Dendritic cell-related hub genes in head-and-neck squamous cell carcinoma: implications for prognosis and immunotherapy
Highlight box
Key findings
• This study reveals the pivotal role of dendritic cells (DCs) in head-and-neck squamous cell carcinoma (HNSCC). Analyzing clinical datasets identifies 222 DC-related genes, pinpointing seven prognostic genes, including ACP2 and CPVL. Differential gene expression unveils 208 genes distinguishing ACP2+ and ACP2− DC cells. Notable findings include top transcription factors (e.g., STAT1) and genomic associations like TP53 and FAT1 mutations. Confirmed STAT1 and ACP2 correlation, along with insights into immunotherapeutic sensitivity, hints at a robust DC-based prognostic system, laying the groundwork for personalized therapeutic strategies.
What is known and what is new?
• DCs play crucial roles in antigen presentation and immune modulation in HNSCC.
• This study innovatively identifies 222 DC-related genes, seven key prognostic genes, and provides insights into differential gene expression, transcription factors, and genomic associations, enhancing our understanding of DCs in HNSCC. These findings introduce potential prognostic markers and guide personalized therapeutic approaches.
What is the implication, and what should change now?
• This research transforms HNSCC understanding, emphasizing integration of identified prognostic markers (e.g., ACP2, CPVL) for precise clinical approaches. Targeting transcription factors, notably STAT1, offers therapeutic potential. Recognizing immunotherapeutic sensitivity variations underscores the urgency of personalized treatment strategies. Immediate changes in clinical practices are urged to usher in precision medicine, promising improved outcomes in HNSCC.
Introduction
In the challenging landscape of oncology, head-and-neck squamous cell carcinoma (HNSCC) remains an enigma, and innovative solutions are required (1-3). Despite recent therapeutic advances, many patients continue to live with unfavorable prognoses (4). The nuanced dynamics of the tumor immune microenvironment (TIME) emerge as a pivotal determinant in the progression of HNSCC (5,6). Dendritic cells (DCs) serve as sentinels of the immune system and orchestrate the responses to malignant cells (7,8). This emphasizes the multifaceted roles played by DCs within the HNSCC TIME; manipulation of such cells may revolutionize therapeutic paradigms (9).
DC antigen presentation and the effects of DCs on immune checkpoint modulation are of major significance in HNSCC (10). Elucidating the functional nuances of DC subsets would help tailor therapies to shift the immune equilibrium in the desired direction (11). Recent DC-based vaccines comprising patient-derived DCs enriched with tumor-specific antigens (12-14) elicit potent anti-tumor responses while minimizing adverse effects (15). This research frontier provides a beacon of hope in the challenging battle against this formidable cancer (16,17).
In recent years, gene expression signatures associated with cancer (including HNSCC) prognoses have been used to predict patient outcomes (18,19). Such signatures identify high-risk patient subgroups that require personalized therapeutic strategies. The prognostic implications aside, exploration of the molecular mechanisms underlying the role of DCs in HNSCC progression would facilitate therapeutic interventions (20,21). However, no reliably prognostic gene signatures for HNSCC are yet available, and we have only a limited comprehension of the genetic principles governing DC behavior.
In this study, we comprehensively investigated potential drug targets within the DC gene signature to address the gaps identified above. Employing a multi-omics approach and advanced computational analyses, novel molecules and signaling networks that serve as prospective therapeutic targets for HNSCC were revealed. The establishment of a robust DC gene signature model via high-throughput genomic profiling enables precise patient stratification. Ultimately, by unraveling the molecular mechanisms and genetic drivers associated with DCs, we contribute to the development of precision medicine to enhance the prognostic assessment and treatment outcomes of HNSCC patients. We present this article in accordance with the TRIPOD reporting checklist (available at https://tcr.amegroups.com/article/view/10.21037/tcr-23-2360/rc).
Methods
Acquisition of clinical and gene expression information
In January 2023, we used the Genomic Data Commons (GDC) portal to acquire clinical and gene expression data from a The Cancer Genome Atlas (TCGA)-HNSCC cohort comprising 500 individuals. At that time, we also obtained clinical and transcriptomic data from 270 HNSCC cases in the GSE65858 cohort from the Gene Expression Omnibus database. The study was conducted in accordance with the Declaration of Helsinki (as revised in 2013).
The abundance of DCs and the application of weighted gene co-expression network analysis (WGCNA)
Quantification of DC abundance in the TCGA-HNSCC as well as GSE65858 cohorts was performed using the “xCells” package of R software (22). We acquired five different DC abundance scores for each cohort. We used the “WGCNA” package of R software to define co-expression networks and to obtain multiple estimates of DC abundance and stromal scores (23).
Random survival forest analysis, least absolute shrinkage and selection operator (LASSO) Cox’s regression model, and survival analysis
We employed random survival forest analysis to identify significantly prognostic genes using the “randomForestSRC” and “randomSurvivalForest” packages of R software (24). The LASSO Cox’s regression method was utilized to construct a prognostic model for hub genes associated with DCs, employing the “glmnet” package within the R software (25). The optimal cutoff values of the LASSO risk scores in terms of overall survival (OS) were determined for both the TCGA-HNSCC and GSE65858 cohorts using the “max stat” approach with an abseps threshold of 0.01. Subsequently, Kaplan-Meier analyses were conducted using the “survival” packages of R software.
Differential gene expression analysis
Differential expression analysis of messenger RNAs was executed utilizing the “EdgeR” package within the R software (26).
Gene set enrichment analysis (GSEA)
We built a GSEA algorithm using the “clusterProfiler” package of R software. This revealed normalized enrichment scores and their statistical significance across various gene sets, including the ontology and Hallmark gene sets, together with Kyoto Encyclopedia of Genes and Genomes (KEGG) pathway terms (27).
Somatic mutation analysis
In March 2023, we utilized cBioPortal to obtain the top 200 somatic mutations with the highest frequency in the TCGA-HNSCC cohort. We employed the Chi-squared test to assess significant mutation enrichment among the different prognostic groups.
Single-cell sequencing analysis
We leveraged the Tumor Immune Single-cell Hub 2 database (http://tisch.comp-genomics.org/) (28) to conduct an analysis of single-cell sequencing data from GSE150430 cohorts.
Prediction of responses to immunotherapy
Predictions of responses to immunotherapy within the TCGA-HNSCC and GSE65858 cohorts were derived using the TIDE (Tumor immune dysfunction and exclusion) available at http://tide.dfci.harvard.edu/.
Calculation of “xCell” scores
We used the “xCell” package of R software to compute immune, stromal, and microenvironment scores and to characterize 64 distinct cell subtypes in both the TCGA-HNSCC and GSE65858 cohorts.
ESTIMATE algorithm
To evaluate the immune system and microenvironment scores, we employed the ESTIMATE algorithm, a computational method implemented through the ESTIMATE R package.
Transcriptional regulators prediction
Lisa (epigenetic Landscape In Silico deletion Analysis and the second descendent of MARGE) was used to predict the transcriptional regulators of differentially expressed or co-expressed gene sets (29).
Laboratory experiments
For a comprehensive understanding of laboratory experiments, please refer to the Appendix 1 for detailed protocols regarding cell culture, quantitative real-time polymerase chain reaction (qRT-PCR), and Luciferase assay procedures.
Statistical analysis
All data analyses and figure generation were performed using R software (version 4.0.1). Results were considered statistically significant at a P value of less than 0.05.
Results
Identification of DC-related gene candidates by WGCNA
In both the TCGA-HNSCC and GSE65858 cohorts, we identified the top 10,000 genes based on their median absolute deviations and used them to construct the WGCNA network. In the TCGA-HNSCC cohort, we removed three evident outliers as determined by hierarchical clustering. In the GSE65858 cohort, no outlier was detected. In constructing a scale-free topology network, a soft thresholding power of 9 was applied to the TCGA-HNSCC cohort (yielding a scale-free R2 of 0.93, a slope of −1.85, and a truncated R2 of 1) (Figure S1A,S1B) and of 5 for the GSE65858 cohort (resulting in a scale-free R2 of 0.95, a slope of −2.07, and a truncated R2 of 0.98) (Figure S1C,S1D). In the TCGA-HNSCC cohort, 10 co-expression gene modules were clustered (Figure 1A,1B, table available at https://cdn.amegroups.cn/static/public/tcr-23-2360-1.xlsx). In the GSE65858 cohort, 10 co-expression gene modules were clustered (Figure 1C,1D, table available at https://cdn.amegroups.cn/static/public/tcr-23-2360-2.xlsx). Specifically, in the TCGA-HNSCC cohort, the yellow module, consisting of 345 genes, exhibited the strongest positive correlation with DC abundance, as detailed in Figure 2A and further elaborated in Figure S2A. Meanwhile, in the GSE65858 cohort, the blue module, comprising 1,676 genes, demonstrated the most pronounced positive correlation with DC abundance, as evidenced in Figure 2B and further detailed in Figure S2B.
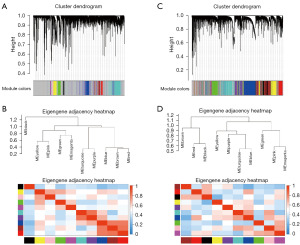
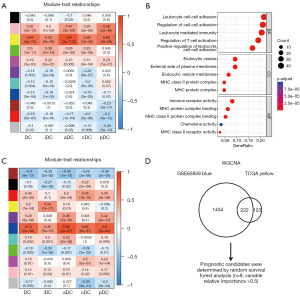
A collective of 222 genes was found to be present in both modules. Through Gene Ontology enrichment analysis, pronounced enrichment was observed in the biological process category for leukocyte cell-cell adhesion (GO:0007159), in the cellular component category for endocytic vesicle (GO:0030139), and in the molecular function category for immune receptor activity (GO:0140375) (Figure 2C).
A DC-based prognostic signature of HNSCC
In the TCGA-HNSCC cohort, a random survival forest analysis was employed to systematically rank the 222 hub gene candidates in terms of OS. The following eight genes exhibited variable importance cutoffs that exceeded 0.5: FGD3, ACP2, IGJ, INPP5D, CCR7, CPVL, CD27, and SPOCK2 (Figures 2D,3A). Utilizing a LASSO Cox’s regression model, we identified significant prognostic candidates for OS based on gene coefficients, including FGD3 =−0.473, ACP2 =0.186, IGJ =−0.043, INPP5D =−0.035, CCR7 =−0.214, CPVL =0.339, and SPOCK2 =−0.056 as illustrated in Figure 3B,3C. Based on the aforementioned coefficients, we deduced the presence of seven DC candidate genes associated with HNSCC prognosis. Among these, ACP2 and CPVL were linked to unfavorable OS, while FGD3, IGJ, INPP5D, CCR7, and SPOCK2 were associated with favorable OS. To further validate these findings, we conducted additional single-gene studies on GSE65858 cohort. Remarkably, our analysis confirmed that nearly all identified genes exhibited prognostic directions consistent with those observed in the TCGA-HNSCC cohort and possessed statistical significance (Figure S3).
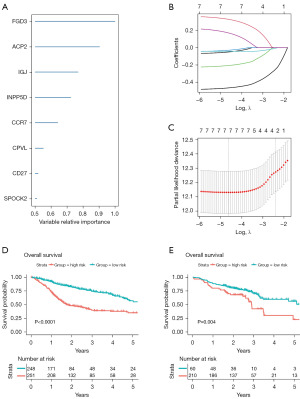
In both the TCGA-HNSCC (Figure 3D) and GSE65858 (Figure 3E) cohorts, we categorized all patients into high- and low-risk groups using the optimal risk score cutoff values determined by the LASSO Cox model. We then derived Spearman correlations between each of the seven candidate genes and DC abundance in both the TCGA-HNSCC (Figure S4A) and GSE65858 (Figure S4B) cohorts, each gene exhibited a positive correlation with overall DC cell and its subpopulation scores. Notably, within the DC cell subpopulations, aDC and pDC showed a higher correlation with these genes compared to other subpopulation scores.
The analysis of single-cell sequences in GSE150430 cohort (Figures 4,5A,5B) revealed that the two candidates associated with unfavorable OS, ACP2 and CPVL, exhibited heightened expression primarily in DCs and Mono/Macro cells. In contrast, the five candidates linked to favorable OS, namely FGD3, IGJ, INPP5D, CCR7, and SPOCK2, were predominantly enriched in B cells, CD4Tconv, Tprolif, CD8Tex, Treg, and Tprolif. Notably, the unfavorable OS candidates co-expressed prominently in DCs, while the five favorable OS candidates co-expressed significantly in subpopulations of T and B cells. Furthermore, in GSE150430, both ACP2 and CPVL demonstrated heightened expression specifically in DCs (table available at https://cdn.amegroups.cn/static/public/tcr-23-2360-3.xlsx).
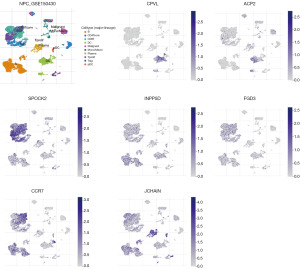
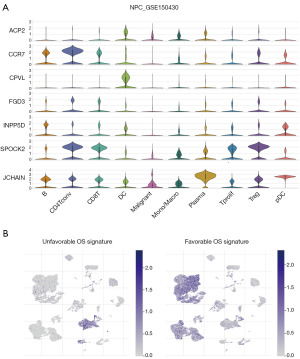
We explored differential gene expression between the ACP2+ DC cells and ACP2− DC cells. This analysis led to the identification of 208 differentially expressed genes, meeting stringent criteria: |log2 fold change (FC)| ≥0.3 and false discovery rate (FDR) ≤0.05. Among these, 176 genes exhibited upregulation in the ACP2+ DC cells, while 32 genes displayed upregulation in the ACP2− DC cells (Figure 6A). Based on the Lisa analysis, top 5 significant transcription factors (STAT1, SPI1, SMAD1, CEBPB, and IRF1) were identified (Figure 6B).
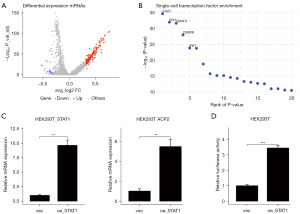
The association between STAT1 and ACP2 (the most crucial candidate among the three noteworthy prognostic DC candidates) has captured our attention. To confirm the correlation between STAT1 and ACP2 in cell line, we conducted qRT-PCR and dual-luciferase assays in HEK293T cells. The qRT-PCR analysis demonstrated elevated mRNA levels of both STAT1 and ACP2 following STAT1 overexpression in HEK293T cells (Figure 6C). Furthermore, the dual-luciferase assay revealed that the transcriptional activity of the ACP2 gene promoter could be enhanced by the binding of STAT1 protein to the promoter of the ACP2 gene in HEK293T cells (Figure 6D).
Consistent with expectations, an elevated risk score was correlated with unfavorable OS in the TCGA-HNSCC cohort (Figure 7A,7B). In the high-risk group, ACP2 and CPVL levels exhibited an increase, while in the low-risk group, FGD3, IGJ, INPP5D, CCR7, and SPOCK2 levels showed elevated expression (Figure 7C).
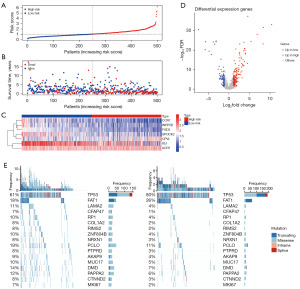
Differential gene expression, immune characteristics, and enrichment pathways among the LASSO risk clusters
Using the TCGA-HNSCC cohort, we explored differential gene expression between the low- and high-risk groups. This analysis led to the identification of 274 differentially expressed genes, meeting stringent criteria: |log2FC| ≥1 and FDR ≤0.05. Among these, 175 genes exhibited upregulation in the high-risk group, while 99 genes displayed upregulation in the low-risk group (Figure 7D, table available at https://cdn.amegroups.cn/static/public/tcr-23-2360-4.xlsx).
The xCell immune characteristics of the various LASSO risk clusters differed in both the TCGA-HNSCC and GSE65858 cohorts. The immune system and microenvironment scores, assessed using the ESTIMATE algorithm, exhibited elevated values in the low-risk groups as opposed to the high-risk groups in both the TCGA-HNSCC cohort (Figure S5A) and the GSE65858 cohort (Figure S5B). This observation suggests a potentially more favorable immune landscape and microenvironment in the low-risk groups across both cohorts. However, of the 64 xCell subtypes, 34 were significantly elevated or reduced in the TCGA-HNSCC cohort and 23 in the GSE65858 cohort. Twenty-one cell subtypes, including DC populations (DC, aDC, cDC, and pDC; Figure S3A,S3B), B cell populations (B cells, plasma cells, class-switched memory B cells, memory B cells, and pro-B cells), T cell populations (CD4 Tem cells, CD4 memory T cells, CD4-naive T cells, CD8 T cells, CD8 Tcm cells, CD8 Tem and Tgd cells, and Tregs), basophils, mast cells, pre-adipocytes, and smooth muscle cells, overlapped in all significant subtypes of the TCGA-HNSCC as well as GSE65858 cohorts (all P<0.01). Only smooth muscle cell abundance was elevated in the high-risk groups (Tables S1,S2).
After risk clustering stratification, we analyzed somatic mutations in the high- as well as low-risk groups. Among the top 200 somatic mutations, we identified 17 with levels that differed significantly between these groups. TP53 and FAT1 mutations were significantly more common in the high-risk groups (Figure 7E).
Additionally, the GSEA was employed to elucidate the functional significance between high- and low-risk groups. Specifically, we utilized the Hallmark and KEGG datasets to explore the enrichment of gene sets related to biological pathways and hallmark processes. Notably, the high-risk group exhibited significant enrichment of the terms KEGG_RIBOSOME and HALLMARK_EPITHELIAL_MESENCHYMAL_TRANSITION, and the low-risk group exhibited significant enrichment of the terms KEGG_PRIMARY_IMMUNODEFICIENCY and HALLMARK_ALLOGRAFT_REJECTION (Figure 8A,8B).
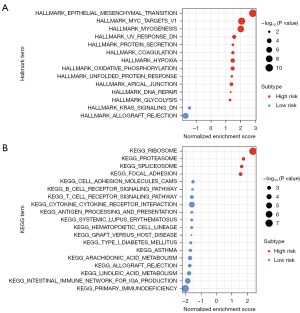
Immunotherapy sensitivity in LASSO risk stratification groups
We next explored the predicted efficacies of established immunotherapies in the high- as well as low-risk groups. TIDE predicted markedly better responses to immunotherapy in the low-risk than high-risk groups in both the TCGA-HNSCC and GSE65858 cohorts (Figure 9A,9B).
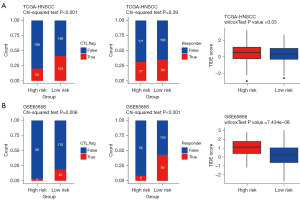
Also, we found notable elevations of four TIDE immunotherapy markers (CD8, CD274, IFNG, and Merck18) in the low-risk group and two markers (MDSC and CAF) in the high-risk group (Figure 10A,10B).
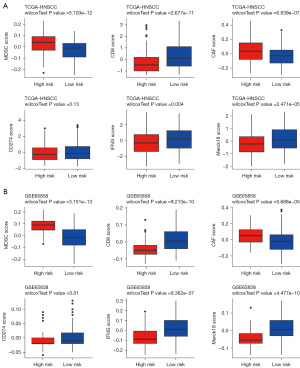
Discussion
Our study unveils the critical role of DCs in HNSCC. Through rigorous analysis of clinical datasets, it identifies 222 DC-related candidate genes and pinpoints seven key prognostic genes, including ACP2 and CPVL. Differential gene expression analysis reveals 208 genes distinguishing ACP2+ and ACP2− DC cells. Notable findings include top transcription factors like STAT1 and genomic associations such as TP53 and FAT1 mutations. The confirmed correlation between STAT1 and ACP2, along with insights into immunotherapeutic sensitivity variations among risk clusters, hints at the potential for a robust DC-based prognostic system. This research lays the groundwork for personalized therapeutic strategies, promising improved outcomes in the challenging landscape of HNSCC.
The intricate interplay among DCs, T cells, and B cells in terms of tumor antigen presentation is pivotal in terms of cancer prognosis (11,30-32). DCs capture and present tumor-specific antigens, thereby activating T cells, including the potent cytotoxic T cells that eliminate tumor cells (33). Concurrently, B cells are stimulated to produce antibodies that play vital roles in antigen neutralization (34). These strongly coordinated collaborations greatly influence cancer outcomes. When such delicate interactions are disrupted or become unbalanced, tumors elude immune system detection, and prognosis deteriorates (35). It is essential to understand and promote the intricate interplay among DCs, T cells, and B cells to enhance prognosis. Effective tumor antigen presentation and immune cell activation increase patient survival and treatment success (36). The immune characteristics of the LASSO CAF risk groups in both the TCGA-HNSCC and GSE65858 cohorts revealed intriguing patterns. The low-risk groups exhibited higher immune system and tumor microenvironment scores, suggesting that the immune milieu was favorable, which was substantiated by the significant involvements of various immune cells (DCs, B cells, and T cells) in both cohorts. The immune signatures were similar. Critically, the low-risk groups were anticipated to exhibit a more favorable response to immunotherapy, as evidenced by heightened levels of markers associated with immunotherapeutic efficacy. In contrast, the high-risk clusters displayed increased proportions of immunosuppressive cells of various types. Such findings underscore the clinical need to stratify patients based on risk profiles and strongly suggest that individuals at low risk may benefit more from immunotherapy. The development of personalized treatment strategies tailored to individual risk profiles is essential. Our study has predominantly focused on identifying and analyzing the correlations among DCs, T cells, and B cells in the context of HNSCC. The universal significance of DCs in capturing and presenting tumor antigens, orchestrating T cell responses, and influencing antibody production is a phenomenon observed not only in HNSCC but also across diverse cancer types such as breast, lung, and colorectal cancers (37-40). Disruptions in these immune interactions have been implicated in immune evasion and prognosis in a broader oncological spectrum. Our findings, particularly in low-risk groups, highlight the potential applicability beyond HNSCC, emphasizing the need to stratify patients based on risk profiles and tailor personalized treatment strategies across a variety of cancer types.
Rigorous random survival forest analysis revealed eight high-potential hub candidates that were strongly associated with ACP2 and CPVL expression in the LASSO Cox model and were thus associated with poor OS. Notably, single-cell database analysis revealed that both genes were prominently expressed in DCs. ACP2 (encoding acid phosphatase 2) and CPVL (encoding cysteine proteinase inhibitor, clade V) exert known functions in cellular processes and protease regulation, respectively (41). Although their precise roles in head-and-neck cancers and DCs remain unclear, they may function within TIMEs. ACP2 action in lysosomes may affect DC-mediated antigen presentation and immune responses within tumors (42). Likewise, the role of CPVL in terms of protease regulation may indirectly affect DC activities; proteases are critical in terms of DC migration and antigen processing (43). However, no clear connections between the activities of these genes and DC functions have yet been described in head-and-neck cancer. Further research is needed to explore whether and how ACP2 and CPVL shape TIMEs and might thus be valuable therapeutic targets. On the other hand, our study investigated the roles of FGD3, JCHAIN (previously known as IGJ), INPP5D, CCR7, and SPOCK2, particularly their association with favorable OS. Of notable significance are JCHAIN (joining chain of multimeric IgA and IgM) and CCR7 (C-C motif chemokine receptor 7). JCHAIN is a key peptide in immunoglobulin assembly, primarily functions in B cells and plasma cells to facilitate the secretion of polymeric IgM and IgA, enhancing immune responses at mucosal surfaces. Within the tumor immune microenvironment, the identified association between JCHAIN and favorable OS suggests its potential role in promoting immune responses against cancer (44,45). The chemokine receptor CCR7 is essential in directing immune cells like T cells and DCs to lymph nodes, which is critical for the enhancement of immune surveillance and the overall immune response. Our findings highlight CCR7’s integral role in predicting favorable OS, emphasizing its significance in orchestrating immune cell localization and modulating anti-tumor immune responses (46). Collectively, these observations underscore the potential of JCHAIN and CCR7 as promising markers for predicting positive outcomes and bolstering immune responses in the realm of cancer.
The analysis of differential gene expression in ACP2+ and ACP2− DC cells revealed distinct transcriptional profiles, showing upregulation of 176 genes in ACP2+ cells and 32 in ACP2− cells. Our investigation into the association between STAT1 and ACP2, a crucial prognostic DC candidate, was validated through qRT-PCR and dual-luciferase assays in HEK293T cells, establishing a positive correlation. The identified top transcription factors, including STAT1, imply a complex regulatory network influencing ACP2 expression. This discovery not only holds implications for targeted therapeutic strategies in HNSCC but also unveils a novel axis in DC biology that may influence the tumor microenvironment. While recognizing the limitation of the experiments being conducted in HEK293T cells rather than DCs, further exploration of the functional consequences and potential therapeutic targeting of this regulatory network is warranted. Such investigations will contribute to a more comprehensive understanding of the molecular dynamics within the tumor microenvironment, fostering advancements in therapeutic interventions for HNSCC.
TP53 and FAT1 are frequently mutated in high-risk cancer subgroups, and these mutations significantly influence the TIME. TP53 is often termed the “guardian of the genome”. Mutations that render TP53 dysfunctional trigger genomic instability and tumor evasion of immune surveillance (47), in turn affecting DC-mediated antigen presentation and thus compromising anti-tumor immune responses (48). FAT1 mutations have been correlated with increased tumor invasiveness and changes in the tumor microenvironment (49). Any role of FAT in DC interactions remains unclear, but changes in the tumor microenvironment could indirectly affect DC function and immune responses (50). However, no direct relationship between TP53 or FAT1 mutations and the DCs in head-and-neck cancers has yet been defined; the mutations may affect DC recruitment, maturation, and antigen presentation and thus patient prognosis. It is essential to understand the intricate interplay between genetic alterations and the TIME, including DCs. Such findings are poised to facilitate the development of targeted therapies aimed at improving the clinical outcomes of patients with HNSCC.
Our study has certain limitations. Large biological datasets may be affected by data quality and consistency issues given the various experimental methods employed by different research groups. Additionally, the inherent complexities of tumors associated with the many cell types and molecular interactions involved render bioinformatics analyses difficult; not all relevant biological processes may be captured. Also, validation of bioinformatics data in experimental or clinical settings can be both resource-intensive and technically challenging. Any bioinformatics data on cancers must be interpreted with caution.
Conclusions
Our study reveals the pivotal role of DCs in HNSCC. Analyzing clinical datasets identifies 222 DC-related genes, pinpointing seven prognostic genes, including ACP2 and CPVL. Differential gene expression unveils 208 genes distinguishing ACP2+ and ACP2− DC cells. Notable findings include top transcription factors (e.g., STAT1) and genomic associations like TP53 and FAT1 mutations. Confirmed STAT1 and ACP2 correlation, along with insights into immunotherapeutic sensitivity, hints at a robust DC-based prognostic system, laying the groundwork for personalized therapeutic strategies.
Acknowledgments
We extend our heartfelt gratitude to the committed participants of the TCGA-HNSCC and GSE65858 cohorts. Their invaluable contributions of clinical data and mRNA expression datasets have been pivotal for our comprehensive analysis.
Funding: The study was supported by fundings from
Footnote
Reporting Checklist: The authors have completed the TRIPOD reporting checklist. Available at https://tcr.amegroups.com/article/view/10.21037/tcr-23-2360/rc
Peer Review File: Available at https://tcr.amegroups.com/article/view/10.21037/tcr-23-2360/prf
Conflicts of Interest: All authors have completed the ICMJE uniform disclosure form (available at https://tcr.amegroups.com/article/view/10.21037/tcr-23-2360/coif). The authors have no conflicts of interest to declare.
Ethical Statement:
Open Access Statement: This is an Open Access article distributed in accordance with the Creative Commons Attribution-NonCommercial-NoDerivs 4.0 International License (CC BY-NC-ND 4.0), which permits the non-commercial replication and distribution of the article with the strict proviso that no changes or edits are made and the original work is properly cited (including links to both the formal publication through the relevant DOI and the license). See: https://creativecommons.org/licenses/by-nc-nd/4.0/.
References
- Johnson DE, Burtness B, Leemans CR, et al. Head and neck squamous cell carcinoma. Nat Rev Dis Primers 2020;6:92. [Crossref] [PubMed]
- Chow LQM. Head and Neck Cancer. N Engl J Med 2020;382:60-72. [Crossref] [PubMed]
- Ruffin AT, Li H, Vujanovic L, et al. Improving head and neck cancer therapies by immunomodulation of the tumour microenvironment. Nat Rev Cancer 2023;23:173-88. [Crossref] [PubMed]
- V B. Approaches for Head and Neck Cancer Research - Current Status and the Way Forward. Cancer Invest 2022;40:151-72. [Crossref] [PubMed]
- Wang CW, Biswas PK, Islam A, et al. The Use of Immune Regulation in Treating Head and Neck Squamous Cell Carcinoma (HNSCC). Cells 2024;13:413. [Crossref] [PubMed]
- Balgobind S, Cheung VKY, Luk P, et al. Prognostic and predictive biomarkers in head and neck cancer: something old, something new, something borrowed, something blue and a sixpence in your shoe. Pathology 2024;56:170-85. [Crossref] [PubMed]
- Zhang S, Chopin M, Nutt SL. Type 1 conventional dendritic cells: ontogeny, function, and emerging roles in cancer immunotherapy. Trends Immunol 2021;42:1113-27. [Crossref] [PubMed]
- Kvedaraite E, Ginhoux F. Human dendritic cells in cancer. Sci Immunol 2022;7:eabm9409. [Crossref] [PubMed]
- Giza HM, Bozzacco L. Unboxing dendritic cells: Tales of multi-faceted biology and function. Immunology 2021;164:433-49. [Crossref] [PubMed]
- Mpakali A, Stratikos E. The Role of Antigen Processing and Presentation in Cancer and the Efficacy of Immune Checkpoint Inhibitor Immunotherapy. Cancers (Basel) 2021;13:134. [Crossref] [PubMed]
- Del Prete A, Salvi V, Soriani A, et al. Dendritic cell subsets in cancer immunity and tumor antigen sensing. Cell Mol Immunol 2023;20:432-47. [Crossref] [PubMed]
- Fu C, Ma T, Zhou L, et al. Dendritic Cell-Based Vaccines Against Cancer: Challenges, Advances and Future Opportunities. Immunol Invest 2022;51:2133-58. [PubMed]
- Yu J, Sun H, Cao W, et al. Research progress on dendritic cell vaccines in cancer immunotherapy. Exp Hematol Oncol 2022;11:3. [Crossref] [PubMed]
- Peil J, Vossen C, Bock F, et al. Combined Osteopontin Blockade and Type 2 Classical Dendritic Cell Vaccination as Effective Synergetic Therapy for Conjunctival Melanoma. J Immunol 2024;212:487-99. [Crossref] [PubMed]
- Liu J, Fu M, Wang M, et al. Cancer vaccines as promising immuno-therapeutics: platforms and current progress. J Hematol Oncol 2022;15:28. [Crossref] [PubMed]
- Luo S, Chen J, Xu F, et al. Dendritic Cell-Derived Exosomes in Cancer Immunotherapy. Pharmaceutics 2023;15:2070. [Crossref] [PubMed]
- Liu Y, Li S, Chen L, et al. Global trends in tumor microenvironment-related research on tumor vaccine: a review and bibliometric analysis. Front Immunol 2024;15:1341596. [Crossref] [PubMed]
- Weusthof C, Burkart S, Semmelmayer K, et al. Establishment of a Machine Learning Model for the Risk Assessment of Perineural Invasion in Head and Neck Squamous Cell Carcinoma. Int J Mol Sci 2023;24:8938. [Crossref] [PubMed]
- Feng B, Shen Y, Pastor Hostench X, et al. Integrative Analysis of Multi-omics Data Identified EGFR and PTGS2 as Key Nodes in a Gene Regulatory Network Related to Immune Phenotypes in Head and Neck Cancer. Clin Cancer Res 2020;26:3616-28. [Crossref] [PubMed]
- Horton JD, Knochelmann HM, Day TA, et al. Immune Evasion by Head and Neck Cancer: Foundations for Combination Therapy. Trends Cancer 2019;5:208-32. [Crossref] [PubMed]
- Yao Y, Fu C, Zhou L, et al. DC-Derived Exosomes for Cancer Immunotherapy. Cancers (Basel) 2021;13:3667. [Crossref] [PubMed]
- Aran D, Hu Z, Butte AJ. xCell: digitally portraying the tissue cellular heterogeneity landscape. Genome Biol 2017;18:220. [Crossref] [PubMed]
- Langfelder P, Horvath S. WGCNA: an R package for weighted correlation network analysis. BMC Bioinformatics 2008;9:559. [Crossref] [PubMed]
- Pickett KL, Suresh K, Campbell KR, et al. Random survival forests for dynamic predictions of a time-to-event outcome using a longitudinal biomarker. BMC Med Res Methodol 2021;21:216. [Crossref] [PubMed]
- Wilkerson MD, Hayes DN. ConsensusClusterPlus: a class discovery tool with confidence assessments and item tracking. Bioinformatics 2010;26:1572-3. [Crossref] [PubMed]
- McCarthy DJ, Chen Y, Smyth GK. Differential expression analysis of multifactor RNA-Seq experiments with respect to biological variation. Nucleic Acids Res 2012;40:4288-97. [Crossref] [PubMed]
- Wu T, Hu E, Xu S, et al. clusterProfiler 4.0: A universal enrichment tool for interpreting omics data. Innovation (Camb) 2021;2:100141. [Crossref] [PubMed]
- Han Y, Wang Y, Dong X, et al. TISCH2: expanded datasets and new tools for single-cell transcriptome analyses of the tumor microenvironment. Nucleic Acids Res 2023;51:D1425-31. [Crossref] [PubMed]
- Qin Q, Fan J, Zheng R, et al. Lisa: inferring transcriptional regulators through integrative modeling of public chromatin accessibility and ChIP-seq data. Genome Biol 2020;21:32. [Crossref] [PubMed]
- Maffuid K, Cao Y. Decoding the Complexity of Immune-Cancer Cell Interactions: Empowering the Future of Cancer Immunotherapy. Cancers (Basel) 2023;15:4188. [Crossref] [PubMed]
- Zhang E, Ding C, Li S, et al. Roles and mechanisms of tumour-infiltrating B cells in human cancer: a new force in immunotherapy. Biomark Res 2023;11:28. [Crossref] [PubMed]
- Wang Q, Shao X, Zhang Y, et al. Role of tumor microenvironment in cancer progression and therapeutic strategy. Cancer Med 2023;12:11149-65. [Crossref] [PubMed]
- Fu C, Jiang A. Dendritic Cells and CD8 T Cell Immunity in Tumor Microenvironment. Front Immunol 2018;9:3059. [Crossref] [PubMed]
- Schriek P, Ching AC, Moily NS, et al. Marginal zone B cells acquire dendritic cell functions by trogocytosis. Science 2022;375:eabf7470. [Crossref] [PubMed]
- Gonzalez H, Hagerling C, Werb Z. Roles of the immune system in cancer: from tumor initiation to metastatic progression. Genes Dev 2018;32:1267-84. [Crossref] [PubMed]
- Xie N, Shen G, Gao W, et al. Neoantigens: promising targets for cancer therapy. Signal Transduct Target Ther 2023;8:9. [Crossref] [PubMed]
- Huang L, Rong Y, Tang X, et al. Engineered exosomes as an in situ DC-primed vaccine to boost antitumor immunity in breast cancer. Mol Cancer 2022;21:45. [Crossref] [PubMed]
- Szpor J, Streb J, Glajcar A, et al. Dendritic Cells Are Associated with Prognosis and Survival in Breast Cancer. Diagnostics (Basel) 2021;11:702. [Crossref] [PubMed]
- Caronni N, Piperno GM, Simoncello F, et al. TIM4 expression by dendritic cells mediates uptake of tumor-associated antigens and anti-tumor responses. Nat Commun 2021;12:2237. [Crossref] [PubMed]
- Katopodi T, Petanidis S, Charalampidis C, et al. Tumor-Infiltrating Dendritic Cells: Decisive Roles in Cancer Immunosurveillance, Immunoediting, and Tumor T Cell Tolerance. Cells 2022;11:3183. [Crossref] [PubMed]
- Bailey K, Rahimi Balaei M, Mehdizadeh M, et al. Spatial and temporal expression of lysosomal acid phosphatase 2 (ACP2) reveals dynamic patterning of the mouse cerebellar cortex. Cerebellum 2013;12:870-81. [Crossref] [PubMed]
- Roche PA, Cresswell P. Antigen Processing and Presentation Mechanisms in Myeloid Cells. Microbiol Spectr 2016;4: [Crossref] [PubMed]
- Robertson L, Williamson SL, Mégy K, et al. Mapping of the porcine serine carboxypeptidase vitellogenic-like gene (CPVL) to chromosome 18. Anim Genet 2005;36:160-1. [Crossref] [PubMed]
- Zheng Y, Lu P, Deng Y, et al. Single-Cell Transcriptomics Reveal Immune Mechanisms of the Onset and Progression of IgA Nephropathy. Cell Rep 2020;33:108525. [Crossref] [PubMed]
- Meeuwsen MH, Wouters AK, Wachsmann TLA, et al. Broadly applicable TCR-based therapy for multiple myeloma targeting the immunoglobulin J chain. J Hematol Oncol 2023;16:16. [Crossref] [PubMed]
- Salem A, Alotaibi M, Mroueh R, et al. CCR7 as a therapeutic target in Cancer. Biochim Biophys Acta Rev Cancer 2021;1875:188499. [Crossref] [PubMed]
- Liu S, Liu T, Jiang J, et al. p53 mutation and deletion contribute to tumor immune evasion. Front Genet 2023;14:1088455. [Crossref] [PubMed]
- Sobhani N, D'Angelo A, Wang X, et al. Mutant p53 as an Antigen in Cancer Immunotherapy. Int J Mol Sci 2020;21:4087. [Crossref] [PubMed]
- Chen ZG, Saba NF, Teng Y. The diverse functions of FAT1 in cancer progression: good, bad, or ugly? J Exp Clin Cancer Res 2022;41:248. [Crossref] [PubMed]
- Irshad K, Srivastava C, Malik N, et al. Upregulation of Atypical Cadherin FAT1 Promotes an Immunosuppressive Tumor Microenvironment via TGF-β. Front Immunol 2022;13:813888. [Crossref] [PubMed]