Stratifying osteosarcoma patients using an epigenetic modification-related prognostic signature: implications for immunotherapy and chemotherapy selection
Highlight box
Key findings
• The current study developed a 6-gene epigenetic modification-related prognostic signature (EMRPS) consisting of DDX24, DNAJC1, HDAC4, SIRT7, SP140 and UHRF2 for osteosarcoma (OS). This EMRPS was validated internally and externally and can accurately predict patient survival, showing potential for guiding treatment selection.
What is known and what is new?
• OS treatment lacks reliable prognostic markers. Epigenetic alterations play a role in disease progression.
• This study identified a novel 6-gene EMRPS which was found to correlate with immune activity and chemotherapy sensitivity, suggesting it may facilitate choosing appropriate immunotherapy or chemotherapy to improve outcomes.
What is the implication, and what should change now?
• The EMRPS demonstrates promise as a new prognostic biomarker for OS. Its capacity to stratify patients and direct immunotherapy or chemotherapy decisions warrants further prospective validation. Once validated, the EMRPS could help personalize OS treatment decisions to potentially enhance survival. Clinically, the EMRPS should be evaluated for its ability to predict treatment response and inform clinical management.
Introduction
Osteosarcoma (OS) is the most common bone tumor, with a bimodal age distribution, occurring around 18 and 60 years (1). The incidence rate in children and adolescents is approximately 3–4.5 cases per million population per year, with slightly higher rates in males than females (2,3). The most common treatment for OS involves a combination of surgical resection and chemotherapy, with individualized treatment plans determined by various factors, including age, gender, and tumor location (4). However, OS treatment still faces many challenges, such as bone defect repair after surgical resection, side effects of chemotherapy, and increased incidence of distant metastasis (5). Immune-based therapies have emerged in recent years, garnering attention due to their ability to stimulate the body’s immune system to combat tumor growth and metastasis (6).
Prognostic markers are widely used to assist in patient treatment selection and assessing the prognosis of those with OS. Various traditional prognostic markers are employed in OS research, such as KI-67 (7), p53 (8), MDR1 (9), and HIF1 (10), and current efforts are underway to establish OS model systems and evaluate novel treatment approaches (11-13). However, current prognostic markers also have certain limitations, which points to the need for continuous research into new prognostic markers to guide personalized OS treatment in clinical practice.
The occurrence and progression of OS involve various molecular and cellular mechanisms, with epigenetic regulation playing an important role (14-17). DNA methylation is a common mechanism of epigenetic regulation and plays an important role in OS. Studies have shown that abnormal methylation of certain key genes is closely associated with the development and progression of OS (18,19). Histone modifications, such as acetylation (20), methylation (21), and phosphorylation (22), also play a crucial role in OS. Dysregulation of histone modifications can lead to aberrant gene expression patterns and contribute to tumor initiation and progression. In addition, non-coding RNAs, including microRNAs (23,24) and long non-coding RNAs (25,26), have been implicated in OS pathogenesis and may serve as potential prognostic markers. In summary, the understanding of molecular mechanisms in OS, particularly the role of epigenetic regulation, is of paramount importance. Hence, the establishment of an OS prognostic model based on core epigenetic modification genes (EMGs) holds great significance, as it can enhance prognostic accuracy, guide personalized treatment strategies, and potentially improve patient outcomes.
With the widespread application of bioinformatics in tumor research, it has become possible to select core genes that influence tumor-specific functional phenotypes from feature gene sets. Lu et al. integrated different datasets and used bioinformatics and statistical methods to construct a robust immune-related risk model to evaluate the prognosis of colon adenocarcinoma (27). Wang et al. have established a pharmacokinetic-pharmacodynamic translation method to identify molecular prognostic biomarker features based on the clearance rate of the anti-programmed death-1 (PD-1) therapeutic drug nivolumab in patients with renal cell carcinoma. In comparison to single cytokine features, this composite biomarker feature can improve the accuracy of long-term clinical outcomes and can be used to ensure patient balance in renal cell carcinoma clinical trials (28). Recently, a study focusing on EMGs found that a risk scoring model consisting of the expression information of MYC, TERT, EIF4E3, and RBM34 genes can be used to indicate OS prognosis (29), and this study is currently the only work that constructs an OS prognosis risk model based on the EMG set. However, because this work mainly focuses on five gene categories, namely RNA m6A modification, histone modification, DNA methylation, RNA binding proteins, and transcription factors, strictly speaking, transcription factors are not EMGs. Therefore, this study is not strictly focused on classic EMGs as its primary subject of research.
The current study aimed to provide new predictive markers and clinical decision-making references for OS prognosis by constructing a risk scoring model based on EMG markers. A nomogram risk model integrating clinical features of OS patients was developed to enhance the accuracy of personalized prognosis assessment. The results highlight the critical role of epigenetic regulation in OS development and progression, emphasizing the need for further research in this area to better understand the role and potential therapeutic implications of epigenetics in OS. We present this article in accordance with the TRIPOD reporting checklist (available at https://tcr.amegroups.com/article/view/10.21037/tcr-23-2300/rc).
Methods
Patient characteristics and epigenetic-related genes
We downloaded RNA-sequencing data and clinical information of 88 OS patients from the Genomic Data Commons Data Portal (https://portal.gdc.cancer.gov, TARGET-OS project). After excluding patients with unclear survival information and overall survival time of less than 30 days, a total of 85 patients were included in this study. The GSE21257 dataset, which includes mRNA expression matrix and clinical information of 53 OS samples, was obtained from the Gene Expression Omnibus (GEO) database (https://www.ncbi.nlm.nih.gov/geo/query/acc.cgi?acc=GSE21257). This dataset was utilized for external validation purposes. The EMGs were collected from previous study (30), including genes involving in DNA methylation, histone modifications, chromatin remodeling and RNA modifications. A total of 319 EMGs were found co-expressed in both the osteosarcoma data from the Therapeutically Applicable Research to Generate Effective Treatments database (TARGET-OS) and the GSE21257 cohort, which was extracted for further analysis. This study was conducted in accordance with the Declaration of Helsinki (as revised in 2013).
Identify survival-associated EMGs
Patients in the TARGET-OS project were initially randomly assigned to training (n=44) and testing (n=41) cohorts. Subsequently, univariate Cox analysis using the “survival” package in the R software was conducted on 319 EMGs in the training cohort to identify genes associated with overall survival in OS patients (https://cdn.amegroups.cn/static/public/tcr-23-2300-1.xls). To further investigate the potential interactions between the survival-associated EMGs, the identified genes were uploaded to the Search Tool for the Retrieval of Interaction Gene/Proteins (STRING) database (https://cn.string-db.org/). The STRING database provides comprehensive information on protein-protein interactions, allowing for the analysis and visualization of the networks formed by the EMGs. This analysis will provide insights into the potential functional relationships and molecular mechanisms among these genes in the context of OS.
Functional enrichment analysis of survival-associated EMGs
To further understand the functions and characteristics of the survival-associated EMGs, we used R package “clusterProfiler” to perform functional enrichment analysis based on the Gene Ontology (GO) database. The significantly identified pathways, with both a P value and a false discovery rate (FDR) less than 0.05, were represented as a chord diagram using the “ggplot2” package.
Construction and validation of epigenetic modification-related prognostic signature (EMRPS)
To identify key survival-associated EMGs in the training cohort, we employed the Cox regression model with a least absolute shrinkage and selection operator (LASSO) penalty. This approach aimed to select a subset of EMGs that were associated with the prognosis of OS patients. By applying a penalty proportional to the size of the regression coefficients, we effectively shrunk the coefficients. Consequently, only a relatively small number of indicators retained non-zero weights, while most of the potential indicators were reduced to zero. The LASSO Cox analysis was conducted using the “glmnet” package. Finally, we established an EMRPS by multiplying the expression level of each EMG with its respective regression coefficient derived from the multivariate Cox regression analysis using the “survival” package. To evaluate the risk associated with each patient, we computed a risk score in both the training, testing, and external validation cohorts. Subsequently, based on the mean risk score, we categorized patients into high-risk or low-risk groups individually for each cohort. Furthermore, we conducted Kaplan-Meier survival analysis and time-dependent receiver operating characteristic (ROC) curves to evaluate the predictive performance of the EMRPS. The Kaplan-Meier survival analysis provided insights into the survival probabilities of patients in different risk groups, allowing us to assess the prognostic value of the signature. Additionally, the time-dependent ROC curves were employed to measure the sensitivity and specificity of the signature at different time points, providing a comprehensive evaluation of its predictive ability. These analyses were instrumental in determining the clinical relevance and utility of the EMRPS.
Development and evaluation of the nomogram
To evaluate the independent prognostic performance of the EMRPS, we performed univariate and multivariate Cox analyses on OS patients with well-defined clinical characteristics, including age, gender, and metastasis status. These analyses aimed to identify whether EMRPS was an independent prognostic factor for overall survival. Additionally, we constructed a novel nomogram that integrated EMRPS with these clinical characteristics. The nomogram served as a visual predictive tool to estimate the survival probability of OS patients. To assess the predictive accuracy of the nomogram, we utilized the C-index and calibration curves. The C-index was computed using the bootstrap method with 1,000 re-samplings, providing a measure of the model’s discrimination power. Calibration curves, generated using the “rms” package, compared the predicted probabilities from the nomogram against the observed outcomes. The optimal calibration curve would closely align with the 45° line, indicating excellent predictive values. These evaluations were crucial in determining the reliability and accuracy of the nomogram as a predictive tool for overall survival in OS patients.
Identify differentially expressed genes between the high-risk and low-risk groups
Differential gene expression analysis between high-risk and low-risk groups was performed via “limma” package, and the log2 (|fold change|) >1 and P value <0.05 were set as the cut-off values to screen out the differential expression genes (DEGs). These DEGs can provide valuable insights into potential molecular mechanisms associated with the different prognoses observed in these groups and can be further explored for their functional implications.
Functional enrichment analysis of DEGs
To further understand the functions and characteristics of the DEGs between the high-risk and low-risk groups, we employed the “clusterProfiler” package for gene functional enrichment analysis. Using this package, we conducted enrichment analysis based on two widely used databases: the Kyoto Encyclopedia of Genes and Genomes (KEGG) and the GO. The GO enrichment analysis comprises three main categories: biological process, cellular component, and molecular function. In the analysis, we identified significantly enriched pathways that had both a P value and an FDR less than 0.05. By performing the enrichment analysis, we gained valuable information about the biological processes, cellular components, and molecular functions associated with the DEGs between the high-risk and low-risk groups. This analysis aids in the understanding of the potential underlying mechanisms and pathways contributing to the observed differences in prognosis between these two groups.
Gene set enrichment analysis (GSEA)
In order to investigate the differences in biological pathways and molecular mechanisms between the high-risk and low-risk groups of OS patients, we utilized GSEA methodology. To conduct the GSEA, we employed annotated gene set files as the reference gene sets. These include: (I) h.all.v2023.1.Hs.symbols.gmt—contains curated gene sets from various databases and sources; (II) c2.cp.kegg.v2023.1.Hs.symbols.gmt—includes gene sets based on the KEGG pathway database; (III) c5.go.bp.v2023.1.Hs.symbols.gmt—comprises gene sets corresponding to GO biological process terms. Using GSEA, we identified the gene sets that were significantly enriched between the high-risk and low-risk groups of OS patients. The significance threshold was set at a nominal P value <0.05. Additionally, we used an FDR cutoff of <0.25 to account for multiple hypothesis testing and control the potential false positive rate. The identified significantly enriched gene sets provide insights into the specific biological pathways and molecular mechanisms that may be differentially regulated between the high-risk and low-risk groups in OS patients. Further analysis and interpretation of these gene sets can help unravel the underlying molecular processes associated with disease progression and may have implications for prognostic stratification or targeted therapy.
Immune cell infiltration and immune score analysis
To further understand the different tumor microenvironment (TME) between the high-risk and low-risk groups of OS patients, we performed estimation of stromal and immune cells in malignant tumor tissues using expression data (ESTIMATE) algorithm to assess the stromal status, immune status, and tumor purity for each sample. This algorithm employs specific characteristics associated with stromal and immune cell infiltration in tumor tissue to calculate the stromal score and immune score of the tumor tissue. The stromal score reflects the estimated level of stromal cells within the tumor tissue, while the immune score reflects the estimated level of immune cell infiltration. These scores provide an indication of the abundance of stromal and immune cells in the TME. Furthermore, the ESTIMATE algorithm can also infer the purity of the tumor tissue. Tumor purity refers to the proportion of tumor cells in the sample, with higher purity indicating a higher proportion of tumor cells and lower contamination from other non-tumor cells. This estimation of tumor purity is valuable for understanding the composition of the TME and its potential impact on tumor behavior and therapeutic response. To further assess immune cell populations within the TME, the single-sample gene set enrichment analysis (ssGSEA) approach was also employed. This method uses predefined gene sets related to specific immune cell types and calculates an enrichment score for each cell type in individual samples. This allows for the quantification of the relative abundance or activity of different immune cell populations within the TME. By applying these methods, researchers can gain insight into the stromal and immune characteristics of tumor samples, infer tumor purity, and explore immune cell composition in the TME. These analyses contribute to a better understanding of the TME and its potential implications for tumor biology and therapeutic interventions.
Tumor mutation burden analysis
We retrieved mutation data of OS patients from the TARGET database and utilized a custom Perl script to extract and compute the tumor mutational burden (TMB) for each patient. TMB was determined as the total number of non-synonymous mutations per megabase, offering a quantitative measure of the genomic mutational load in the cancer samples. Subsequently, employing the “maftools” package in R, we systematically analyzed and visualized the mutational landscape across the OS patient cohort. To effectively illustrate the mutation frequencies, we constructed waterfall plots that highlighted the top 20 most frequently mutated genes within both the high-risk and low-risk groups, as stratified by our prognostic signature. These plots served not only to delineate the mutational profiles of these groups but also to enable a comparative assessment of the mutational spectra. Additionally, an overall mutation frequency plot was created to showcase the distribution of mutations across the entire genome for all OS patients included in the study.
Drug sensitivity analysis
To identify potential therapeutic agents for OS patients in different risk groups, we utilized the “pRRophetic” package in the R software to conduct a round-robin comparison of drug half maximal inhibitory concentration (IC50). This analysis aimed to determine the varying sensitivities of drugs between high-risk and low-risk groups. The resulting differences were visualized through box plots using the “ggplot2” package (with a significance level of P<0.05).
Statistical analysis
All analysis was performed with R software (version 3.6.1) and GraphPad Prism (version 8.3.0), and P<0.05 was considered to be statistically significant unless noted otherwise. Moreover, the heatmaps were generated using the “pheatmap” package, the violin plots and bar plots were generated using the “ggplot2” and “ggpubr” package.
Results
Research design
Analysis flowchart of the study is as Figure 1.
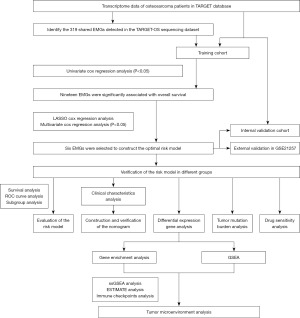
Identification of prognostic EMGs
Using a survival analysis, we screened 319 EMGs and identified 19 genes with prognostic significance. Among these genes, 5 genes were found to be associated with survival protection, while the remaining 14 genes were associated with survival risk (Figure 2A). The GO analysis results revealed that the identified genes are primarily involved in regulating processes such as histone modification and chromatin remodeling (Figure 2B). In addition, our protein interaction analysis suggested that some of these genes may have potential functional interactions with one another (Figure 2C).
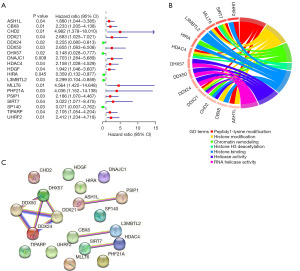
Establish an EMRPS for survival prediction in OS patients
To construct a predictive survival model for OS using these 19 survival-related EMGs, we randomly assigned 85 TARGET-OS patients with complete and reliable clinical information to training (n=44) and testing (n=41) cohorts. LASSO Cox regression analysis was conducted on the training cohort to identify 11 critical genes using the λ value (Figure 3A,3B), which were further reduced to six genes after a multivariate logistic regression analysis. Six core genes, namely DDX24, DNAJC1, HDAC4, SIRT7, SP140 and UHRF2, were selected to develop an EMRPS based on their expression and regression coefficients (Figure 3C). Next, we evaluated the performance of EMRPS in predicting survival outcomes. The resulting survival curves showed a significant reduction in survival in the high-risk group across the training and testing cohorts, as well as across the entire TARGET-OS cohort (Figure 3D-3F, Table S1). Analysis of risk scores and survival status trends further demonstrated a significant increase in the probability of death in patients with higher risk scores (Figure 3G). The ROC curves revealed excellent survival predictive efficacy with area under curve (AUC) values of 0.853, 0.869 and 0.862 for the 1-, 3- and 5-year survival analyses, respectively (Figure 3H). Collectively, these findings provide strong evidence that the EMRPS based on six core genes are an effective tool for predicting survival outcomes in OS patients.
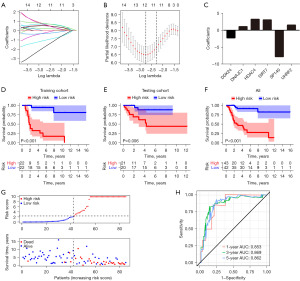
Validation of the EMRPS for survival prediction in OS patients: an external dataset analysis in GEO database
To further validate the predictive ability of this EMRPS, we selected the GEO database [GSE21257 (31)] containing RNA sequencing data from 53 OS tumor tissues for external validation (Table S2). The results of the survival analysis (Figure 4A) and the risk score-survival status trend analysis (Figure 4B) were consistent with those of the TARGET-OS cohort, where OS patients in the high-risk group had significantly lower overall survival and significantly increased risk of death. In addition, the ROC curve analysis showed that the AUCs of the survival analysis at 1, 3 and 5 years are 0.679, 0.736 and 0.729, respectively (Figure 4C), highlighting the excellent survival prediction efficacy of the EMRPS in the external validation dataset.
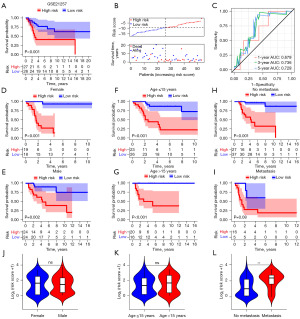
In addition, we evaluated if there were disparities in overall survival across various risk groups based on the EMRPS, stratified by gender (male and female), age (≤15 and >15 years old), and metastasis status (absence and presence). Survival curve analysis revealed a significant decline in overall survival for individuals in the high-risk group, regardless of their sex (Figure 4D,4E) and age (Figure 4F,4G), as well as poorer outcomes in the non-metastatic subgroup (Figure 4H). Moreover, even though there was no statistically significant distinction in overall survival between the high-risk and low-risk groups in the metastatic subgroup, there was a trend towards a worse prognosis in the high-risk group (P<0.001) (Figure 4I). To further elucidate the relationship between risk scores and clinical characteristics such as gender, age, and metastasis status, we compared the risk scores among OS patients stratified by gender, age, and metastasis status. The findings revealed that EMPRS were not associated with gender or age, but were significantly elevated in patients with metastasis (Figure 4J-4L), suggesting that higher risk score are indicative of an increased risk of metastasis.
Although it has been demonstrated that the EMRPS can be used to predict overall survival in OS patients, the specific predictive methods have not been established. Considering that age, gender, and metastasis status are potential clinical features influencing overall survival, we conducted univariate and multivariate analyses incorporating the EMRPS and the aforementioned clinical features. The results showed that the EMRPS and metastasis status were independent prognostic factors for overall survival in OS patients, with the EMRPS exhibiting a higher hazard ratio (Figure 5A). Additionally, we constructed a nomogram for 1-, 3-, and 5-year overall survival, where the EMRPS had the highest weight, confirming its importance in predicting overall survival (Figure 5B). The prediction discrimination of the C-index model demonstrated that the nomogram had a C-index range of 0.81–0.90, while EMRPS and metastasis status had C-index ranges of 0.73–0.79 and 0.66–0.75, respectively (Figure 5C). The calibration curve illustrated that the nomogram had high accuracy in predicting 1-, 3-, and 5-year survival (Figure 5D). These findings suggest that the nomogram has superior predictive power for overall survival in OS patients.
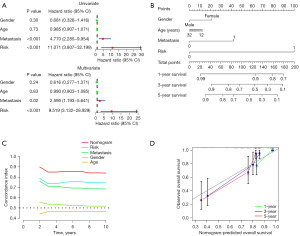
EMRPS influence the prognosis of OS patients through tumor immune mechanisms
In order to uncover the potential mechanisms underlying the impact of EMRPS on overall survival prognosis, we divided the TARGET-OS cohort into low- and high-risk groups, and performed differential gene expression analysis. We observed 339 upregulated genes and 279 downregulated genes in the high-risk group (Figure 6A, https://cdn.amegroups.cn/static/public/tcr-23-2300-2.xls). KEGG analysis revealed that the DEGs were involved in signaling pathways related to hematopoietic cell lineage development, T-cell receptor signaling, cell adhesion molecules, and neuroactive ligand-receptor interaction (Figure 6B). GO analysis showed that DEGs were involved in biological processes such as immunoglobulin production, production of molecular mediator of immune response, activation of immune response, and B-cell receptor signaling (Figure 6C), as well as cellular components such as immunoglobulin complex, T-cell receptor complex, plasma membrane signaling receptor complex, and IgG immunoglobulin complex (Figure 6D). Additionally, DEGs were related to molecular functions, such as antigen binding, immunoglobulin receptor binding, and T-cell receptor binding (Figure 6E). GSEA demonstrated that the low-risk group showed enrichment of inflammation-related pathways, including the IL6/JAK/STAT3 pathway and INFγ pathway. Furthermore, apoptotic and complement-related feature markers were found to be enriched (Figure 6F). Additionally, biological processes such as activation of immune response, antibody production, and lymphocyte co-stimulation signaling were also enriched in the low-risk group (Figure 6G). Furthermore, pathways related to antigen presentation, chemokine signaling, cytokine and its corresponding receptor interaction were also significantly enriched in the low-risk group (Figure 6H). These results suggest that the immune response is more active in the tumor tissue of the low-risk group, involving the activation and interaction of multiple types of immune cells.
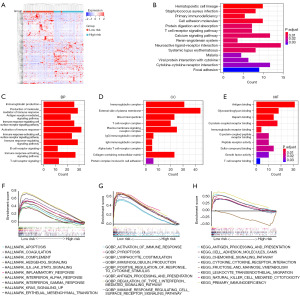
To further elucidate the differences in the immune microenvironment between high- and low-risk groups, we compared the levels of tumor purity, immune score, stromal score, ESTIMATE score (immune score + stromal score), as well as the levels of immune cell infiltration types, immune checkpoint molecules, and other factors in tumor tissues of OS patients in the high- and low-risk groups. The results showed that the immune infiltration level in the low-risk group was significantly higher compared to the high-risk group. This was mainly manifested by increased infiltration of immune cells such as tumor-infiltrating lymphocytes, B cells, CD8+ T cells, Th1 cells, Th2 cells, and T follicular helper cells. Additionally, we observed significant differences in immune regulation signals between the high- and low-risk groups, with antigen-presenting cell (APC) co-stimulation and co-inhibition signals, T cell co-stimulation and co-inhibition signals, as well as human leukocyte antigen (HLA) expression levels and type I interferon response being significantly enhanced in the low-risk group (Figure 7A). These findings suggest that the TME in the low-risk group is immunologically activated. Overall, the low-risk group showed higher immune scores, stromal scores, and ESTIMATE scores, and tumor purity (Figure 7B-7E). This suggests that the tumor composition in the high-risk group is relatively simpler and reflects a lesser impact of the immune system on tumor progression. Immune checkpoint analysis results demonstrated that most differentially expressed immune checkpoints, including immunosuppressive checkpoints such as CTLA-4 and CD274, as well as immune-activating molecules such as CD86, were upregulated in the low-risk group (Figure 7F), indicating a possible coexistence of immune cell-mediated tumor killing and tumor cell resistance to immune killing within the low-risk group.
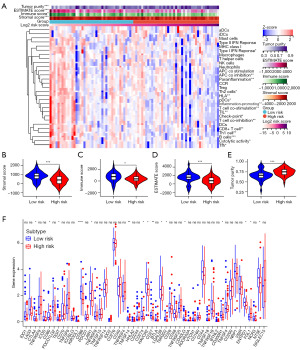
Gene mutations are a key factor influencing tumor heterogeneity and an important predictive indicator for different treatment responses in cancer. Here, we compared the gene mutation profiles of OS patients with different risk scores and found no significant differences in the mutated genes or mutation frequencies (Figure 8A,8B). Furthermore, there was no significant difference in the overall TMB between the low- and high-risk groups (Figure 8C). However, when evaluating drug treatment responsiveness, we observed remarkable variation in the sensitivity of up to 25 targeted or chemotherapy drugs among OS patients with different risk scores (Figure 9). Notably, we discovered a significant increase in sensitivity to cisplatin, a first-line chemotherapy drug for OS, in the low-risk group (Figure 9).
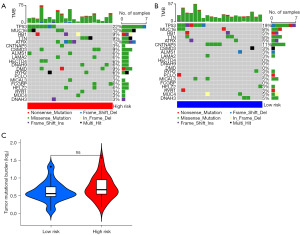
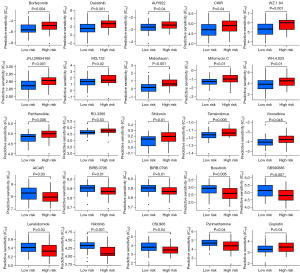
Discussion
In the current study, leveraging bioinformatics analysis of OS sequencing data from the TARGET and GEO databases, we have constructed an EMRPS comprising six EMGs. This signature not only serves as an efficient predictor of OS prognosis but also shows promise in forecasting responses to medical treatments, thereby enhancing our understanding of the disease’s trajectory and potential therapeutic strategies.
Building upon this foundation, our research methodology in the field of OS prognosis notably diverges from that of Liu et al. (29), particularly in our refined selection and analysis of EMGs. Liu et al.’s gene set included a range of non-canonical EMGs, such as transcription factors, whereas our study’s gene set was more narrowly focused on the classic EMGs associated with DNA and histone modifications, aligning with the approach of Wong et al. (30). Although Liu et al. screened for differentially expressed EMGs between TARGET-OS samples and normal skeletal muscle from the GTEx database, we did not perform differential analysis with normal tissues. Instead, we directly targeted EMGs with prognostic relevance in OS. This decision was primarily due to the heterogeneity in the origins of OS, leading us to believe that skeletal muscle, which has relatively uniform biological characteristics, may not be an ideal normal control for OS. Furthermore, the differences in sequencing platforms between the TARGET and GTEx databases also cast doubt on the validity of directly comparing sequencing data from these two types of tissues. Additionally, the bimodal distribution of ages at which OS patients develop the disease contrasts sharply with the predominantly adult population in the GTEx database. Considering these factors collectively, it is inappropriate to use skeletal muscle samples from the GTEx database as controls for OS samples in the TARGET database. Moreover, in terms of potential mechanisms, the EMRPS we developed is significantly associated with immune regulation pathways, whereas the EMG signature constructed by Liu et al. is more closely related to biological pathways such as tumor metabolism. This divergence in potential mechanisms reflects the significant impact that gene selection and analytical methods can have when interpreting epigenetic data within the context of OS prognosis.
Our findings identified six critical EMGs significantly associated with OS survival. The EMRPS, developed based on these genes, was able to identify high-risk patients with poorer survival outcomes, a result consistently validated across internal and external validation sets. This validation underscores the EMRPS’s potential as a robust prognostic tool for OS. Moreover, our results indicate that the EMRPS was independent of age and gender but correlates with metastatic status, a factor that significantly influences survival outcomes in OS patients. Despite age and gender not being independent prognostic factors in our cohort, they were considered in the nomogram construction due to their demographic relevance in OS patient populations (1). The C-index analysis further confirmed the predictive superiority of the nomogram and EMRPS over metastatic status alone, highlighting the EMRPS’s robustness as a prognostic marker and its potential to guide personalized treatment strategies in OS.
Continuing our exploration of the EMRPS’s prognostic value, we delved into the immune characteristics of the TME in OS patients. Our analysis revealed significant differences in immune cell infiltration and molecular expression between low- and high-risk groups as categorized by the EMRPS. Notably, low-risk patients exhibited increased infiltration of immune cells, including B cells, CD8+ T cells, Th1 cells, Th2 cells, and T follicular helper cells. Concurrently, there was a higher expression of co-stimulatory and co-inhibitory signals for APCs and T cells, as well as HLA molecules. These findings suggest a more active and “hot” TME in low-risk patients, indicative of a more robust immune response. The immune checkpoint molecules, which include both immune activation and immune inhibitory molecules, were also more highly expressed in low-risk patients. This points to a complex interplay between immune cell-mediated tumor cell killing and tumor cell immune escape events, potentially influencing the metastatic status and survival outcomes in OS patients.
While the mutational profiles and TMB did not show significant differences across the risk groups defined by the EMRPS, the distinct immune signatures provide a supplementary perspective to TMB. This could be particularly relevant for predicting the efficacy of immunotherapies in OS, an area that requires further validation through multicenter studies with larger patient samples. Building on this premise, our research also shed light on the EMRPS’s role in predicting the sensitivity to target and chemotherapy drugs. We observed that low-risk patients exhibited heightened sensitivity to cisplatin, the standard first-line chemotherapy for OS. Furthermore, these patients also demonstrated an increased response to various targeted or chemotherapy drugs that have shown efficacy in clinical or preclinical settings for other cancer types, such as bortezomib (32,33) and dasatinib (34,35). This correlation suggests that the EMRPS could be a valuable tool in selecting OS patients who are likely to benefit from these specific therapies. The ability of the EMRPS to predict drug sensitivity not only enhances our understanding of OS treatment responses but also paves the way for more personalized treatment strategies. By identifying patients who are more likely to respond favorably to certain drugs, including cisplatin and other targeted therapies, the EMRPS can guide clinicians in tailoring treatments that are most likely to be effective. This approach has the potential to improve patient outcomes by optimizing therapeutic efficacy and minimizing unnecessary side effects from treatments that may not be beneficial.
However, this study also has several limitations. Firstly, although the EMRPS was primarily constructed using EMGs, specifically those associated with DNA and histone modification, the inclusion of non-coding RNA genes (such as lncRNA and microRNA) and RNA modification-related genes (like m6A modification and Ac4C modification) was relatively limited. Secondly, the availability of RNA sequencing data for OS with a sufficient number of diverse samples is scarce, with only the TARGET-OS dataset and the GSE21257 OS sequencing data from GEO providing a relatively larger sample size (n=53) that could serve as an external validation cohort, albeit with certain compromises. Thirdly, the sequencing data from GSE21257 contained significantly fewer EMGs compared to TARGET-OS, mainly due to differences in sequencing depth. Consequently, these missing EMGs were not included in the construction of the EMRPS. Furthermore, the limited resources within our research team prevented us from further validating the predictive performance of the EMRPS in clinical samples. We hope that future studies will continue to investigate the epigenetic mechanisms of OS and conduct experimental research to address these limitations.
Conclusions
This study highlights the significance of epigenetic regulation in OS prognosis and presents a novel risk scoring model (EMRPS) based on EMG markers. The integration of EMRPS and clinical features in the nomogram holds great potential in improving prognostic accuracy, guiding personalized treatment strategies, and ultimately enhancing patient outcomes in OS. Further research is needed to gain a deeper understanding of the role of epigenetic regulation in OS and explore its potential therapeutic implications.
Acknowledgments
Thanks to Dr. Yipei Huang of Guangzhou First People’s Hospital for his help in bioinformatics analysis.
Funding: None.
Footnote
Reporting Checklist: The authors have completed the TRIPOD reporting checklist. Available at https://tcr.amegroups.com/article/view/10.21037/tcr-23-2300/rc
Peer Review File: Available at https://tcr.amegroups.com/article/view/10.21037/tcr-23-2300/prf
Conflicts of Interest: All authors have completed the ICMJE uniform disclosure form (available at https://tcr.amegroups.com/article/view/10.21037/tcr-23-2300/coif). The authors have no conflicts of interest to declare.
Ethical Statement: The authors are accountable for all aspects of the work in ensuring that questions related to the accuracy or integrity of any part of the work are appropriately investigated and resolved. The study was conducted in accordance with the Declaration of Helsinki (as revised in 2013).
Open Access Statement: This is an Open Access article distributed in accordance with the Creative Commons Attribution-NonCommercial-NoDerivs 4.0 International License (CC BY-NC-ND 4.0), which permits the non-commercial replication and distribution of the article with the strict proviso that no changes or edits are made and the original work is properly cited (including links to both the formal publication through the relevant DOI and the license). See: https://creativecommons.org/licenses/by-nc-nd/4.0/.
References
- Beird HC, Bielack SS, Flanagan AM, et al. Osteosarcoma. Nat Rev Dis Primers 2022;8:77. [Crossref] [PubMed]
- Mirabello L, Troisi RJ, Savage SA. International osteosarcoma incidence patterns in children and adolescents, middle ages and elderly persons. Int J Cancer 2009;125:229-34. [Crossref] [PubMed]
- Brown HK, Schiavone K, Gouin F, et al. Biology of Bone Sarcomas and New Therapeutic Developments. Calcif Tissue Int 2018;102:174-95. [Crossref] [PubMed]
- Xin S, Wei G. Prognostic factors in osteosarcoma: A study level meta-analysis and systematic review of current practice. J Bone Oncol 2020;21:100281. [Crossref] [PubMed]
- Bläsius F, Delbrück H, Hildebrand F, et al. Surgical Treatment of Bone Sarcoma. Cancers (Basel) 2022;14:2694. [Crossref] [PubMed]
- Hattinger CM, Salaroglio IC, Fantoni L, et al. Strategies to Overcome Resistance to Immune-Based Therapies in Osteosarcoma. Int J Mol Sci 2023;24:799. [Crossref] [PubMed]
- Zeng M, Zhou J, Wen L, et al. The relationship between the expression of Ki-67 and the prognosis of osteosarcoma. BMC Cancer 2021;21:210. [Crossref] [PubMed]
- Wu X, Cai ZD, Lou LM, et al. Expressions of p53, c-MYC, BCL-2 and apoptotic index in human osteosarcoma and their correlations with prognosis of patients. Cancer Epidemiol 2012;36:212-6. [Crossref] [PubMed]
- Serra M, Maurici D, Scotlandi K, et al. Relationship between P-glycoprotein expression and p53 status in high-grade osteosarcoma. Int J Oncol 1999;14:301-7. [Crossref] [PubMed]
- Zhao H, Wu Y, Chen Y, et al. Clinical significance of hypoxia-inducible factor 1 and VEGF-A in osteosarcoma. Int J Clin Oncol 2015;20:1233-43. [Crossref] [PubMed]
- Zhang Z, Shi Y, Zhu Z, et al. Characterization of myeloid signature genes for predicting prognosis and immune landscape in Ewing sarcoma. Cancer Sci 2023;114:1240-55. [Crossref] [PubMed]
- Liu Z, Xie Y, Zhang C, et al. Survival nomogram for osteosarcoma patients: SEER data retrospective analysis with external validation. Am J Cancer Res 2023;13:900-11. [PubMed]
- Tang H, Liu S, Luo X, et al. A novel molecular signature for predicting prognosis and immunotherapy response in osteosarcoma based on tumor-infiltrating cell marker genes. Front Immunol 2023;14:1150588. [Crossref] [PubMed]
- Strepkos D, Markouli M, Klonou A, et al. Histone Methyltransferase SETDB1: A Common Denominator of Tumorigenesis with Therapeutic Potential. Cancer Res 2021;81:525-34. [Crossref] [PubMed]
- Li B, Wang Z, Wu H, et al. Epigenetic Regulation of CXCL12 Plays a Critical Role in Mediating Tumor Progression and the Immune Response In Osteosarcoma. Cancer Res 2018;78:3938-53. [Crossref] [PubMed]
- Sun R, Shen J, Gao Y, et al. Overexpression of EZH2 is associated with the poor prognosis in osteosarcoma and function analysis indicates a therapeutic potential. Oncotarget 2016;7:38333-46. [Crossref] [PubMed]
- Twenhafel L, Moreno D, Punt T, et al. Epigenetic Changes Associated with Osteosarcoma: A Comprehensive Review. Cells 2023;12:1595. [Crossref] [PubMed]
- Lillo Osuna MA, Garcia-Lopez J, El Ayachi I, et al. Activation of Estrogen Receptor Alpha by Decitabine Inhibits Osteosarcoma Growth and Metastasis. Cancer Res 2019;79:1054-68. [Crossref] [PubMed]
- Ulaner GA, Vu TH, Li T, et al. Loss of imprinting of IGF2 and H19 in osteosarcoma is accompanied by reciprocal methylation changes of a CTCF-binding site. Hum Mol Genet 2003;12:535-49. [Crossref] [PubMed]
- La Noce M, Paino F, Mele L, et al. HDAC2 depletion promotes osteosarcoma's stemness both in vitro and in vivo: a study on a putative new target for CSCs directed therapy. J Exp Clin Cancer Res 2018;37:296. [Crossref] [PubMed]
- Chang SL, Lee CW, Yang CY, et al. IOX-1 suppresses metastasis of osteosarcoma by upregulating histone H3 lysine trimethylation. Biochem Pharmacol 2023;210:115472. [Crossref] [PubMed]
- Kong D, Ying B, Zhang J, et al. PCAF regulates H3 phosphorylation and promotes autophagy in osteosarcoma cells. Biomed Pharmacother 2019;118:109395. [Crossref] [PubMed]
- Yang D, Xu T, Fan L, et al. microRNA-216b enhances cisplatin-induced apoptosis in osteosarcoma MG63 and SaOS-2 cells by binding to JMJD2C and regulating the HIF1α/HES1 signaling axis. J Exp Clin Cancer Res 2020;39:201. Erratum in: J Exp Clin Cancer Res 2021;40:17. [Crossref] [PubMed]
- De Vito C, Riggi N, Cornaz S, et al. A TARBP2-dependent miRNA expression profile underlies cancer stem cell properties and provides candidate therapeutic reagents in Ewing sarcoma. Cancer Cell 2012;21:807-21. [Crossref] [PubMed]
- Gong H, Tao Y, Xiao S, et al. LncRNA KIAA0087 suppresses the progression of osteosarcoma by mediating the SOCS1/JAK2/STAT3 signaling pathway. Exp Mol Med 2023;55:831-43. [Crossref] [PubMed]
- Moonmuang S, Chaiyawat P, Jantrapirom S, et al. Circulating Long Non-Coding RNAs as Novel Potential Biomarkers for Osteogenic Sarcoma. Cancers (Basel) 2021;13:4214. [Crossref] [PubMed]
- Lu J, Annunziata F, Sirvinskas D, et al. Establishment and evaluation of module-based immune-associated gene signature to predict overall survival in patients of colon adenocarcinoma. J Biomed Sci 2022;29:81. [Crossref] [PubMed]
- Wang R, Zheng J, Shao X, et al. Development of a prognostic composite cytokine signature based on the correlation with nivolumab clearance: translational PK/PD analysis in patients with renal cell carcinoma. J Immunother Cancer 2019;7:348. [Crossref] [PubMed]
- Liu S, Wu B, Li X, et al. Construction and Validation of a Potent Epigenetic Modification-Related Prognostic Signature for Osteosarcoma Patients. J Oncol 2021;2021:2719172. [Crossref] [PubMed]
- Wong CM, Wei L, Law CT, et al. Up-regulation of histone methyltransferase SETDB1 by multiple mechanisms in hepatocellular carcinoma promotes cancer metastasis. Hepatology 2016;63:474-87. [Crossref] [PubMed]
- Buddingh EP, Kuijjer ML, Duim RA, et al. Tumor-infiltrating macrophages are associated with metastasis suppression in high-grade osteosarcoma: a rationale for treatment with macrophage activating agents. Clin Cancer Res 2011;17:2110-9. [Crossref] [PubMed]
- LoRusso PM, Venkatakrishnan K, Ramanathan RK, et al. Pharmacokinetics and safety of bortezomib in patients with advanced malignancies and varying degrees of liver dysfunction: phase I NCI Organ Dysfunction Working Group Study NCI-6432. Clin Cancer Res 2012;18:2954-63. [Crossref] [PubMed]
- Sonneveld P, Chanan-Khan A, Weisel K, et al. Overall Survival With Daratumumab, Bortezomib, and Dexamethasone in Previously Treated Multiple Myeloma (CASTOR): A Randomized, Open-Label, Phase III Trial. J Clin Oncol 2023;41:1600-9. [Crossref] [PubMed]
- Hunger SP, Tran TH, Saha V, et al. Dasatinib with intensive chemotherapy in de novo paediatric Philadelphia chromosome-positive acute lymphoblastic leukaemia (CA180-372/COG AALL1122): a single-arm, multicentre, phase 2 trial. Lancet Haematol 2023;10:e510-20. [Crossref] [PubMed]
- Foà R, Bassan R, Vitale A, et al. Dasatinib-Blinatumomab for Ph-Positive Acute Lymphoblastic Leukemia in Adults. N Engl J Med 2020;383:1613-23. [Crossref] [PubMed]