, T cell receptor signaling pathway (Figure 2C,2D). These findings indicat that CD8 T cells played a crucial role in LUAD immunity-related pathways and were worthy of further investigation.
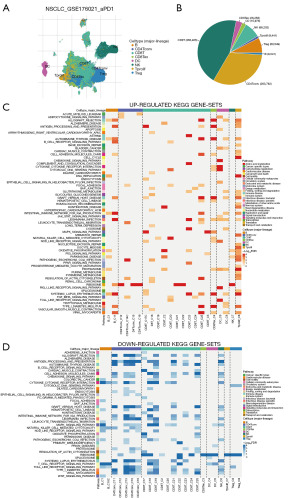
Construction of a prognosis signature based on integrative machine-learning
Our approach involved multiple steps in constructing a prognosis signature. We initially identified significantly differentially expressed genes between tumor and normal samples in the TCGA-LUAD dataset using the Wilcoxon rank-sum test. Next, we determined significant prognosis-related genes through univariate Cox regression analysis within the TCGA-LUAD dataset. We further obtained CD8 T-related genes by comparing CD8 T cells with other cells. These three sets of genes were then intersected, resulting in 33 CD8TRPGs (Figure 3A). In the TCGA-LUAD training cohort, we employed 101 algorithm combinations through ten-fold cross-validation to construct prediction models. We calculated the average C-index for each algorithm across the remaining three testing cohorts. Based on the results, we selected the combination of RSF and StepCox[forward], which achieved the highest average C-index (0.707). This combination identified a final model named riskScore consisting of 23 CD8TRPGs (Figure 3B). Subsequently, we calculated a riskScore for each patient using the expression of 23 CD8TRPGs weighted by their regression coefficients in a Cox model. Patients were divided into high-risk and low-risk subgroups based on the optimal cut-off value determined by the survminer package. As illustrated in Figure 3C-3F, high-risk group patients exhibited significantly poorer OS, DFI, disease-specific survival (DSS), PFI relative to the low-risk group in the TCGA-LUAD training dataset. Different gene expression clusters often exhibit varying immune microenvironments, which could result in diverse immunotherapeutic strategies and responses. To investigate this phenomenon, we conducted consensus clustering based on the expression of 23 CD8TRPGs which formed the riskScore. Two distinct clusters were displayed, and survival analysis displayed a significant difference between the two clusters (Figure 3G). Similarly, OS was significantly better in the low-risk group than in the high-risk group in three validation datasets (Figure 4A-4C). The GEO meta cohort combining three GEO validation cohorts (GSE31210, GSE3141, GSE72094) and TCGA-GEO meta cohort (TCGA-LUAD, GSE31210, GSE3141, GSE72094) also exhibited the same trend (Figure 4D,4E). To measure the discrimination of the riskScore, we plotted ROC curves. The area under the ROC curve (AUC) of 1-, 3-, and 5-year OS were 0.866, 0.732, 0.757 in the dataset GSE31210; 0.800, 0.799, 0.700 in the dataset GSE3141; 0.687, 0.620, 0.613 in the dataset GSE72094; 0.712, 0.654, 0.661 in the GEO meta cohort; 0.723, 0.687, 0.668 in the dataset TCGA-LUAD; 0.715, 0.671, 0.660 in the TCGA-GEO meta cohort (Figure 4F-4K). These results confirmed the good predictive performance of riskScore.
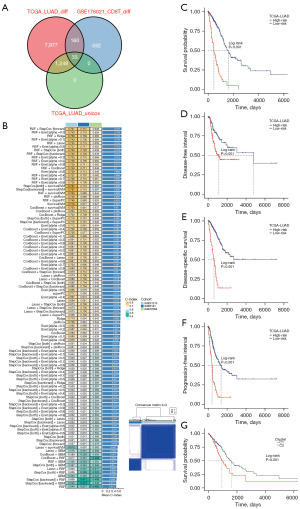
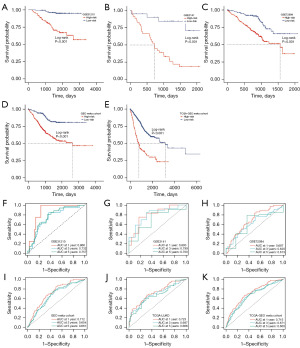
Establishment and validation of a nomogram combined with clinical characteristics
To evaluate the independent prognostic significance of the riskScore in LUAD, we conducted univariate and multivariate Cox regression analyses on OS, DSS, and PFI in the TCGA-LUAD dataset. Our findings showed that riskScore was a significant risk factor for OS, DSS, and PFI in the univariate analysis [hazard ratio (HR) >1; P<0.001]. Importantly, in the multivariate analysis, the riskScore remained an independent prognostic factor for OS [HR =1.800; 95% confidence interval (CI): 1.377–2.354; P<0.001], DSS (HR =2.052; 95% CI: 1.472–2.860; P<0.001), and PFI (HR =1.519; 95% CI: 1.204–1.918; P<0.001), indicating its robust prognostic ability in LUAD patients (Figure 5A). Further reinforcing our findings, we performed univariate and multivariate Cox regression analyses on OS in the validation datasets. The results consistently affirmed the riskScore was an independent prognostic factor for LUAD patients (HR =1.612; CI: 1.239–2.097; P<0.001) in the GSE72094 dataset (Figure 5B) and (HR =1.949; CI: 1.299–2.923; P=0.001) in the GSE31210 dataset (Figure 5C). These results underscored the reliability and consistency of our findings across diverse datasets. To enhance the clinical applicability of the riskScore, we constructed a nomogram that incorporated both the riskScore and clinical characteristics (Figure 5D). The calibration curves demonstrated excellent agreement between the predictions of the nomogram and actual observations (Figure 5E). Moreover, when comparing the AUC values for different clinical characteristics, including age (AUC =0.497), gender (AUC =0.561), stage (AUC =0.711), T (AUC =0.645), M (AUC =0.500), and N (AUC =0.670), there was a higher AUC value 0.713 at a 1-year OS time for the riskScore. In the dataset GSE72094, compared with other clinical characteristics including age (AUC =0.539), gender (AUC =0.561), and stage (AUC =0.638), there was a higher AUC value of 0.683 at a 1-year OS time for the riskScore (Figure 5F). These data suggested that the riskScore might possess higher sensitivity and accuracy in predicting the prognosis of patients with LUAD. Kaplan-Meier analysis was performed after riskScore stratification using age, M, stage, and T. Patients in the low-risk group showed improved OS compared with patients with high-risk for age >65 years (P<0.001), M0 (P<0.001), stage II–III–IV (P=0.02), T2 (P=0.004), and T3 (P=0.02) (Figure 5G).
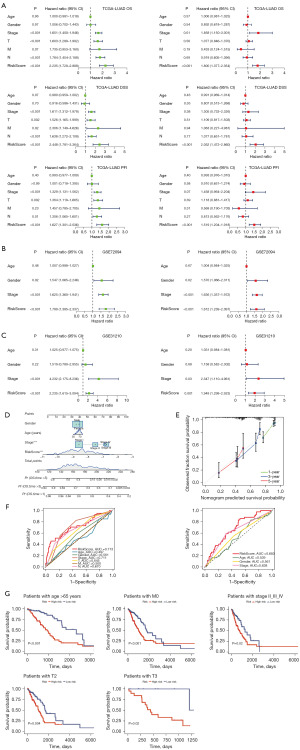
Analysis of LUAD single-cell sequencing data
Based on the TISCH2 database, we obtained the GSE176021 scRNA-seq dataset (10× genomics) and re-analyzed using R package Seurat. It was easy to find the classical marker, CD8A, CD8B, GZMK mainly expressed on the CD8 T subset (Figure 6A). Figure 6B illustrates the presentation of classical markers associated with various cell subset. In addition, we delved into the metabolic profiles of distinct cell types. Our findings revealed an enrichment of CD8TRPGs in chondroitin sulfate dermatan sulfate metabolism, glycosaminoglycan metabolism (Figure 6C). To provide further insights, we visualized the expression of 23 CD8TRPGs in different cell subsets by dotplot and violin plot (Figure 6D,6E).
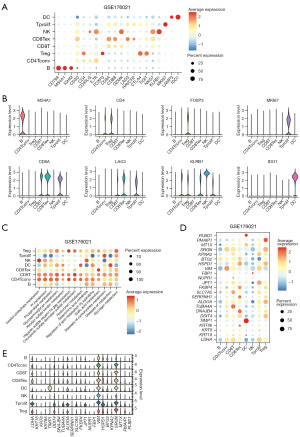
The relationship between riskScore and TME
To gain insights into the role of the TME in the progression and metastasis of LUAD, which is crucial for developing novel therapeutics, we explored the distribution of immune cell infiltration and the enrichment of immune-related functional pathways in the high- and low-risk subgroups. Notably, we observed that immune cell infiltration levels were significantly higher in the low-risk group, and immune-related functional pathways were notably enriched in this group (Figure 7A). We also evaluated estimate scores and immune scores in LUAD using the estimate algorithm, revealing a tendency for higher scores in the low-risk groups (Figure 7B). In Figure 7C, we presented the correlation between immune infiltration levels and riskScore, as determined by various algorithms, including TIMER, CIBERSORT, CIBERSORT_ABS, QUANTISEQ, MCPCOUNTER, XCELL, and EPIC. These analyses consistently showed that most immune cell infiltration levels were negatively correlated with riskScore, such as T cell CD4+ and B cell as determined by the TIMER algorithm, and T cell CD8+ and T cell NK as determined by the XCELL algorithm (Figure 7D). Furthermore, riskScore demonstrated a significant association with genomic instability, TMB, NEO load, and MSI score, with marked differences observed between the high-risk and low-risk subgroups (Figure 7E). Using the Tumor Immune Dysfunction and Exclusion (TIDE) web tool, we found that the low-risk group had significantly lower TIDE scores and Exclusion scores (Figure 7F). We also investigated the expression of immune checkpoint genes. The differential expression of immune checkpoint genes, such as CD274 (PD-L1) and PDCD1LG2 (PD-L2), indicated that the high-risk group was more susceptible to immune invasion (Figure 7G). To validate the predictive ability of riskScore regarding patients’ response to immunotherapy, we incorporated the IMvigor210 cohort, which received atezolizumab treatment. Using the risk model, we calculated the cohor’s riskScore and divided patients into high- and low-risk subgroups. Strikingly, the high-risk group exhibited significantly lower OS (Figure 7H). We also incorporated the GSE135222 cohort, a cohort of advanced NSCLC who were treated with anti-PD-1/PD-L1, the high-risk group exhibited significantly lower OS (Figure 7I). We further explored the role of riskScore in immunotherapy using the TCIA database, revealing that patients in the low-risk group were more likely to benefit from immunotherapy (Figure 7J). Thorsson et al. identified six immune subtypes across 33 diverse cancer types, providing a resource for exploring immunogenicity in cancer (40). Importantly, we observed a significant difference in immune subtype composition between the high- and low-risk subgroups (Figure 7K). In summary, these results strongly supported the notion that the low-risk group is more likely to derive substantial benefits from immunotherapy.
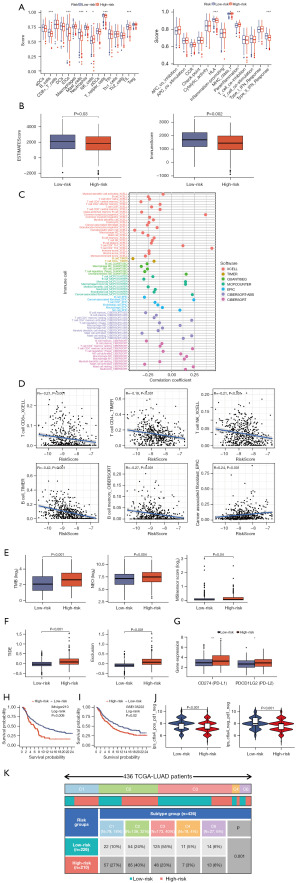
Analysis of drug sensitivity potential in high- and low-risk group
Chemotherapy and targeted therapies were prevalent in treating LUAD. It was crucial to understand how patient subgroups respond to these drugs. To this end, we assessed the response of high- and low-risk subgroups to commonly used agents in LUAD treatment. Figure 8A shows that the group with low-risk scores was more sensitive to afuresertib, and axitinib, whereas that with high-risk scores was more sensitive to BI-2536, and 5-fluorouracil (Figure 8B).
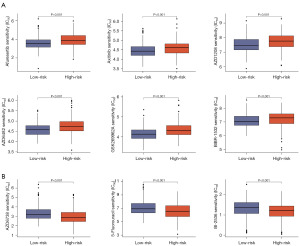
The underlying biological mechanisms of risk model
GSEA was employed to clarify the potential functional pathways underlying the significant prognostic differences observed between risk subgroups in the TCGA-LUAD dataset. Figure 9A demonstrates that the high-risk group exhibited significant enrichment in GOBP CELL CYCLE G2 M PHASE TRANSITION, GOBP CELLULAR RESPONSE TO OXIDATIVE STRESS, GOBP DNA BIOSYNTHETIC PROCESS, GOBP MITOTIC SPINDLE ASSEMBLY, GOBP POSITIVE REGULATION OF CELL CYCLE PHASE TRANSITION, GOBP POSITIVE REGULATION OF MITOTIC CELL CYCLE in the GO genesets. As illustrated in Figure 9B, the high-risk group was remarkably enriched for KEGG AMINO SUGAR AND NUCLEOTIDE SUGAR METABOLISM, KEGG CELL CYCLE, KEGG ERBB SIGNALING PATHWAY, KEGG PATHWAYS IN CANCER, KEGG PYRIMIDINE METABOLISM, KEGG WNT SIGNALING PATHWAY in the KEGG genesets. As illustrated in Figure 9C, the high-risk group was remarkably enriched for HALLMARK EPITHELIAL MESENCHYMAL TRANSITION, HALLMARK GLYCOLYSIS, HALLMARK HYPOXIA, HALLMARK KRAS SIGNALING UP, HALLMARK NOTCH SIGNALING, HALLMARK PI3K AKT MTOR SIGNALING, HALLMARK WNT BETA CATENIN SIGNALING in the hallmark genesets. The high-risk group showed a positive correlation with tumor-promoting pathways, partially explaining its association with poorer prognosis.
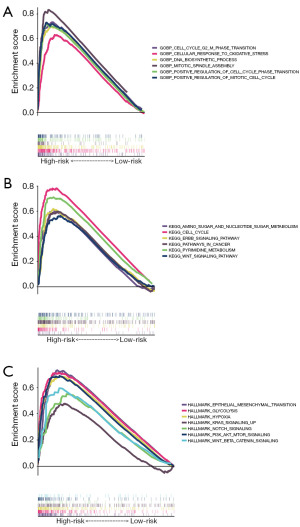
Risk gene expression in CCLE
To assess the RNA expression levels of genes in our risk model, we analyzed gene data from all LUAD cell lines in the CCLE database. Figure 10 reveals that none of these genes exhibited very low expression, which potentially enhanced the accuracy and operability of the risk model detection.
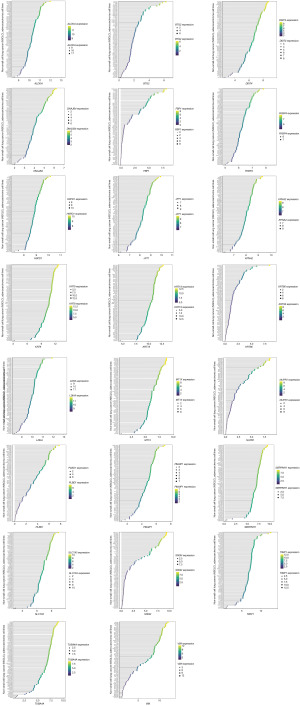
Analysis of mutations in high- and low-risk group
Genetic variations have been reported to affect the immune microenvironment. To address this point, we have carefully analyzed the TCGA-LUAD mutations and find that mutations in TP53, PTEN, and SMARCA4 are indeed associated with significantly higher riskScores (Figure S1A-S1C), which is consistent with our earlier conclusion that higher riskScores indicate poorer prognosis. However, In our study, the results show no statistically significant difference between the two groups (Figure S1D). This finding suggests that although EGFR mutations are of great clinical and basic research significance in the occurrence, progression, and treatment of LUAD, the EGFR mutation status may not be the main factor causing prognostic differences in our riskScore model.
Discussion
Lung cancer, one of the most common and severe cancers, is the leading cause of cancer-related incidence and mortality globally in both genders (41). Despite its prevalence, the survival rate for lung cancer has not seen significant improvement. This high mortality rate poses a major challenge in developing individualized treatments and predicting outcomes for LUAD patients (42). Ghiringhelli et al. highlighted the significance of a spatial quantitative analysis of CD8 and PD-L1 markers, which is predictive of the efficacy of anti-PD1/PD-L1 immunotherapy in NSCLC (43). Tostes et al. reviewed biomarkers for immune checkpoint inhibitor response, emphasizing the need for efficient tools in clinical decision-making (44). Rizvi et al. found that higher TMB was associated with improved survival in LUAD patients treated with immune checkpoint inhibitors (45). Wang et al. found that predictive power of TMB in lung cancer immunotherapy response is influenced by patients’ sex (46). Given LUAD’s heterogeneous outcomes and varied therapeutic responses, identifying robust markers for guiding clinical treatment is crucial. The absence of effective biomarkers for screening, stratified management, and prognostic follow-up remains a pressing issue for clinicians and researchers, potentially leading to over- or undertreatment. Notably, CD8 T cells infiltrating the infiltrating the TME of LUAD play a pivotal role in antitumor immunity (42,47). To address these challenges, our research focused on exploring the relationship between CD8 T-related gene profiles, prognosis, TME, and drug benefits.
In this study, we initially analyzed the differentially expressed genes between tumor and normal samples in the TCGA-LUAD dataset. Subsequently, univariate Cox analysis helped identify prognostic genes within this dataset. We further isolated CD8 T-related genes by comparing expression levels between the CD8 T subset and other subsets. By intersecting these three gene sets, we identified 33 CD8TRPGs. Addressing the challenge of overfitting, common in artificial intelligence and machine-learning for biomedical models, we noted that many models fit well in training cohorts but underperform in external validation (48,49). Leveraging the expression profiles of CD8TRPGs, we devised a novel computational framework. This framework integrates 10 machine-learning algorithms, along with their 101 combinations, to develop a unified riskScore. A total of 101 model variants were evaluated on the TCGA-LUAD training dataset using the LOOCV framework. Subsequent validations on three independent datasets pinpointed the RSF + StepCox[forward] combination as the most effective model. The primary advantage of this integrative approach was its capacity to establish a model with consistent performance in LUAD prognosis. By employing a diverse range of algorithmic combinations, it effectively reduced variable dimensionality, thereby simplifying the model for practical translational applications. We demonstrated that riskScore served as an independent risk factor for OS, PFI, and DSS of LUAD in the training TCGA-LUAD dataset. It also independently predicts OS in two validation datasets, with the third dataset lacking additional clinical features. Further, riskScore effectively stratified both the GEO meta dataset and the TCGA-GEO meta dataset into distinct OS subgroups. Notably, it showed impressive ROC performance across the training, validation, and meta datasets. Compared to traditional clinical variables like cancer stage, riskScore offers markedly enhanced accuracy. We also examined riskScore across various clinical characteristics to broaden its application scope. Significant prognostic differences were observed between high- and low-risk subgroups in the TCGA-LUAD dataset, particularly in patients age over 65 years, those with M0 status, and those in stages II–III–IV, and T2 and T3 status. Regarding molecular mechanisms, our results revealed a significant enrichment of CD8TRPGs in chondroitin sulfate dermatan sulfate metabolism, glycosaminoglycan metabolism in the scRNA-seq dataset GSE176021. Additionally, a strong positive correlation was observed between the high-risk group and tumor-promoting biological functions. These functions included the GOBP DNA BIOSYNTHETIC PROCESS, KEGG AMINO SUGAR AND NUCLEOTIDE SUGAR METABOLISM, and HALLMARK NOTCH SIGNALING. The activation of these pathways suggested elevated metabolic activity in cells of the high-risk group, a phenomenon typically associated with the heightened energy requirements of cancer cells (48-51). Remarkably, the low-risk group also showed a higher TMB, NEO, and MSI scores, with TMB being a known predictor of immunotherapy response (52,53). Clinical trials have confirmed the safety and effectiveness of tumor vaccines targeting NEOs (54). Additionally, MSI has gained approval for clinical use across tumor types (55,56). To delve deeper into specific immune cell infiltration differences, we applied the ssGSEA algorithm to each sample. We observed significantly lower immune cell and function infiltration in the low-risk group. Cancer-associated fibroblasts (CAFs) were known to facilitate tumor growth, angiogenesis, invasion, and metastasis through various pathways (57). Our analysis also showed a significant negative correlation between multiple immune cells and riskScore, while tumor-promoting CAFs positively correlated with riskScore, as identified through platforms like TIMER, XCELL, and EPIC. Cancers frequently develop the ability to evade destruction by the immune system (58). The high-risk group exhibited high TIDE and exclusion scores, indicating a higher likelihood of immune evasion in the. A higher IPS score typically suggested a better response to immunotherapy (59), and our findings showed a marked difference in IPS scores between risk subgroups, hinting at a better immunotherapy response in the low-risk group. To evaluate the efficacy of our prognostic signature in predicting immunotherapy responses, we included the IMvigor210 cohort, comprising metastatic urothelial cancer (mUC) patients treated with atezolizumab. We also incorporated the GSE135222 cohort, a cohort of advanced NSCLC who were treated with anti-PD-1/PD-L1. The riskScore effectively stratified patients by survival in these two cohorts. PD-L1 and PD-L2 have been detected in the nucleus in multiple malignancies, playing an oncogenic role independent of immune checkpoint regulation (60-63). We also found that immune checkpoint genes PD-L1 and PD-L2 were highly expressed in the high-risk group. Chemotherapy and immunotherapy were crucial adjuvant therapies for LUAD, significantly enhancing patient prognosis and quality of life. We screened a batch of small molecule chemotherapeutics using the GDSC drug susceptibility database, with the aim of improving personalized medication guidance for LUAD patients. Based on the half-maximal inhibitory concentration (IC50) prediction, the low-risk group of patients was more sensitive to AZD6738, 5-fluorouracil, and BI-2536, the low-risk group of patients was more sensitive to afuresertib, axitinib, AZD1208, AZD6482, GSK269962A, and BIBR-1532. In summary, riskScore emerged as a robust and promising tool for guiding clinical management and tailoring individualized treatment in LUAD patients. Regarding the 23 CD8TRPGs included in the risk model, research into their specific roles in tumor development is still in its early stages. Lactate dehydrogenase A (LDHA) was a key enzyme involved in glucose metabolism, whilst its aberrant expressions were often associated with tumorigenesis (64). TIMP1 was identified as related to energy metabolism and ribosome synthesis that was upregulated in the early stages of LUAD and may promote progression (65). DDIT4 was a gene of a three TKI resistant-related gene signature in LUAD (13). KRT8 could serve as a novel biomarker for LUAD and promotes metastasis and EMT via NF-κB signaling (66). Using LUAD tissues and clinical samples, SERPINH1 was shown to be a prognostic biomarker for LUAD (67). LINC01614 led to the upregulation of the glutamine transporters SLC38A2 and SLC7A5 and eventually enhanced the glutamine influx of cancer cells in LUAD (68). FKBP4 integrated FKBP4/Hsp90/IKK with FKBP4/Hsp70/RelA complex to promote LUAD progression via IKK/NF-κB signaling (69). DARS2 expression could inhibit the proliferation and migration of LUAD cells, promote cell apoptosis, and inhibit the glycolytic activity of tumor cells by inhibiting the expression of glycolytic-related gene ALDOA (70). FBP1 blockade upregulated HIF1α, triggered the switch to anaerobic glycolysis, and enhanced glucose uptake in LUAD (71). HSPD1 may play a role in the regulation of ribosome biogenesis and B cell-mediated immunity in LUAD (72). BTG2 and SerpinB5 might serve as potential prognostic biomarkers and novel therapeutic targets for LUAD (73). Integrated scRNA-seq analysis revealed that KPNA2 was associated with survival in LUAD (74). SRGN played a pivotal role in tumor-stromal interaction and reprogramming into an aggressive and immunosuppressive TME in TTF-1-negative LUAD (75). The other genes in our risk model, such as KRT18, DNAJB4, JPT1, KRT86, NUPR1, MT1X, PMAIP1, and PLBD1 had no or fewer studies reported. While we have endeavored to maintain rigor and comprehensiveness in our research, it was important to acknowledge certain limitations. Firstly, despite incorporating several independent multicenter cohorts, the necessity for further validation in a prospective study was evident. Secondly, some of the 23 CD8TRPGs that constitute the riskScore have been frequently featured in various LUAD prognostic signatures, underscoring their consistent prognostic value. For instance, LDHA was also identified as one of the genes within a disulfidoptosis signature in LUAD (76). KRT18 was also identified as one of the genes within a programmed cell death signature in LUAD (77). DDIT4 was also identified as one of the genes within a TKI resistant-based prognostic immune-related gene signature in LUAD (13). BTG2 was also identified as one of the genes within a novel mTOR-associated gene signature for predicting prognosis and evaluating tumor immune microenvironment in LUAD (78). The identification of common genes, such as LDHA, KRT18, DDIT4, and BTG2, across multiple prognostic models highlights their consistent prognostic value in LUAD. Despite the different methodologies and focus of these studies, the recurrent inclusion of these genes underscores their reliability as prognostic markers and supports the validity of our findings. This also highlighted the potential complementarity of these prognostic models, suggesting that integrating our riskScore with other models could provide a more comprehensive and accurate assessment of LUAD prognosis. Furthermore, our study extended the current understanding of the prognostic landscape in LUAD by introducing a novel CD8 T cell-related gene signature. While building upon the existing knowledge, our riskScore offered a new angle by specifically investigating the impact of the tumor immune microenvironment on LUAD prognosis. We believe that integrating our findings with those from other studies would facilitate a more comprehensive and precise prognostic assessment in LUAD. However, their specific roles in LUAD were yet to be fully understood, necessitating additional functional experimental validation. Despite these limitations, our study served as a valuable resource and a foundational proof-of-concept for future research. It paved the way for the identification of biomarkers and treatment targets, facilitating personalized therapeutic decisions for LUAD patients.
Conclusions
In conclusion, our study, utilizing 23 CD8TRPGs from the training cohort and three testing cohorts, successfully constructed and validated a consensus prognostic signature, which we termed “riskScore”. This signature was developed through 101 machine-learning algorithm combinations, proving to be a stable and effective tool for prognostic assessment. Notably, riskScore held significant clinical implications for managing and personalizing treatment for LUAD patients. It was particularly observed that patients with a lower riskScore demonstrated greater sensitivity to immunotherapy. Overall, this study presented an innovative and practical tool for prognostic evaluation, risk stratification, and tailoring individual treatments for LUAD patients in clinical settings.
Acknowledgments
We would like to express our sincere gratitude to the individuals and organizations who have contributed to the public databases that were essential for conducting this study.
Funding: None.
Footnote
Reporting Checklist: The authors have completed the TRIPOD reporting checklist. Available at https://tcr.amegroups.com/article/view/10.21037/tcr-23-2332/rc
Peer Review File: Available at https://tcr.amegroups.com/article/view/10.21037/tcr-23-2332/prf
Conflicts of Interest: All authors have completed the ICMJE uniform disclosure form (available at https://tcr.amegroups.com/article/view/10.21037/tcr-23-2332/coif). The authors have no conflicts of interest to declare.
Ethical Statement: The authors are accountable for all aspects of the work in ensuring that questions related to the accuracy or integrity of any part of the work are appropriately investigated and resolved. This study was conducted in accordance with the Declaration of Helsinki (as revised in 2013).
Open Access Statement: This is an Open Access article distributed in accordance with the Creative Commons Attribution-NonCommercial-NoDerivs 4.0 International License (CC BY-NC-ND 4.0), which permits the non-commercial replication and distribution of the article with the strict proviso that no changes or edits are made and the original work is properly cited (including links to both the formal publication through the relevant DOI and the license). See: https://creativecommons.org/licenses/by-nc-nd/4.0/.
References
- Siegel RL, Giaquinto AN, Jemal A. Cancer statistics, 2024. CA Cancer J Clin 2024;74:12-49. [Crossref] [PubMed]
- Voruganti T, Soulos PR, Mamtani R, et al. Association Between Age and Survival Trends in Advanced Non-Small Cell Lung Cancer After Adoption of Immunotherapy. JAMA Oncol 2023;9:334-41. [Crossref] [PubMed]
- Lin JJ, Cardarella S, Lydon CA, et al. Five-Year Survival in EGFR-Mutant Metastatic Lung Adenocarcinoma Treated with EGFR-TKIs. J Thorac Oncol 2016;11:556-65. [Crossref] [PubMed]
- Wu J, Li L, Zhang H, et al. A risk model developed based on tumor microenvironment predicts overall survival and associates with tumor immunity of patients with lung adenocarcinoma. Oncogene 2021;40:4413-24. [Crossref] [PubMed]
- Potter AL, Costantino CL, Suliman RA, et al. Recurrence After Complete Resection for Non-Small Cell Lung Cancer in the National Lung Screening Trial. Ann Thorac Surg 2023;116:684-92. [Crossref] [PubMed]
- Giles JR, Globig AM, Kaech SM, et al. CD8(+) T cells in the cancer-immunity cycle. Immunity 2023;56:2231-53. [Crossref] [PubMed]
- Wu F, Fan J, He Y, et al. Single-cell profiling of tumor heterogeneity and the microenvironment in advanced non-small cell lung cancer. Nat Commun 2021;12:2540. [Crossref] [PubMed]
- Maimela NR, Liu S, Zhang Y. Fates of CD8+ T cells in Tumor Microenvironment. Comput Struct Biotechnol J 2018;17:1-13. [Crossref] [PubMed]
- Comprehensive molecular profiling of lung adenocarcinoma. Nature 2014;511:543-50. [Crossref] [PubMed]
- Xu JY, Zhang C, Wang X, et al. Integrative Proteomic Characterization of Human Lung Adenocarcinoma. Cell 2020;182:245-261.e17. [Crossref] [PubMed]
- Jones GD, Brandt WS, Shen R, et al. A Genomic-Pathologic Annotated Risk Model to Predict Recurrence in Early-Stage Lung Adenocarcinoma. JAMA Surg 2021;156:e205601. [Crossref] [PubMed]
- Zhang Z, Zhu H, Wang X, et al. A novel basement membrane-related gene signature for prognosis of lung adenocarcinomas. Comput Biol Med 2023;154:106597. [Crossref] [PubMed]
- Shi Y, Xu Y, Xu Z, et al. TKI resistant-based prognostic immune related gene signature in LUAD, in which FSCN1 contributes to tumor progression. Cancer Lett 2022;532:215583. [Crossref] [PubMed]
- Liao K, Yang Q, Xu Y, et al. Identification of signature of tumor-infiltrating CD8 T lymphocytes in prognosis and immunotherapy of colon cancer by machine learning. Clin Immunol 2023;257:109811. [Crossref] [PubMed]
- Bhattacharjee J, Kirby M, Softic S, et al. Hepatic Natural Killer T-cell and CD8+ T-cell Signatures in Mice with Nonalcoholic Steatohepatitis. Hepatol Commun 2017;1:299-310. [Crossref] [PubMed]
- Okayama H, Kohno T, Ishii Y, et al. Identification of genes upregulated in ALK-positive and EGFR/KRAS/ALK-negative lung adenocarcinomas. Cancer Res 2012;72:100-11. [Crossref] [PubMed]
- Bild AH, Yao G, Chang JT, et al. Oncogenic pathway signatures in human cancers as a guide to targeted therapies. Nature 2006;439:353-7. [Crossref] [PubMed]
- Jung H, Kim HS, Kim JY, et al. DNA methylation loss promotes immune evasion of tumours with high mutation and copy number load. Nat Commun 2019;10:4278. [Crossref] [PubMed]
- Schabath MB, Welsh EA, Fulp WJ, et al. Differential association of STK11 and TP53 with KRAS mutation-associated gene expression, proliferation and immune surveillance in lung adenocarcinoma. Oncogene 2016;35:3209-16. [Crossref] [PubMed]
- Mariathasan S, Turley SJ, Nickles D, et al. TGFβ attenuates tumour response to PD-L1 blockade by contributing to exclusion of T cells. Nature 2018;554:544-8. [Crossref] [PubMed]
- Han Y, Wang Y, Dong X, et al. TISCH2: expanded datasets and new tools for single-cell transcriptome analyses of the tumor microenvironment. Nucleic Acids Res 2023;51:D1425-31. [Crossref] [PubMed]
- Stuart T, Butler A, Hoffman P, et al. Comprehensive Integration of Single-Cell Data. Cell 2019;177:1888-1902.e21. [Crossref] [PubMed]
- Wu Y, Yang S, Ma J, et al. Spatiotemporal Immune Landscape of Colorectal Cancer Liver Metastasis at Single-Cell Level. Cancer Discov 2022;12:134-53. [Crossref] [PubMed]
- Li T, Fu J, Zeng Z, et al. TIMER2.0 for analysis of tumor-infiltrating immune cells. Nucleic Acids Res 2020;48:W509-14. [Crossref] [PubMed]
- Chen B, Khodadoust MS, Liu CL, et al. Profiling Tumor Infiltrating Immune Cells with CIBERSORT. Methods Mol Biol 2018;1711:243-59. [Crossref] [PubMed]
- Finotello F, Mayer C, Plattner C, et al. Molecular and pharmacological modulators of the tumor immune contexture revealed by deconvolution of RNA-seq data. Genome Med 2019;11:34. [Crossref] [PubMed]
- Becht E, Giraldo NA, Lacroix L, et al. Estimating the population abundance of tissue-infiltrating immune and stromal cell populations using gene expression. Genome Biol 2016;17:218. [Crossref] [PubMed]
- Aran D, Hu Z, Butte AJ. xCell: digitally portraying the tissue cellular heterogeneity landscape. Genome Biol 2017;18:220. [Crossref] [PubMed]
- Racle J, Gfeller D. EPIC: A Tool to Estimate the Proportions of Different Cell Types from Bulk Gene Expression Data. Methods Mol Biol 2020;2120:233-48. [Crossref] [PubMed]
- Van Allen EM, Miao D, Schilling B, et al. Genomic correlates of response to CTLA-4 blockade in metastatic melanoma. Science 2015;350:207-11. [Crossref] [PubMed]
- Hugo W, Zaretsky JM, Sun L, et al. Genomic and Transcriptomic Features of Response to Anti-PD-1 Therapy in Metastatic Melanoma. Cell 2016;165:35-44. [Crossref] [PubMed]
- Mootha VK, Lindgren CM, Eriksson KF, et al. PGC-1alpha-responsive genes involved in oxidative phosphorylation are coordinately downregulated in human diabetes. Nat Genet 2003;34:267-73. [Crossref] [PubMed]
- Subramanian A, Tamayo P, Mootha VK, et al. Gene set enrichment analysis: a knowledge-based approach for interpreting genome-wide expression profiles. Proc Natl Acad Sci U S A 2005;102:15545-50. [Crossref] [PubMed]
- Iorio F, Knijnenburg TA, Vis DJ, et al. A Landscape of Pharmacogenomic Interactions in Cancer. Cell 2016;166:740-54. [Crossref] [PubMed]
- Yang W, Soares J, Greninger P, et al. Genomics of Drug Sensitivity in Cancer (GDSC): a resource for therapeutic biomarker discovery in cancer cells. Nucleic Acids Res 2013;41:D955-61. [Crossref] [PubMed]
- Garnett MJ, Edelman EJ, Heidorn SJ, et al. Systematic identification of genomic markers of drug sensitivity in cancer cells. Nature 2012;483:570-5. [Crossref] [PubMed]
- Maeser D, Gruener RF, Huang RS. oncoPredict: an R package for predicting in vivo or cancer patient drug response and biomarkers from cell line screening data. Brief Bioinform 2021;22:bbab260. [Crossref] [PubMed]
- Wilkerson MD, Hayes DN. ConsensusClusterPlus: a class discovery tool with confidence assessments and item tracking. Bioinformatics 2010;26:1572-3. [Crossref] [PubMed]
- Barretina J, Caponigro G, Stransky N, et al. The Cancer Cell Line Encyclopedia enables predictive modelling of anticancer drug sensitivity. Nature 2012;483:603-7. [Crossref] [PubMed]
- Thorsson V, Gibbs DL, Brown SD, et al. The Immune Landscape of Cancer. Immunity 2018;48:812-830.e14. [Crossref] [PubMed]
- Siegel RL, Miller KD, Fuchs HE, et al. Cancer Statistics, 2021. CA Cancer J Clin 2021;71:7-33. [Crossref] [PubMed]
- Lung Cancer Incidence and Mortality with Extended Follow-up in the National Lung Screening Trial. J Thorac Oncol 2019;14:1732-42. [Crossref] [PubMed]
- Ghiringhelli F, Bibeau F, Greillier L, et al. Immunoscore immune checkpoint using spatial quantitative analysis of CD8 and PD-L1 markers is predictive of the efficacy of anti- PD1/PD-L1 immunotherapy in non-small cell lung cancer. EBioMedicine 2023;92:104633. [Crossref] [PubMed]
- Tostes K, Siqueira AP, Reis RM, et al. Biomarkers for Immune Checkpoint Inhibitor Response in NSCLC: Current Developments and Applicability. Int J Mol Sci 2023;24:11887. [Crossref] [PubMed]
- Rizvi NA, Hellmann MD, Snyder A, et al. Cancer immunology. Mutational landscape determines sensitivity to PD-1 blockade in non-small cell lung cancer. Science 2015;348:124-8. [Crossref] [PubMed]
- Wang S, Zhang J, He Z, et al. The predictive power of tumor mutational burden in lung cancer immunotherapy response is influenced by patients' sex. Int J Cancer 2019;145:2840-9. [Crossref] [PubMed]
- Cui C, Wang J, Fagerberg E, et al. Neoantigen-driven B cell and CD4 T follicular helper cell collaboration promotes anti-tumor CD8 T cell responses. Cell 2021;184:6101-6118.e13. [Crossref] [PubMed]
- DeBerardinis RJ, Chandel NS. Fundamentals of cancer metabolism. Sci Adv 2016;2:e1600200. [Crossref] [PubMed]
- Lane AN, Fan TW. Regulation of mammalian nucleotide metabolism and biosynthesis. Nucleic Acids Res 2015;43:2466-85. [Crossref] [PubMed]
- Finley LWS. What is cancer metabolism? Cell 2023;186:1670-88. [Crossref] [PubMed]
- Martínez-Reyes I, Chandel NS. Cancer metabolism: looking forward. Nat Rev Cancer 2021;21:669-80. [Crossref] [PubMed]
- Cristescu R, Mogg R, Ayers M, et al. Pan-tumor genomic biomarkers for PD-1 checkpoint blockade-based immunotherapy. Science 2018;362:eaar3593. [Crossref] [PubMed]
- Leader AM, Grout JA, Maier BB, et al. Single-cell analysis of human non-small cell lung cancer lesions refines tumor classification and patient stratification. Cancer Cell 2021;39:1594-1609.e12. [Crossref] [PubMed]
- Peng M, Mo Y, Wang Y, et al. Neoantigen vaccine: an emerging tumor immunotherapy. Mol Cancer 2019;18:128. [Crossref] [PubMed]
- Duffy MJ, Crown J. Biomarkers for Predicting Response to Immunotherapy with Immune Checkpoint Inhibitors in Cancer Patients. Clin Chem 2019;65:1228-38. [Crossref] [PubMed]
- Cilona M, Locatello LG, Novelli L, et al. The Mismatch Repair System (MMR) in Head and Neck Carcinogenesis and Its Role in Modulating the Response to Immunotherapy: A Critical Review. Cancers (Basel) 2020;12:3006. [Crossref] [PubMed]
- Mao X, Xu J, Wang W, et al. Crosstalk between cancer-associated fibroblasts and immune cells in the tumor microenvironment: new findings and future perspectives. Mol Cancer 2021;20:131. [Crossref] [PubMed]
- Zeng Q, Saghafinia S, Chryplewicz A, et al. Aberrant hyperexpression of the RNA binding protein FMRP in tumors mediates immune evasion. Science 2022;378:eabl7207. [Crossref] [PubMed]
- Charoentong P, Finotello F, Angelova M, et al. Pan-cancer Immunogenomic Analyses Reveal Genotype-Immunophenotype Relationships and Predictors of Response to Checkpoint Blockade. Cell Rep 2017;18:248-62. [Crossref] [PubMed]
- Lin X, Lin K, Lin C, et al. Prognostic and clinicopathological utility of PD-L2 expression in patients with digestive system cancers: A meta-analysis. Int Immunopharmacol 2020;88:106946. [Crossref] [PubMed]
- Yearley JH, Gibson C, Yu N, et al. PD-L2 Expression in Human Tumors: Relevance to Anti-PD-1 Therapy in Cancer. Clin Cancer Res 2017;23:3158-67. [Crossref] [PubMed]
- Yu J, Zhuang A, Gu X, et al. Nuclear PD-L1 promotes EGR1-mediated angiogenesis and accelerates tumorigenesis. Cell Discov 2023;9:33. [Crossref] [PubMed]
- Gao Y, Nihira NT, Bu X, et al. Acetylation-dependent regulation of PD-L1 nuclear translocation dictates the efficacy of anti-PD-1 immunotherapy. Nat Cell Biol 2020;22:1064-75. [Crossref] [PubMed]
- Zhou Y, Guo Y, Ran M, et al. Combined inhibition of pyruvate dehydrogenase kinase 1 and lactate dehydrogenase a induces metabolic and signaling reprogramming and enhances lung adenocarcinoma cell killing. Cancer Lett 2023;577:216425. [Crossref] [PubMed]
- Wang Z, Li Z, Zhou K, et al. Deciphering cell lineage specification of human lung adenocarcinoma with single-cell RNA sequencing. Nat Commun 2021;12:6500. [Crossref] [PubMed]
- Chen H, Chen X, Pan B, et al. KRT8 Serves as a Novel Biomarker for LUAD and Promotes Metastasis and EMT via NF-κB Signaling. Front Oncol 2022;12:875146. [Crossref] [PubMed]
- Zhang H, Yan X, Gu H, et al. High SERPINH1 expression predicts poor prognosis in lung adenocarcinoma. J Thorac Dis 2022;14:4785-802. [Crossref] [PubMed]
- Liu T, Han C, Fang P, et al. Cancer-associated fibroblast-specific lncRNA LINC01614 enhances glutamine uptake in lung adenocarcinoma. J Hematol Oncol 2022;15:141. [Crossref] [PubMed]
- Zong S, Jiao Y, Liu X, et al. FKBP4 integrates FKBP4/Hsp90/IKK with FKBP4/Hsp70/RelA complex to promote lung adenocarcinoma progression via IKK/NF-κB signaling. Cell Death Dis 2021;12:602. [Crossref] [PubMed]
- Liu XS, Yuan LL, Gao Y, et al. DARS2 overexpression is associated with PET/CT metabolic parameters and affects glycolytic activity in lung adenocarcinoma. J Transl Med 2023;21:574. [Crossref] [PubMed]
- Li L, Yang L, Fan Z, et al. Hypoxia-induced GBE1 expression promotes tumor progression through metabolic reprogramming in lung adenocarcinoma. Signal Transduct Target Ther 2020;5:54. [Crossref] [PubMed]
- Aluksanasuwan S, Somsuan K, Ngoenkam J, et al. Potential association of HSPD1 with dysregulations in ribosome biogenesis and immune cell infiltration in lung adenocarcinoma: An integrated bioinformatic approach. Cancer Biomark 2024;39:155-70. [Crossref] [PubMed]
- Yang W, Wei C, Cheng J, et al. BTG2 and SerpinB5, a novel gene pair to evaluate the prognosis of lung adenocarcinoma. Front Immunol 2023;14:1098700. [Crossref] [PubMed]
- Zhang L, Zhang Y, Wang C, et al. Integrated single-cell RNA sequencing analysis reveals distinct cellular and transcriptional modules associated with survival in lung cancer. Signal Transduct Target Ther 2022;7:9. [Crossref] [PubMed]
- Tanaka I, Dayde D, Tai MC, et al. SRGN-Triggered Aggressive and Immunosuppressive Phenotype in a Subset of TTF-1-Negative Lung Adenocarcinomas. J Natl Cancer Inst 2022;114:290-301. [Crossref] [PubMed]
- He D, Tang H, Yang X, et al. Elaboration and validation of a prognostic signature associated with disulfidoptosis in lung adenocarcinoma, consolidated with integration of single-cell RNA sequencing and bulk RNA sequencing techniques. Front Immunol 2023;14:1278496. [Crossref] [PubMed]
- Wang S, Wang R, Hu D, et al. Machine learning reveals diverse cell death patterns in lung adenocarcinoma prognosis and therapy. NPJ Precis Oncol 2024;8:49. [Crossref] [PubMed]
- Zheng Z, Li Y, Lu X, et al. A novel mTOR-associated gene signature for predicting prognosis and evaluating tumor immune microenvironment in lung adenocarcinoma. Comput Biol Med 2022;145:105394. [Crossref] [PubMed]