The role of dysregulated metabolism and associated genes in gastric cancer initiation and development
Introduction
Gastric cancer (GC) remains a significant global health issue, ranking as the fifth-most common cancer worldwide and the fourth-leading cause of cancer-related deaths. Notably, the highest incidence of GC is observed in East Asia, including countries such as Japan, Korea, and China (1,2). GC remains a significant global healthcare challenge, with more than 1 million new cases reported worldwide annually. A substantial proportion of GC cases are associated with various pathogenic infections, including Helicobacter pylori and Epstein-Barr virus (3).
Abnormal metabolism is increasingly recognized as a significant hallmark of cancer, as supported by a growing body of evidence from clinical and laboratory studies (4). Among the enzymes implicated in metabolic dysregulation across various types of cancer, lactate dehydrogenase (LDH) stands out as an important player (5). Metabolites exert intracellular activity by interacting with genes and proteins, with alterations in upstream macromolecules ultimately manifested at the metabolic level. These changes encompass neurotransmitter modifications, hormonal regulation, receptor actions, cellular signaling release, energy transfer, and intercellular communication (6).
In the context of GC, metabolic disorders assume a pivotal role in its development, treatment, and prognosis (7). Studies have revealed elevated expression of proteins associated with lipid metabolism in numerous cancers (8). Epidemiological investigations have identified a higher prevalence of GC among obese populations, while pathological tissues of GC exhibit lipid accumulation (9).
Metabolic disorders have a significant impact on the emergence and progression of tumors. The metabolic processes in normal cells are tightly regulated to ensure the necessary energy and materials for cellular function and survival. However, cancer cells exhibit abnormal changes in their metabolic network, resulting in disrupted energy production, substance synthesis, and regulation of signal transmission. These metabolic disorders play a critical role in tumor development (10).
Understanding the significance of metabolic disorders in tumor development enables us to develop novel treatment strategies (11). By targeting the metabolic characteristics of tumor cells, such as glycolysis inhibitors and drugs that target lipid metabolism, we can disrupt the survival and proliferation of cancer cells, thus providing more effective treatment options (12). In this review, we discuss the role of dysregulated metabolism and associated genes in GC initiation and development.
Dysregulated metabolism and GC
Characteristics of metabolic reprogramming
Abnormal glycolysis
Glycolysis is the process by which cells generate energy, typically oxidizing glucose to produce carbon dioxide and water while simultaneously producing a large amount of ATP, a high-energy molecule (13). However, in GC cells, there are alterations in the glycolytic process. GC cells tend to preferentially generate energy through anaerobic glycolysis, converting glucose into lactate rather than completely metabolizing it through oxidative phosphorylation (14). Aberrations in glycolysis play a significant role in the development and progression of GC. The lactate produced by anaerobic glycolysis can alter intracellular pH, leading to acidification of the cellular environment, thereby promoting the invasive and metastatic capabilities of tumor cells (15,16). Furthermore, abnormal glycolysis is associated with the prognosis of GC. High levels of lactate accumulation are associated with the aggressiveness of tumors, lymph node metastasis, and other factors (17).
Warburg effect
GC is a common tumor in which the Warburg effect is closely associated with metabolism. The Warburg effect refers to the tendency of cancer cells to produce energy through glycolysis, rather than oxidative phosphorylation (which is the main energy production pathway in normal cells) under hypoxic conditions. This metabolic shift allows cancer cells to rapidly generate ATP and provide the energy and materials needed for synthesis (18).
The Warburg effect plays different roles in GC. Cancer cells express high levels of key enzymes in the glycolytic pathway, such as phosphofructokinase-1 (PFK-1). This leads to an increased rate of glucose conversion to lactate, producing more ATP to meet their high energy demands (19,20). Lactate produced by glycolysis cannot be fully cleared by GC cells, resulting in its accumulation in the tumor microenvironment, leading to acidification. This acidic environment not only facilitates the survival and growth of GC cells but also inhibits the function of immune cells (21). The Warburg effect reduces the dependence of cancer cells on oxidative phosphorylation (22). This could be due to mitochondrial dysfunction or regulation in response to the high energy demand state in GC cells. As a result, GC cells tend to rely on glycolysis to generate energy (23).
Aberrant lipid metabolism
In normal cellular physiology, there exists a finely tuned equilibrium between lipid synthesis, lipid droplet storage, and fatty acid (FA) oxidation. However, GC cells deviate from this norm, undergoing a metabolic reprogramming to fulfill the exigent demands of relentless proliferation. They augment their uptake of exogenous fats via CD36 receptors in order to replenish the raw materials necessary for sustained expansion (24). As GC cells adapt to their heightened metabolic demands, they increasingly rely on de novo synthesis pathways, particularly in the context of lipid metabolism. Pyruvate, the ultimate product of glycolysis, traverses the tricarboxylic acid cycle to generate citric acid as an intermediate metabolite. Subsequently, this citric acid undergoes cleavage facilitated by ATP citrate lyase (ACLY), yielding acetyl-CoA. This acetyl-CoA serves as a pivotal precursor for the synthesis of both cholesterol and FA, thus underscoring its indispensability in sustaining the aberrant lipid metabolism characteristic of GC cells (25). The activation of sterol regulatory element-binding protein-1 (SREBP-1) and the PI3K-AKT-mTOR signaling pathway orchestrates this distinctive conversion from sugar to lipid within malignant cells (26). It’s notable to mention that acetate can also undergo conversion into citric acid catalyzed by the enzyme ACSS2, proceeding subsequently to acetyl-CoA via the action of ACLY. However, this pathway for acetyl-CoA synthesis is relatively less prevalent compared to other routes (27,28). Acetyl-CoA serves as a synthetic raw material in two ways. One of them involves the irreversible conversion to malonyl-CoA by acetyl-CoA carboxylase (ACC).
Two different types of ACC play a crucial role in regulating the synthesis and oxidation capacity of FA. Malonyl-CoA is continuously generated and used to form palmitic acid through several cycles of acyl transfer, condensation, reduction, dehydration, and redox reactions by fatty acid synthase (FASN). Saturated FAs can undergo further elongation or desaturation by stearoyl-CoA desaturases (SCDs), resulting in the formation of monounsaturated fatty acids (MUFA)/polyunsaturated fatty acids (PUMA). These eventually contribute to the synthesis of cholesterol esters and triglycerides, which are stored in lipid droplets or utilized for cell membrane production.
On the other hand, acetyl-CoA participates in the mevalonate pathway (MVP) to produce cholesterol, a vital raw material for cell membranes (29). During the initial phase, acetyl-CoA undergoes conversion into acetoacetyl-CoA catalyzed by acetoacetyl-CoA thiolase. Subsequently, this compound is further metabolized into hydroxymethylglutaryl-CoA (HMG-CoA) by the pivotal enzyme HMG-CoA synthetase. In the subsequent stage, HMG-CoA is transformed into mevalonate through the action of HMG-CoA reductase. Finally, via a series of intricate chemical transformations involving phosphorylation, decarboxylation, and dehydroxylation, isoprene and 27 C cholesterol are synthesized (30). Ultimately, this process culminates in the generation of acetyl-CoA, liberating a substantial amount of energy and producing reduction equivalents like NADPH and FADH2. These vital molecules play pivotal roles in supporting the proliferation, metastasis, and mitigation of oxidative stress damage within GC cells (31) (Figure 1).
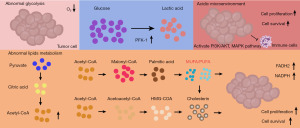
Abnormalities in amino acid metabolism
Recent research has emphasized the significance of amino acid consumption therapy in targeting tumor cell metabolism (32). This therapy focuses on amino acid metabolism pathways, including proline and aromatic amino acid metabolism, as well as branched-chain amino acid metabolism. Tumor cells commonly regulate these pathways through signaling pathways and transcription factors (33). Targeting tumor cell metabolism is a valuable approach in cancer treatment as it has a crucial role in cancer stem cell survival, tumor cell transformation, immune evasion, drug resistance, and disease recurrence. Amino acids play a critical role in the function of immune cells, such as T cells, which enhance amino acid absorption and improve immune function by upregulating the expression of amino acid transporters during proliferation, differentiation, and immune response (34). Cancer cells heavily rely on external sources for amino acids and upregulate the expression of amino acid transporters to meet their increasing demand. Manipulating the availability of amino acids presents a unique vulnerability specific to cancer cells (35).
Regarding drug resistance, amino acids play a crucial role in bolstering cancer cells against therapy through several mechanisms. They sustain biosynthetic processes, regulate redox homeostasis, modulate epigenetic modifications, and furnish metabolic intermediates essential for energy production (36). For instance, the administration of leucine or branched-chain amino acids amplifies cisplatin sensitivity in cancer cells. This is accomplished by inhibiting cisplatin- or bcat1-mediated autophagy while simultaneously stimulating mTOR signaling (37). By targeting tumor cell metabolism, particularly through amino acid consumption therapy, treatment responses can be improved, especially in drug-resistant cancers, while minimizing the toxicity associated with genotoxic drugs.
Dysregulated metabolism and GC cell proliferation
Changes in tumor energy metabolism
The energy metabolism of tumors undergoes significant changes. In normal cells, energy is mainly produced through oxidative phosphorylation and relies on the mitochondrial respiratory chain. However, in cancer cells, a phenomenon called the “Warburg effect” occurs (38), where even under sufficient oxygen conditions, cancer cells preferentially generate energy through lactate fermentation (39). This phenomenon, known as aerobic glycolysis, has been widely observed in various types of tumors. The main characteristic of aerobic glycolysis is the conversion of glucose into lactate instead of complete oxidation into carbon dioxide. This pathway produces less ATP but helps meet the energy demand of rapidly proliferating cancer cells (40).
Furthermore, tumor cells undergo alterations in the utilization of nutrients. They tend to rely more on glucose as the primary source of energy and increase the utilization of FA and amino acids. Glucose and FA enter tumor cells through different pathways and participate in energy metabolism and biosynthesis processes (41,42). These changes in energy metabolism in tumor cells are primarily regulated by various factors, including transcription factors, signaling pathways, and metabolic enzyme activity. These regulatory mechanisms interact to form a complex network to adapt to the rapid proliferation demands of tumor cells (43).
To manipulate metabolism in GC, several potential strategies can be considered. Firstly, targeting dysregulated metabolic pathways like glycolysis, the Warburg effect, lipid metabolism, and amino acid metabolism is crucial. This involves inhibiting key enzymes involved in these pathways, such as hexokinase, LDH, ACLY, FASN, and glutaminase. Additionally, exploiting metabolic vulnerabilities specific to cancer cells and employing combination therapies with conventional chemotherapeutic agents or immunotherapy are promising approaches. Personalized metabolic targeting based on individual tumor metabolic profiles can enhance treatment efficacy. Lastly, advancing clinical trials and drug development efforts focusing on the safety and efficacy of metabolic-targeting agents is essential for the development of effective therapeutic strategies for GC.
Accumulation and impact of metabolic products
The accumulation of metabolites has an impact on GC. In GC cells, due to changes and abnormalities in metabolic pathways, some metabolites may accumulate and have a negative impact on cell function and survival. For example, in the process of lactic acid fermentation, due to the large production of lactic acid, the intracellular concentration of lactic acid increases (44,45). This may lead to a decrease in intracellular pH value, thereby interfering with normal cellular metabolism and function (46). In addition, the accumulation of lactic acid may also lead to increased intracellular oxidative stress, leading to cell damage and inflammatory reactions. Another example is the accumulation of ketone bodies. In some cases, GC cells may experience excessive production and accumulation of ketone bodies. This may lead to ketotoxicity, further affecting the energy metabolism and normal function of cells. In addition, the accumulation of other metabolites in GC cells may also have negative effects on the cells. For example, some metabolites may have toxicity or mutagenicity, leading to cell DNA damage and gene mutations (47).
The accumulation of metabolites has a negative impact on the function and survival of GC cells. Further research on the mechanisms of metabolic abnormalities and accumulation of metabolites can help reveal the molecular mechanisms underlying the development of GC and provide new targets for the development of new treatment strategies.
The potential value of metabolic dysregulation in early diagnosis of GC
When it comes to early diagnosis of GC, metabolic dysregulation demonstrates potential value. The development of GC is closely associated with cellular metabolic processes, including glucose metabolism, lipid metabolism, and amino acid metabolism. These metabolic processes undergo alterations before tumor cells transform into malignant cells, leading to abnormal accumulation of metabolic byproducts within the cells. Recent research suggests that by analyzing metabolites in blood, urine, or breath samples and utilizing machine learning and pattern recognition techniques, metabolic profiling models can be established for the diagnosis of early-stage GC (48). These models are capable of accurately identifying metabolic features specific to GC and exhibit high sensitivity and specificity. For instance, changes in the concentrations of specific metabolites or metabolic markers can serve as biomarkers for early GC, aiding in accurate diagnosis (49).
Furthermore, metabolic dysregulation can also serve as an indicator for prognostic assessment. Studies have shown a correlation between metabolic abnormalities and the aggressiveness of GC, risk of recurrence, and response to treatment (50). Monitoring alterations in metabolic profiles can provide insights into patients’ treatment outcomes and prognosis, thereby supporting personalized treatment decision-making.
Interplay between dysregulated metabolism and epigenetics in GC
Dysregulated metabolism profoundly influences epigenetic processes in GC, contributing to tumorigenesis through various mechanisms. Altered metabolite levels, such as elevated acetyl-CoA, α-ketoglutarate, and S-adenosylmethionine (SAM), directly impact histone modifications and DNA methylation patterns. Metabolism-derived metabolites like acetyl-CoA play pivotal roles in histone acetylation, modulating chromatin accessibility and gene expression profiles. Changes in histone acetylation patterns, driven by dysregulated metabolism, activate oncogenes or silence tumor suppressor genes, promoting tumorigenesis. Additionally, dysregulated metabolism influences DNA methylation patterns by altering SAM levels, leading to aberrant DNA methylation patterns characterized by hypermethylation of tumor suppressor gene promoters.
Conversely, epigenetic alterations exert profound effects on dysregulated metabolism in GC. Epigenetic modifications control the expression of metabolic genes involved in glycolysis, lipid metabolism, and amino acid metabolism, thereby dysregulating metabolic pathways. Epigenetic regulation of microRNAs (miRNAs) further impacts metabolic pathways by modulating the expression of metabolic genes. Moreover, epigenetic modifications can directly regulate the activity of metabolic enzymes, influencing the metabolic phenotype of GC cells.
The interplay between dysregulated metabolism and epigenetics in GC results in synergistic effects driving tumorigenesis. Metabolic reprogramming can drive epigenetic changes that enhance the expression of oncogenes or silence tumor suppressor genes, promoting cell proliferation, survival, and metastasis. Conversely, epigenetic alterations sustain dysregulated metabolism by maintaining the expression of metabolic genes essential for tumor growth and adaptation to the tumor microenvironment. This positive feedback loop between metabolism and epigenetics amplifies tumorigenic states in GC, fostering tumor progression and therapeutic resistance.
The role of metabolic related gene abnormalities in GC
MYC
One of the most extensively studied coding genes in this region is MYC, also known as c-MYC. Numerous studies have shown that activation of MYC can contribute to the development of tumors (51,52). In GC, MYC amplification and increased expression are commonly observed in both GC cell lines and tissues. Patients with local or distant metastasis have been found to have the highest levels of MYC expression in their tumors (53). The significance of MYC in the progression of GC has been underscored by findings from a nonhuman primate model. In this model, MYC expression and copy number exhibit a continuous escalation across various stages of intestinal-type gastric carcinogenesis (54). Significantly, MYC not only holds a pivotal role in the etiology of GC but also stands out as one of the most dependable and substantial prognostic markers for GC (55).
Its functional domains include a nuclear localization sequence, a DNA binding domain, a helix-loop-helix (HLH) dimerization domain, and a transcriptional regulatory domain. Initially identified as the cellular counterpart of the v-myc oncogene found in oncogenic retroviruses (56), MYC was later found to be involved in chromosomal translocations in Burkitt lymphomas, where it becomes juxtaposed to immunoglobulin enhancers (57). In normal non-cancerous cells, MYC is tightly regulated. However, it is now recognized as one of the most frequently deregulated oncogenes. It is often translocated in hematopoietic cancers and is the third most amplified gene across various human cancers, as revealed by pan-cancer copy number analyses (58,59) (Figure 2).
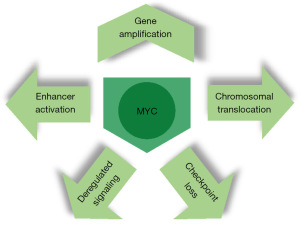
P53
TP53, a well-known tumor suppressor gene, plays a significant role in the development of GC (60). P53 mutations are commonly observed and rank among the top five most important mutations in GC (61). Extensive research on P53 function has revealed its involvement in regulating autophagy, apoptosis, the cell cycle, and DNA repair (62). However, when P53 is mutated, cells can proliferate abnormally and transform into cancer cells. Patients with P53 mutations tend to have a poorer prognosis compared to those without mutations in GC (63).
Furthermore, emerging evidence suggests that P53 is also involved in modulating tumor immune responses (64). P53 mutations in GC have been associated with suppressed immune activity (65). Recent studies propose a connection between P53’s tumor-suppressive properties and enhanced immune responses (66). P53 affects the innate immune system by regulating macrophage function. Additionally, increased expression of immune checkpoints induced by P53 has been reported in tumors (67,68) (Figure 3).
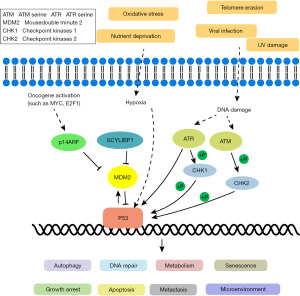
B-cell translocation gene 3 (BTG3)
BTG3 is a tumor suppressor gene belonging to the family of receptor tyrosine kinases, ErbB2. Its N-terminal region binds to E2F1 protein, inhibiting the binding of DP1 and E2F1 transcription factors to DNA and thereby blocking DNA synthesis, playing an important role in regulating cell differentiation, cell cycle, and inhibiting uncontrolled proliferation of cancer cells. BTG3 expression is significantly upregulated when cells experience DNA damage or stimulation by growth factors, reaching its peak during the G1/S transition, thereby inhibiting continuous cell proliferation. BTG3 can induce the interaction between the Smad8 and Smad4 proteins, leading to their entry into the nucleus and participating in the regulation of cell proliferation and apoptosis. Studies have reported that the C-terminal domain of the BTG3 protein, due to its proline-rich structure, can bind to Src protein, reducing the activity of Src tyrosine kinase, which in turn impedes the Ras/MAP kinase signaling pathway, ultimately inhibiting cell proliferation, participating in DNA damage repair, and regulating cell cycle (69). In GC research, most studies have shown significantly reduced expression of BTG3 at the protein level in GC tissues compared to adjacent normal tissues (Figure 4).
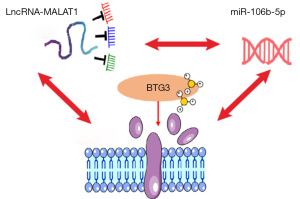
miR106
miR106, a small RNA molecule, has been identified as having abnormal expression in various tumors, including GC. Recent studies have revealed its role in regulating metabolic processes specifically in GC (70). Notably, the expression level of MIR106 is significantly increased in GC tissue. Functional studies have further demonstrated that MIR106 can impact GC cell metabolism in multiple ways (71).
MIR106 can suppress the process of glycolysis in GC cells. Glycolysis is a crucial metabolic pathway that provides energy and biosynthetic components required by GC cells. miR106 has been found to target and regulate the expression of key enzymes involved in glycolysis, such as PFK and pyruvate kinase (PKM), resulting in reduced glycolysis product production. MIR106 can also modulate lipid metabolism in GC cells. Abnormal lipid metabolism is a common occurrence in GC. Overexpression of miR106 inhibits the expression of key enzymes like FASN and 3-hydroxy-3-methylglutarate carbonyl synthase (HMGCR), consequently reducing lipid synthesis in GC cells. Additionally, MIR106 influences amino acid metabolism in GC cells. It has been observed that the expression level of MIR106 negatively correlates with the expression level of amino acid transporters. Increased expression of MIR106 impedes the entry and utilization of amino acids, thereby affecting the reliance of GC cells on this nutrient source (72,73).
The role of other metabolic related genes
In addition to MYC, TP53, many other metabolism-related genes play important roles in the occurrence and development of GC. These genes are involved in key processes such as cell proliferation, apoptosis, DNA repair, cell cycle regulation, and activation of signaling pathways. Mutations or abnormal expression of these genes can disrupt metabolic pathways, promote abnormal cell proliferation, enhance invasive capabilities, and contribute to the formation and progression of GC. In-depth research on the functions and mechanisms of these genes helps uncover the mechanisms underlying GC and provides new targets and strategies for its diagnosis and treatment (Table 1).
Table 1
Gene name | Function | Reference |
---|---|---|
PTEN | Inhibiting the PI3K/AKT signaling pathway, regulating cell proliferation and apoptosis | (74) |
APC | Regulating Wnt/β-catenin signaling pathway, involved in cell proliferation and stem cell self-renewal | (75,76) |
KRAS | Participate in cell proliferation and differentiation, mutations can promote tumor development | (77) |
CDH1 | The mutation of the gene encoding Tight junction protein E-cadherin can lead to the loss of cell adhesion function | (78) |
IDH1/2 | It encodes Isocarpic acid dehydrogenase, and mutation can change the metabolic state and proliferation ability of cells | (79) |
LDHA | Encoding lactate dehydrogenase A, mutation can increase lactate production and affect cell energy metabolism | (80) |
HK2 | Encoding phosphorylated glucokinase 2, mutation can increase the glucose uptake ability of GC cells | (81) |
GLUT1 | Encoding glucose transporter 1, mutation can increase the ability of GC cells to transport glucose | (82) |
EGFR | Regulating cell proliferation and survival signaling pathways | (83) |
PIK3CA | Key enzymes encoding the PI3K-AKT signaling pathway, mutations can promote cell growth and metastasis | (84) |
PTGS2 | Coding cyclooxygenase-2 and participates in inflammatory reaction and tumor development | (85) |
MUC1 | It encodes mucoprotein 1, and mutations can lead to excessive mucus production and tumor invasion | (86,87) |
MMPs | It encodes matrix metalloproteinase and participates in tumor cell invasion and invasion | (88) |
VEGF | It encodes vascular endothelial growth factor and participates in angiogenesis and metastasis of tumors | (89) |
HIF-1α | Encoding hypoxia inducible factor 1 α, participate in the adaptation of tumor cells to hypoxic environments | (90) |
SIRT1 | Encoding sirtuin 1, affecting cell energy metabolism and antioxidant stress capacity | (91) |
SLC7A5 | Encoding large amino acid transporter 5, involved in the uptake of amino acids by GC cells | (92) |
CYP1A1 | Its codes for cytochrome P450 family 1 subtype A1 and participates in the metabolism of exogenous compounds | (93) |
Dysregulated metabolism-associated genes can profoundly influence the tumor microenvironment in GC. For instance, overexpression of genes like MYC, TP53, and miR106 can modulate the metabolic landscape within the tumor, leading to alterations in nutrient availability, immune cell infiltration, and extracellular matrix remodeling. These changes shape the tumor microenvironment, influencing tumor progression, metastasis, and therapeutic responses. Moreover, dysregulated metabolism-associated genes serve as valuable prognostic markers in GC patients. Elevated expression of MYC has been correlated with poor prognosis and increased metastatic potential in GC. Similarly, mutations in TP53 are associated with worse survival outcomes and resistance to chemotherapy in GC patients. Additionally, dysregulated expression of miR106 has been linked to altered metabolic phenotypes and aggressive tumor behavior in GC. Furthermore, dysregulated metabolism-associated genes play a crucial role in predicting drug sensitivity in GC patients. For instance, tumors with aberrant MYC expression may exhibit increased sensitivity to drugs targeting glycolytic pathways or MYC signaling. Similarly, TP53 mutations can confer resistance to certain chemotherapeutic agents, influencing treatment outcomes in GC patients. Additionally, miR106-mediated dysregulation of metabolic pathways can affect drug metabolism and response in GC. GC, gastric cancer.
Roles of dysregulated metabolism associated genes in predicting immunotherapy response
Dysregulated metabolism-associated genes play pivotal roles in modulating the tumor immune microenvironment (TIME) and influencing immunotherapy response in GC. Metabolic alterations within tumor cells can create an immunosuppressive milieu, impacting the efficacy of immune checkpoint blockade (ICB) therapies. Conversely, metabolic reprogramming can also influence the expression of immune-related genes and pathways, thereby serving as potential biomarkers for predicting immunotherapy response. Metabolic pathways, including glycolysis, glutaminolysis, and FA metabolism, have been implicated in shaping the immunosuppressive phenotype of GC tumors. For instance, upregulation of glycolytic enzymes such as lactate dehydrogenase A (LDHA) and hexokinase 2 (HK2) promotes lactate accumulation and extracellular acidification, fostering an immunosuppressive microenvironment characterized by impaired T cell function and enhanced regulatory T cell (Treg) infiltration.
Moreover, dysregulated lipid metabolism, exemplified by increased FA synthesis and cholesterol metabolism, can contribute to immune evasion mechanisms in GC. Lipid metabolic enzymes, such as FASN and ACC, are associated with immunosuppressive phenotypes and resistance to immunotherapy. Conversely, alterations in amino acid metabolism, particularly glutamine metabolism, influence immune cell function and polarization, impacting immunotherapy response in GC.
Integration of metabolic gene signatures with immune-related biomarkers offers a holistic approach to predicting immunotherapy response and stratifying patients for personalized treatment strategies. Combining metabolic profiling with established immune biomarkers, such as programmed death-ligand 1 (PD-L1) expression and tumor mutational burden (TMB), enables more accurate patient selection for immunotherapy. Furthermore, emerging evidence suggests crosstalk between metabolic pathways and immune checkpoints, providing opportunities for therapeutic interventions targeting both dysregulated metabolism and immune evasion mechanisms. Combinatorial approaches, such as metabolic inhibitors in conjunction with ICB therapies, hold promise for overcoming resistance and enhancing immunotherapy efficacy in GC.
Metabolic disorders and potential applications for GC
Opportunities for combination therapy strategies have significant relevance in the treatment of GC. Firstly, there is cross-talk and interaction between metabolic pathways and other signaling pathways, providing an opportunity to simultaneously target multiple pathways. By employing combination therapy to concurrently intervene in these pathways, it is possible to inhibit tumor cell proliferation and survival more effectively (94,95).
Further research is expected for the intricate relationship between metabolic pathways and signaling networks. Understanding the cross-talk and interplay between these pathways will contribute to a comprehensive picture of cellular metabolism and its impact on disease pathogenesis (96). This knowledge will enable the development of personalized treatment strategies that leverage the interconnections between different pathways to optimize therapeutic outcomes and improve patient care. Ultimately, continued research in this field holds great potential for advancing our understanding of disease biology and fostering the development of innovative therapeutic approaches.
New biomarkers hold significant promise for improving clinical outcomes in GC through their applications in early detection, prognosis prediction, and personalized therapeutic interventions. These biomarkers offer non-invasive approaches for early diagnosis, allowing for timely interventions and enhanced patient survival rates. Additionally, they provide valuable prognostic information, guiding treatment decisions and patient management strategies based on tumor aggressiveness, metastatic potential, and treatment response.
Moreover, biomarker-driven targeted therapies exemplify the paradigm of personalized medicine in GC treatment. Identification of specific molecular alterations enables the selection of targeted therapeutic interventions, such as HER2-targeted therapy in HER2-positive GC, leading to improved treatment efficacy and minimized adverse effects. Furthermore, emerging immunotherapeutic biomarkers, including PD-L1 expression and tumor-infiltrating lymphocytes (TILs), offer insights into immunotherapy response prediction and patient stratification, thereby optimizing therapeutic outcomes.
Continued research efforts leveraging advanced omics technologies, bioinformatics, and machine learning algorithms are essential for the discovery and validation of novel biomarkers. Collaborative initiatives, such as large-scale multicenter studies and international consortia, facilitate biomarker translation into clinical practice, ultimately enhancing GC management strategies.
To achieve more precise and personalized immunotherapy responses in GC based on the findings of this study, several strategies can be considered. Firstly, leveraging the insights gained from dysregulated metabolism-associated genes and their impact on the tumor microenvironment, personalized immunotherapy approaches can be tailored to target specific metabolic vulnerabilities in individual patients. For instance, combining immunotherapy with metabolic inhibitors targeting dysregulated pathways such as glycolysis or lipid metabolism may enhance treatment efficacy by disrupting tumor-promoting metabolic processes and potentiating antitumor immune responses. Integrating multi-omics data, including genomic, transcriptomic, proteomic, and metabolomic profiles, can provide a comprehensive understanding of the molecular landscape of GC tumors. This holistic approach enables the identification of predictive biomarkers for immunotherapy response, such as tumor mutation burden, neoantigen load, immune cell infiltration patterns, and metabolic signatures. Utilizing machine learning algorithms and computational modeling to analyze these complex datasets can facilitate the development of predictive models for patient stratification and treatment selection, enabling more precise and personalized immunotherapy strategies.
Additionally, exploring novel immunotherapeutic targets based on dysregulated metabolism-associated genes and their interactions with the tumor microenvironment holds promise for improving treatment outcomes in GC patients. Targeting metabolic checkpoints or immune-metabolic axes may overcome immune evasion mechanisms employed by tumor cells and enhance the efficacy of immunotherapy. Moreover, investigating the crosstalk between dysregulated metabolism and immune signaling pathways in GC can uncover synergistic therapeutic combinations that exploit metabolic vulnerabilities while boosting antitumor immune responses.
As for the next plan, conducting prospective clinical trials to validate the utility of personalized immunotherapy strategies guided by dysregulated metabolism-associated genes and tumor microenvironment profiling is essential. These trials should focus on assessing treatment responses, survival outcomes, and biomarker-driven patient selection criteria to optimize therapeutic efficacy and minimize potential adverse effects. Additionally, ongoing research efforts should continue to elucidate the molecular mechanisms underlying dysregulated metabolism-immune interactions in GC, paving the way for the development of innovative immunotherapeutic interventions and precision medicine approaches.
Conclusions
Currently, targeted metabolic pathway therapy strategies involve the inhibition of specific metabolic pathways in cancer cells. One approach is to target glycolysis, which is responsible for glucose breakdown and energy production. By inhibiting key enzymes like hexokinase or PKM, the glycolytic process can be disrupted, leading to reduced cancer cell growth (97). Another strategy focuses on lipid metabolism, which involves the synthesis and breakdown of FA and cholesterol (98). Inhibiting enzymes such as FASN or HMG-CoA reductase can hinder lipid biosynthesis and impair cancer cell proliferation and survival.
Additionally, targeting amino acid metabolism pathways, including the synthesis and degradation of amino acids, has shown promise. Inhibiting enzymes like glutaminase or serine hydroxy methyltransferase can weaken cancer cell viability and proliferation (99). These targeted approaches aim to specifically disrupt crucial metabolic processes in cancer cells, potentially leading to improved treatment outcomes. Continued research and development in this field may offer new therapeutic options for cancer patients (100).
Acknowledgments
Funding: The study was supported by
Footnote
Peer Review File: Available at https://tcr.amegroups.com/article/view/10.21037/tcr-23-2244/prf
Conflicts of Interest: All authors have completed the ICMJE uniform disclosure form (available at https://tcr.amegroups.com/article/view/10.21037/tcr-23-2244/coif). The authors have no conflicts of interest to declare.
Ethical Statement: The authors are accountable for all aspects of the work in ensuring that questions related to the accuracy or integrity of any part of the work are appropriately investigated and resolved.
Open Access Statement: This is an Open Access article distributed in accordance with the Creative Commons Attribution-NonCommercial-NoDerivs 4.0 International License (CC BY-NC-ND 4.0), which permits the non-commercial replication and distribution of the article with the strict proviso that no changes or edits are made and the original work is properly cited (including links to both the formal publication through the relevant DOI and the license). See: https://creativecommons.org/licenses/by-nc-nd/4.0/.
References
- Cui MY, Yi X, Zhu DX, et al. Aberrant lipid metabolism reprogramming and immune microenvironment for gastric cancer: a literature review. Transl Cancer Res 2021;10:3829-42. [Crossref] [PubMed]
- Kawamura M, Uedo N, Koike T, et al. Kyoto classification risk scoring system and endoscopic grading of gastric intestinal metaplasia for gastric cancer: Multicenter observation study in Japan. Dig Endosc 2022;34:508-16. [Crossref] [PubMed]
- Sexton RE, Al Hallak MN, Diab M, et al. Gastric cancer: a comprehensive review of current and future treatment strategies. Cancer Metastasis Rev 2020;39:1179-203. [Crossref] [PubMed]
- Park JH, Pyun WY, Park HW. Cancer Metabolism: Phenotype, Signaling and Therapeutic Targets. Cells 2020;9:2308. [Crossref] [PubMed]
- Jurisic V, Radenkovic S, Konjevic G. The Actual Role of LDH as Tumor Marker, Biochemical and Clinical Aspects. Adv Exp Med Biol 2015;867:115-24. [Crossref] [PubMed]
- Yang GX, Li X, Snyder M. Investigating metabolite-protein interactions: an overview of available techniques. Methods 2012;57:459-66. [Crossref] [PubMed]
- Bhattacharyya A, Chattopadhyay R, Mitra S, et al. Oxidative stress: an essential factor in the pathogenesis of gastrointestinal mucosal diseases. Physiol Rev 2014;94:329-54. [Crossref] [PubMed]
- Garber K. Energy deregulation: licensing tumors to grow. Science 2006;312:1158-9. [Crossref] [PubMed]
- Battista S, Ambrosio MR, Limarzi F, et al. Molecular Alterations in Gastric Preneoplastic Lesions and Early Gastric Cancer. Int J Mol Sci 2021;22:6652. [Crossref] [PubMed]
- Meric-Bernstam F, Johnson AM, Dumbrava EEI, et al. Advances in HER2-Targeted Therapy: Novel Agents and Opportunities Beyond Breast and Gastric Cancer. Clin Cancer Res 2019;25:2033-41. [Crossref] [PubMed]
- Echizen K, Hirose O, Maeda Y, et al. Inflammation in gastric cancer: Interplay of the COX-2/prostaglandin E2 and Toll-like receptor/MyD88 pathways. Cancer Sci 2016;107:391-7. [Crossref] [PubMed]
- Xie J, Fu L, Jin L. Immunotherapy of gastric cancer: Past, future perspective and challenges. Pathol Res Pract 2021;218:153322. [Crossref] [PubMed]
- Yang H, Li Y, Hu B. Potential role of mitochondria in gastric cancer detection: Fission and glycolysis. Oncol Lett 2021;21:439. [Crossref] [PubMed]
- Romo-Perez A, Dominguez-Gomez G, Chavez-Blanco A, et al. Progress in Metabolic Studies of Gastric Cancer and Therapeutic Implications. Curr Cancer Drug Targets 2022;22:703-16. [Crossref] [PubMed]
- Yun M. Imaging of Gastric Cancer Metabolism Using 18 F-FDG PET/CT. J Gastric Cancer 2014;14:1-6. [Crossref] [PubMed]
- Minchenko OH, Tsuchihara K, Minchenko DO, et al. Mechanisms of regulation of PFKFB expression in pancreatic and gastric cancer cells. World J Gastroenterol 2014;20:13705-17. [Crossref] [PubMed]
- Zhao M, Wei F, Sun G, et al. Natural compounds targeting glycolysis as promising therapeutics for gastric cancer: A review. Front Pharmacol 2022;13:1004383. [Crossref] [PubMed]
- Liberti MV, Locasale JW. The Warburg Effect: How Does it Benefit Cancer Cells? Trends Biochem Sci 2016;41:211-8. [Crossref] [PubMed]
- Zhong X, He X, Wang Y, et al. Warburg effect in colorectal cancer: the emerging roles in tumor microenvironment and therapeutic implications. J Hematol Oncol 2022;15:160. [Crossref] [PubMed]
- Vaupel P, Schmidberger H, Mayer A. The Warburg effect: essential part of metabolic reprogramming and central contributor to cancer progression. Int J Radiat Biol 2019;95:912-9. [Crossref] [PubMed]
- Schwartz L, Supuran CT, Alfarouk KO. The Warburg Effect and the Hallmarks of Cancer. Anticancer Agents Med Chem 2017;17:164-70. [Crossref] [PubMed]
- Koppenol WH, Bounds PL, Dang CV. Otto Warburg's contributions to current concepts of cancer metabolism. Nat Rev Cancer 2011;11:325-37. Erratum in: Nat Rev Cancer 2011;11:618. [Crossref] [PubMed]
- Lebelo MT, Joubert AM, Visagie MH. Warburg effect and its role in tumourigenesis. Arch Pharm Res 2019;42:833-47. [Crossref] [PubMed]
- Wang J, Wen T, Li Z, et al. CD36 upregulates DEK transcription and promotes cell migration and invasion via GSK-3β/β-catenin-mediated epithelial-to-mesenchymal transition in gastric cancer. Aging (Albany NY) 2020;13:1883-97. [Crossref] [PubMed]
- Luengo A, Gui DY, Vander Heiden MG. Targeting Metabolism for Cancer Therapy. Cell Chem Biol 2017;24:1161-80. [Crossref] [PubMed]
- Cheng C, Geng F, Cheng X, et al. Lipid metabolism reprogramming and its potential targets in cancer. Cancer Commun (Lond) 2018;38:27. [Crossref] [PubMed]
- Yoshii Y, Furukawa T, Yoshii H, et al. Cytosolic acetyl-CoA synthetase affected tumor cell survival under hypoxia: the possible function in tumor acetyl-CoA/acetate metabolism. Cancer Sci 2009;100:821-7. [Crossref] [PubMed]
- Yoshii Y, Waki A, Furukawa T, et al. Tumor uptake of radiolabeled acetate reflects the expression of cytosolic acetyl-CoA synthetase: implications for the mechanism of acetate PET. Nucl Med Biol 2009;36:771-7. [Crossref] [PubMed]
- Butler LM, Perone Y, Dehairs J, et al. Lipids and cancer: Emerging roles in pathogenesis, diagnosis and therapeutic intervention. Adv Drug Deliv Rev 2020;159:245-93. [Crossref] [PubMed]
- Huang B, Song BL, Xu C. Cholesterol metabolism in cancer: mechanisms and therapeutic opportunities. Nat Metab 2020;2:132-41. [Crossref] [PubMed]
- Qu Q, Zeng F, Liu X, et al. Fatty acid oxidation and carnitine palmitoyltransferase I: emerging therapeutic targets in cancer. Cell Death Dis 2016;7:e2226. [Crossref] [PubMed]
- Tabe Y, Lorenzi PL, Konopleva M. Amino acid metabolism in hematologic malignancies and the era of targeted therapy. Blood 2019;134:1014-23. [Crossref] [PubMed]
- Jones CL, Stevens BM, D'Alessandro A, et al. Inhibition of Amino Acid Metabolism Selectively Targets Human Leukemia Stem Cells. Cancer Cell 2018;34:724-740.e4. [Crossref] [PubMed]
- Bian Y, Li W, Kremer DM, et al. Cancer SLC43A2 alters T cell methionine metabolism and histone methylation. Nature 2020;585:277-82. [Crossref] [PubMed]
- Butler M, van der Meer LT, van Leeuwen FN. Amino Acid Depletion Therapies: Starving Cancer Cells to Death. Trends Endocrinol Metab 2021;32:367-81. [Crossref] [PubMed]
- Yoo HC, Han JM. Amino Acid Metabolism in Cancer Drug Resistance. Cells 2022;11:140. [Crossref] [PubMed]
- Zheng YH, Hu WJ, Chen BC, et al. BCAT1, a key prognostic predictor of hepatocellular carcinoma, promotes cell proliferation and induces chemoresistance to cisplatin. Liver Int 2016;36:1836-47. [Crossref] [PubMed]
- Liu Y, Gu R, Gao M, et al. Emerging role of substance and energy metabolism associated with neuroendocrine regulation in tumor cells. Front Endocrinol (Lausanne) 2023;14:1126271. [Crossref] [PubMed]
- Floridi A, Paggi MG, D'Atri S, et al. Effect of lonidamine on the energy metabolism of Ehrlich ascites tumor cells. Cancer Res 1981;41:4661-6. [PubMed]
- Moreno-Sánchez R, Rodríguez-Enríquez S, Marín-Hernández A, et al. Energy metabolism in tumor cells. FEBS J 2007;274:1393-418. [Crossref] [PubMed]
- Li C, Zhang L, Qiu Z, et al. Key Molecules of Fatty Acid Metabolism in Gastric Cancer. Biomolecules 2022;12:706. [Crossref] [PubMed]
- Currie E, Schulze A, Zechner R, et al. Cellular fatty acid metabolism and cancer. Cell Metab 2013;18:153-61. [Crossref] [PubMed]
- Pavlova NN, Thompson CB. The Emerging Hallmarks of Cancer Metabolism. Cell Metab 2016;23:27-47. [Crossref] [PubMed]
- Muthyalaiah YS, Jonnalagadda B, John CM, et al. Impact of Advanced Glycation End products (AGEs) and its receptor (RAGE) on cancer metabolic signaling pathways and its progression. Glycoconj J 2021;38:717-34. [Crossref] [PubMed]
- Aguilar-Ballester M, Herrero-Cervera A, Vinué Á, et al. Impact of Cholesterol Metabolism in Immune Cell Function and Atherosclerosis. Nutrients 2020;12:2021. [Crossref] [PubMed]
- Metformin He L, Metabolism Systemic. Trends Pharmacol Sci 2020;41:868-81. [Crossref] [PubMed]
- Chaurasia B, Summers SA. Ceramides in Metabolism: Key Lipotoxic Players. Annu Rev Physiol 2021;83:303-30. [Crossref] [PubMed]
- Dinges SS, Hohm A, Vandergrift LA, et al. Cancer metabolomic markers in urine: evidence, techniques and recommendations. Nat Rev Urol 2019;16:339-62. [Crossref] [PubMed]
- O'Connell TM. Recent advances in metabolomics in oncology. Bioanalysis 2012;4:431-51. [Crossref] [PubMed]
- Yang Q, Zhang RW, Sui PC, et al. Dysregulation of non-coding RNAs in gastric cancer. World J Gastroenterol 2015;21:10956-81. [Crossref] [PubMed]
- Venkateswaran N, Conacci-Sorrell M. MYC leads the way. Small GTPases 2020;11:86-94. [Crossref] [PubMed]
- Stine ZE, Walton ZE, Altman BJ, et al. MYC, Metabolism, and Cancer. Cancer Discov 2015;5:1024-39. [Crossref] [PubMed]
- Burbano RR, Assumpção PP, Leal MF, et al. C-MYC locus amplification as metastasis predictor in intestinal-type gastric adenocarcinomas: CGH study in Brazil. Anticancer Res 2006;26:2909-14. [PubMed]
- da Costa Jde F, Leal MF, Silva TC, et al. Experimental gastric carcinogenesis in Cebus apella nonhuman primates. PLoS One 2011;6:e21988. [Crossref] [PubMed]
- Liu Y, Shi Y, Han R, et al. Signaling pathways of oxidative stress response: the potential therapeutic targets in gastric cancer. Front Immunol 2023;14:1139589. [Crossref] [PubMed]
- Duesberg PH, Bister K, Vogt PK. The RNA of avian acute leukemia virus MC29. Proc Natl Acad Sci U S A 1977;74:4320-4. [Crossref] [PubMed]
- Dalla-Favera R, Bregni M, Erikson J, et al. Human c-myc onc gene is located on the region of chromosome 8 that is translocated in Burkitt lymphoma cells. Proc Natl Acad Sci U S A 1982;79:7824-7. [Crossref] [PubMed]
- Zack TI, Schumacher SE, Carter SL, et al. Pan-cancer patterns of somatic copy number alteration. Nat Genet 2013;45:1134-40. [Crossref] [PubMed]
- Li A, Chapuy B, Varelas X, et al. Identification of candidate cancer drivers by integrative Epi-DNA and Gene Expression (iEDGE) data analysis. Sci Rep 2019;9:16904. [Crossref] [PubMed]
- Zhang L, Li W, Cao L, et al. PKNOX2 suppresses gastric cancer through the transcriptional activation of IGFBP5 and p53. Oncogene 2019;38:4590-604. [Crossref] [PubMed]
- Cheng LL, Itahana Y, Lei ZD, et al. TP53 genomic status regulates sensitivity of gastric cancer cells to the histone methylation inhibitor 3-deazaneplanocin A (DZNep). Clin Cancer Res 2012;18:4201-12. [Crossref] [PubMed]
- Leader AM, Grout JA, Maier BB, et al. Single-cell analysis of human non-small cell lung cancer lesions refines tumor classification and patient stratification. Cancer Cell 2021;39:1594-1609.e12. [Crossref] [PubMed]
- Chiaravalli AM, Klersy C, Vanoli A, et al. Histotype-based prognostic classification of gastric cancer. World J Gastroenterol 2012;18:896-904. [Crossref] [PubMed]
- Menendez D, Inga A, Resnick MA. The expanding universe of p53 targets. Nat Rev Cancer 2009;9:724-37. [Crossref] [PubMed]
- Lyu H, Li M, Jiang Z, et al. Correlate the TP53 Mutation and the HRAS Mutation with Immune Signatures in Head and Neck Squamous Cell Cancer. Comput Struct Biotechnol J 2019;17:1020-30. [Crossref] [PubMed]
- Muñoz-Fontela C, Mandinova A, Aaronson SA, et al. Emerging roles of p53 and other tumour-suppressor genes in immune regulation. Nat Rev Immunol 2016;16:741-50. [Crossref] [PubMed]
- Chen Y, Sun Z, Chen W, et al. The Immune Subtypes and Landscape of Gastric Cancer and to Predict Based on the Whole-Slide Images Using Deep Learning. Front Immunol 2021;12:685992. [Crossref] [PubMed]
- Bordon Y. Cell death: Tumour suppressor p53 helps phagocytes clean up. Nat Rev Immunol 2015;15:525. [Crossref] [PubMed]
- Cheng YC, Chiang HY, Cheng SJ, et al. Loss of the tumor suppressor BTG3 drives a pro-angiogenic tumor microenvironment through HIF-1 activation. Cell Death Dis 2020;11:1046. [Crossref] [PubMed]
- Lo YH, Kolahi KS, Du Y, et al. A CRISPR/Cas9-Engineered ARID1A-Deficient Human Gastric Cancer Organoid Model Reveals Essential and Nonessential Modes of Oncogenic Transformation. Cancer Discov 2021;11:1562-81. [Crossref] [PubMed]
- Peng Q, Shen Y, Lin K, et al. Comprehensive and integrative analysis identifies microRNA-106 as a novel non-invasive biomarker for detection of gastric cancer. J Transl Med 2018;16:127. [Crossref] [PubMed]
- Wan X, Ding X, Chen S, et al. The functional sites of miRNAs and lncRNAs in gastric carcinogenesis. Tumour Biol 2015;36:521-32. [Crossref] [PubMed]
- Jiang L, Li X, Cheng Q, et al. Plasma microRNA might as a potential biomarker for hepatocellular carcinoma and chronic liver disease screening. Tumour Biol 2015;36:7167-74. [Crossref] [PubMed]
- Hu M, Zhu S, Xiong S, et al. MicroRNAs and the PTEN/PI3K/Akt pathway in gastric cancer Oncol Rep 2019;41:1439-54. (Review). [Crossref] [PubMed]
- Deng S, Zhang X, Qin Y, et al. miRNA-192 and -215 activate Wnt/β-catenin signaling pathway in gastric cancer via APC. J Cell Physiol 2020;235:6218-29. [Crossref] [PubMed]
- Yang XZ, Cheng TT, He QJ, et al. LINC01133 as ceRNA inhibits gastric cancer progression by sponging miR-106a-3p to regulate APC expression and the Wnt/β-catenin pathway. Mol Cancer 2018;17:126. [Crossref] [PubMed]
- Strickler JH, Yoshino T, Graham RP, et al. Diagnosis and Treatment of ERBB2-Positive Metastatic Colorectal Cancer: A Review. JAMA Oncol 2022;8:760-9. [Crossref] [PubMed]
- Shenoy S. CDH1 (E-Cadherin) Mutation and Gastric Cancer: Genetics, Molecular Mechanisms and Guidelines for Management. Cancer Manag Res 2019;11:10477-86. [Crossref] [PubMed]
- Xu C, Ooi WF, Qamra A, et al. HNF4α pathway mapping identifies wild-type IDH1 as a targetable metabolic node in gastric cancer. Gut 2020;69:231-42. [Crossref] [PubMed]
- Wang XH, Jiang ZH, Yang HM, et al. Hypoxia-induced FOXO4/LDHA axis modulates gastric cancer cell glycolysis and progression. Clin Transl Med 2021;11:e279. [Crossref] [PubMed]
- Wang J, Huang Q, Hu X, et al. Disrupting Circadian Rhythm via the PER1-HK2 Axis Reverses Trastuzumab Resistance in Gastric Cancer. Cancer Res 2022;82:1503-17. [Crossref] [PubMed]
- Zeng Z, Nian Q, Chen N, et al. Ginsenoside Rg3 inhibits angiogenesis in gastric precancerous lesions through downregulation of Glut1 and Glut4. Biomed Pharmacother 2022;145:112086. [Crossref] [PubMed]
- Lei ZN, Teng QX, Tian Q, et al. Signaling pathways and therapeutic interventions in gastric cancer. Signal Transduct Target Ther 2022;7:358. [Crossref] [PubMed]
- Sukawa Y, Yamamoto H, Nosho K, et al. HER2 expression and PI3K-Akt pathway alterations in gastric cancer. Digestion 2014;89:12-7. [Crossref] [PubMed]
- Xiang L, Wang W, Zhou Z, et al. COX-2 promotes metastasis and predicts prognosis in gastric cancer via regulating mTOR. Biomark Med 2020;14:421-32. [Crossref] [PubMed]
- Oue N, Sentani K, Sakamoto N, et al. Clinicopathologic and molecular characteristics of gastric cancer showing gastric and intestinal mucin phenotype. Cancer Sci 2015;106:951-8. [Crossref] [PubMed]
- Kim YI, Pecha RL, Keihanian T, et al. MUC1 Expressions and Its Prognostic Values in US Gastric Cancer Patients. Cancers (Basel) 2023;15:998. [Crossref] [PubMed]
- Sokolova O, Naumann M. Matrix Metalloproteinases in Helicobacter pylori-Associated Gastritis and Gastric Cancer. Int J Mol Sci 2022;23:1883. [Crossref] [PubMed]
- Xie M, Yu T, Jing X, et al. Exosomal circSHKBP1 promotes gastric cancer progression via regulating the miR-582-3p/HUR/VEGF axis and suppressing HSP90 degradation. Mol Cancer 2020;19:112. [Crossref] [PubMed]
- Lin Z, Song J, Gao Y, et al. Hypoxia-induced HIF-1α/lncRNA-PMAN inhibits ferroptosis by promoting the cytoplasmic translocation of ELAVL1 in peritoneal dissemination from gastric cancer. Redox Biol 2022;52:102312. Erratum in: Redox Biol 2022;55:102402. [Crossref] [PubMed]
- An Y, Wang B, Wang X, et al. SIRT1 inhibits chemoresistance and cancer stemness of gastric cancer by initiating an AMPK/FOXO3 positive feedback loop. Cell Death Dis 2020;11:115. [Crossref] [PubMed]
- Ma Q, Yang F, Huang B, et al. CircARID1A binds to IGF2BP3 in gastric cancer and promotes cancer proliferation by forming a circARID1A-IGF2BP3-SLC7A5 RNA-protein ternary complex. J Exp Clin Cancer Res 2022;41:251. [Crossref] [PubMed]
- Hidaka A, Sasazuki S, Matsuo K, et al. CYP1A1, GSTM1 and GSTT1 genetic polymorphisms and gastric cancer risk among Japanese: A nested case-control study within a large-scale population-based prospective study. Int J Cancer 2016;139:759-68. [Crossref] [PubMed]
- Zhao X, Wang M, Wen Z, et al. GLP-1 Receptor Agonists: Beyond Their Pancreatic Effects. Front Endocrinol (Lausanne) 2021;12:721135. [Crossref] [PubMed]
- Dorraki N, Ghale-Noie ZN, Ahmadi NS, et al. miRNA-148b and its role in various cancers. Epigenomics 2021;13:1939-60. [Crossref] [PubMed]
- Mehmel M, Jovanović N, Spitz U. Nicotinamide Riboside-The Current State of Research and Therapeutic Uses. Nutrients 2020;12:1616. [Crossref] [PubMed]
- Kalluri R, LeBleu VS. The biology, function, and biomedical applications of exosomes. Science 2020;367:eaau6977. [Crossref] [PubMed]
- Kullmann S, Heni M, Hallschmid M, et al. Brain Insulin Resistance at the Crossroads of Metabolic and Cognitive Disorders in Humans. Physiol Rev 2016;96:1169-209. [Crossref] [PubMed]
- Macchione IG, Lopetuso LR, Ianiro G, et al. Akkermansia muciniphila: key player in metabolic and gastrointestinal disorders. Eur Rev Med Pharmacol Sci 2019;23:8075-83. [PubMed]
- Cheon H, Borden EC, Stark GR. Interferons and their stimulated genes in the tumor microenvironment. Semin Oncol 2014;41:156-73. [Crossref] [PubMed]