Bioinformatics reveals the potential mechanisms and biomarkers of necroptosis in neuroblastoma
Highlight box
Key findings
• This work identified six differentially expressed genes associated with necroptosis in neuroblastoma: BIRC2, CAMK2G, CASP3, DAPK1, IL8, and FLOT2.
What is known and what is new?
• Results revealed significant enrichment in pathways such as tuberculosis, apoptosis-multiple species, Salmonella infection, legionellosis, and platinum drug resistance.
• The study revealed 38 potential drugs corresponding to BIRC2, CAMK2G, CASP3, and IL8.
What is the implication, and what should change now?
• The study revealed important pathways, regulatory mechanisms, and immune infiltration patterns in neuroblastoma.
Introduction
Background
Neuroblastoma (NB), a pediatric tumor originating from neural crest tissue, is commonly found in infants and young children. Pathologically, it presents with features of immature neuroblastic cells and typically arises in the abdomen or paraspinal region of infants (1). Accounting for 7–10% of childhood tumors, NB displays a wide range of biological behaviors, from benign to extremely aggressive. While some NB cases might regress spontaneously or respond well to treatment, others show invasive growth and distant metastasis, rendering them resistant to therapy (2). Therefore, further research is essential to explore the varied response patterns and resistance mechanisms in NB, enhancing our understanding of their development and progression, and aiding in the identification of novel therapeutic approaches and prognostic biomarkers for predicting survival rates.
Multiple modes of programmed cell death are crucial targets for combating NB (3). Studies have demonstrated that interventions like gene expression modulation and pharmacological treatments, aimed at promoting apoptosis, have been successful in halting NB progression (4,5). Specifically, by triggering failure in cytoplasmic division, which subsequently activates the caspase family, ultimately leading to heightened levels of apoptosis in NB cells (6,7). Alongside the classical caspase-dependent apoptosis, other forms of programmed cell death, such as necroptosis, are present in tumor cells and may be launched in response to cellular stress. In NB, impaired caspase 8-induced pro-apoptotic activity at late stage results in marked resistance to drug-induced apoptosis (8). Therefore, provoking necroptosis to eliminate NB cells represents an alternative strategy to enhance therapeutic efficiency. Research indicates that genes associated with necroptosis play a significant role in NB (9). These genes might regulate the activation of necroptotic signaling pathways, the expression of key factors involved in necroptosis execution, and the balance between cell survival and death (10). However, our comprehensive understanding of genes related to necroptosis in NB is still limited.
Objectives
The objective of this study is to investigate genes involved in necroptosis pathways in NB and their potential roles in disease development and treatment. Through analysis of NB Gene Expression Omnibus (GEO) datasets, differentially expressed genes (DEGs) associated with necroptotic pathways were identified. Additionally, we performed enrichment analyses using Gene Ontology (GO) and Kyoto Encyclopedia of Genes and Genomes (KEGG) to elucidate the functional and regulatory mechanisms of these genes in cellular processes and signaling pathways. Enrichment analyses using GO and KEGG were conducted to understand the functional and regulatory roles of these genes in cellular processes and signaling pathways. A protein-protein interaction (PPI) network was constructed to explore interactions among mRNA-microRNA (miRNA), mRNA-transcription factor (TF), and mRNA-drugs. Furthermore, receiver operating characteristic (ROC) curve analysis of necroptosis-related DEGs (NRDEGs) and immune infiltration analysis were also performed to study their regulation and interactions within the immune system.
In summary, this study provides in-depth insights into the genes associated with necroptosis pathways in NB, revealing their potential roles in disease development and treatment. Our findings not only contribute to a better understanding of NB’s pathogenesis and regulatory networks but also provide novel perspectives and strategies for diagnosing and treating this challenging disease. We present this article in accordance with the STREGA reporting checklist (available at https://tcr.amegroups.com/article/view/10.21037/tcr-24-14/rc).
Methods
Data processing
The technology roadmap of data and analysis processing was executed according to Figure 1. Firstly, the expression profile datasets GSE19274 (11), GSE73517 (12), and GSE85047 (13) of NB patients were downloaded from the GEO database (14) using the R package GEOquery (15). Detailed information for the datasets were shown in Table 1. The species information for these databases was Homo sapiens. The GSE19274 dataset included 100 primary NB samples and 38 cell lines, making a total of 138 samples, with data platform GPL6102 Illumina human-6 v2.0 expression beadchip. GSE73517 included 105 primary NB samples, with data platform GPL16876 Agilent-020382 Human Custom Microarray 44k (Feature Number version). GSE85047 consisted of 280 primary NB samples, with the data platform being GPL5175 [HuEx-1_0-st] Affymetrix Human Exon 1.0 ST Array [transcript (gene) version]. The study was conducted in accordance with the Declaration of Helsinki (as revised in 2013).
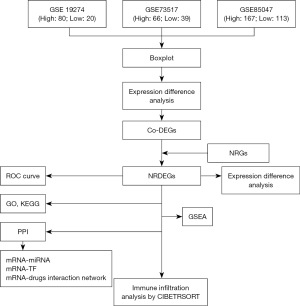
Table 1
Information | GSE19274 | GSE73517 | GSE85047 |
---|---|---|---|
Platform | GPL6102 | GPL16876 | GPL5175 |
Species | Homo sapiens | Homo sapiens | Homo sapiens |
Tissue | Primary NB tumors | Primary NB tumors | Colorectal tissues |
Samples in high-risk group | 80 | 66 | 167 |
Samples in low-risk group | 20 | 39 | 113 |
Reference | RNAi screen of the protein kinome identifies checkpoint kinase 1 (CHK1) as a therapeutic target in NB | Integrative Genome-Scale Analysis Identifies Epigenetic Mechanisms of Transcriptional Deregulation in Unfavorable NB | Cross-Cohort Analysis Identifies a TEAD4-MYCN Positive Feedback Loop as the Core Regulatory Element of High-Risk NB |
NB, neuroblastoma; CHK1, checkpoint kinase 1.
The probe name annotations for each dataset were derived from the respective GPL platform files. In the subsequent analyses, we selected 100 tumor samples from the GSE19274 dataset, which originated from primary NB. Samples with inss scores of one, three, and six were classified into the low-risk group (group: Low), while samples with inss scores of four and five were allocated to the high-risk group (group: High). Additionally, we included all the expression profile data samples from GSE73517, including 105 primary NB tumors, samples with inss stages one, 2.1, 2.2, and 4S were classified as low-risk, while stages four and five were classified as high-risk. We also included all the expression profile data samples from GSE85047 containing clinical staging information, including 280 primary NB tumors, where samples with inss=st1, st2, and st4s were classified as a low-risk group (group: Low), and those with inss=st3 and st4 were classified as a high-risk group (group: High).
Necroptosis is a significant form of cellular demise. We collected necroptosis-related genes (NRGs) from the GeneCards database (16) (https://www.genecards.org/) by using “Necroptosis” as the search keyword and only retained NRGs that were “Protein Coding” and had a “Relevance score >0.500”. Additionally, we obtained the list of NRGs from published articles on necroptosis-related genes by using “Necroptosis” as the search keyword in PubMed (17-19). The GeneCards database contained 201 NRGs, and the published literature in PubMed contained 286 NRGs. Finally, using “Necroptosis” as the search keyword in MSigDB (20) (https://www.gsea-msigdb.org/), we obtained a reference gene set named GOBP_NECROPTOTIC_SIGNALING_PATHWAY, which contained eight NRGs. After merging and removing duplicates, we obtained a total of 425 NRGs.
NB-related differentially expressed genes
To investigate the pathways, potential mechanisms, and biological features associated with DEGs in NB, we first performed normalization using the limma package (21) on GSE19274, GSE73517, and GSE85047 datasets, and subsequently conducted differential analysis to find the DEGs between the low-risk group and high-risk groups in the three NB datasets. Genes selected as DEGs for further study were those with |logfold change (FC)| >0 and P.adj <0.05. Genes with significant up-regulation if they had a logFC >0 and P.adj <0.05, while genes with significant down-regulation if they had a logFC <0 and P.adj <0.05.
To begin with, we performed an intersection of the DEGs obtained from the differential analysis of GSE19274, GSE73517, and GSE85047, considering only those genes that satisfied the criteria of |logFC|>0 and P.adj <0.05, and obtained the common DEGs (Co-DEGs) by drawing a Venn diagram. Subsequently, we identified the overlapping genes between the Co-DEGs and NRGs and drew a Venn diagram to obtain the NRDEGs. We first used the ggplot2 package in R to draw a volcano map to show the difference analysis, and then used the pheatmap package to draw a heat map.
GO and KEGG analysis of NRDEGs
GO analysis (22) provides standardized descriptions of gene products in terms of molecular function (MF), involvement in biological process (BP), and cellular component (CC). In other words, it offers concise annotations for gene products. KEGG (23) is a repository of vast information that includes genomes, diseases, drugs, biological pathways, and more. It is widely used by researchers. Based on three GEO datasets, we used the R package clusterProfiler (24) to perform GO annotation analysis on NRDEGs, and used the Benjamini-Hochberg (BH) method for P value correction. We also selected criteria where Q value (false discovery rate) <0.05 and P value <0.05 were considered statistically significant.
Gene set enrichment analysis (GSEA)
GSEA (25) is commonly used to assess the distribution trend of predefined gene sets within gene expression data. It ranks the gene sets based on their correlation with phenotypes, enabling the determination of their contribution to the phenotype. In this study, we first divided genes from GSE19274, GSE73517, and GSE85047 into two groups, high-risk and low-risk, based on their ranking in disease severity. Then, by using the clusterProfiler package, we used it to profile all DEGs associated with necroptosis in both groups. For the GSEA enrichment analysis, the following parameters were utilized: a minimum gene set the size of 10, a seed value of 2022, 10,000 permutations, a maximum gene set the size of 500, and the BH method for P value correction. In the Molecular Signature Database (MSigDB), we acquired the c2.cp.v7.2.symbols gene set, and set when the FDR (false discovery rate) value (Q value) <0.1, P<0.05, as significantly enriched.
PPI analysis of NRDEGs
The PPI consists of proteins engaging in mutual interactions, thereby contributing to multiple aspects of cellular processes such as cell cycle regulation, energy metabolism, substance metabolism, biological signal transduction, and gene expression regulation. Studying the interplay of numerous proteins in a biological system through systematic analysis holds crucial significance in unraveling the working mechanisms of proteins and gaining insights into the functional associations among proteins. Functional linkages are all important. The STRING database (26) can be searched for known and predicted interactions between proteins. So we used it to construct a PPI network related to NRDEGs, and visualized using Cytoscape (version 3.9.1) (27), and finally set a medium confidence level (0.400), which was the minimum required interaction score, and identified these NRDEGs as hub genes in NB.
Construction of mRNA interaction networks
The MiRDB database (28) is essentially a database of predicted miRNA target genes and functional annotations. We used it to predict miRNAs that interact with the hub gene, then mapped the mRNA-miRNA interaction network, compiling data from Target Score >80.
The CHIPBase database (29) (version 3.0) (https://rna.sysu.edu.cn/chipbase/) collected chip_seq data from more than 10,000 samples from 10 species, sorting out TFs and various genes. The hTFtarget database (30) (http://bioinfo.life.hust.edu.cn/hTFtarget) contains the most comprehensive data available on human TF-targets, integrating large-scale human TF target gene data. We used the CHIPBase database and the hTFtarget database to search for TFs that bind to central genes and visualize them using Cytoscape software.
Furthermore, we employed the Drug Gene Interaction Database (DGIdb) (31) (https://www.dgidb.org) to forecast potential interactions between central genes and drug/small molecule compounds. Additionally, we utilized the Cytoscape software to visualize.
ROC analysis
ROC curve (32) can be used to select the optimal model, evaluate or compare the applicability of diagnostic experiments, or choose suitable cutoff values. It is a graphical analysis tool. Area under the ROC curve (AUC) typically ranges from 0.5 to 1. When the AUC value is closer to 1, it indicates better diagnostic performance. An AUC value ranging from 0.5 to 0.7 suggests low accuracy, while a range of values from 0.7 to 0.9 indicates moderate accuracy. Values above 0.9 indicate high accuracy. We used the survival ROC package in R to draw the ROC curve of prognostic differential expression genes related to necrosis in the NB dataset TCGA-NB and the AUC value was used as a means to evaluate diagnostic accuracy.
Immune infiltration analysis (CIBERSORT)
CIBERSORT (33) analyzes gene expression data with an immune infiltration analysis algorithm to estimate cell type abundance. We uploaded the matrix data of prognostic DEGs related to necrosis in high and low-risk groups of the NB dataset to CIBERSORT and filtered data with an immune cell enrichment score above zero in combination with the LM22 feature gene matrix to obtain and provide a detailed representation of the abundance matrix for immune cell infiltration. The immune cell composition in high and low-risk group samples of the NB dataset was displayed through a box plot. The relationship between immune cells and prognostic DEGs related to necrosis in different risk groups was calculated by the spearman algorithm and displayed using the R package pheatmap to draw a correlation heatmap.
Statistical analysis
Data processing and analysis in this study were conducted using R software (version 4.1.2) as the primary tool. Continuous variables were reported as mean ± standard deviation. The Wilcoxon rank-sum test was utilized to compare two groups, whereas the Kruskal-Wallis test was employed for comparisons involving three or more groups. Unless otherwise stated, all results were based on Spearman correlation analysis to calculate the correlation coefficients between different molecules. Statistically significant differences were considered when the P value was less than 0.05.
Results
Normalization of the NB dataset
Normalization was initially conducted on three NB datasets (GSE19274, GSE73517, and GSE85047) using the R package limma. The GSE19274 dataset comprised 100 NB samples, the GSE73517 dataset had 105 NB samples, and the GSE85047 dataset included 280 NB samples. As shown in Figure 2A-2F, the batch effects among samples in the NB datasets [GSE19274 pre-batch correction (Figure 2A) and post-batch correction (Figure 2B), GSE73517 pre-batch correction (Figure 2C) and post-batch correction (Figure 2D), and GSE85047 pre-batch correction (Figure 2E) and post-batch correction (Figure 2F)] were largely removed after standardization. This process resulted in a more consistent expression profile across all samples.
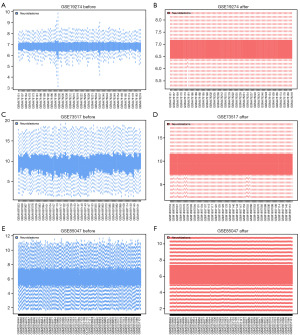
Analysis of NB-related differential genes
The data of NB tumor tissue in the high-risk group and low-risk group from GSE19274, GSE73517, and GSE85047 was normalized using the limma package. Subsequently, a differential analysis was conducted on each dataset.
The results showed that 42,763 DEGs were obtained from GSE19274, of which 1,749 genes met the threshold of |logFC|>0 and P.adj <0.05. Among these genes, 279 were upregulated, and 1,470 were downregulated. We generated a volcano plot (Figure 3A) to visualize the results of the differential analysis conducted on this dataset. A total of 19,860 DEGs were obtained from GSE73517, and a total of 9,131 genes fulfilled the criteria of having an absolute log fold change (|logFC|) >0 and an adjusted P value (P adj) <0.05. Among these genes, 412 were upregulated (positive logFC values) and 8,719 were downregulated (negative logFC values). A volcano plot depicting the outcomes of the differential analysis conducted on this dataset was generated and labeled as Figure 3B. GSE85047 yielded 7,272 DEGs, of which 1,909 genes met the threshold of |logFC| >0 and P.adj <0.05. Among these genes, 1,024 were upregulated and 885 were downregulated. Figure 3C illustrated a volcano plot depicting the findings of the differential analysis performed on this dataset.
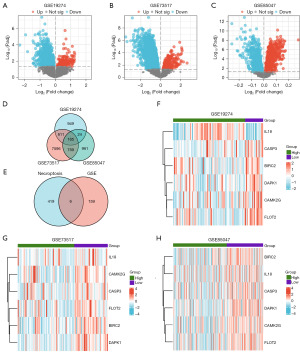
An intersection analysis was conducted on DEGs from GSE19274, GSE73517, and GSE85047, resulting in 165 Co-DEGs specific to NB datasets. A Venn diagram (Figure 3D) was created to illustrate this intersection. By further intersecting the Co-DEGs with the NRGs within the dataset, six NRDEGs were identified in NB. We plotted a Venn diagram of these genes (Figure 3E). The six NRDEGs were BIRC2, CAMK2G, CASP3, DAPK1, FLOT2, and IL8.
Differential expression analyses of the six NRDEGs across different groups were then performed on GSE19274 (Figure 3F), GSE73517 (Figure 3G), and GSE85047 (Figure 3H). Subsequently, heatmaps were generated using the R package pheatmap to visually represent the results of the differential expression analysis for the six NRDEGs.
GO and KEGG analysis of NRDEGs
To investigate the biological pathways, MF, CC and BP associated with six NRDEGs (BIRC2, CAMK2G, CASP3, DAPK1, FLOT2, IL8) and NB, GO enrichment analysis was initially conducted on NRDEGs (Table 2). The results showed that the six NRDEGs were mainly enriched in BP such as regulation of leukocyte cell-cell adhesion, amyloid precursor protein catabolic process, regulation of activated T cell proliferation, regulation of amyloid precursor protein catabolic process, activated T cell proliferation, as well as CC such as membrane raft, CD40 receptor complex, membrane microdomain, membrane region, endocytic vesicle. In addition, they were also enriched in MF including calmodulin-dependent protein kinase activity, protease binding, cytokine receptor binding, serine/threonine kinase inhibitor activity cyclin-dependent protein. Figure 4A demonstrated the findings of the GO functional enrichment analysis. Moreover, we visualized the results of the BP (Figure 4B), CC (Figure 4C), and MF (Figure 4D) analyses in the form of network diagrams.
Table 2
Ontology | ID | Description | GeneRatio | BgRatio | P value | P adjust | Q value |
---|---|---|---|---|---|---|---|
Biological process | GO:1902991 | Regulation of amyloid precursor protein catabolic process | 2/6 | 35/18670 | 5.10E−05 | 0.007 | 0.003 |
Biological process | GO:0046006 | Regulation of activated T cell proliferation | 2/6 | 37/18670 | 5.70E−05 | 0.007 | 0.003 |
Biological process | GO:0050798 | Activated T cell proliferation | 2/6 | 41/18670 | 7.02E−05 | 0.007 | 0.003 |
Biological process | GO:0042987 | Amyloid precursor protein catabolic process | 2/6 | 44/18670 | 8.09E−05 | 0.007 | 0.003 |
Biological process | GO:1903037 | Regulation of leukocyte cell-cell adhesion | 3/6 | 304/18670 | 8.24E−05 | 0.007 | 0.003 |
Cellular component | GO:0045121 | Membrane raft | 3/6 | 315/19717 | 7.79E−05 | 0.001 | 5.86e−04 |
Cellular component | GO:0098857 | Membrane microdomain | 3/6 | 316/19717 | 7.87E−05 | 0.001 | 5.86e−04 |
Cellular component | GO:0098589 | Membrane region | 3/6 | 328/19717 | 8.79E−05 | 0.001 | 5.86e−04 |
Cellular component | GO:0035631 | CD40 receptor complex | 1/6 | 11/19717 | 0.003 | 0.02 | 0.009 |
Cellular component | GO:0030139 | Endocytic vesicle | 2/6 | 303/19717 | 0.003 | 0.02 | 0.009 |
Molecular function | GO:0004683 | Calmodulin-dependent protein kinase activity | 2/6 | 28/17697 | 3.61E−05 | 0.002 | 9.49e−04 |
Molecular function | GO:0002020 | Protease binding | 2/6 | 128/17697 | 7.64E−04 | 0.022 | 0.01 |
Molecular function | GO:0005516 | Calmodulin binding | 2/6 | 200/17697 | 0.002 | 0.03 | 0.02 |
Molecular function | GO:0005126 | Cytokine receptor binding | 2/6 | 286/17697 | 0.004 | 0.03 | 0.02 |
Molecular function | GO:0004861 | Cyclin-dependent protein serine/threonine kinase inhibitor activity | 1/6 | 12/17697 | 0.004 | 0.03 | 0.02 |
GO, Gene Ontology; NRGs, necroptosis-related genes.
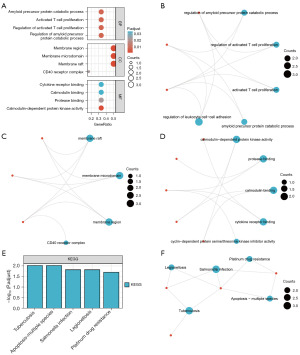
The results (Table 3) showed that the six NRDEGs were significantly enriched in KEGG pathways such as tuberculosis, apoptosis-multiple species, Salmonella infection, legionellosis, platinum drug resistance. We presented the gene expression of the top five enriched KEGG pathways in the form of a bar chart (Figure 4E) and a network diagram (Figure 4F).
Table 3
Ontology | ID | Description | GeneRatio | BgRatio | P value | P adjust | Q value |
---|---|---|---|---|---|---|---|
KEGG | hsa05152 | Tuberculosis | 3/6 | 180/8076 | 2.07E−04 | 0.01 | 0.007 |
KEGG | hsa04215 | Apoptosis-multiple species | 2/6 | 32/8076 | 2.26E−04 | 0.01 | 0.007 |
KEGG | hsa05132 | Salmonella infection | 3/6 | 249/8076 | 5.41E−04 | 0.02 | 0.012 |
KEGG | hsa05134 | Legionellosis | 2/6 | 57/8076 | 7.21E−04 | 0.02 | 0.012 |
KEGG | hsa01524 | Platinum drug resistance | 2/6 | 73/8076 | 0.001 | 0.02 | 0.015 |
KEGG, Kyoto Encyclopedia of Genes and Genomes; NRGs, necroptosis-related genes.
GSEA
GSEA was administered on the GSE19274, GSE73517, and GSE85047 datasets. Initially, the analysis revealed the major biological characteristics involved in GSE19274 (Figure 5A, Table 4). The DEGs in GSE19274 indicated significant enrichment in pathways such as marzec IL2 signaling up (Figure 5B), IL6 signaling up (Figure 5C), apoptosis by cdkn1a via TP53 (Figure 5D), and IL6 deprivation dn (Figure 5E). Similarly, the major biological characteristics about GSE73517 were also present in Figure 5F and Table 5, the DEGs in GSE73517 exhibited significant enrichment in pathways including cell cycle literature (Figure 5G), multiple myeloma PR up (Figure 5H), cell cycle genes in ir responsE_6HR (Figure 5I), and doxorubicin resistance up (Figure 5J). In the analysis of major biological characteristics with GSE85047 (Figure 6A, Table 6), the DEGs in GSE85047 revealed significant enrichment in pathways such as transcriptional_regulation_by_TP53 (Figure 6B), regulation_of_TP53_activity (Figure 6C), cell_cycle_mitotic (Figure 6D), and cell_cycle_checkpoints (Figure 6E). Furthermore, the significantly enriched pathways identified in all three NB datasets were intersected and visualized using a Venn diagram (Figure 6F), which displayed 70 common pathways, including chromosome maintenance, eukaryotic translation_initiation and activation of the MRNA upon binding of the CAP binding complex and EIFS and subsequent binding to 43S.
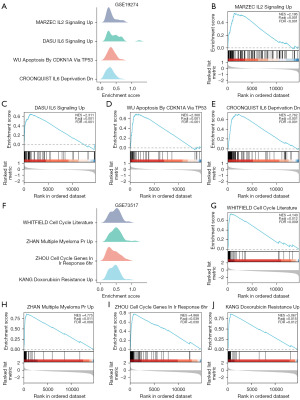
Table 4
Description | Set size | Enrichment score | NES | P adjust | Q values |
---|---|---|---|---|---|
CROONQUIST_IL6_DEPRIVATION_DN | 88 | 0.711962903 | 2.762151126 | 4.15783E−05 | 0.001177307 |
WU_APOPTOSIS_BY_CDKN1A_VIA_TP53 | 43 | 0.697420392 | 2.360270769 | 3.27065E−05 | 0.001177307 |
DASU_IL6_SIGNALING_UP | 52 | 0.656739469 | 2.31115816 | 3.45853E−05 | 0.001177307 |
MARZEC_IL2_SIGNALING_UP | 107 | 0.549082423 | 2.19546032 | 4.55851E−05 | 0.001177307 |
TANG_SENESCENCE_TP53_TARGETS_DN | 50 | 0.628130053 | 2.192834622 | 3.42149E−05 | 0.001177307 |
PLASARI_TGFB1_TARGETS_1HR_UP | 31 | 0.66771651 | 2.103176359 | 9.17291E−05 | 0.001381075 |
MAHAJAN_RESPONSE_TO_IL1A_UP | 75 | 0.525350477 | 1.983260896 | 3.8915E−05 | 0.001177307 |
PLASARI_TGFB1_SIGNALING_VIA_NFIC_10HR_DN | 25 | 0.611304035 | 1.830648744 | 0.003 | 0.017840239 |
VERRECCHIA_RESPONSE_TO_TGFB1_C4 | 11 | 0.748485714 | 1.816875934 | 0.004 | 0.026945006 |
VERRECCHIA_DELAYED_RESPONSE_TO_TGFB1 | 36 | 0.557716667 | 1.815594033 | 0.001 | 0.011620236 |
GSEA, gene set enrichment analysis; NES, normalized enrichment scores.
Table 5
Description | Set size | Enrichment score | NES | P adjust | Q values |
---|---|---|---|---|---|
KANG_DOXORUBICIN_RESISTANCE_UP | 49 | 0.869784383 | 5.067248971 | 0.02 | 0.011938429 |
ZHOU_CELL_CYCLE_GENES_IN_IR_RESPONSE_6HR | 72 | 0.779329749 | 4.868419982 | 0.04 | 0.029939209 |
ZHAN_MULTIPLE_MYELOMA_PR_UP | 40 | 0.861289825 | 4.775303577 | 0.01 | 0.008359071 |
WHITFIELD_CELL_CYCLE_LITERATURE | 42 | 0.737468739 | 4.140157161 | 0.01 | 0.009124105 |
EGUCHI_CELL_CYCLE_RB1_TARGETS | 22 | 0.891485144 | 4.131421427 | 0.006 | 0.004501181 |
ODONNELL_TARGETS_OF_MYC_AND_TFRC_DN | 40 | 0.733087465 | 4.064503136 | 0.01 | 0.008359071 |
YU_MYC_TARGETS_UP | 36 | 0.746744079 | 4.03236663 | 0.01 | 0.007664377 |
WU_APOPTOSIS_BY_CDKN1A_VIA_TP53 | 47 | 0.681625644 | 3.972138642 | 0.01 | 0.011382769 |
TANG_SENESCENCE_TP53_TARGETS_DN | 50 | 0.595559451 | 3.489173505 | 0.02 | 0.0121448 |
SCIAN_CELL_CYCLE_TARGETS_OF_TP53_AND_TP73_DN | 22 | 0.745457834 | 3.454685127 | 0.006 | 0.004501181 |
GSEA, gene set enrichment analysis; NES, normalized enrichment scores.
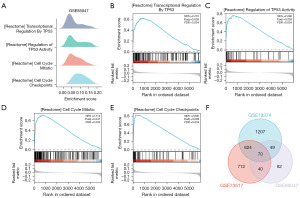
Table 6
Description | Set size | Enrichment score | NES | P adjust | Q values |
---|---|---|---|---|---|
REACTOME_CELL_CYCLE_CHECKPOINTS | 75 | 0.825606444 | 2.800184698 | 0.04 | 0.033539129 |
REACTOME_CELL_CYCLE_MITOTIC | 158 | 0.719599475 | 2.713584698 | 0.04 | 0.034562342 |
REACTOME_REGULATION_OF_TP53_ACTIVITY | 39 | 0.767478516 | 2.295656827 | 0.04 | 0.033539129 |
REACTOME_TRANSCRIPTIONAL_REGULATION_BY_TP53 | 115 | 0.633550796 | 2.270386264 | 0.04 | 0.033539129 |
REACTOME_G2_M_CHECKPOINTS | 24 | 0.925706686 | 2.478896419 | 0.04 | 0.033539129 |
REACTOME_MITOTIC_SPINDLE_CHECKPOINT | 44 | 0.766508628 | 2.357685435 | 0.04 | 0.033539129 |
REACTOME_G2_M_DNA_DAMAGE_CHECKPOINT | 14 | 0.896526991 | 2.130580729 | 0.04 | 0.033539129 |
REACTOME_REGULATION_OF_TP53_ACTIVITY_THROUGH_PHOSPHORYLATION | 22 | 0.836644197 | 2.207323956 | 0.04 | 0.033539129 |
REACTOME_TP53_REGULATES_TRANSCRIPTION_OF_CELL_CYCLE_GENES | 27 | 0.798110644 | 2.201255531 | 0.04 | 0.033539129 |
REACTOME_REGULATION_OF_TP53_EXPRESSION_AND_DEGRADATION | 11 | 0.825152231 | 1.874452071 | 0.04 | 0.033539129 |
GSEA, gene set enrichment analysis; NES, normalized enrichment scores.
PPI network analysis of NRDEGs
PPI analysis was conducted on BIRC2, CAMK2G, CASP3, DAPK1, FLOT2, and IL8 utilizing the STRING database with default parameters. The resulting PPI network was constructed and interaction relationships were visualized using the Cytoscape software (Figure 7A). These six genes were identified as hub genes for NB. The hub genes were BIRC2, CAMK2G, CASP3, DAPK1, FLOT2, and IL8.
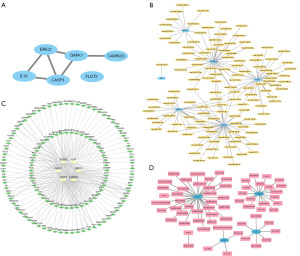
Utilizing the miRDB database, we performed miRNA predictions for the interaction with hub genes and visualized the results using Cytoscape, as shown in Figure 7B. The analysis revealed a mRNA-miRNA network comprising five hub genes (BIRC2, CAMK2G, CASP3, DAPK1, and FLOT2) and 125 miRNA molecules.
We identified TFs interacting with hub genes from the hTFtarget and CHIPBase databases. After downloading the interaction relationship data found in both databases and taking the intersection with 10 key genes, we obtained interaction data between 6 hub genes and 47 TFs. This interaction data was visualized in Cytoscape (Figure 7C), where pale yellow elliptical blocks represented mRNAs, and light green diamond blocks represented TFs. Notably, the mRNA-TF network highlighted that the gene NQO1, which is associated with necroptosis, had the most interaction relationships with TFs. CAMK2G was found to be associated with 83 pairs of mRNA-TF interactions.
Subsequently, we collected 38 potential drugs linked to BIRC2, CAMK2G, CASP3, and IL8, which were visualized in the mRNA-drug interaction network (Figure 7D). We also found 33 drugs that targeted CASP3.
Differential expression analysis of NRDEGs
Further analysis was conducted to explore the expression differences of BIRC2, CAMK2G, CASP3, DAPK1, FLOT2, and IL8 in relation to high/low-risk groups of NB across GSE19274, GSE73517, and GSE85047 datasets.
The results showed significant statistical differences (***P<0.001) in the expression levels of BIRC2, CAMK2G, CASP3, DAPK1, and FLOT2 between the high and low-risk groups, while the IL8 gene did not show statistical significance across different groups (Figure 8A). ROC curves indicated good predictive ability for BIRC2 (AUC =0.742, Figure 8B), CAMK2G (AUC =0.728, Figure 8C), CASP3 (AUC =0.717, Figure 8D), DAPK1 (AUC =0.789, Figure 8E), FLOT2 (AUC =0.850, Figure 8F), and moderate predictive ability for IL8 (AUC =0.606, Figure 8G).
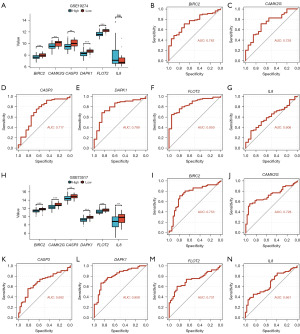
In GSE73517, the results showed significant statistical differences (***P<0.001) in the expression levels of all NRDEGs between the high and low-risk groups (Figure 8H). Subsequently, we generated ROC curves for the six NRDEGs in the GSE73517 dataset and displayed the results (Figure 8I-8N). Based on the ROC curves, it revealed that BIRC2 (AUC =0.753, Figure 8I) and CAMK2G (AUC =0.728, Figure 8J) had good predictive ability, CASP3 (AUC =0.692, Figure 8K) had moderate predictive ability, DAPK1 (AUC =0.808, Figure 8L) and FLOT2 (AUC =0.731, Figure 8M) had good predictive ability, while IL8 (AUC =0.661, Figure 8N) had moderate predictive ability.
In GSE85047, the results showed significant statistical differences in the expression levels of six NRDEGs between the high and low-risk groups in the GSE85047 dataset (Figure 9A) (***P<0.001). Subsequently, we performed ROC curve analysis for these six NRDEGs in the GSE85047 dataset. And the results displayed that the BIRC2 (AUC =0.687, Figure 9B), CAMK2G (AUC =0.688, Figure 9C), and CASP3 (AUC =0.660, Figure 9D) had low diagnostic value for NB. Besides, the DAPK1 (AUC =0.727, Figure 9E) and FLOT2 (AUC =0.723, Figure 9F) exhibited good diagnostic value for NB, while IL8 (AUC =0.600, Figure 9G) had low diagnostic value.
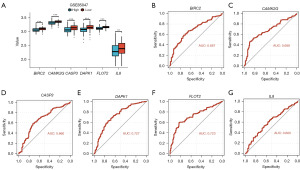
CIBERSORT analysis of NB dataset
In GSE19274, a bar plot (Figure 10A) was generated to visualize associations. The results indicated significant correlations (P<0.05) between the abundance of seven immune cell infiltrates and the expression of BIRC2, CASP3, DAPK1, FLOT2, and IL8 in the low-risk group of GSE19274 (Figure 10B). Particularly, significant correlations were found between immune cell types, including M2 macrophages, and DAPK1. In the high-risk group, significant correlations were observed between the expression of BIRC2, CAMK2G, CASP3, and the abundance of seven immune cell types (Figure 10C). Notably, the immune cells such as Macrophages M2, exhibited a significant positive correlation with BIRC2.
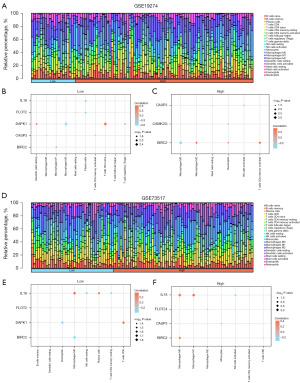
Next, we performed similar analyses to assess the expression differences of BIRC2, CAMK2G, CASP3, DAPK1, FLOT2, and IL8 in GSE73517 and GSE85047 datasets. Figure 10D illustrated the immune cell infiltration in each sample of GSE73517. In the group low-risk of GSE73517, significant correlations (P<0.05) were discovered between the abundance of seven immune cell infiltrates and the expression of BIRC2, DAPK1, FLOT2, and IL8 (Figure 10E). Specifically, immune cells such as eosinophils, plasma cells, T cells CD8, NK cells resting demonstrated significant correlations with BIRC2, DAPK1, and IL8. In the high group (Figure 10F), immune cells macrophages M0, macrophages M1 were significantly correlated with IL8.
Figure 11A manifested the immune cell infiltration in each sample of the GSE85047 dataset. In the low-risk group of GSE85047, significant correlations (P<0.05) were observed between the expression of six NRDEGs and the abundance of seven immune cell infiltrates (Figure 11B). The immune cells macrophages M1, macrophages M2, T cells CD8 were significantly correlated with IL8 and CAMK2G. Similarly, in the high-risk group (Figure 11C), monocytes, and NK cells resting, macrophages M2 showed significant correlations with IL8.
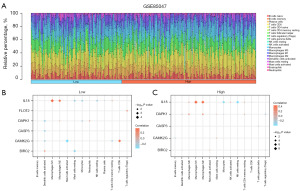
Discussion
NB is a pressing research area given its high incidence in children and its propensity to progress into a highly aggressive tumor (34). The identification of specific targets and treatment mechanisms for this disease is crucial to improving patient outcomes. Nevertheless, there is a scarcity of knowledge regarding the fundamental molecular mechanisms and viable therapeutic targets in this particular realm of NB research.
Our study analyzed three GEO datasets (GSE19274, GSE73517, and GSE85047) to investigate necroptosis in NB. We identified six DEGs linked to necroptosis: BIRC2, CAMK2G, CASP3, DAPK1, IL8, and FLOT2, which have been extensively studied in NB and other similar diseases (35,36). Interestingly, some of our findings (BIRC2, CAMK2G, CASP3, and FLOT2) contradicted previous research, highlighting the novelty and innovation of our discoveries. To gain insights into the biological functions and pathways related to the DEGs, we conducted GO, KEGG, and GSEA enrichment analyses. Our results revealed significant enrichment in pathways such as tuberculosis, apoptosis-multiple species, Salmonella infection, legionellosis, and platinum drug resistance, providing valuable information about underlying mechanisms and pathways in NB. Additionally, we constructed PPI networks and explored mRNA-miRNA, mRNA-TF, and mRNA-drug interactions to further understand the regulatory mechanisms and signaling pathways implicated in necroptosis. These analyses contributed to our comprehensive understanding of the complex regulatory networks and potential therapeutic targets in NB. Furthermore, we performed an immune infiltration analysis to probe the immune characteristics in NB. The results of this analysis shed light on the immune response and its potential impact on tumor progression and prognosis in NB patients. Overall, our study offers novel insights into the key genes associated with necroptosis in NB, and uncovers important pathways, regulatory mechanisms, and immune infiltration patterns that contribute to develop potential treatment strategies for this disease.
It is important to acknowledge several limitations in the current study. First, the sample size and heterogeneity of the analyzed GEO datasets might restrict the generalizability of the findings. NB is a heterogeneous disease with diverse genetic alterations, and the limited number of samples used in this study might not fully capture its complexity. Secondly, despite efforts to choose high-quality datasets, inherent biases and technical variations might still exist, potentially affecting the accuracy and reproducibility of the results. Furthermore, the lack of experimental validation of the identified genes and pathways might be a notable limitation. Functional studies, such as in vitro or in vivo experiments, are necessary to confirm the functional roles of the identified DEGs and validate their interactions as potential therapeutic targets. Integration of clinical data, including patient survival, treatment response, and disease stage, would improve the clinical relevance of the findings. Lastly, the integration and comparison of data from different platforms might pose challenges in terms of data harmonization and consistency.
Conclusions
In summary, our study focused on exploring NB-specific research and identified key genes associated with necroptosis. Through functional analysis, we revealed the involvement of specific pathways and regulatory mechanisms. Additionally, we gained deeper insights into the phenotypic characteristics and clinical significance of NB. Despite limitations, our research adds to the knowledge of NB and provides potential targets and mechanisms for future research and therapeutic interventions.
Acknowledgments
Funding: This study was jointly supported by
Footnote
Reporting Checklist: The authors have completed the STREGA reporting checklist. Available at https://tcr.amegroups.com/article/view/10.21037/tcr-24-14/rc
Peer Review File: Available at https://tcr.amegroups.com/article/view/10.21037/tcr-24-14/prf
Conflicts of Interest: All authors have completed the ICMJE uniform disclosure form (available at https://tcr.amegroups.com/article/view/10.21037/tcr-24-14/coif). The authors have no conflicts of interest to declare.
Ethical Statement: The authors are accountable for all aspects of the work in ensuring that questions related to the accuracy or integrity of any part of the work are appropriately investigated and resolved. The study was conducted in accordance with the Declaration of Helsinki (as revised in 2013).
Open Access Statement: This is an Open Access article distributed in accordance with the Creative Commons Attribution-NonCommercial-NoDerivs 4.0 International License (CC BY-NC-ND 4.0), which permits the non-commercial replication and distribution of the article with the strict proviso that no changes or edits are made and the original work is properly cited (including links to both the formal publication through the relevant DOI and the license). See: https://creativecommons.org/licenses/by-nc-nd/4.0/.
References
- Chou SW, Chang HH. Evolution and contemporary role of metronomic chemotherapy in the treatment of neuroblastoma. Cancer Lett 2024;588:216617. [Crossref] [PubMed]
- Gerges A, Canning U. Neuroblastoma and its Target Therapies: A Medicinal Chemistry Review. ChemMedChem 2024;19:e202300535. [Crossref] [PubMed]
- Valter K, Zhivotovsky B, Gogvadze V. Cell death-based treatment of neuroblastoma. Cell Death Dis 2018;9:113. [Crossref] [PubMed]
- Kocak H, Ackermann S, Hero B, et al. Hox-C9 activates the intrinsic pathway of apoptosis and is associated with spontaneous regression in neuroblastoma. Cell Death Dis 2013;4:e586. [Crossref] [PubMed]
- Mohan N, Chakrabarti M, Banik NL, et al. Combination of LC3 shRNA plasmid transfection and genistein treatment inhibited autophagy and increased apoptosis in malignant neuroblastoma in cell culture and animal models. PLoS One 2013;8:e78958. [Crossref] [PubMed]
- Joshi S, Braithwaite AW, Robinson PJ, et al. Dynamin inhibitors induce caspase-mediated apoptosis following cytokinesis failure in human cancer cells and this is blocked by Bcl-2 overexpression. Mol Cancer 2011;10:78. [Crossref] [PubMed]
- Paccosi E, Costantino M, Balzerano A, et al. Neuroblastoma Cells Depend on CSB for Faithful Execution of Cytokinesis and Survival. Int J Mol Sci 2021;22:10070. [Crossref] [PubMed]
- Nicolai S, Pieraccioli M, Peschiaroli A, et al. Neuroblastoma: oncogenic mechanisms and therapeutic exploitation of necroptosis. Cell Death Dis 2015;6:e2010. [Crossref] [PubMed]
- Chu Jing. Development of a prognostic model for children with neuroblastoma based on necroptosis-related genes. Front Genet 2022;13:947000. [Crossref] [PubMed]
- Watanabe S, Inoue M, Suzuki T, et al. Polyphyllin D induces necroptosis in neuroblastoma cells (IMR-32 and LA-N-2) in mice. Pediatr Surg Int 2023;39:196. [Crossref] [PubMed]
- Cole KA, Huggins J, Laquaglia M, et al. RNAi screen of the protein kinome identifies checkpoint kinase 1 (CHK1) as a therapeutic target in neuroblastoma. Proc Natl Acad Sci U S A 2011;108:3336-41. [Crossref] [PubMed]
- Henrich KO, Bender S, Saadati M, et al. Integrative Genome-Scale Analysis Identifies Epigenetic Mechanisms of Transcriptional Deregulation in Unfavorable Neuroblastomas. Cancer Res 2016;76:5523-37. [Crossref] [PubMed]
- Rajbhandari P, Lopez G, Capdevila C, et al. Cross-Cohort Analysis Identifies a TEAD4-MYCN Positive Feedback Loop as the Core Regulatory Element of High-Risk Neuroblastoma. Cancer Discov 2018;8:582-99. [Crossref] [PubMed]
- Barrett T, Troup DB, Wilhite SE, et al. NCBI GEO: mining tens of millions of expression profiles--database and tools update. Nucleic Acids Res 2007;35:D760-5. [Crossref] [PubMed]
- Davis S, Meltzer PS. GEOquery: a bridge between the Gene Expression Omnibus (GEO) and BioConductor. Bioinformatics 2007;23:1846-7. [Crossref] [PubMed]
- Stelzer G, Rosen N, Plaschkes I, et al. The GeneCards Suite: From Gene Data Mining to Disease Genome Sequence Analyses. Curr Protoc Bioinformatics 2016;54:1.30.1-1.30.33.
- Liu F, Wei T, Liu L, et al. Role of Necroptosis and Immune Infiltration in Human Stanford Type A Aortic Dissection: Novel Insights from Bioinformatics Analyses. Oxid Med Cell Longev 2022;2022:6184802. [Crossref] [PubMed]
- Shi H, Peng Q, Zhou X, et al. An Efficient Signature Based on Necroptosis-Related Genes for Prognosis of Patients With Pancreatic Cancer. Front Genet 2022;13:848747. [Crossref] [PubMed]
- Xie H, Xu J, Xie Z, et al. Identification and Validation of Prognostic Model for Pancreatic Ductal Adenocarcinoma Based on Necroptosis-Related Genes. Front Genet 2022;13:919638. [Crossref] [PubMed]
- Liberzon A, Birger C, Thorvaldsdóttir H, et al. The Molecular Signatures Database (MSigDB) hallmark gene set collection. Cell Syst 2015;1:417-25. [Crossref] [PubMed]
- Ritchie ME, Phipson B, Wu D, et al. limma powers differential expression analyses for RNA-sequencing and microarray studies. Nucleic Acids Res 2015;43:e47. [Crossref] [PubMed]
- Yu G. Gene Ontology Semantic Similarity Analysis Using GOSemSim. Methods Mol Biol 2020;2117:207-15. [Crossref] [PubMed]
- Kanehisa M, Goto S. KEGG: kyoto encyclopedia of genes and genomes. Nucleic Acids Res 2000;28:27-30. [Crossref] [PubMed]
- Yu G, Wang LG, Han Y, et al. clusterProfiler: an R package for comparing biological themes among gene clusters. OMICS 2012;16:284-7. [Crossref] [PubMed]
- Subramanian A, Tamayo P, Mootha VK, et al. Gene set enrichment analysis: a knowledge-based approach for interpreting genome-wide expression profiles. Proc Natl Acad Sci U S A 2005;102:15545-50. [Crossref] [PubMed]
- Szklarczyk D, Gable AL, Lyon D, et al. STRING v11: protein-protein association networks with increased coverage, supporting functional discovery in genome-wide experimental datasets. Nucleic Acids Res 2019;47:D607-13. [Crossref] [PubMed]
- Shannon P, Markiel A, Ozier O, et al. Cytoscape: a software environment for integrated models of biomolecular interaction networks. Genome Res 2003;13:2498-504. [Crossref] [PubMed]
- Chen Y, Wang X. miRDB: an online database for prediction of functional microRNA targets. Nucleic Acids Res 2020;48:D127-31. [Crossref] [PubMed]
- Zhou KR, Liu S, Sun WJ, et al. ChIPBase v2.0: decoding transcriptional regulatory networks of non-coding RNAs and protein-coding genes from ChIP-seq data. Nucleic Acids Res 2017;45:D43-50. [Crossref] [PubMed]
- Zhang Q, Liu W, Zhang HM, et al. hTFtarget: A Comprehensive Database for Regulations of Human Transcription Factors and Their Targets. Genomics Proteomics Bioinformatics 2020;18:120-8. [Crossref] [PubMed]
- Freshour SL, Kiwala S, Cotto KC, et al. Integration of the Drug-Gene Interaction Database (DGIdb 4.0) with open crowdsource efforts. Nucleic Acids Res 2021;49:D1144-51. [Crossref] [PubMed]
- Roumeliotis S, Schurgers J, Tsalikakis DG, et al. ROC curve analysis: a useful statistic multi-tool in the research of nephrology. Int Urol Nephrol 2024; Epub ahead of print. [Crossref] [PubMed]
- Chen J, Sun M, Chen C, et al. Construction of a novel anoikis-related prognostic model and analysis of its correlation with infiltration of immune cells in neuroblastoma. Front Immunol 2023;14:1135617. [Crossref] [PubMed]
- Kennedy PT, Zannoupa D, Son MH, et al. Neuroblastoma: an ongoing cold front for cancer immunotherapy. J Immunother Cancer 2023;11:e007798. [Crossref] [PubMed]
- Kiss NB, Kogner P, Johnsen JI, et al. Quantitative global and gene-specific promoter methylation in relation to biological properties of neuroblastomas. BMC Med Genet 2012;13:83. [Crossref] [PubMed]
- Moreno-Guerrero SS, Ramírez-Pacheco A, Rocha-Ramírez LM, et al. Association of Genetic Polymorphisms and Serum Levels of IL-6 and IL-8 with the Prognosis in Children with Neuroblastoma. Cancers (Basel) 2021;13:529. [Crossref] [PubMed]