SCG2 and CPE may be novel markers for the identification of pancreatic neuroendocrine tumors and solid pseudopapillary neoplasms
Highlight box
Key findings
• Secretogranin II (SCG2) and carboxypeptidase E (CPE) were identified as novel and effective markers for differentiating pancreatic neuroendocrine tumors (pNETs) and solid pseudopapillary neoplasms (SPNs), surpassing traditional markers like β-catenin and chromogranin A (CgA).
What is known and what is new?
• It is crucial to accurately differentiate between pNETs and SPNs due to significant differences in treatment and prognosis. However, due to their similar pathological features, traditional markers are unable to provide an accurate differential diagnosis.
• There have been no studies on integrating bioinformatics analysis with immunohistochemistry to seek new makers for distinguishing between pNETs and SPNs.
What is the implication, and what should change now?
• SCG2 and CPE were identified as novel and effective markers for differentiating pNETs and SPNs through bioinformatics analysis and immunohistochemical validation. These markers demonstrated high sensitivity (98.7% for SCG2, 97.4% for CPE) and specificity (100%) surpassing traditional markers like β-catenin and CgA.
Introduction
Distinguishing pancreatic neuroendocrine tumors (pNETs) from solid pseudopapillary neoplasms (SPNs) based on morphology alone is challenging, especially when limited pathological tissue is available, such as from needle biopsies. This difficulty stems from their similar histopathological features. Accurate differentiation is crucial due to the significant treatment and prognosis differences between pNETs and SPNs. SPN patients exhibit malignant behavior in 9–28% of cases, yet the 5-year survival rate following radical surgical resection exceeds 95% (1,2). In contrast, pNETs, more malignant in nature, often require endocrine therapy or chemotherapy post-surgery, with a 5-year survival rate around 85.4% (3). Immunohistochemistry, a prevalent technique for differentiating between these tumors, faces challenges due to overlapping antibody staining profiles with conventional markers like synaptophysin, β-catenin, and vimentin, leading to limited diagnostic sensitivity and specificity (4). Recent research has focused on identifying novel biomarkers to improve differentiation (5,6). Some studies advocate for using a combination of multiple biomarkers to enhance diagnostic reliability (7,8). However, the scarcity of validated samples and limited evidence necessitate further research in this area. Given the ongoing debate over the most effective immunohistochemical markers for these tumor types, this study investigates the gene expression profiles of pNETs, SPNs, and normal pancreatic tissues, using data from the Gene Expression Omnibus (GEO) database. Our aim is to identify key genes with distinct expression patterns in pNETs and SPNs, which could serve as genetic-level discriminators. These potential biomarkers are further validated through immunohistochemical analysis. We present this article in accordance with the REMARK reporting checklist (available at https://tcr.amegroups.com/article/view/10.21037/tcr-24-229/rc).
Methods
Data source and data processing
The gene chip dataset GSE43795, obtained from the GEO database (platform GPL10558) (9), includes 14 SPN cases, six pancreatic cancer cases, six pNET cases, and five normal pancreatic tissue samples. We normalized the gene expression data for SPNs, pNETs, and normal pancreas samples using the “FactoMineR” and “factoextra” packages in R software (version 4.1.2). Principal component analysis (PCA) was conducted to analyze and visualize the gene expression patterns and differences between groups.
Identification of differential expression genes (DEGs) in SPNs and pNETs
Differential gene expression analysis was carried out using the “limma” package (R version 4.1.2). This analysis included 14 SPN cases, six pNET cases, and five normal pancreatic tissue samples, with the screening criteria set to |log2fold change (FC)| >2 and P<0.05. The results were visualized using the “ggplot2” package (R version 4.1.2), and DEGs in pNETs were identified by comparing expression levels to SPNs and normal pancreatic tissue groups.
Enrichment analysis of DEGs
Enrichment analysis on the DEGs was performed using the “ClusterProfiler” package (R version 4.1.2). This involved Gene Ontology (GO) analysis for biological processes (BPs), cellular components (CCs), and molecular functions (MFs), as well as Kyoto Encyclopedia of Genes and Genomes (KEGG) analysis. The top 5 pathways were identified and visualized using the “GOplot” and “ggnewscale” packages (R version 4.1.2).
Protein-protein interaction (PPI) analysis of DEGs
Protein interactions of the DEGs in pNETs were analyzed using the STRING online database (https://string-db.org/) with a confidence level of 0.4. The network was visualized using Cytoscape software, and the cytoHubba plugin helped identify the top 10 key DEGs based on network positions. The top 3 key genes were selected for further immunohistochemical validation.
Validation by immunohistochemistry
Patient selection
We enrolled 78 pNET patients and 85 SPN patients treated at the Fourth Hospital of Hebei Medical University from January 2017 to June 2021. Each patient had a single pancreatic lesion surgically removed, with a definitive diagnosis made by two chief physicians based on pathological results. The study was conducted in accordance with the Declaration of Helsinki (as revised in 2013). The study was approved by the Institutional Research Ethics Committee at the Fourth Hospital of Hebei Medical University (No. 2022KY234) and informed consent was taken from all the patients.
Immunohistochemistry
The tumor samples were fixed in 3.7% paraformaldehyde for 24 hours, dehydrated, embedded in paraffin, and then sectioned into 4-µm thick slices. These sections were heated at 75 ℃ for 2 hours, dewaxed in xylene, and rehydrated through a graded alcohol series. Antigen retrieval was performed using ethylenediaminetetraacetic acid (EDTA) buffer (MaxVision MVS-0098/0099, diluted 1:50). To suppress endogenous peroxidase, 3% H2O2 was applied, followed by blocking non-specific staining with goat serum for 20 minutes. Primary antibodies (details in Table S1) were applied and incubated at room temperature for 2 hours, then overnight at 4 ℃. Secondary antibodies [horseradish peroxidase (HRP)-polymer anti-mouse/rabbit or mouse/rabbit immunoglobulin G (IgG), MaxVision, number: 231109S407n] were added for 30 minutes at room temperature, followed by development with 3,3’-diaminobenzidine (DAB) chromogenic solution (MaxVision Fuzhou), counterstaining with hematoxylin (LEICA auto STAINER XL CV5030), dehydration in graded ethanol, clearing in xylene, and mounting with neutral rubber.
Assessment of immunohistochemical staining
Two independent pathologists evaluated the immunohistochemical staining. They examined 10 high-power fields (200× magnification) per specimen, assessing 100 tumor cells to determine the average percentage of positively stained cells. Cytoplasmic staining was scored as 0 (no staining), 1 (weak), 2 (moderate), or 3 (strong). The percentage of positive cells was categorized into 0 (0–5%), 1 (6–25%), 2 (26–50%), or 3 (>50%). The final staining score, a product of intensity and percentage scores, determined expression levels: scores above 3 indicated high expression, and scores of 3 or below suggested low expression. For nuclear staining, positivity rates over 30% were considered positive, while rates below 30% were negative. Retrieved immunohistochemical stains from the archive include β-catenin, vimentin, chromogranin A (CgA, CHGA).
Clinical statistical analysis
Data analysis was conducted using SPSS 21.0. For numerical variables, the t-test was applied for comparisons between two groups when data conformed to a normal distribution and passed the homogeneity of variance test. The Wilcoxon test was used if the data did not conform to a normal distribution. Categorical variables were analyzed using the chi-squared test, chi-squared test with Yates’ correction, or Fisher’s exact test as appropriate. The Kaplan-Meier method and log-rank test were utilized for survival analysis. A P value <0.05 was considered statistically significant.
Results
Quality control of samples
The GSE43795 dataset, containing samples from pNETs, SPNs, and normal pancreatic tissues, underwent normalization to ensure uniform data distribution, as verified by a box plot (Figure 1A). Additionally, PCA was used for cluster analysis, revealing clear clustering within each group and distinct separations between them (Figure 1B).
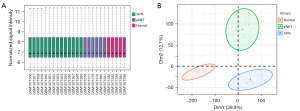
DEGs screening
Comparative analysis between pNET and SPN groups identified 491 DEGs. Among these, 397 genes were upregulated in pNETs compared to SPNs (Figure 2A). A comparison between pNETs and normal pancreatic tissues revealed 211 upregulated and 299 downregulated genes in pNETs (Figure 2B). We utilized a Venn diagram (Figure 2C) to identify the overlap of upregulated genes in pNETs against both normal pancreatic tissues and SPNs, resulting in 121 DEGs uniquely associated with pNETs.
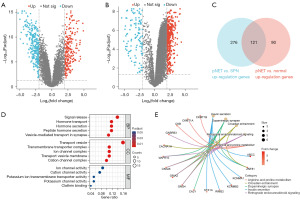
DEGs enrichment analysis
GO analysis
DEGs in pNETs predominantly exhibited enrichment in processes like signal release, vesicle transport, and ion pathway activation (Figure 2D).
KEGG analysis
DEGs specific to pNETs were mainly linked to pathways involved in insulin secretion, dopamine synaptic functions, and circadian rhythms (Figure 2E).
PPI analysis of DEG-related proteins in pNETs
The PPI network analysis focused on proteins related to DEGs in pNETs, using the STRING database. The data were visualized with Cytoscape software (Figure 3). The top ten most significant DEGs were identified using the CytoHubba plug-in, from which three hub genes—CHGA, carboxypeptidase E (CPE), and secretogranin II (SCG2)—were selected for further validation via immunohistochemistry.
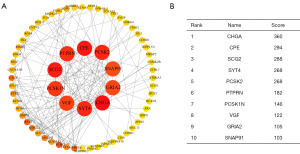
Immunohistochemical validation of proteins corresponding to hub genes
Clinical characteristics
The study involved 78 pNET patients (39 males, 39 females, average age 55 years) and 85 SPN patients (13 males, 72 females, average age 28 years). General characteristics are presented in Table 1.
Table 1
Characteristics | pNETs (n=78) | SPNs (n=85) | P value |
---|---|---|---|
Sex | <0.001 | ||
Female | 39 (50.0) | 72 (84.7) | |
Male | 39 (50.0) | 13 (15.3) | |
Age (years) | 55 [45.5, 63.5] | 28 [19, 43] | <0.001 |
Symptom | 0.001 | ||
Asymptomatic | 32 (41.0) | 48 (56.5) | |
Abdominal pain | 33 (42.3) | 21 (24.7) | |
Diarrhea | 3 (3.8) | 0 (0.0) | |
Abdominal distension | 2 (2.6) | 12 (14.1) | |
Apsychia | 4 (5.1) | 0 (0.0) | |
Others | 4 (5.1) | 4 (4.7) | |
Location | 0.36 | ||
Body | 32 (41.0) | 29 (34.1) | |
Head | 27 (34.6) | 25 (29.4) | |
Tail | 19 (24.4) | 31 (36.5) | |
Surgical methods | 0.78 | ||
Laparoscope | 25 (32.1) | 29 (34.1) | |
Open | 53 (67.9) | 56 (65.9) | |
Tumor size (cm) | <0.001 | ||
≤2 | 18 (23.1) | 6 (7.1) | |
>2 and ≤4 | 40 (51.3) | 22 (25.9) | |
>4 | 20 (25.6) | 57 (67.1) | |
Lymph node metastasis | <0.001 | ||
No | 67 (85.9) | 85 (100.0) | |
Yes | 11 (14.1) | 0 (0.0) | |
Neutrophil (×109) | 3.665 [2.72, 4.9025] | 3.68 [2.87, 5.11] | 0.63 |
Lymphocyte (×109) | 1.435 [1.2025, 1.815] | 1.64 [1.3, 1.92] | 0.12 |
CEA (U/mL) | 1.97 [1.46, 2.815] | 1.23 [0.88, 1.92] | <0.001 |
CA-199 (U/mL) | 12.045 [8.765, 19.885] | 8.76 [6.12, 16.32] | 0.003 |
Ki67 (%) | <0.001 | ||
<3 | 21 (26.9) | 49 (57.6) | |
≥3 and ≤20 | 48 (61.5) | 35 (41.2) | |
>20 | 9 (11.5) | 1 (1.2) | |
Recurrent status | <0.001 | ||
No | 59 (75.6) | 85 (100.0) | |
Yes | 19 (24.4) | 0 (0.0) | |
Survival status | 0.02 | ||
Alive | 71 (91.0) | 85 (100.0) | |
Dead | 7 (9.0) | 0 (0.0) |
Data are presented as n (%) or median [interquartile range]. SPN, solid pseudopapillary neoplasm; pNET, pancreatic neuroendocrine tumor; CEA, carcinoembryonic antigen; CA-199, carbohydrate antigen-199.
Follow-up data
Follow-up data collection continued until November 2021. Among the 85 SPN patients, no recurrence or mortality was reported. In contrast, 19 out of 78 pNET patients experienced disease recurrence, with seven deaths. The SPN group showed significantly better disease-free survival (DFS) and overall survival (OS) compared to the pNET group. DFS and OS curves for both groups are depicted in Figure 4.
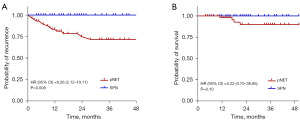
Immunohistochemical analysis
SCG2, CPE, and CHGA staining were performed in pancreatic tumors (Figure 5). In pNET samples, sensitivity were 98.7% for SCG2, 97.4% for CPE, and 88.5% for CHGA. SPN samples showed negative staining for all three antibodies. Additionally, classical immunohistochemical markers [β-catenin, lymphoid enhancer-binding factor 1 (LEF1), vimentin] were used for differential staining of pNETs and SPNs (Figure 6). The sensitivity of these markers was 96.5% for β-catenin, 84.7% for LEF1, and 97.6% for vimentin, with specificities of 87.2%, 83.3%, and 78.2%, respectively. Comprehensive details are presented in Table 2.
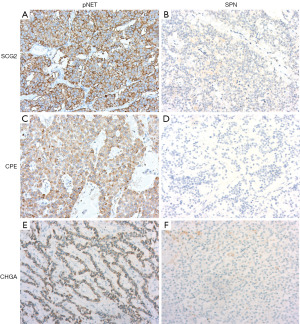
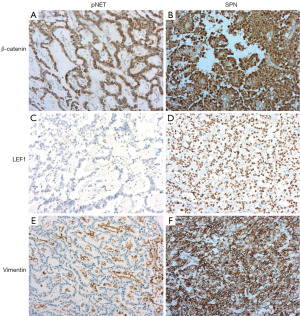
Table 2
Biomarker | SCG2 | CPE | CHGA | β-catenin | LEF1 | Vimentin |
---|---|---|---|---|---|---|
pNET (−) | 1 | 2 | 9 | 68 | 65 | 61 |
pNET (+) | 77 | 76 | 69 | 10 | 13 | 17 |
SPN (−) | 85 | 85 | 85 | 3 | 13 | 2 |
SPN (+) | 0 | 0 | 0 | 82 | 72 | 83 |
Sensitivity (%) | 98.7 | 97.4 | 88.5 | 96.5 | 84.7 | 97.6 |
Specificity (%) | 100 | 100 | 100 | 87.2 | 83.3 | 78.2 |
Youden index | 0.987 | 0.974 | 0.885 | 0.837 | 0.680 | 0.758 |
SCG2, secretogranin II; CPE, carboxypeptidase E; CHGA, chromogranin A; LEF1, lymphoid enhancer-binding factor 1; pNET, pancreatic neuroendocrine tumor; SPN, solid pseudopapillary neoplasm.
Discussion
Both pNETs and SPNs are rare pancreatic tumors characterized by diverse shapes and overlapping morphologies, posing significant challenges in differential diagnosis. Accurate diagnosis is crucial due to the distinct prognosis and treatment strategies for each. Immunohistochemical staining is currently the most effective method to distinguish between pNETs and SPNs. In our study, bioinformatics analysis was employed to select DEGs in pNETs. Key DEGs associated with pNETs were identified through PPI network analysis, providing a reference for novel immunohistochemical markers. Specific DEGs in pNETs, identified via KEGG analysis, were primarily associated with signaling pathways including insulin secretion, dopamine synapse, and circadian rhythm. Notably, key genes CHGA, SCG2, and CPE, involved in hormone peptide regulation, were identified. Considering pNETs originate from endocrine cells, screening diagnostic markers around neurohormone secretion-related pathway genes is vital. The CHGA gene encodes CgA, a subtype of chromogranin granules in neuroendocrine cells critical for secretory granule production, hormone accumulation, and calcium homeostasis. CgA is a classic immunohistochemical marker for pNETs diagnosis, widely used in clinical practice. A meta-analysis of 1,260 neuroendocrine tumor (NET) cases and 967 normal tissue cases reported CgA’s sensitivity at 73% and specificity at 95% for NETs diagnosis (10). Literature suggests CgA’s sensitivity at 66%, specificity at 95%, and overall accuracy at 71% in pNETs diagnosis (11). In our study, CgA showed a sensitivity of 88.5% and specificity of 100%, consistent with previous research. High CgA expression in pNET patients correlates with poorer biological characteristics and reduced postoperative survival (12-15). However, external factors like liver cirrhosis and oral proton pump inhibitors can influence CgA expression (16). Therefore, it is advisable to analyze CgA alongside other markers for accurate pNETs differential diagnosis.
SCG2, a member of the secretogranin family, plays a vital role in secreting neuropeptides like EM66, which are key in enhancing neurotransmission, inflammatory responses, endothelial cell proliferation, and angiogenesis (17,18). Recent studies have demonstrated that SCG2 can serve as a prognostic marker for malignant tumors (19,20). Another significant marker, CPE, functions as a neuropeptide processing enzyme within the M14 family of metal carboxypeptidases and is essential in activating hormones involved in various BPs (21). Currently, there is no relevant literature on the use of SCG2, CPE, as immunohistochemical markers for identifying pNETs and SPNs. Our study indicates that both SCG2 and CPE have a higher discriminative power in differentiating these two tumor types. Notably, despite being cytoplasmic stains, SCG2 and CPE demonstrated superior differentiation capabilities when compared to conventional markers like β-catenin, LEF1, and vimentin.
In our study, β-catenin exhibited high specificity but also showed positive cytoplasmic staining in some pNET samples, as illustrated in Figure 6A. We quantified this non-specific staining intensity, which was found to be as high as 40%. Consequently, the use of β-catenin as a differential marker becomes challenging, especially with limited or lower-quality samples like those obtained from biopsy pathology. LEF1, functioning as a downstream target gene of β-catenin in the Wnt signaling pathway, is known to enhance Wnt signaling (22). LEF1’s nuclear staining pattern offers an advantage over β-catenin by minimizing the diagnostic interference from non-specific cytoplasmic staining, suggesting its potential as an effective marker for distinguishing between SPNs and pNETs (23). In our study, LEF1 showed superior nuclear staining compared to β-catenin and did not exhibit non-specific cytoplasmic staining, as depicted in Figure 6D. However, its sensitivity and specificity were 84.7% and 83.3%, respectively, which are lower than those of SCG2 and CPE. SPNs are classically characterized by mutations in the CTNNB1 gene’s third exon, leading to β-catenin protein phosphorylation evasion in the cytoplasm, its accumulation in the nucleus and cytoplasm, and activation of the Wnt signaling pathway (24). This provides the rationale for using Wnt pathway molecules (LEF1, β-catenin) in diagnosing SPNs. However, the Wnt pathway is also implicated in various other tumors, including pNETs (25), which could explain the strong LEF1 and β-catenin staining observed in some pNETs. In contrast, SCG2 and CPE, associated with endocrine secretion, demonstrate more specificity. Bioinformatics analysis reveals their high expression in pNETs and significant underexpression in SPNs, providing a theoretical basis for employing SCG2 and CPE as diagnostic markers. This evidence suggests their greater reliability and trustworthiness as markers for differential diagnosis.
The novelty of this research lies in employing bioinformatics analysis to identify novel diagnostic markers for pNETs and SPNs at a genetic level, followed by their immunohistochemical validation. We have found that CPE and SCG2 can effectively differentiate between these tumor types have shown promising sensitivity and specificity. However, this study has certain limitations. Being conducted at a single center, its results require further confirmation through multicenter prospective studies. Additionally, the specific roles of CPE and SCG2 in pNETs have yet to be fully elucidated. Future experiments are required to validate the functions of these proteins in pNETs.
Conclusions
In conclusion, SCG2 and CPE are effective, novel immunohistochemical biomarkers for differentiating pNETs from SPNs. They showed superior differentiation capabilities when compared to conventional markers like β-catenin, LEF1, and vimentin.
Acknowledgments
We would like to thank Dr. Huichai Yang (pathologist in the Fourth Hospital of Hebei Medical University) for the technical guidance on immunohistochemical staining.
Funding: This study was supported by
Footnote
Reporting Checklist: The authors have completed the REMARK reporting checklist (Available at https://tcr.amegroups.com/article/view/10.21037/tcr-24-229/rc).
Data Sharing Statement: Available at https://tcr.amegroups.com/article/view/10.21037/tcr-24-229/dss
Peer Review File: Available at https://tcr.amegroups.com/article/view/10.21037/tcr-24-229/prf
Conflicts of Interest: All authors have completed the ICMJE uniform disclosure form (available at https://tcr.amegroups.com/article/view/10.21037/tcr-24-229/coif). The authors have no conflicts of interest to declare.
Ethical Statement: The authors are accountable for all aspects of the work in ensuring that questions related to the accuracy or integrity of any part of the work are appropriately investigated and resolved. The study was conducted in accordance with the Declaration of Helsinki (as revised in 2013). The study was approved by the Institutional Research Ethics Committee at the Fourth Hospital of Hebei Medical University (No. 2022KY234) and informed consent was taken from all the patients.
Open Access Statement: This is an Open Access article distributed in accordance with the Creative Commons Attribution-NonCommercial-NoDerivs 4.0 International License (CC BY-NC-ND 4.0), which permits the non-commercial replication and distribution of the article with the strict proviso that no changes or edits are made and the original work is properly cited (including links to both the formal publication through the relevant DOI and the license). See: https://creativecommons.org/licenses/by-nc-nd/4.0/.
References
- Lim SY, Chae H, Jeong H, et al. Twenty-one years of experience with resected solid pseudopapillary neoplasm: a retrospective single-institutional cohort study. J Gastrointest Surg 2024;28:226-31. [Crossref] [PubMed]
- Liu Q, Dai M, Guo J, et al. Long-term Survival, Quality of Life, and Molecular Features of the Patients With Solid Pseudopapillary Neoplasm of the Pancreas: A Retrospective Study of 454 Cases. Ann Surg 2023;278:1009-17. [Crossref] [PubMed]
- Singh S, Moody L, Chan DL, et al. Follow-up Recommendations for Completely Resected Gastroenteropancreatic Neuroendocrine Tumors. JAMA Oncol 2018;4:1597-604. [Crossref] [PubMed]
- Ohara Y, Oda T, Hashimoto S, et al. Pancreatic neuroendocrine tumor and solid-pseudopapillary neoplasm: Key immunohistochemical profiles for differential diagnosis. World J Gastroenterol 2016;22:8596-604. [Crossref] [PubMed]
- Jiang Y, Xie J, Wang B, et al. TFE3 is a diagnostic marker for solid pseudopapillary neoplasms of the pancreas. Hum Pathol 2018;81:166-75. [Crossref] [PubMed]
- Dinarvand P, Wang WL, Roy-Chowdhuri S. Utility of SOX11 for the diagnosis of solid pseudopapillary neoplasm of the pancreas on cytological preparations. Cytopathology 2022;33:216-21. [Crossref] [PubMed]
- Lu H, Allende D, Liu X, et al. Lymphoid Enhancer Binding Factor 1 (LEF1) and Paired Box Gene 8 (PAX8): A Limited Immunohistochemistry Panel to Distinguish Solid Pseudopapillary Neoplasms and Pancreatic Neuroendocrine Tumors. Appl Immunohistochem Mol Morphol 2020;28:776-80. [Crossref] [PubMed]
- Guo W, Farzaneh T, Lee W, et al. A limited panel of INSM1 and LEF1 immunostains accurately distinguishes between pancreatic neuroendocrine tumor and solid pseudopapillary neoplasm. Pathol Res Pract 2021;223:153462. [Crossref] [PubMed]
- Park M, Kim M, Hwang D, et al. Characterization of gene expression and activated signaling pathways in solid-pseudopapillary neoplasm of pancreas. Mod Pathol 2014;27:580-93. [Crossref] [PubMed]
- Yang X, Yang Y, Li Z, et al. Diagnostic value of circulating chromogranin a for neuroendocrine tumors: a systematic review and meta-analysis. PLoS One 2015;10:e0124884. [Crossref] [PubMed]
- Pulvirenti A, Rao D, Mcintyre CA, et al. Limited role of Chromogranin A as clinical biomarker for pancreatic neuroendocrine tumors. HPB (Oxford) 2019;21:612-8. [Crossref] [PubMed]
- Fisher AV, Lopez-Aguiar AG, Rendell VR, et al. Predictive Value of Chromogranin A and a Pre-Operative Risk Score to Predict Recurrence After Resection of Pancreatic Neuroendocrine Tumors. J Gastrointest Surg 2019;23:651-8. [Crossref] [PubMed]
- Raoof M, Jutric Z, Melstrom LG, et al. Prognostic significance of Chromogranin A in small pancreatic neuroendocrine tumors. Surgery 2019;165:760-6. [Crossref] [PubMed]
- Concors SJ, Sinnamon AJ, Ecker BL, et al. The impact of surgery for metastatic pancreatic neuroendocrine tumor: a contemporary evaluation matching for chromogranin a level. HPB (Oxford) 2020;22:83-90. [Crossref] [PubMed]
- Nielsen K, Binderup T, Langer SW, et al. P53, Somatostatin receptor 2a and Chromogranin A immunostaining as prognostic markers in high grade gastroenteropancreatic neuroendocrine neoplasms. BMC Cancer 2020;20:27. [Crossref] [PubMed]
- Di Giacinto P, Rota F, Rizza L, et al. Chromogranin A: From Laboratory to Clinical Aspects of Patients with Neuroendocrine Tumors. Int J Endocrinol 2018;2018:8126087. [Crossref] [PubMed]
- Zhou J, Dong C, Tan J, et al. Promoting effect and immunologic role of secretogranin II on bladder cancer progression via regulating MAPK and NF-κB pathways. Apoptosis 2024;29:121-41. [Crossref] [PubMed]
- Cheng X, Zhang M, Xie Y, et al. Bone marrow-derived mesenchymal stem cells accelerate angiogenesis in pregnant experimentally induced deep venous thrombosis rat model via up-regulation of pro-angiogenic secretogranin II. Int Immunopharmacol 2023;118:110025. [Crossref] [PubMed]
- Cury SS, de Moraes D, Freire PP, et al. Tumor Transcriptome Reveals High Expression of IL-8 in Non-Small Cell Lung Cancer Patients with Low Pectoralis Muscle Area and Reduced Survival. Cancers (Basel) 2019;11:1251. [Crossref] [PubMed]
- Wang H, Yin J, Hong Y, et al. SCG2 is a Prognostic Biomarker Associated With Immune Infiltration and Macrophage Polarization in Colorectal Cancer. Front Cell Dev Biol 2022;9:795133. [Crossref] [PubMed]
- Mizutani A, Inoko H, Tanaka M, Carboxypeptidase E. Identified As a Direct Interactor of Growth Hormone, Is Important for Efficient Secretion of the Hormone. Mol Cells 2016;39:756-61. [Crossref] [PubMed]
- Zhan T, Rindtorff N, Boutros M. Wnt signaling in cancer. Oncogene 2017;36:1461-73. [Crossref] [PubMed]
- Farzaneh T, Nowroozizadeh B, Han M, et al. Diagnostic Utility of LEF1 Immunostain in Cytology Specimens of Solid Pseudopapillary Neoplasm of Pancreas. Acta Cytol 2021;65:250-6. [Crossref] [PubMed]
- Wang J, Gerrard G, Poskitt B, et al. Targeted next generation sequencing of pancreatic solid pseudopapillary neoplasms show mutations in Wnt signaling pathway genes. Pathol Int 2019;69:193-201. [Crossref] [PubMed]
- Lu F, Ye M, Hu C, et al. FABP5 regulates lipid metabolism to facilitate pancreatic neuroendocrine neoplasms progression via FASN mediated Wnt/β-catenin pathway. Cancer Sci 2023;114:3553-67. [Crossref] [PubMed]