Identification of a cancer driver gene-associated lncRNA signature for prognostic prediction and immune response evaluation in clear cell renal cell carcinoma
Highlight box
Key findings
• We present a novel 5-gene signature comprising HOXA11-AS, AP002807.1, APCDD1L-DT, AC124067.2, and SNHG3, accurately predicting the survival and prognosis of clear cell renal cell carcinoma (ccRCC) patients.
What is known and what is new?
• Prognostic assessment is crucial in managing ccRCC patients, yet reliable markers are lacking.
• Our study introduces a concise cancer driver gene-related long non-coding RNA (CDG-RlncRNA) signature, emphasizing SNHG3’s pivotal role in ccRCC progression. This signature demonstrates robust predictive accuracy, stratifying patients and revealing immune-related gene expression patterns. SNHG3 emerges as a key ccRCC cell cycle regulator, suggesting therapeutic potential.
What is the implication, and what should change now?
• Our work supplements ccRCC prognostic marker identification. Large-scale trials are needed to validate our signature’s reliability and applicability in clinical settings.
Introduction
Clear cell renal cell carcinoma (ccRCC) represents a prevalent subtype of malignancy in adults, constituting approximately 3% of cases (1,2). A significant proportion of patients, around 30%, are diagnosed at an advanced or metastatic stage, precluding the opportunity for surgical intervention (3-5). Despite the emergence of targeted therapy and immunotherapy as principal adjuncts for advanced ccRCC, the rates of complete and partial remission remain relatively modest (6-8). In light of advancements in bioinformatics and sequencing technologies, researchers have commenced leveraging existing data to construct prognostic markers for tumors, with the aim of predicting patient outcomes and disease progression. This approach has emerged as a promising strategy to enhance the clinical management of oncology patients.
Within the domain of ccRCC, investigators have undertaken bioinformatics analyses to identify various potential prognostic biomarkers. Notably, Zhang et al. have delineated chromatin regulation-related gene features that exhibit promising predictive efficacy for ccRCC treatment and prognosis (9). Similarly, Shao et al. have identified ALDOB as a putative prognostic biomarker for ccRCC patients through comprehensive data integration analyses (10). Furthermore, Chen et al. have proposed SPOCK1 as another potential prognostic biomarker for ccRCC based on comprehensive bioinformatics analyses (11). Despite these advancements, it is imperative to note that these biomarkers alone may not suffice for the comprehensive prediction of patient prognosis and treatment outcomes, underscoring the pressing need for the exploration of novel biomarkers to complement existing research.
It is well-known that cancer driver genes (CDGs) confer growth advantages to cancer cells, promoting tumorigenesis and progression across various malignancies (12-14), including hepatocellular carcinoma (HCC) (15,16), lung adenocarcinoma (17,18), bladder cancer (19,20), prostate cancer (20,21), and numerous other malignancies. Previously, a summary containing 568 CDGs was reported in the journal “Nature Reviews Cancer”, identified from over 28,000 tumors across 66 cancer types (22). Tumor development is intricately linked with alterations in the tumor microenvironment, with CDGs playing pivotal regulatory roles within cancer cells, thereby shaping the microenvironment and modulating responses to immunotherapy. Within the realm of ccRCC, CDG-RlncRNA assumes a crucial role as a CDG-driven tumor. Thus, elucidating the functional role of CDG-RlncRNA in ccRCC offers a promising avenue for the discovery and supplementation of prognostic biomarkers.
Given the constrained availability of ccRCC patient samples at our research center, we opted to conduct our study utilizing publicly accessible databases, such as The Cancer Genome Atlas (TCGA) and the International Cancer Genome Consortium (ICGC). In this study, we systematically analyzed the expression profiles of CDG-RlncRNA and the immune microenvironment landscape. Subsequently, we developed a prognostic risk model, termed CDG-RlncRNA model (CDG-RlncM), based on five CDG-related long non-coding RNAs (CDG-RlncRNAs), to predict the survival outcomes of ccRCC patients. We validated the predictive performance of CDG-RlncM using the TCGA database (training set) and the ICGC database (validation set), thereby corroborating its status as a reliable independent prognostic factor. Notably, within the CDG-RlncM, we identified SNHG3 as a gene of interest, exhibiting elevated expression in ccRCC and closely associated with adverse patient prognosis. Moreover, knockdown experiments demonstrated the ability of SNHG3 to induce G2/M phase cell cycle arrest. In summary, the construction of the CDG-RlncM in this study offers potential avenues for personalized treatment strategies for ccRCC patients, effectively complementing existing prognostic markers for the disease. Furthermore, this study underscores the significance of CDG-RlncRNA in ccRCC by elucidating its expression levels and role in cell cycle regulation. We present this article in accordance with the TRIPOD reporting checklist (available at https://tcr.amegroups.com/article/view/10.21037/tcr-24-127/rc).
Methods
Research process and preliminary data processing
Following the flowchart (Figure 1A), the research process commenced by retrieving expression data for 568 CDG-related genes from the TCGA database. Subsequently, Pearson correlation analysis (Pearson correlation coefficients >0.7 and P<0.001) identified 1,808 CDG-RlncRNAs. Additionally, Cox regression analysis revealed 97 CDG-RlncRNAs significantly associated with the prognosis of clear ccRCC (P<0.001). These genes underwent Least Absolute Shrinkage and Selection Operator (LASSO) regression analysis, resulting in the construction of a prognostic model comprising 5 CDG-RlncRNAs. Finally, this model underwent evaluation in both the TCGA database (as the training set) and the ICGC database (as the validation set), demonstrating robust predictive performance.
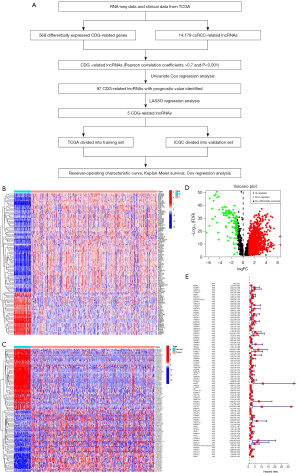
Research data acquisition
On October 10, 2022, the RNA-seq data and clinical information of ccRCC patients were meticulously obtained from two distinct sources: TCGA database, serving as the training dataset (https://portal.gdc.cancer.gov), and the ICGC database, utilized as the validation dataset (https://dcc.icgc.org). Specimens with incomplete clinical information in the databases were excluded. The selection of these datasets was guided by a rigorous consideration of their appropriateness and relevance for our study. The compilation of a comprehensive catalog comprising 568 CDGs was sourced from Nature Reviews Cancer, a highly esteemed academic publication (22). These genes were methodically identified through an extensive research endeavor encompassing a vast spectrum of over 28,000 tumors spanning 66 diverse cancer types. Detailed and specific information regarding the CDGs can be found in table available at https://cdn.amegroups.cn/static/public/TCR-24-127-1.xlsx, providing valuable insights into their characterization and significance in the context of our research.
Identification of CDG-RlncRNA and clustering of ccRCC patients
Using Spearman correlation analysis, we assessed the correlation between CDGs and their related lncRNAs. CDG-RlncRNAs were identified based on a rigorous criterion, specifically requiring an absolute correlation coefficient exceeding 0.7 and a P value below 0.001. This meticulous approach aimed to pinpoint CDG-RlncRNAs that exhibit a robust and significant relationship with CDGs. To delve into the prognostic value of CDG-RlncRNAs, we conducted univariate Cox regression analysis concerning patient overall survival (OS). Within this analysis, we selected 97 CDG-RlncRNAs and calculated hazard ratios (HR values) and Cox P values, unveiling the potential impact of these CDG-RlncRNAs on patient prognosis. Subsequently, building upon this cohort of 97 CDG-RlncRNAs, we employed non-negative matrix factorization (NMF) analysis to classify ccRCC patients. This classification facilitated the categorization of patients into distinct clusters. A comprehensive analysis was then undertaken to explore the disparities and unique characteristics among these clusters. This multifaceted approach provides valuable insights into the potential subtypes within the ccRCC patient population, shedding light on the underlying heterogeneity of this cancer type.
Assessment of immune infiltration and prediction of response to immune checkpoint inhibitors (ICIs) therapy
In this study, we employed the CIBERSORT algorithm to estimate the infiltration levels of distinct immune cell types. Gene expression data were submitted to the CIBERSORT website (http://cibersort.stanford.edu), and results with a significance level (P<0.05) were utilized to evaluate the immune and stromal components of the tumor microenvironment. This analysis enables a comprehensive assessment of the distribution and activity of various immune cell populations within the tumor microenvironment. The Tumor Immune Dysfunction and Exclusion (TIDE) score is an evaluative strategy utilized to predict the potential therapeutic response of cancer patients to ICIs. In this study, TIDE scores were calculated for patients categorized into high- and low-risk groups to assess their sensitivity to ICI treatments. This assessment aids in identifying the therapeutic response potential of patients and provides crucial insights for personalized treatment decisions.
Assessment and validation of prognostic model accuracy and construction of nomograms
We performed LASSO Cox analysis on 97 CDG-RlncRNAs associated with prognosis, obtained from the TCGA dataset, to establish the optimal CDG-RlncRNA model (CDG-RlncM). The model was constructed using the formula: Risk score = (lncRNA1 coefficient × lncRNA1 expression) + (lncRNA2 coefficient × lncRNA2 expression) + ... + (lncRNAn coefficient × lncRNAn expression). Based on the risk scores generated by the model, patients were stratified into high- and low-risk groups. Kaplan-Meier survival analysis was subsequently employed to determine survival disparities between these groups.
Additionally, we evaluated the predictive performance of the model using time receiver operating characteristic (ROC) and calibration curves. The area under the curve (AUC) represents the area under the ROC curve, ranging from 0 to 1. A higher AUC indicates better discrimination between positive and negative samples. An AUC close to 1 suggests superior model performance, while an AUC of 0.5 indicates performance equivalent to random guessing. Furthermore, calibration curves closer to the 45-degree diagonal line indicate greater consistency between predicted and observed risks. External validation was conducted using the ICGC database to confirm the reproducibility and robustness of the model. Moreover, we created column plots based on patient clinical characteristics and risk scores to comprehensively understand the associations between specific patient features (age, gender, stage, grade) and risk scores. This multifaceted analysis aids in gaining deeper insights into the clinical utility and patient stratification potential of the CDG-RlncRNA model.
Functional enrichment analysis
The “LIMMA” R package was utilized to analyze potential pathways and processes associated with differentially expressed genes (DEGs) in both high -and low-risk groups. Gene Ontology (GO) and Kyoto Encyclopedia of Genes and Genomes (KEGG) enrichment analyses of DEGs were conducted using the “clusterProfiler” package in R. The enrichment analyses encompassed all GO categories, specifically biological process (BP), molecular function (MF), and cellular component (CC).
Patient and tissue samples
A total of 18 pairs of ccRCC tissue specimens were obtained from the People’s Hospital of Chongqing Hechuan, China. This research was conducted in accordance with the principles outlined in the Helsinki Declaration (as revised in 2013) and received explicit approval and consent from the Ethics Committee at the People’s Hospital of Chongqing Hechuan (No. Hcry-Yn-01). Written informed consent was obtained from all participating patients, affirming their voluntary participation in the study.
Cell culture and treatment
The ccRCC cell lines, including 786-O, RCC-JF, and Caki-1, were cultured in RPMI 1640 medium (Corning Inc., Corning, NW, USA). Caki-1 cells were cultured using McCoy’s 5A medium (Biological Industries, Israel). All cells were cultured and transfected according to the manufacturer’s instructions. The siRNAs for SNHG3 and the corresponding control were purchased from Tsingke Biotechnology Co., Ltd., as follows:
- Negative control: F: UUCUCCGAACGUGUCACGUTT; R: ACGUGACACGUUCGGAGAATT;
- siSNHG3-1: F: GCAUUUAGCUAGGAAUGCATT; R: UGCAUUCCUAGCUAAAUGCTT;
- siSNHG3-2: F: GGGAUCAUCUAGAAGGUAATT; R: UUACCUUCUAGAUGAUCCCTT.
Real-time quantitative polymerase chain reaction (RT-qPCR)
Total RNA was meticulously isolated employing a state-of-the-art RNA extraction kit (FOREGENE, Chengdu, China), following rigorous protocols. Subsequently, cDNA synthesis was conducted using a high-quality reverse transcription kit (Takara Biotechnology Co., Ltd., Beijing, China). Quantitative analysis of SNHG3 expression was carried out through RT-qPCR, with GAPDH serving as the internal reference gene, ensuring data accuracy and reliability. The primer sequences utilized for SNHG3 in this study were:
Forward: 5'-TTCAAGCGATTCTCGTGCC-3';
Reverse: 5'-AAGATTGTCAAACCCTCCCTGT-3'.
The GAPDH primers applied in this research were as follows:
Forward: 5'-GGCTGTAGACCAGGATGAAG-3';
Reverse: 5'-TTGGAGGGGATCTCGCTCCT-3'.
Cell cycle detection
Transfected cells, cultured for 48 hours, were harvested by trypsinization, fixed in 75% ethanol at 4 ℃ overnight, and subsequently stained with propidium iodide (PI) at room temperature for 30 minutes. Subsequent cell analysis was performed using flow cytometry, following the manufacturer’s instructions.
Statistical analysis
The study presented continuous variables as mean values. Statistical analyses were conducted using R software and GraphPad Prism 8. Both Student’s t-tests and analysis of variance (ANOVA) were employed for statistical comparisons, as per the relevance of the experimental design. A significance level of *P<0.05 was chosen to denote statistical significance.
Results
Identification of CDG-RlncRNAs in ccRCC
Our study was conducted following the outlined procedures (Figure 1A). Initially, we performed a differential expression analysis of CDG-related genes using TCGA samples, which included 539 ccRCC cases and 72 normal kidney samples. Our selection criteria included a significance level of P<0.05 and an absolute log-fold change (|logFC|) greater than 1. This rigorous analysis identified 109 CDG-related genes displaying significant expression differences, as illustrated in Figure 1B. Subsequently, we conducted Spearman correlation analysis to identify long non-coding RNAs (lncRNAs) significantly associated with CDG-related genes, termed CDG-RlncRNA. Applying the same criteria (P<0.05 and |logFC| >1) led to the identification of 1,808 CDG-RlncRNAs. Further analysis revealed that among these 1,808 CDG-RlncRNAs, 1,221 exhibited pronounced differential expression between ccRCC and normal kidney samples, as depicted in Figure 1C and Figure 1D. We then proceeded to perform univariate Cox regression analysis to investigate the clinical significance of CDG-RlncRNAs. Our findings uncovered 97 CDG-RlncRNAs significantly associated with adverse patient outcomes (Figure 1E).
Identification of ccRCC immune subtypes based on CDG-RlncRNA expression
Precision medicine has emerged as a crucial focus in contemporary clinical practice, emphasizing molecular subtyping of patients as an effective strategy. To explore this, we conducted a NMF analysis based on the expression profiles of 97 CDG-RlncRNAs closely associated with the prognostic outlook of ccRCC patients. Our analysis resulted in the stratification of ccRCC samples into three distinct clusters, as illustrated in Figure 2A. Subsequent survival analysis revealed that patients within cluster 3 exhibited the most unfavorable OS, as demonstrated in Figure 2B. Furthermore, we thoroughly investigated the correlations between different clusters and the clinical attributes of ccRCC, visually presented in Figure 2C. Moreover, we delved into the differential expression of immune checkpoint molecules among the distinct clusters. Our findings illuminated that, among the six immune checkpoints scrutinized (PD1, CTLA-4, ICOS, LAG3, TIGIT, and PD-L1), five manifested elevated expression levels within cluster 3, as portrayed in Figure 2D-2I. This observation implies that despite the diminished OS within cluster 3, immunotherapeutic interventions might hold promise as a viable strategy for ameliorating the prognosis of this specific patient subset.
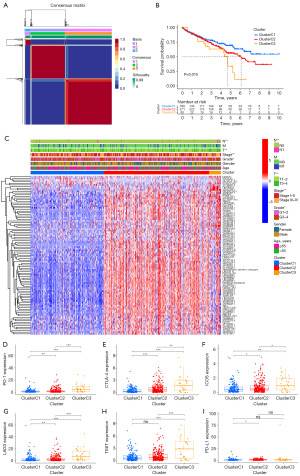
Construction of CDG-RlncRNA model for predicting prognosis
To enhance the prognostic prediction for ccRCC patients, we conducted LASSO regression analysis on 97 CDG-related genes closely associated with patient prognosis. We established a 5-gene model (CDG-RlncM) specifically related to ccRCC patient OS, as illustrated in Figure 3A-3C. Subsequently, a nomogram was developed based on patient clinical characteristics and risk scores (Figure 3D), and the predictive accuracy of CDG-RlncM was evaluated using calibration curves (Figure 3E). Through time-dependent receiver operating characteristic (ROC) curve analysis, we determined that the CDG-RlncM risk score reached 0.712 (Figure 3F). Additionally, we established a nomogram associated with ccRCC patient progression-free survival (PFS) (Figure 3G). Similarly, we observed good predictive accuracy for this nomogram through calibration curves (Figure 3H).
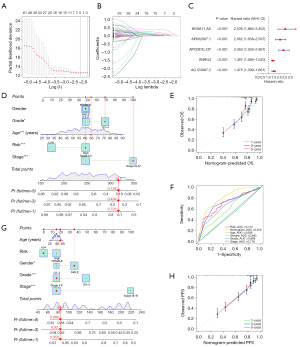
Validating the CDG-RlncRNA prognostic model
We successfully established the CDG-RlncM using the TCGA database as our training set. Subsequently, we utilized the coefficients of the five CDG-RlncRNAs within the model to calculate the median risk score, thereby categorizing patients into high-risk and low-risk groups. As the risk score increased, there was a corresponding decrease in the number of surviving clear ccRCC patients, as depicted in Figure 4A. The ROC curve illustrated that CDG-RlncM exhibited highly effective diagnostic performance in predicting 5-year survival, with an AUC of 0.712 (Figure 4B). Survival analysis revealed a noticeable reduction in OS among high-risk patients (Figure 4C). Furthermore, we conducted a similar validation using the ICGC database as our validation set. The ROC AUC for 5-year survival prediction was found to be 0.682, indicating a similarly poorer prognosis for high-risk ccRCC patients (Figure 4D-4F). These results collectively suggest that the CDG-RlncM we constructed demonstrated excellent applicability and predictive performance.
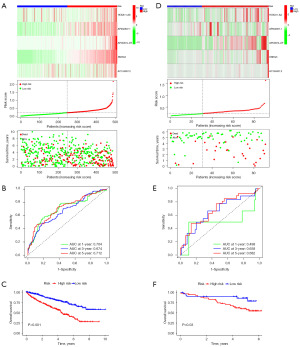
Correlation between CDG-RlncRNA prognostic model and clinical characteristics of ccRCC patients
We conducted an in-depth exploration of the correlation between CDG-RlncM and clinical characteristics. Initially, a heatmap was utilized to illustrate the associations between the five CDG-RlncRNAs within the model and various clinical attributes (Figure 5A). Subsequently, we observed a progressive elevation in risk scores with increasing grade (Figure 5B), stage (Figure 5C), or T-stage (Figure 5D). Patients with distant metastasis (Figure 5E) or lymph node metastasis (Figure 5F) exhibited a noticeable increase in their risk scores. However, there were no significant differences in risk scores based on patient age (Figure 5G) or gender (Figure 5H).
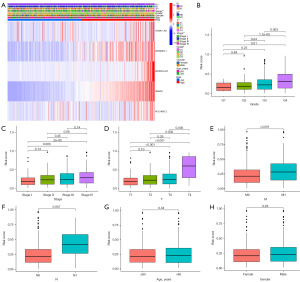
Predictive value of CDG-RlncM signature
We conducted separate univariate and multivariate Cox regression risk analyses for CDG-RlncM and the nomogram. Both analyses revealed a close association between CDG-RlncM and the OS of ccRCC patients (Figure 6A-6D). This suggests that the impact of CDG-RlncM on the survival prognosis of ccRCC patients can be considered as an independent risk factor. Furthermore, stratified analysis of clinical characteristics in ccRCC patients demonstrated that, in nearly all subgroups, the high-risk group exhibited significantly shorter OS compared to the low-risk group (Figure 6E-6J).
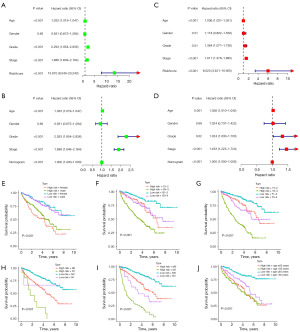
Relationship between the CDG-RlncM signature and immunotherapy and immune cell infiltration
To explore the biological characteristics of DEGs in the high- and low-risk groups within the training set, we conducted GO enrichment and KEGG pathway analyses. The GO analysis revealed significant enrichment of DEGs in pathways related to immune responses (Figure 7A), while the KEGG functional enrichment analysis indicated that DEGs were primarily enriched in various cytokine signaling pathways (Figure 7B). These findings underscore the close association of CDG-RlncM with the immune system. Furthermore, we observed a strong correlation between risk scores and immune checkpoint molecules such as CTLA4, PD1, PDL1, HAVCR2, and PDCD1LG2 (Figure 7C). In addition, comparative analysis of immune cell infiltration levels between the two risk groups using CIBERSORT revealed higher infiltration of follicular helper T cells and regulatory T cells in patients with higher risk scores, while resting dendritic cells and resting mast cells exhibited higher infiltration levels in patients with lower risk scores (Figure 7D). Moreover, we unveiled the correlation between the five genes in our model and immune cell infiltration (Figure 7E). Subsequent single-sample gene set enrichment analysis (ssGSEA) demonstrated that co-stimulatory T cells were significantly activated in the high-risk group, while type II interferon responses were notably suppressed (Figure 7F). We also employed the TIDE algorithm to evaluate the correlation between risk groups and the potential effectiveness of ICIs. The results indicated that the high-risk group had higher TIDE scores than the low-risk group (Figure 7G). In summary, these results suggest that patients in the high-risk group are more likely to experience immune evasion. Therefore, caution is warranted when considering immunotherapeutic strategies for such patients.
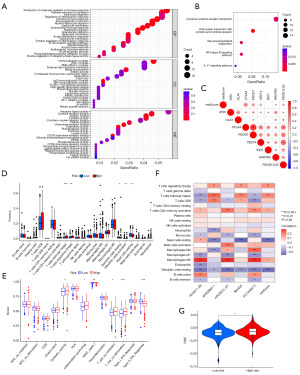
Knockdown of SNHG3 induces G2/M phase arrest in ccRCC cells
In the five genes of CDG-RlncM, we identified a remarkable gene, namely SNHG3. SNHG3 exhibited elevated expression in ccRCC (Figure 8A), and high SNHG3 expression was associated with adverse outcomes in ccRCC patients (Figure 8B). Furthermore, by collecting ccRCC tissues and adjacent normal tissues, we confirmed the elevated expression level of SNHG3 in ccRCC (Figure 8C). In renal cancer cells, we observed the most significant upregulation of SNHG3 in 786-O cells (Figure 8D). Subsequently, we conducted online analysis of SNHG3 using GTBAdb, and the results demonstrated that SNHG3 was primarily involved in the cell cycle, particularly the G2/M checkpoint (Figure 8E-8H). Furthermore, we performed a knockdown experiment of SNHG3 in 786-O cells (Figure 8I). The results revealed that it induced G2/M phase arrest in the cells (Figure 8J,8K), confirming the role of SNHG3 in cell cycle regulation in ccRCC.
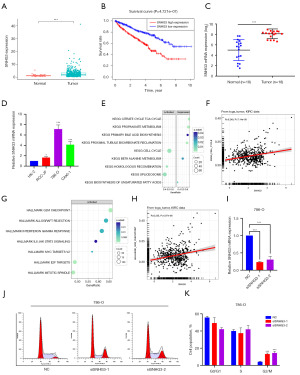
Discussion
ccRCC stands as the most prevalent type of kidney cancer, constituting 80% of all cases (22). About 30% of patients are already in the advanced stages of ccRCC or present with metastases at the time of initial diagnosis, missing the opportunity for surgical intervention (23,24). Although targeted therapy and immunotherapy have emerged as the primary adjunctive treatment modalities for advanced ccRCC, the rates of complete remission and partial remission remain relatively modest (25,26). With the highly developed bioinformatics technology today, it is necessary to conduct research aimed at identifying tumor-specific biomarkers associated with the occurrence and progression of ccRCC, in order to improve the survival rates of ccRCC patients and provide new strategies. In previous studies, researchers have mainly focused on exploring single-gene prognostic biomarkers in ccRCC using this method, such as CREB1 (27), ACSL4 (28), NR3C2 (29), which have been identified as potential prognostic markers for ccRCC. However, research on the role of gene models as prognostic markers is scarce. It is necessary to study the role of gene signatures as prognostic markers for ccRCC, providing new strategies for the management of ccRCC patients in clinical practice.
A recent study extensively analyzed 28,076 tumor samples from 66 different cancer types and successfully identified 568 CDGs (22). These crucial genes play specific roles in regulating cell growth, the cell cycle, and DNA replication. Mutations in these genes enable malignant cells to proliferate rapidly and uncontrollably, evade the immune system and other defense mechanisms, spread, invade other tissues, and alter the surrounding environment to fulfill their own needs (30,31). Long non-coding RNAs (lncRNAs) are a class of non-coding RNAs with a length exceeding 200 nucleotides (32), and some lncRNAs play pivotal roles in the occurrence and progression of cancer, possessing characteristics similar to CDGs (33). Therefore, the identification of CDG-RlncRNAs and the development of signatures based on them for predicting the prognosis of ccRCC patients will hopefully provide new strategies to improve the survival rate of ccRCC patients.
In our study, we initially conducted univariate Cox regression analysis to identify CDG-RlncRNAs closely associated with the prognosis of 97 ccRCC patients. Subsequently, using their expression profiles, we employed NMF analysis to classify ccRCC patients into three clusters. Results indicated that, despite cluster 3 having the poorest prognosis, these patients exhibited elevated expression of immune checkpoint markers compared to the other two clusters, suggesting that cluster 3 patients possessed higher immunogenicity. Consequently, immunotherapy targeting cluster 3 patients may potentially yield more favorable outcomes. Subsequently, we constructed a CDG-RlncM consisting of 5 CDG-RlncRNAs (HOXA11-AS, AP002807.1, APCDD1L-DT, AC124067.2, and SNHG3) using LASSO regression analysis to predict the prognosis and survival of ccRCC patients. Among these, HOXA11-AS has been reported to be upregulated in various tumors, including gliomas (34-36), lung adenocarcinomas (37,38), hypopharyngeal squamous cell carcinomas (39,40), colorectal cancers (41), gastric cancers (42,43), osteosarcomas (44), liver cancers (45,46), and breast cancers (47,48), promoting the progression of these malignancies. AP002807.1 has been reported to participate in the construction of a prognostic model related to autophagy in ccRCC (49). Similarly, APCDD1L-DT has been reported to be involved in the construction of a prognostic model related to cuproptosis in ccRCC (50), indicating their crucial roles in the development of ccRCC. Additionally, AC124067.2 has been implicated in constructing a prognostic model related to autophagy in non-small cell lung cancer, predicting survival risk in this malignancy (22). Furthermore, SNHG3 has been found to be upregulated in a variety of tumors, including bladder cancer (51), HCC (52,53), prostate cancer (54,55), cervical cancer (56), colorectal carcinoma (57), non-small cell lung cancer (58-60), ovarian cancer (61), gastric cancer (62), and acute myeloid leukemia (63), with its upregulation promoting the malignant progression of these cancers. In ccRCC, our study revealed an association between SNHG3 and adverse prognosis, and we validated the upregulation of SNHG3 in ccRCC patient samples and cell lines. An online analysis using GTBAdb database highlighted the significant correlation of SNHG3 with the cell cycle. Therefore, we conducted experiments to knock down SNHG3 in 786-O cells, which confirmed that silencing SNHG3 induced G2/M phase arrest in ccRCC. In conclusion, our findings suggest that SNHG3 may influence the progression of ccRCC by regulating the cell cycle.
In summary, we constructed the CDG-RlncM signature using data from TCGA database. Subsequently, successful validation of this signature was achieved using the ICGC database, confirming its effectiveness and reliability in predicting survival and prognosis of ccRCC patients. Additionally, preliminary validation of the key gene SNHG3 within the model confirmed its role in regulating the cell cycle of ccRCC. However, it is essential to note that our model has only been validated in the TCGA and ICGC databases, and further validation in additional databases or ones constructed independently is necessary to establish the universality of CDG-RlncM. Furthermore, our current validation of SNHG3 is limited to phenotype assessment, requiring further research to delve into its primary functions and specific mechanisms. These endeavors will contribute valuable insights for the clinical treatment strategies of ccRCC.
Conclusions
This study explores the prognostic value of CDG-RlncRNA in ccRCC. We conducted further clustering of ccRCC patients, investigated differences between clusters, and constructed a risk model composed of CDG-RlncRNAs. The model successfully predicts the OS of ccRCC patients and their response to immunotherapy. Additionally, a representative gene in the model, SNHG3, was validated in ccRCC cells.
Acknowledgments
Funding: None.
Footnote
Reporting Checklist: The authors have completed the TRIPOD reporting checklist. Available at https://tcr.amegroups.com/article/view/10.21037/tcr-24-127/rc
Data Sharing Statement: Available at https://tcr.amegroups.com/article/view/10.21037/tcr-24-127/dss
Peer Review File: Available at https://tcr.amegroups.com/article/view/10.21037/tcr-24-127/prf
Conflicts of Interest: All authors have completed the ICMJE uniform disclosure form (available at https://tcr.amegroups.com/article/view/10.21037/tcr-24-127/coif). The authors have no conflicts of interest to declare.
Ethical Statement: The authors are accountable for all aspects of the work in ensuring that questions related to the accuracy or integrity of any part of the work are appropriately investigated and resolved. The research was conducted following the principles of the Helsinki Declaration (as revised in 2013). This study has been approved by the Ethics Committee at the People’s Hospital of Chongqing Hechuan (No. Hcry-Yn-01), and informed consent was obtained from all individual participants.
Open Access Statement: This is an Open Access article distributed in accordance with the Creative Commons Attribution-NonCommercial-NoDerivs 4.0 International License (CC BY-NC-ND 4.0), which permits the non-commercial replication and distribution of the article with the strict proviso that no changes or edits are made and the original work is properly cited (including links to both the formal publication through the relevant DOI and the license). See: https://creativecommons.org/licenses/by-nc-nd/4.0/.
References
- Padala SA, Barsouk A, Thandra KC, et al. Epidemiology of Renal Cell Carcinoma. World J Oncol 2020;11:79-87. [Crossref] [PubMed]
- Siegel RL, Giaquinto AN, Jemal A. Cancer statistics, 2024. CA Cancer J Clin 2024;74:12-49. [Crossref] [PubMed]
- Signoretti S, Flaifel A, Chen YB, et al. Renal Cell Carcinoma in the Era of Precision Medicine: From Molecular Pathology to Tissue-Based Biomarkers. J Clin Oncol 2018; Epub ahead of print. [Crossref] [PubMed]
- Srigley JR, Delahunt B, Eble JN, et al. The International Society of Urological Pathology (ISUP) Vancouver Classification of Renal Neoplasia. Am J Surg Pathol 2013;37:1469-89. [Crossref] [PubMed]
- Lane BR, Kattan MW. Prognostic models and algorithms in renal cell carcinoma. Urol Clin North Am 2008;35:613-25. vii. [Crossref] [PubMed]
- Motzer RJ, Escudier B, McDermott DF, et al. Nivolumab versus Everolimus in Advanced Renal-Cell Carcinoma. N Engl J Med 2015;373:1803-13. [Crossref] [PubMed]
- Majer W, Kluzek K, Bluyssen H, et al. Potential Approaches and Recent Advances in Biomarker Discovery in Clear-Cell Renal Cell Carcinoma. J Cancer 2015;6:1105-13. [Crossref] [PubMed]
- Riazalhosseini Y, Lathrop M. Precision medicine from the renal cancer genome. Nat Rev Nephrol 2016;12:655-66. [Crossref] [PubMed]
- Zhang C, Zeng J, Ye C, et al. Construction and validation of a chromatin regulator-related gene signature for prognostic and therapeutic significance of clear cell renal cell carcinoma. Transl Cancer Res 2024;13:150-72. [Crossref] [PubMed]
- Shao Y, Wu B, Yang Z, et al. ALDOB represents a potential prognostic biomarker for patients with clear cell renal cell carcinoma. Transl Androl Urol 2023;12:549-71. [Crossref] [PubMed]
- Chen J, Ye Z, Liu L, et al. Assessment of the prognostic value of SPOCK1 in clear cell renal cell carcinoma: a bioinformatics analysis. Transl Androl Urol 2022;11:509-18. [Crossref] [PubMed]
- Leroi AM, Koufopanou V, Burt A. Cancer selection. Nat Rev Cancer 2003;3:226-31. [Crossref] [PubMed]
- Hanahan D. Hallmarks of Cancer: New Dimensions. Cancer Discov 2022;12:31-46. [Crossref] [PubMed]
- Hanahan D, Weinberg RA. Hallmarks of cancer: the next generation. Cell 2011;144:646-74. [Crossref] [PubMed]
- Zou J, Qin W. Comprehensive analysis of the cancer driver genes constructs a seven-gene signature for prediction of survival and tumor immunity in hepatocellular carcinoma. Front Genet 2022;13:937948. [Crossref] [PubMed]
- Guo H, Lu F, Lu R, et al. A novel tumor 4-driver gene signature for the prognosis of hepatocellular carcinoma. Heliyon 2023;9:e17054. [Crossref] [PubMed]
- Tessema M, Rossi MR, Picchi MA, et al. Common cancer-driver mutations and their association with abnormally methylated genes in lung adenocarcinoma from never-smokers. Lung Cancer 2018;123:99-106. [Crossref] [PubMed]
- Zhao Y, Dong Y, Zhao R, et al. Expression Profiling of Driver Genes in Female Never-smokers With Non-adenocarcinoma Non-small-cell Lung Cancer in China. Clin Lung Cancer 2020;21:e355-62. [Crossref] [PubMed]
- Zhang X, Pan C, Wei X, et al. Cancer-keeper genes as therapeutic targets. iScience 2023;26:107296. [Crossref] [PubMed]
- Zhao X, Lei Y, Li G, et al. Integrative analysis of cancer driver genes in prostate adenocarcinoma. Mol Med Rep 2019;19:2707-15. [Crossref] [PubMed]
- Schinke EN, Bii V, Nalla A, et al. A novel approach to identify driver genes involved in androgen-independent prostate cancer. Mol Cancer 2014;13:120. [Crossref] [PubMed]
- Martínez-Jiménez F, Muiños F, Sentís I, et al. A compendium of mutational cancer driver genes. Nat Rev Cancer 2020;20:555-72. [Crossref] [PubMed]
- Gong J, Maia MC, Dizman N, et al. Metastasis in renal cell carcinoma: Biology and implications for therapy. Asian J Urol 2016;3:286-92. [Crossref] [PubMed]
- Heo JH, Park C, Ghosh S, et al. A network meta-analysis of efficacy and safety of first-line and second-line therapies for the management of metastatic renal cell carcinoma. J Clin Pharm Ther 2021;46:35-49. [Crossref] [PubMed]
- Krishna C, DiNatale RG, Kuo F, et al. Single-cell sequencing links multiregional immune landscapes and tissue-resident T cells in ccRCC to tumor topology and therapy efficacy. Cancer Cell 2021;39:662-677.e6. [Crossref] [PubMed]
- Hu J, Chen Z, Bao L, et al. Single-Cell Transcriptome Analysis Reveals Intratumoral Heterogeneity in ccRCC, which Results in Different Clinical Outcomes. Mol Ther 2020;28:1658-72. [Crossref] [PubMed]
- Zhang Z, Guan B, Li Y, et al. Increased phosphorylated CREB1 protein correlates with poor prognosis in clear cell renal cell carcinoma. Transl Androl Urol 2021;10:3348-57. [Crossref] [PubMed]
- Guo N. Identification of ACSL4 as a biomarker and contributor of ferroptosis in clear cell renal cell carcinoma. Transl Cancer Res 2022;11:2688-99. [Crossref] [PubMed]
- Chen D, Yin Z, Chen Y, et al. Validation of prognostic signature and exploring the immune-related mechanisms for NR3C2 in clear cell renal cell carcinoma. Transl Cancer Res 2023;12:2518-32. [Crossref] [PubMed]
- Porta-Pardo E, Godzik A. Mutation Drivers of Immunological Responses to Cancer. Cancer Immunol Res 2016;4:789-98. [Crossref] [PubMed]
- Sinkala M. Mutational landscape of cancer-driver genes across human cancers. Sci Rep 2023;13:12742. [Crossref] [PubMed]
- Fedorko M. Long non-coding RNAs and renal cell carcinoma. Klin Onkol 2020;33:340-9. [PubMed]
- Deng Y, Luo S, Zhang X, et al. A pan-cancer atlas of cancer hallmark-associated candidate driver lncRNAs. Mol Oncol 2018;12:1980-2005. [Crossref] [PubMed]
- Wei C, Zhang X, Peng D, et al. LncRNA HOXA11-AS promotes glioma malignant phenotypes and reduces its sensitivity to ROS via Tpl2-MEK1/2-ERK1/2 pathway. Cell Death Dis 2022;13:942. [Crossref] [PubMed]
- Xu CH, Xiao LM, Liu Y, et al. The lncRNA HOXA11-AS promotes glioma cell growth and metastasis by targeting miR-130a-5p/HMGB2. Eur Rev Med Pharmacol Sci 2019;23:241-52. [PubMed]
- Xu C, He T, Li Z, et al. Regulation of HOXA11-AS/miR-214-3p/EZH2 axis on the growth, migration and invasion of glioma cells. Biomed Pharmacother 2017;95:1504-13. [Crossref] [PubMed]
- Lv X, Fang Z, Qi W, et al. Long Non-coding RNA HOXA11-AS Facilitates Proliferation of Lung Adenocarcinoma Cells via Targeting the Let-7c-5p/IGF2BP1 Axis. Front Genet 2022;13:831397. [Crossref] [PubMed]
- Zhao X, Li X, Zhou L, et al. LncRNA HOXA11-AS drives cisplatin resistance of human LUAD cells via modulating miR-454-3p/Stat3. Cancer Sci 2018;109:3068-79. [Crossref] [PubMed]
- Meng Y, Jin M, Yuan D, et al. Solamargine Inhibits the Development of Hypopharyngeal Squamous Cell Carcinoma by Decreasing LncRNA HOXA11-As Expression. Front Pharmacol 2022;13:887387. [Crossref] [PubMed]
- Xu J, Bo Q, Zhang X, et al. lncRNA HOXA11-AS Promotes Proliferation and Migration via Sponging miR-155 in Hypopharyngeal Squamous Cell Carcinoma. Oncol Res 2020;28:311-9. [Crossref] [PubMed]
- Ren YL, Zhang W. Propofol promotes apoptosis of colorectal cancer cells via alleviating the suppression of lncRNA HOXA11-AS on miRNA let-7i. Biochem Cell Biol 2020;98:90-8. [Crossref] [PubMed]
- Liu Y, Zhang YM, Ma FB, et al. Long noncoding RNA HOXA11-AS promotes gastric cancer cell proliferation and invasion via SRSF1 and functions as a biomarker in gastric cancer. World J Gastroenterol 2019;25:2763-75. [Crossref] [PubMed]
- Liu Z, Chen Z, Fan R, et al. Over-expressed long noncoding RNA HOXA11-AS promotes cell cycle progression and metastasis in gastric cancer. Mol Cancer 2017;16:82. [Crossref] [PubMed]
- Cao K, Fang Y, Wang H, et al. The lncRNA HOXA11-AS regulates Rab3D expression by sponging miR-125a-5p promoting metastasis of osteosarcoma. Cancer Manag Res 2019;11:4505-18. [Crossref] [PubMed]
- Wang S, Zhang S, He Y, et al. HOXA11-AS regulates JAK-STAT pathway by miR-15a-3p/STAT3 axis to promote the growth and metastasis in liver cancer. J Cell Biochem 2019;120:15941-51. [Crossref] [PubMed]
- Guo JC, Yang YJ, Zheng JF, et al. Silencing of long noncoding RNA HOXA11-AS inhibits the Wnt signaling pathway via the upregulation of HOXA11 and thereby inhibits the proliferation, invasion, and self-renewal of hepatocellular carcinoma stem cells. Exp Mol Med 2019;51:1-20. [Crossref] [PubMed]
- Li W, Jia G, Qu Y, et al. Long Non-Coding RNA (LncRNA) HOXA11-AS Promotes Breast Cancer Invasion and Metastasis by Regulating Epithelial-Mesenchymal Transition. Med Sci Monit 2017;23:3393-403. [Crossref] [PubMed]
- Su JC, Hu XF. Long non‑coding RNA HOXA11‑AS promotes cell proliferation and metastasis in human breast cancer. Mol Med Rep 2017;16:4887-94. [Crossref] [PubMed]
- Li X, Yu H, Wei Z, et al. A Novel Prognostic Model Based on Autophagy-Related Long Non-Coding RNAs for Clear Cell Renal Cell Carcinoma. Front Oncol 2021;11:711736. [Crossref] [PubMed]
- Hong P, Huang W, Du H, et al. Prognostic value and immunological characteristics of a novel cuproptosis-related long noncoding RNAs risk signature in kidney renal clear cell carcinoma. Front Genet 2022;13:1009555. [Crossref] [PubMed]
- Dai G, Huang C, Yang J, et al. LncRNA SNHG3 promotes bladder cancer proliferation and metastasis through miR-515-5p/GINS2 axis. J Cell Mol Med 2020;24:9231-43. [Crossref] [PubMed]
- Zhang PF, Wang F, Wu J, et al. LncRNA SNHG3 induces EMT and sorafenib resistance by modulating the miR-128/CD151 pathway in hepatocellular carcinoma. J Cell Physiol 2019;234:2788-94. [Crossref] [PubMed]
- Zhang F, Lu J, Yang J, et al. SNHG3 regulates NEIL3 via transcription factor E2F1 to mediate malignant proliferation of hepatocellular carcinoma. Immunogenetics 2023;75:39-51. [Crossref] [PubMed]
- Hu M, Ren M, Zhao Z, et al. Long non-coding RNA SNHG3 promotes prostate cancer progression by sponging microRNA-1827. Oncol Lett 2022;24:281. [Crossref] [PubMed]
- Wang X, Song Y, Shi Y, et al. SNHG3 could promote prostate cancer progression through reducing methionine dependence of PCa cells. Cell Mol Biol Lett 2022;27:13. [Crossref] [PubMed]
- Zhu H, Zhu C, Feng X, et al. Long noncoding RNA SNHG3 promotes malignant phenotypes in cervical cancer cells via association with YAP1. Hum Cell 2022;35:320-32. [Crossref] [PubMed]
- Zhang Y, Li L, Lu KX, et al. LncRNA SNHG3 is responsible for the deterioration of colorectal carcinoma through regulating the miR-370-5p/EZH1 axis. Eur Rev Med Pharmacol Sci 2021;25:6131-7. [PubMed]
- Li Y, Gao L, Zhang C, et al. LncRNA SNHG3 Promotes Proliferation and Metastasis of Non-Small-Cell Lung Cancer Cells Through miR-515-5p/SUMO2 Axis. Technol Cancer Res Treat 2021;20:15330338211019376. [Crossref] [PubMed]
- Zhao L, Song X, Guo Y, et al. Long non‑coding RNA SNHG3 promotes the development of non‑small cell lung cancer via the miR‑1343‑3p/NFIX pathway. Int J Mol Med 2021;48:147. [Crossref] [PubMed]
- He WW, Ma HT, Guo X, et al. lncRNA SNHG3 accelerates the proliferation and invasion of non-small cell lung cancer by downregulating miR-340-5p. J Biol Regul Homeost Agents 2020;34:2017-27. [PubMed]
- Liu EL, Zhou YX, Li J, et al. Long-Chain Non-Coding RNA SNHG3 Promotes the Growth of Ovarian Cancer Cells by Targeting miR-339-5p/TRPC3 Axis. Onco Targets Ther 2020;13:10959-71. [Crossref] [PubMed]
- Xie Y, Rong L, He M, et al. LncRNA SNHG3 promotes gastric cancer cell proliferation and metastasis by regulating the miR-139-5p/MYB axis. Aging (Albany NY) 2021;13:25138-52. [Crossref] [PubMed]
- Peng L, Zhang Y, Xin H. lncRNA SNHG3 facilitates acute myeloid leukemia cell growth via the regulation of miR-758-3p/SRGN axis. J Cell Biochem 2020;121:1023-31. [Crossref] [PubMed]