Development and validation of a glioma prognostic model based on telomere-related genes and immune infiltration analysis
Highlight box
Key findings
• We constructed prognostic models with ten telomere-related genes (GANAB, PPP1R1B, CDC73, IGF2BP3, FEN1, RMI2, HNRNPA0, ANXA2, LCK, ZGPAT) and validated eight of them in the Human Protein Atlas (HPA) database.
• Three R packages for analysis of differences were used to take the intersection of the results; and the prognostic analysis of the difference genes was performed by univariate Cox analysis and log-rank tests, which increased the reliability of the prognostic model genes.
• The study showed that the abnormal expression of telomere related genes has a significant effect on the prognosis of glioma patients, which provides reference for the treatment of glioma by telomere targets.
What is known and what is new?
• Increasing evidence suggests that telomere maintenance mechanisms play a crucial role in glioma development.
• This study aimed to construct a prognostic model of telomere-related genes and further elucidate the potential association between the two.
What is the implication, and what should change now?
• This study successfully constructed a prognostic model of telomere-related genes, which can more accurately predict glioma patient prognosis, offer potential targets and a theoretical basis for glioma treatment, and serve as a reference for immunotherapy through immune infiltration analysis.
Introduction
Gliomas represent the most prevalent malignant tumors in the central nervous system and are classified by the World Health Organization as low-grade gliomas (LGGs) and high-grade gliomas (HGGs). Although LGGs exhibit a more favorable prognosis compared to HGGs, treatment resistance and tumor recurrence remain inevitable following a combination of surgery, radiotherapy, and chemotherapy. Consequently, over half of the patients will ultimately develop highly aggressive gliomas (1-3). Glioblastoma (GBM) is the most common and aggressive grade IV glioma, characterized by the highest malignancy, poorest prognosis, and lowest overall survival rate. The median survival time for GBM patients is approximately fifteen months, with a five-year survival rate of about 7.2% (4,5). Despite comprehensive multimodal treatment approaches, significant breakthroughs remain elusive, primarily due to factors such as tumor heterogeneity, tumor microenvironment, and the blood-brain barrier. Thus, a comprehensive understanding of the molecular mechanisms underlying glioma development will provide novel theoretical foundations and insights for glioma diagnosis, treatment, and prognosis.
Human telomeres are located at the ends of chromosomes and consist of DNA repetitive sequences (TTAGGG) and several related telomere-binding proteins (6). Cell division results in telomere shortening, and when telomere length falls below a critical threshold, cells undergo senescence and apoptosis (7). However, cancer cells can circumvent progressive telomere shortening and activate telomere maintenance mechanisms (TMM) to preserve telomere length (8). Currently, two TMMs have been identified in cancer research: the most common mechanism, telomerase activation (9), and the alternative lengthening of telomeres (ALT) mechanism, which does not rely on telomerase to extend telomeres (10). Studies have indicated that TMM activity in osteosarcoma and neuroblastoma may exhibit intratumor heterogeneity, with ALT and telomerase functioning in different cells within the same tumor (11,12).
Gliomas have a strong association with TMM, and alterations in telomerase reverse transcriptase (TERT), specifically TERT promoter (TERTp) mutations, are considered the primary mechanism of telomerase activation in both GBM and LGG (13-15). ALT is typically observed in World Health Organization (WHO) grade II or III isocitrate dehydrogenase (IDH)-mutated astrocytomas and IDH-mutated GBMs (16), and abnormalities in the ATRX/DAXX/H3.3 complex have been found to be closely related to the ALT mechanism (17). Based on these findings, it is evident that TMM plays a significant role in glioma development; however, the prognostic role of telomere-related genes in glioma remains unclear. Therefore, in this study, we aimed to construct a prognostic model of telomere-related genes in glioma to provide novel research targets and insights for telomere-related therapy in glioma management. We present this article in accordance with the TRIPOD reporting checklist (available at https://tcr.amegroups.com/article/view/10.21037/tcr-23-2294/rc).
Methods
Data collection and processing
RNA-seq data and clinical follow-up information for LGG and GBM were obtained from The Cancer Genome Atlas (TCGA) database (https://portal.gdc.cancer.gov/). Cases with unknown survival dates or life and death statuses were excluded, resulting in a total of 664 cases for the training set. Similarly, RNA-seq data and corresponding clinical information for the Chinese Glioma Genome Atlas (CGGA) cohorts (mRNAseq_693, mRNAseq_325) were acquired from the CGGA database (http://www.cgga.org.cn) as the validation set. Moreover, 1,148 normal brain samples were procured from the Genotype-Tissue Expression (GTEx) database via the UCSC Xena website (https://xenabrowser.net/datapages/) for differential analysis. Telomere-associated genes were sourced from www.cancertelsys.org/telnet (18). Batch effects were removed using the limma package. This study was conducted in accordance with the Declaration of Helsinki (as revised in 2013).
Differential expression and functional enrichment analysis
By integrating the RNA-seq data for LGG and GBM from the TCGA database with the 1,148 normal brain samples from the GTEx database on the UCSC XENA website, we conducted a differential analysis using the DESeq2, limma, and edgeR packages, with a threshold at P<0.05 and |logfold change (FC)| >1. Overlapping differentially expressed genes were identified using Venn diagrams for subsequent analysis. Additionally, we intersected telomere-related genes with the differentially expressed genes. The ClusterProfiler R package was then employed for Gene Ontology (GO) and Kyoto Encyclopedia of Genes and Genomes (KEGG) enrichment analyses (19,20), with an adjusted P value threshold of <0.05.
Construction of telomere-related gene risk model
To construct a risk model for telomere-related genes, we performed univariate Cox regression analysis, log-rank test, least absolute shrinkage and selection operator (LASSO) regression analysis, and multivariate Cox regression analysis on telomere-associated differentially expressed genes. Genes with a P value <0.05 were deemed to have significant prognostic value, resulting in the identification of 10 prognostic-associated genes. We then calculated the risk score using the formula: risk score = gene expression level 1 × β1 + gene expression level 2 × β2 + ... + gene expression level n × βn, where β is the regression coefficient for each gene determined by multivariate Cox regression. Based on median risk scores, patients were stratified into high-risk and low-risk groups. The relationship between risk groups and overall survival (OS) was assessed through Kaplan-Meier (K-M) survival analysis, and the accuracy of predicting patient outcomes at 1, 3, and 5 years was evaluated using receiver operating characteristic (ROC) curves with the timeROC R package.
Assessment of independent prognostic factors and construction of nomogram
We conducted a multivariate Cox regression analysis, incorporating risk scores and clinicopathological characteristics (e.g., sex, age), to determine if telomere-related risk models could serve as independent prognostic factors. A nomogram was subsequently constructed using the rms R package (21).
Immune infiltration analysis
CIBERSORT (22) is a method for estimating and analyzing immune cell infiltration based on the principle of deconvolution. We employed CIBERSORT to estimate the proportions of immune cell types in the low-risk and high-risk groups, setting the number of permutations to 1,000 and using a P value threshold of <0.05 for screening.
Immunohistochemistry
To validate the expression of candidate genes in the prognostic models for gliomas, we obtained immunohistochemical images of protein-level expression for eight prognostic genes in normal brain tissue and glioma tissue from the Human Protein Atlas (HPA) (www.proteinatlas.org).
Statistical analysis
Statistical analyses and chart generation were conducted using R software (version 4.1.3). The R package “survival” was utilized for univariate and multivariate Cox regression analyses to evaluate prognostic value; the R package “survminer” was employed for Kaplan-Meier survival analysis to estimate OS between patient groups; the R package “rms” was used for creating nomograms and calibration curves; and the R package “timeROC” was used for generating ROC curves to assess the accuracy of patient outcome predictions. A P value <0.05 was considered statistically significant.
Results
Identification of telomere-related differentially expressed genes in glioma patients and enrichment analysis
We integrated glioma patient samples with normal brain tissue samples and assessed the differences using the DESeq2, limma, and edgeR packages, respectively (Figure 1A-1C), applying thresholds of P<0.05 and |logFC| >1. We then identified overlapping differentially expressed genes through a Venn diagram, resulting in a total of 2,318 downregulated and 2,836 upregulated differentially expressed genes (Figure 2A,2B). By intersecting the 2,086 telomere-related genes acquired from TelNet with the aforementioned differentially expressed genes and requiring gene expression levels to be greater than 0 in at least 350 samples, we ultimately obtained 214 upregulated and 282 downregulated genes. Furthermore, we conducted functional enrichment analysis of the telomere-related differentially expressed genes. In the GO enrichment analysis, biological processes (BP) (Figure 3A) were primarily linked to DNA recombination and replication, DNA conformational change, telomere maintenance, regulation of DNA biosynthesis process, and negative regulation of cell cycle process. Cellular components (CC) (Figure 3B) were predominantly associated with chromosome (telomeric region) and DNA replication preinitiation complex, while molecular functions (MF) (Figure 3C) were chiefly related to catalytic activity acting on DNA, protein serine/threonine kinase activity, ATP hydrolysis activity, and telomeric DNA binding. The KEGG enrichment analysis (Figure 3D) indicated that the main pathways involved were DNA replication, cell cycle, homologous recombination, cellular senescence, and calcium signaling pathway.
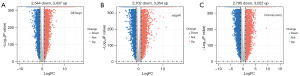
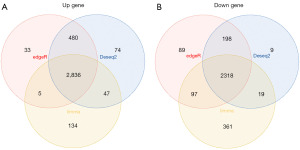
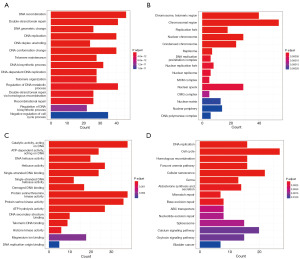
Construction and validation of a prognostic model for telomere-related genes
A total of 496 differentially expressed genes were subjected to univariate Cox regression and log-rank test analyses, resulting in the identification of 372 genes associated with glioma prognosis. To validate these prognostic genes in the CGGA-693 and CGGA-325 datasets, univariate Cox regression and log-rank test analyses were performed on both datasets, and the prognostic genes from all three datasets were intersected, yielding 252 genes. To avoid overfitting and enhance prediction accuracy, the 252 prognostic genes were subjected to LASSO regression analysis. With an optimal λ value of 0.01033, 43 genes were obtained for further investigation (Figure 4A,4B). Ultimately, 10 genes significantly linked to poor prognosis were identified through multivariate Cox regression analysis (Figure 5). In Figure 5, GANAB, PPP1R1B, IGF2BP3, RMI2 and ANXA2 are located on the right side of the invalid line, with HR >1, indicating that glioma patients have increased expression levels of these genes compared with normal population, suggesting poor prognosis and belonging to risk factors. CDC73, FEN1 and HNRNPA0 were located on the left side of the null line, with HR <1, indicating that glioma patients had lower expression levels of these genes compared with the normal population, indicating poor prognosis and belonging to protective factors. The risk score for each patient was calculated using the formula [risk score = GANAB×1.32108 + PPP1R1B×0.14476 + CDC73×(−0.84312) + IGF2BP3×0.18173 + FEN1×(−0.53136) + RMI2×0.54494 + HNRNPA0×(−0.95043) + ANXA2×0.25578 + LCK×(−0.08713) + ZGPAT×0.08506]. Patients were then classified into high-risk and low-risk groups based on median risk score values. Kaplan-Meier survival analysis was performed on both patient groups, revealing that the OS was significantly higher in the low-risk group compared to the high-risk group in the TCGA dataset, CGGA-693 dataset, and CGGA-325 dataset (Figure 6A-6C). The difference in survival curves between the low-risk and high-risk groups was statistically significant (P<0.001). Furthermore, the distribution of risk scores, survival status, and risk gene expression for the TCGA cohort and the two validation cohorts are depicted in the figure (Figure 7A-7C).
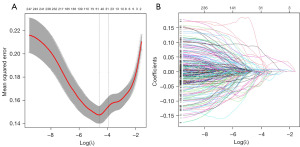
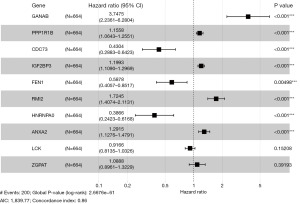
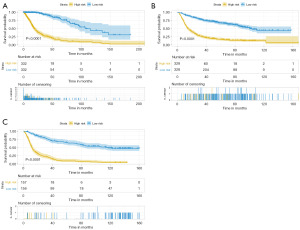
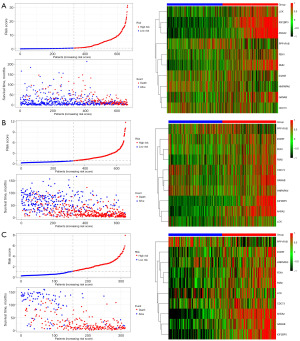
Utilization of telomere-related risk scores as independent prognostic factors and construction of a nomogram
To determine whether telomere-related risk scores can function as an independent predictor of OS in patients, we incorporated age, gender, and risk score into a multivariate Cox regression analysis. The findings demonstrated that both risk score and age were independent prognostic factors for glioma patients (Figure 8A-8C). Subsequently, we evaluated the sensitivity and specificity of this prognostic model using time-dependent ROC curves. The 1-, 3-, and 5-year area under the curve (AUC) values for the TCGA cohort (Figure 9A) were 0.878, 0.920, and 0.902, respectively. For the CGGA-693 cohort (Figure 9B), the 1-year, 3-year, and 5-year AUC values were 0.768, 0.822, and 0.839, respectively, while for the CGGA-325 cohort (Figure 9C), they were 0.795, 0.866, and 0.899, respectively. These results indicate strong predictive performance. We subsequently constructed a nomogram (Figure 10), and calibration curves at 1, 3, and 5 years revealed the nomogram accurately predicted the survival time of patients in the TCGA cohort (Figure 11A). Similar results were observed in the CGGA-693 and CGGA-325 cohorts (Figure 11B,11C).
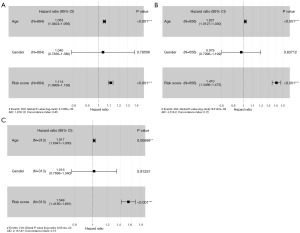
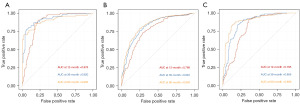
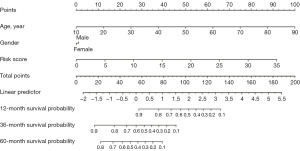
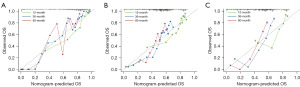
Immune infiltration analysis
We employed the CIBERSORT algorithm to assess the differences in immune cell proportions between high-risk and low-risk groups in the TCGA cohort. Notably, the high-risk group exhibited increased proportions of T cells CD4 memory resting, NK cells resting, NK cells activated, macrophages M1, macrophages M2, mast cells resting, and neutrophils compared to the low-risk group. In contrast, the high-risk group demonstrated lower proportions of B cells naïve, plasma cells, T cells CD4 naïve, T cells regulatory (Tregs), T cells gamma delta, dendritic cells resting, mast cells activated, and eosinophils (Figure 12).
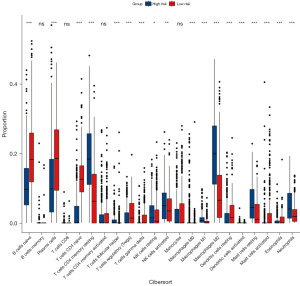
Immunohistochemistry
To validate protein-level expression in normal brain tissue and glioma tissue, we examined GANAB (Figure 13A,13B), PPP1R1B (Figure 13C,13D), CDC73 (Figure 14A,14B), IGF2BP3 (Figure 14C,14D), FEN1 (Figure 15A,15B), RMI2 (Figure 15C,15D), HNRNPA0 (Figure 16A,16B), and ANXA2 (Figure 16C,16D) using the HPA database for immunohistochemical analysis.
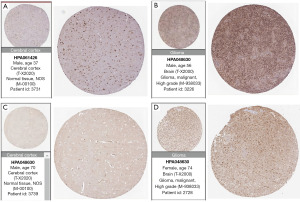
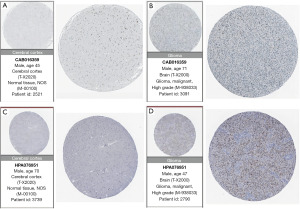
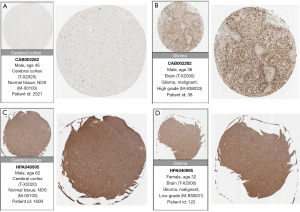
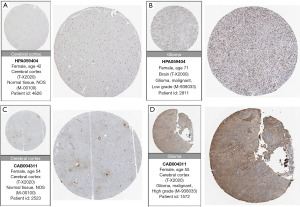
Discussion
Gliomas represent approximately 30% of primary brain tumors and 80% of malignant brain tumors, with their pathogenesis remaining unclear (23). Fluorescence-guided techniques have been employed to enhance tumor resection rates (24,25), and a combination of multiple modalities, including radiotherapy, immunotherapy, targeted therapy, and tumor electric field therapy, has been utilized (26). However, these approaches have not significantly improved the survival and prognosis of patients with high-grade gliomas. The release of the fifth edition of the World Health Organization Classification of Tumors of the Central Nervous System (WHO CNS5) (27) emphasized the importance of molecular markers in classifying CNS tumors, thereby providing a foundation for precise treatment.
Research has demonstrated that TMM can grant cancer cells the ability to proliferate indefinitely and inhibit senescence (28). In glioma, the telomerase activation mechanism and the ALT mechanism are particularly significant. Nonetheless, the impact of telomere-related genes on glioma remains inadequately understood. Consequently, this study aimed to identify differentially expressed telomere-related genes within glioma datasets and construct a prognostic model. This model may offer insights for discovering tumor molecular markers to evaluate prognostic effects and identify potential drug targets for inhibiting glioma proliferation.
In this study, we analyzed glioma sequencing data and corresponding clinical data from the TCGA and CGGA databases, identifying 496 differentially expressed telomere-related genes, including 214 upregulated and 282 downregulated genes. GO and KEGG enrichment analyses revealed that these genes were closely associated with DNA replication, telomere maintenance, and cell cycle regulation. Utilizing univariate Cox regression analysis, log-rank test, LASSO regression analysis, and multivariate Cox regression analysis, we constructed a prognostic model comprising 10 telomere-related genes (GANAB, PPP1R1B, CDC73, IGF2BP3, FEN1, RMI2, HNRNPA0, ANXA2, LCK, and ZGPAT). IGF2BP3, a member of the conserved IGF2BP family, has been demonstrated to play a crucial role in maintaining and promoting tumor heterogeneity in GBM (29). Zhang et al. (30) also discovered a significant positive correlation between IGF2BP3 expression and glioma grading. FEN1, a structure-specific endonuclease and a typical member of the Rad2 nuclease family (31), is involved in regulatory processes such as Okazaki fragment maturation, base excision repair (BER), telomere stability maintenance, and stalled DNA replication fork repair (32). The study has shown that FEN1 silencing can enhance cisplatin sensitivity in gliomas (33), offering potential strategies for glioma treatment. Annexin A2 (ANXA2), a calcium-dependent phospholipid-binding protein, has been found to be expressed on the surface of various cancer cells (34) and is implicated in numerous pathophysiological processes, including epithelial-mesenchymal transition, tumor proliferation, migration, and cancer drug resistance (35,36). AXNA2 is also closely related to glioma grade and poor prognosis of GBM (37), and its expression is closely associated with glioma-related inflammatory activity and immunosuppression (38). Lymphocyte-specific protein tyrosine kinase (LCK) is a member of the Src family (39) and plays a significant role in cell cycle control, cell adhesion, migration, and proliferation (40). Evidence suggests that LCK-targeted inhibitors can modulate human glioma cell migration, tumor growth, and stemness gene expression (41). Moreover, LCK is involved in the expansion of radiation-induced glioma-initiating cell populations and contributes to decreased cell sensitivity to anticancer therapy (42). Furthermore, we stratified patients into high-risk and low-risk categories based on median risk score calculations. Kaplan-Meier survival analyses within the TCGA, CGGA-693, and CGGA-325 datasets consistently demonstrated that OS was significantly greater for the low-risk group compared to the high-risk group, with both exhibiting statistical differences. Utilizing multivariate Cox regression analysis, we confirmed that the risk score served as an independent predictor for glioma prognosis. Subsequently, we developed a nomogram to estimate OS for glioma patients, and calibration curve validation indicated robust predictive performance. In accordance with the CIBERSORT algorithm, we observed statistically significant differences in the proportions of various immune cells between the high-risk and low-risk groups, offering insights for immunotherapy strategies in these distinct risk categories. Lastly, we validated eight candidate genes within the HPA database, which will facilitate the identification of reliable genes for future experimental investigations.
Conclusions
In the present investigation, we developed a glioma prognostic model centered on telomere-associated genes utilizing bioinformatics analysis, substantiating its dependability using three distinct datasets. This offers a robust foundation for clinical investigations and experimental confirmation. Despite these advancements, several limitations persist in this study. Future work will involve further validation of the identified candidate genes and exploration of their intrinsic regulatory mechanisms through cellular and animal experimentation. Additionally, large-scale population sequencing remains necessary to corroborate the reliability of candidate genes and evaluate their potential influence on glioma patient outcomes.
Acknowledgments
We want to thank the data contributors and curators of the TCGA, GTEx, CGGA, HPA databases.
Funding: None.
Footnote
Reporting Checklist: The authors have completed the TRIPOD reporting checklist. Available at https://tcr.amegroups.com/article/view/10.21037/tcr-23-2294/rc
Peer Review File: Available at https://tcr.amegroups.com/article/view/10.21037/tcr-23-2294/prf
Conflicts of Interest: All authors have completed the ICMJE uniform disclosure form (available at https://tcr.amegroups.com/article/view/10.21037/tcr-23-2294/coif). The authors have no conflicts of interest to declare.
Ethical Statement: The authors are accountable for all aspects of the work in ensuring that questions related to the accuracy or integrity of any part of the work are appropriately investigated and resolved. The study was conducted in accordance with the Declaration of Helsinki (as revised in 2013).
Open Access Statement: This is an Open Access article distributed in accordance with the Creative Commons Attribution-NonCommercial-NoDerivs 4.0 International License (CC BY-NC-ND 4.0), which permits the non-commercial replication and distribution of the article with the strict proviso that no changes or edits are made and the original work is properly cited (including links to both the formal publication through the relevant DOI and the license). See: https://creativecommons.org/licenses/by-nc-nd/4.0/.
References
- Zhang YA, Zhou Y, Luo X, et al. SHOX2 is a Potent Independent Biomarker to Predict Survival of WHO Grade II-III Diffuse Gliomas. EBioMedicine 2016;13:80-9. [Crossref] [PubMed]
- Cancer Genome Atlas Research Network. Comprehensive, Integrative Genomic Analysis of Diffuse Lower-Grade Gliomas. N Engl J Med 2015;372:2481-98. [Crossref] [PubMed]
- Hayes J, Yu Y, Jalbert LE, et al. Genomic analysis of the origins and evolution of multicentric diffuse lower-grade gliomas. Neuro Oncol 2018;20:632-41. [Crossref] [PubMed]
- Li M, Song X, Zhu J, et al. The interventional effect of new drugs combined with the Stupp protocol on glioblastoma: A network meta-analysis. Clin Neurol Neurosurg 2017;159:6-12. [Crossref] [PubMed]
- Li Y, Sharma A, Maciaczyk J, et al. Recent Development in NKT-Based Immunotherapy of Glioblastoma: From Bench to Bedside. Int J Mol Sci 2022;23:1311. [Crossref] [PubMed]
- Walsh KM, Wiencke JK, Lachance DH, et al. Telomere maintenance and the etiology of adult glioma. Neuro Oncol 2015;17:1445-52. [Crossref] [PubMed]
- Bodnar AG, Ouellette M, Frolkis M, et al. Extension of life-span by introduction of telomerase into normal human cells. Science 1998;279:349-52. [Crossref] [PubMed]
- De Vitis M, Berardinelli F, Sgura A. Telomere Length Maintenance in Cancer: At the Crossroad between Telomerase and Alternative Lengthening of Telomeres (ALT). Int J Mol Sci 2018;19:606. [Crossref] [PubMed]
- Shay JW, Wright WE. Role of telomeres and telomerase in cancer. Semin Cancer Biol 2011;21:349-53. [Crossref] [PubMed]
- Bryan TM, Englezou A, Dalla-Pozza L, et al. Evidence for an alternative mechanism for maintaining telomere length in human tumors and tumor-derived cell lines. Nat Med 1997;3:1271-4. [Crossref] [PubMed]
- Gocha AR, Nuovo G, Iwenofu OH, et al. Human sarcomas are mosaic for telomerase-dependent and telomerase-independent telomere maintenance mechanisms: implications for telomere-based therapies. Am J Pathol 2013;182:41-8. [Crossref] [PubMed]
- Pezzolo A, Pistorio A, Gambini C, et al. Intratumoral diversity of telomere length in individual neuroblastoma tumors. Oncotarget 2015;6:7493-503. [Crossref] [PubMed]
- Arita H, Narita Y, Takami H, et al. TERT promoter mutations rather than methylation are the main mechanism for TERT upregulation in adult gliomas. Acta Neuropathol 2013;126:939-41. [Crossref] [PubMed]
- Lee DD, Leão R, Komosa M, et al. DNA hypermethylation within TERT promoter upregulates TERT expression in cancer. J Clin Invest 2019;129:223-9. [Crossref] [PubMed]
- Shay JW, Wright WE. Telomeres and telomerase: three decades of progress. Nat Rev Genet 2019;20:299-309. [Crossref] [PubMed]
- Pekmezci M, Rice T, Molinaro AM, et al. Adult infiltrating gliomas with WHO 2016 integrated diagnosis: additional prognostic roles of ATRX and TERT. Acta Neuropathol 2017;133:1001-16. [Crossref] [PubMed]
- Fan HC, Chen CM, Chi CS, et al. Targeting Telomerase and ATRX/DAXX Inducing Tumor Senescence and Apoptosis in the Malignant Glioma. Int J Mol Sci 2019;20:200. [Crossref] [PubMed]
- Braun DM, Chung I, Kepper N, et al. TelNet - a database for human and yeast genes involved in telomere maintenance. BMC Genet 2018;19:32. [Crossref] [PubMed]
- Kanehisa M, Goto S. KEGG: kyoto encyclopedia of genes and genomes. Nucleic Acids Res 2000;28:27-30. [Crossref] [PubMed]
- Yu G, Wang LG, Han Y, et al. clusterProfiler: an R package for comparing biological themes among gene clusters. OMICS 2012;16:284-7. [Crossref] [PubMed]
- Iasonos A, Schrag D, Raj GV, et al. How to build and interpret a nomogram for cancer prognosis. J Clin Oncol 2008;26:1364-70. [Crossref] [PubMed]
- Newman AM, Liu CL, Green MR, et al. Robust enumeration of cell subsets from tissue expression profiles. Nat Methods 2015;12:453-7. [Crossref] [PubMed]
- Chen R, Smith-Cohn M, Cohen AL, et al. Glioma Subclassifications and Their Clinical Significance. Neurotherapeutics 2017;14:284-97. [Crossref] [PubMed]
- Díez Valle R, Tejada Solis S, Idoate Gastearena MA, et al. Surgery guided by 5-aminolevulinic fluorescence in glioblastoma: volumetric analysis of extent of resection in single-center experience. J Neurooncol 2011;102:105-13. [Crossref] [PubMed]
- Eljamel S. 5-ALA Fluorescence Image Guided Resection of Glioblastoma Multiforme: A Meta-Analysis of the Literature. Int J Mol Sci 2015;16:10443-56. [Crossref] [PubMed]
- Dongpo S, Zhengyao Z, Xiaozhuo L, et al. Efficacy and Safety of Bevacizumab Combined with Other Therapeutic Regimens for Treatment of Recurrent Glioblastoma: A Network Meta-analysis. World Neurosurg 2022;160:e61-79. [Crossref] [PubMed]
- Louis DN, Perry A, Wesseling P, et al. The 2021 WHO Classification of Tumors of the Central Nervous System: a summary. Neuro Oncol 2021;23:1231-51. [Crossref] [PubMed]
- Blasco MA. Telomeres and human disease: ageing, cancer and beyond. Nat Rev Genet 2005;6:611-22. [Crossref] [PubMed]
- Dixit D, Prager BC, Gimple RC, et al. The RNA m6A Reader YTHDF2 Maintains Oncogene Expression and Is a Targetable Dependency in Glioblastoma Stem Cells. Cancer Discov 2021;11:480-99. [Crossref] [PubMed]
- Zhang GH, Zhong QY, Gou XX, et al. Seven genes for the prognostic prediction in patients with glioma. Clin Transl Oncol 2019;21:1327-35. [Crossref] [PubMed]
- Hopkins JL, Lan L, Zou L. DNA repair defects in cancer and therapeutic opportunities. Genes Dev 2022;36:278-93. [Crossref] [PubMed]
- Yang F, Hu Z, Guo Z. Small-Molecule Inhibitors Targeting FEN1 for Cancer Therapy. Biomolecules 2022;12:1007. [Crossref] [PubMed]
- Chen YD, Zhang X, Qiu XG, et al. Functional FEN1 genetic variants and haplotypes are associated with glioma risk. J Neurooncol 2013;111:145-51. [Crossref] [PubMed]
- Sharma MC. Annexin A2 (ANX A2): An emerging biomarker and potential therapeutic target for aggressive cancers. Int J Cancer 2019;144:2074-81. [Crossref] [PubMed]
- Huang Y, Jia M, Yang X, et al. Annexin A2: The diversity of pathological effects in tumorigenesis and immune response. Int J Cancer 2022;151:497-509. [Crossref] [PubMed]
- Wang T, Wang Z, Niu R, et al. Crucial role of Anxa2 in cancer progression: highlights on its novel regulatory mechanism. Cancer Biol Med 2019;16:671-87. [Crossref] [PubMed]
- Gonias SL, Zampieri C. Plasminogen Receptors in Human Malignancies: Effects on Prognosis and Feasibility as Targets for Drug Development. Curr Drug Targets 2020;21:647-56. [Crossref] [PubMed]
- Ma K, Chen X, Liu W, et al. ANXA2 is correlated with the molecular features and clinical prognosis of glioma, and acts as a potential marker of immunosuppression. Sci Rep 2021;11:20839. [Crossref] [PubMed]
- Bommhardt U, Schraven B, Simeoni L. Beyond TCR Signaling: Emerging Functions of Lck in Cancer and Immunotherapy. Int J Mol Sci 2019;20:3500. [Crossref] [PubMed]
- Ge L, Xu L, Lu S, et al. LCK expression is a potential biomarker for distinguishing primary central nervous system lymphoma from glioblastoma multiforme. FEBS Open Bio 2020;10:904-11. [Crossref] [PubMed]
- Zepecki JP, Snyder KM, Moreno MM, et al. Regulation of human glioma cell migration, tumor growth, and stemness gene expression using a Lck targeted inhibitor. Oncogene 2019;38:1734-50. [Crossref] [PubMed]
- Kim RK, Yoon CH, Hyun KH, et al. Role of lymphocyte-specific protein tyrosine kinase (LCK) in the expansion of glioma-initiating cells by fractionated radiation. Biochem Biophys Res Commun 2010;402:631-6. [Crossref] [PubMed]