Identifying key pathogenic mechanisms and potential intervention targets for recurrence after laryngeal cancer treatment through bioinformatics screening
Highlight box
Key findings
• Through bioinformatics methods, we successfully identified the key gene ATP6V1B1 associated with laryngeal cancer recurrence, whose high expression may be closely related to the recurrence of laryngeal cancer, and plays a role by affecting pro-inflammatory and fibrotic mediators, glutathione metabolism, and other mechanisms.
What is known and what is new?
• Metagenomics has revealed a strong correlation between specific gene activation/expression levels and liver cancer recurrence.
• We have systematically explored the molecular mechanisms behind laryngeal cancer recurrence by combining bioinformatics analysis and clinical data for the first time. Through gene expression profiling analysis, protein-protein interaction network construction, and enrichment analysis, we can discover new potential biomarkers and gain a deeper understanding of how these genes affect the progression and recurrence of laryngeal cancer.
What is the implication, and what should change now?
• The significance of this study lies in providing new theoretical basis for the clinical diagnosis and treatment of laryngeal cancer. The discovery of ATP6V1B1 provides a potential new target for developing targeted therapies for laryngeal cancer recurrence. In addition, our study also emphasizes the importance of early intervention and personalized treatment, which is crucial for improving the survival rate and quality of life of laryngeal cancer patients. Therefore, future research should further explore the regulatory mechanism of ATP6V1B1 and develop effective therapeutic methods targeting this gene.
Introduction
Laryngeal cancer (LC) is an aggressive tumor that develops from the laryngeal mucosal epithelium. It comprises around 13.9% of head and neck malignancies and 2.1% of all malignant tumors worldwide (1,2). In 2019, there were 209,000 new cases of LC worldwide, with a standardized incidence rate that decreased by 2.5% (3). The exact causes of LC remain unclear but it is thought to be associated with environmental factors, viruses, sex hormones, deficiency in trace elements, and radiation exposure (3).
The mainstay of clinical treatment of LC is surgery that aims to excise the lesion as thoroughly as possible while also striving to restore and reconstruct the patient’s ability to speak, breathe, and swallow (4,5). However, some patients already exhibit metastasis at the time of surgery, leading to incomplete treatment and an increased risk of postoperative metastasis or recurrence. Recurrence is a leading cause of surgical treatment failure and has a direct effect on the long-term survival of LC patients (6).
Following the refinement of targeted therapy and precision radiotherapy treatment plans, the early initiation of these treatments in LC patients at risk of recurrence has recently been shown to effectively extend their disease-free survival time and reduce the likelihood of disease recurrence and metastasis (7). Research on patient prognosis has always been a hot topic in clinical studies of LC (7). Previous clinical studies have constructed prognostic prediction models based on LC patient clinical data, and laboratory, and radiological findings. However, due to environmental, ethnic, and lifestyle differences, the reproducibility of such studies has been poor.
Recently, with the formation of metagenomics, there has been an increase in studies examining the correlation between individual genes and LC recurrence (8-10). These studies have reported a high correlation between the activation and expression levels of specific human genes and LC. This correlation has been reproducibly demonstrated in patients of the same ethnicity, providing better reference values for early intervention (8-10).
This study employed bioinformatics analysis methods to conduct a comprehensive search of gene expression microarray chips related to LC samples in the Gene Expression Omnibus (GEO) database (https://www.ncbi.nlm.nih.gov/geo/). We investigated the specific enriched pathways of pivotal genes to provide evidence of the pathologic mechanisms related to disease recurrence. Finally, we also analyzed the clinical data of LC patients who underwent surgery in the First Affiliated Hospital of Wannan Medical College. The primary aim of the study was to elucidate the clinical significance of crucial genes by immunohistochemically examining their expression in LC tissues, while also evaluating the association between that expression and the recurrence of LC in patients. We present this article in accordance with the STREGA reporting checklist (available at https://tcr.amegroups.com/article/view/10.21037/tcr-24-1015/rc).
Methods
Microarray data information
The present study identified the differentially expressed genes (DEGs) from the tumor tissues of early stage LC patients with and without postoperative recurrence as documented in the GSE25727 data set. These data underwent normalization and filtering preprocessing. Based on annotation information from the GEO database, all the identification numbers were associated with their respective gene symbols, and duplicate gene names were removed using the average method.
Detection of DEGs
The limma package (https://www.bioconductor.org/) was employed to screen for the DEGs across all data sets. The following selection criteria were applied: P<0.05 and log |fold change (FC)| ≥1. The DEGs with a log FC ≥1 and a log FC ≤−1 were considered upregulated and downregulated, respectively.
Functional enrichment analysis
The clusterProfiler package in R (https://www.bioconductor.org/) was used to conduct a functional enrichment analysis of the DEGs. These genes were classified into two high- and low-expression groups, depending on the levels of the crucial genes. The variations in the biological states of these groups were then evaluated using a Gene Set Enrichment Analysis (GSEA, V.4.2.3 https://www.gsea-msigdb.org/gsea/index.jsp). The gene data set needed for this study was obtained from the Molecular Signatures Database v.7.5.1 (https://www.gsea-msigdb.org/gsea/index.jsp). The C2 and C5 subsets of the Kyoto Encyclopedia of Genes and Genomes (KEGG) and Gene Ontology (GO) databases, respectively, were employed to perform the functional enrichment analyses. Screening criteria: false discovery rate (FDR) <25% and P<0.05 are considered significantly enriched.
Construction of the protein-protein interaction (PPI) network and detection of the hub genes
To analyze the PPI network of the DEGs, the Search Tool for the Retrieval of Interacting Genes/Proteins (STRING) database (https://cn.string-db.org/) was employed. Cytoscape v.3.9.1 (https://cytoscape.org/) was used to visualize the positions and relationships of the key DEGs in the PPI network. The Molecular Complex Detection (MCODE) plugin in Cytoscape was employed to filter central clusters, which aided in the identification of potential functional modules and activated signaling pathways.
Construction of the transcription factor (TF)-DEG-microRNA (miRNA) network
The interaction data for the TF-DEG, and miRNA-DEG analyses were obtained from the MiRTarBase v.8.0 (https://mirtarbase.cuhk.edu.cn/) and ENCODE databases (https://www.encodeproject.org/). The regulators of the miRNAs and TFs that underwent reciprocal changes were identified in the DEGs, and the respective TFs and miRNAs were isolated. Finally, an integrated TF-DEG-miRNA network was established and displayed using Cytoscape.
GSEA
An online functional analysis was conducted via Metascape (https://metascape.org/) to identify the DEGs for a further functional analysis. A GSEA was used to reveal whether the abundance of a whole set of genomes significantly increased or decreased in biological processes. Identifying shared signaling pathways and regulating network modules helps to understand the underlying mechanisms of biological differences between different samples.
Clinical data of LC patients
The clinical information of the LC patients who were surgically treated at the First Affiliated Hospital of Wannan Medical College between January 2020 and December 2023 was included in this retrospective analysis. To be eligible for inclusion in this study, the patients had to meet the following inclusion criteria: (I) be aged ≥18 years; (II) have positive detection results for LC based on the standard surgical treatment; (III) have histopathological result of squamous cell carcinoma with negative margins; (IV) have attended postoperative follow-up through outpatient visits, including laryngoscopy and imaging examinations, for which the follow-up period ended on December 31, 2023. Patients were excluded from the study if they met any of the following exclusion criteria: (I) had insufficient clinical data; (II) had concomitant other tumors; (III) had uncertain results as to postoperative recurrence; and/or (IV) were lost to follow up. The study was conducted in accordance with the Declaration of Helsinki (as revised in 2013). The study was approved by the Ethics Committee of the First Affiliated Hospital of Wannan Medical College (No. LLSC-2020-003) and informed consent was taken from all the patients.
Observational indicators and follow-up
The general clinical data of the patients were gathered, including their gender, age, body mass index (BMI), smoking status, comorbidities, tumor location, tumor-regional lymph node-metastasis (TNM) staging, differentiation degree, initial surgical approach, and postoperative radiotherapy and chemotherapy (yes/no). The follow-up period for all the study subjects began on the day of surgery and lasted until the occurrence of recurrence, or the follow-up cut-off date. Based on recurrence, the study subjects were divided into recurrence and non-recurrence groups.
Tissue microarray construction and immunohistochemistry
The tissue was fixed overnight at 4 ℃ in 10% formalin, and paraffin-embedded, and the sections were then mounted onto slides. The sections were cleared using xylene for 10 minutes. After thoroughly cleaning the slides with deionized water for 5 minutes, the sections were immersed in an 80% solution of alcohol (100%) for 2 minutes. These sections were kept in a solution of 90% methanol/3% H2O at 25 ℃ for 15 minutes to inhibit endogenous peroxidases. Next, the sections were blocked in a blocking solution (host serum diluted with tris buffered saline (TBS) at a 1:10 ratio) for 1 hour at 37 ℃. The sections were covered with ATP6V1B1 antibody (A6876, ABclonal, Wuhan, China) diluted in blocking solution (ratio: 1:50) and kept for 1 hour at 37 ℃. Prediluted universal secondary antibody [Alexa Fluor 594-conjugated AffiniPure Goat Anti-Rabbit Immunoglobulin G (IgG) (H+L) # AS074, Wuhan Fine Biological Technology Co., LTD, Wuhan, China] was used to counterstain the tissue sections that were then incubated at 37 ℃ for 30 minutes. Hematoxylin was used to stain the sections, and the sections were sealed with nail polish.
Grading scores were assigned based on the percentage of the positive-stained cells: 0 (negative), 1 (≤25%), 2 (26–50%), 3 (51–75%), and 4 (>75%), and the staining intensity: 0 (negative or no stain), 1 (weak), 2 (moderate), and 3 (strong). These two scores were multiplied to yield the final score for each specimen. The arithmetic mean of these scores was calculated to determine the cut-off value for the high and low expression of the key genes.
Statistical analysis
The statistical analysis was conducted using SPSS 24.0 software (https://www.ibm.com/spss). The categorical data were expressed as the case (percentage), and were compared using the χ2 test. The quantitative data were expressed as the mean ± standard deviation, and were analyzed using the t-test. GraphPad Prism 7.0 software (https://www.graphpad-prism.cn/) was employed to graph the data and statistical analysis results. For the DEG analysis, the t-test was employed to calculate the P values and adjusted P values. The P values were adjusted using the FDR, and a P value <0.05 was considered statistically significant.
Results
Data normalization
We analyzed the DEGs of the tumor tissues of early stage LC patients with and without postoperative recurrence from the GSE25727 data set in the GEO database. To address any technical and systematic variability, the data underwent preprocessing, normalization, and cross-comparability adjustments. To determine the biological variability among each sample, a principal component analysis (PCA) was performed. The PCA plot illustrates the different expressions of the gene profiles (Figure 1A), and the boxplot displays the range of gene expression for each sample (Figure 1B). The raw data were robustly normalized to ensure the reliability of the data for the downstream analysis.
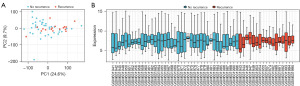
Detection of DEGs
All the DEGs were detected using the limma program in R as per the following criteria: |log (FC)| ≥1, and P<0.05. In total, 248 upregulated genes and 34 downregulated genes were detected. Figure 2A shows a volcano plot of the DEGs, while Figure 2B shows the considerable variations in the gene expression levels.
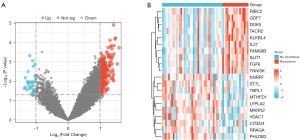
Functional enrichment analysis of the DEGs
A GO enrichment analysis was carried out using clusterProfiler (Figure 3A,3B) to investigate the biological roles of the known DEGs. The DEGs in the cellular component (CC) category were shown to be enriched in various structures, such as the photoreceptor cell cilium, non-motile cilium, inactive cilium, nucleomorph, and mitochondrial nucleomorph. In terms of their molecular functions (MFs), the DEGs were primarily involved in glycosaminoglycan binding and olfactory receptor activity.
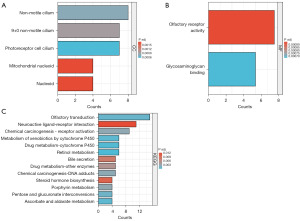
A KEGG pathway enrichment analysis was conducted to identify the main pathways associated with the DEGs (Figure 3C). These genes were found to be considerably higher in the pathways associated with retinol, ascorbate and aldarate, olfactory transduction, pentose and glucuronate interconversions, porphyrin metabolisms, bile secretion, steroid hormone biosynthesis, and the interaction of neuroactive ligand-receptors. Table 1 details the five most enriched DEGs.
Table 1
Ontology | ID | Description | GeneRatio | BgRatio | P value | p. adjust |
---|---|---|---|---|---|---|
CC | GO:0097733 | Photoreceptor cell cilium | 7/230 | 121/19,594 | 0.0006 | 0.0006 |
GO:0097730 | Non-motile cilium | 8/230 | 166/19,594 | 0.0008 | 0.0008 | |
GO:0097731 | 9+0 non-motile cilium | 7/230 | 132/19,594 | 0.0010 | 0.0010 | |
GO:0009295 | Nucleoid | 4/230 | 44/19,594 | 0.0017 | 0.0017 | |
GO:0042645 | Mitochondrial nucleoid | 4/230 | 44/19,594 | 0.0017 | 0.0017 | |
MF | GO:0005539 | Glycosaminoglycan binding | 10/227 | 234/18,410 | 0.0007 | 0.0007 |
GO:0004984 | Olfactory receptor activity | 14/227 | 430/18,410 | 0.0009 | 0.0009 | |
KEGG | hsa00830 | Retinol metabolism | 6/114 | 68/8,164 | 0.0004 | 0.0004 |
hsa00982 | Drug metabolism-cytochrome P450 | 6/114 | 72/8,164 | 0.0005 | 0.0005 | |
hsa00980 | Metabolism of xenobiotics by cytochrome P450 | 6/114 | 78/8,164 | 0.0007 | 0.0007 | |
hsa00053 | Ascorbate and aldarate metabolism | 4/114 | 30/8,164 | 0.0007 | 0.0007 | |
hsa04740 | Olfactory transduction | 15/114 | 439/8,164 | 0.0011 | 0.0011 |
GO, Gene Ontology; KEGG, Kyoto Encyclopedia of Genes and Genomes; CC, cellular component; MF, molecular functions.
Construction of the PPI network of the DEGs
The PPI network, which was constructed using the STRING database, demonstrates the functional connections between the proteins encoded by the DEGs (Figure 4A). Most of the proteins encoded by the DEGs were highly interconnected with other proteins. In addition, based on module analysis using MCODE, the most important modules were selected from the PPI network, including five genes (RRAGA, SLC38A9, WDR24, ATP6V1B1, and LAMTOR3) (Figure 4B). As per the GEO database, the expression changes of these genes also varied considerably between the two groups (Figure 4C).
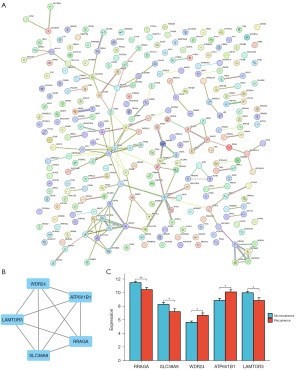
Development of the TF-DEG-miRNA network
To examine the functional activities of the DEGs, we explored the possible regulatory connections between the DEGs and TFs, as well as those between the DEGs and miRNAs. A network analysis of the DEGs was conducted using the miRTarBase database, and the miRNA-DEG pairs were identified. Based on the genotypic coordinates and TF binding site data presented by ENCODE, potential regulatory associations between the DEGs and TFs were detected (Figure 5A). The results revealed that ATP6V1B1 may interact with 17 miRNAs and is primarily regulated by one TF (Figure 5B).
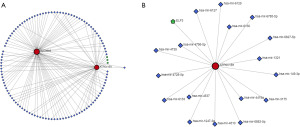
Signaling pathway prediction using a GSEA
Metascape was used to conduct the GSEA and functional analysis of ATP6V1B1 online. Our findings suggest that ATP6V1B1 may influence the occurrence and development of LC by participating in pathways that affect pro-inflammatory and pro-fibrotic mediators, glutathione metabolism, matrix metalloproteases, immune regulation, and interactions between lymphocytes (Figure 6).
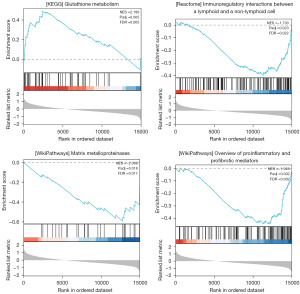
General clinical data of LC patients in the postoperative recurrence and non-recurrence groups
This study included 83 LC patients, of whom 16 (19.28%) experienced postoperative recurrence, and 67 (80.72%) did not experience postoperative recurrence. Based on the arithmetic mean calculated from the immunohistochemistry results, specimens with a score <6 were defined as having a low expression of ATP6V1B1. The immunohistochemical results of the tissues are shown in Figure 7.
There were no substantial differences between the two groups in terms of gender, age, BMI, history of hypertension, history of diabetes, smoking history, primary site, M stage, histopathological type, and type of initial surgery (P>0.05). However, compared to patients in the non-recurrence group, those in the recurrence group had higher T stages, higher N stages, and lower tissue differentiation, and higher levels of ATP6V1B1 (P<0.05). Further, a significant difference was observed between the two groups in terms of postoperative radiotherapy and chemotherapy (P<0.05) (Table 2).
Table 2
Characteristics | Recurrence group (n=16) | No-recurrence group (n=67) | t/χ2 | P |
---|---|---|---|---|
Age (year), mean ± SD | 63.27±5.28 | 64.08±4.99 | 0.5770 | 0.57 |
Male/female | 14/2 | 55/12 | 0.2696 | 0.60 |
BMI (kg/m2), mean ± SD | 20.88±2.64 | 21.09±2.15 | 0.3356 | 0.74 |
History of hypertension, n | 0.0093 | 0.92 | ||
Yes | 6 | 26 | ||
No | 10 | 41 | ||
History of diabetes mellitus, n | 0.0563 | 0.81 | ||
Yes | 2 | 7 | ||
No | 14 | 60 | ||
Smoking, n | 0.3098 | 0.58 | ||
Yes | 13 | 50 | ||
No | 3 | 17 | ||
Primary site, n | 0.0526 | 0.82 | ||
Supraglottic | 5 | 19 | ||
Glottic | 11 | 48 | ||
T stage, n | 18.88 | <0.001 | ||
T1 | 1 | 36 | ||
T2 | 1 | 10 | ||
T3 | 4 | 10 | ||
T4 | 10 | 11 | ||
N stage, n | 9.130 | 0.01 | ||
N0 | 9 | 57 | ||
N1 | 2 | 6 | ||
N2 | 5 | 4 | ||
M stage, n | 0.0283 | 0.87 | ||
M0 | 15 | 62 | ||
M1 | 1 | 5 | ||
Tissue differentiation, n | 12.66 | 0.002 | ||
High | 3 | 11 | ||
Medium | 6 | 50 | ||
Low | 7 | 6 | ||
Primary surgical procedure, n | 3.715 | 0.16 | ||
Partial laryngectomy | 9 | 46 | ||
Total laryngectomy | 2 | 13 | ||
CO2 laser therapy | 5 | 8 | ||
Postoperative radiotherapy, n | 4.212 | 0.04 | ||
Yes | 5 | 40 | ||
No | 11 | 27 | ||
Postoperative chemotherapy, n | 4.270 | 0.04 | ||
Yes | 4 | 36 | ||
No | 12 | 31 | ||
ATP6V1B1 expression, n | 11.11 | <0.001 | ||
High | 12 | 20 | ||
Low | 4 | 47 |
LC, laryngeal cancer; SD, standard deviation; BMI, body mass index.
Risk factor analysis of the postoperative recurrence of LC
The variables with a P value ≤0.1 were incorporated as covariates in the univariate logistic regression. The results showed that tumor T3 stage (P=0.04), tumor T4 stage (P=0.01), and a high expression of ATP6V1B1 (P=0.02) were risk factors for recurrence after surgical treatment in patients with LC (Table 3).
Table 3
Characteristics | EXP(B) (95% CI) | P |
---|---|---|
T stage | ||
T1 | 1.00 | |
T2 | 1.956 (0.052–73.725) | 0.72 |
T3 | 26.269 (1.223–564.076) | 0.04 |
T4 | 42.803 (2.499–733.112) | 0.01 |
N stage | ||
N0 | 1.00 | |
N1 | 2.431 (0.188–31.376) | 0.50 |
N2 | 2.893 (0.314–26.666) | 0.35 |
Tissue differentiation | ||
High | 1.00 | |
Medium | 1.348 (0.122–14.889) | 0.81 |
Low | 19.024 (0.966–374.653) | 0.053 |
Postoperative radiotherapy | ||
No | 1.00 | |
Yes | 0.588 (0.096–3.603) | 0.57 |
Postoperative chemotherapy | ||
No | 1.00 | |
Yes | 0.273 (0.043–1.742) | 0.17 |
ATP6V1B1 expression | ||
High | 1.00 | |
Low | 11.272 (1.541–82.430) | 0.02 |
LC, laryngeal cancer; CI, confidence interval.
Discussion
In China, LC is commonly treated with a comprehensive strategy that prioritizes surgery, radiotherapy, and chemotherapy. However, recurrence after the surgical treatment of LC is relatively common in clinical practice and severely affects patient prognosis. This may be related to the complex anatomical and physiological structure of the larynx, along with its rich blood circulation and lymphatic drainage. A domestic study reported that after recurrence, the 3-, 5-, and 10-year survival rates of LC patients were 68.9%, 53.6%, and 35.7%, respectively (11). Despite significant advances in the treatment of LC in recent years, its recurrence rate remains high at 16–40% (12,13).
In recent years, owing to the ongoing advancements in molecular biology methods, gene chip technology, and second-generation sequencing technology, the exploration of DEGs at the transcriptome level and the analysis of key genes have emerged as crucial methodologies for investigating the risk factors and molecular mechanisms underlying LC recurrence. Moreover, there is increasing evidence that the abnormal expression of certain genes plays a significant role in the occurrence and development of LC (14). The identification of crucial genes implicated in the pathogenesis, prevention, and treatment of LC is contingent on the detection of the DEG profiles associated with the disease.
In this study, through the analysis of the GSE25727 data set and the use of bioinformatics techniques, we identified 248 upregulated and 34 downregulated DEGs. The functional enrichment analyses of the GO and KEGG data revealed that these genes were involved in various pathways, such as retinol metabolism, the biosynthesis of steroid hormones, ascorbate and aldarate metabolism, interconversions between pentose and glucuronate, porphyrin metabolism, bile secretion, and neuroactive ligand-receptor interactions. Moreover, these pathways have been shown to be related to the development and advancement of LC in related studies. For example, Baek et al. (15) found that ascorbate induces tumor cell death in laryngeal squamous cell carcinoma Hep2 cells through the generation of reactive oxygen species, the activation of protein kinase C, and cytosolic calcium signaling. Min et al. (16) demonstrated that INPP4B-mediated tumor resistance is related to the regulation of glucose metabolism through hexokinase 2 in LC cells. Wang et al. (17) confirmed through transfection experiments that Wnt1-induced signaling protein 1 regulates glucose metabolism and cisplatin resistance in LC by modulating the expression of glucose transporter 1. Based on research that the Warburg effect promotes radioresistance in various malignancies, Zhong et al. (18) speculated that targeting the inhibition of the hexokinase-II signaling pathway in LC could enhance its radiosensitivity.
The construction of the PPI network of the DEGs and the TF-DEG-miRNA network revealed that only ATP6V1B1 was subject to TF regulation and the potential miRNA interaction. ATP6V1B1 encodes a non-catalytic subunit of the V1 complex of Vacuolar-type H+-ATPase (V-ATPase) (19), a multisubunit enzyme composed of peripheral (V1) and integral (VO) complexes that hydrolyzes ATP and translocates protons. V-ATPase acidifies and maintains the pH of intracellular compartments. In certain cell types, V-ATPase targets the plasma membrane and is responsible for acidifying the extracellular environment. Studies have shown that the proton pump properties of V-ATPases help cancer cells transfer excess intracellular hydrogen ions to the outside, reversing the transmembrane proton concentration gradient, avoiding the apoptosis of cancer cells, and creating a highly acidic extracellular environment crucial for cancer metastasis and invasion (20).
There has been no research on ATP6V1B1 in LC specifically, but some studies of other cancers, such as the study by Han et al. (21) have shown that the high expression of ATP6V1B1 is related to a poor prognosis and platinum chemotherapy resistance in epithelial ovarian cancer through immunohistochemistry, RNA sequencing, and analysis of public data sets. Nishie et al. (22) established trastuzumab-mediated antibody-dependent cellular cytotoxicity-resistant cells and compared the expression of intracellular pH regulation genes with wild-type cells. It was discovered that cancer cells could develop resistance to antibody-dependent cellular toxicity if the intracellular environment became more acidic due to the downregulation of ATP6V1B1.
The GSEA results suggest that ATP6V1B1 may influence LC recurrence through its involvement in pathways affecting pro-inflammatory and pro-fibrotic mediators, glutathione metabolism, matrix metalloproteinases, immune regulation, and interactions between lymphocytes. Multiple studies suggest that immune regulation plays a role in the recurrence of LC tumors, such as the study by Gong et al. (23), who showed that genomic instability and compromised immune responses are key characteristics of immune surveillance escape and relapse after early LC surgery. This study also revealed a weakened immune phenotype in recurrent tumors. Such findings provide valuable insights into the management of these high-risk patients. Chatzopoulos et al. (24) assessed tumor-infiltrating lymphocyte density in entire LC tumor tissue sections from a morphological perspective, and found that high cytotoxic T lymphocytes 8+ (CD8+) tumor patients had a 77% lower risk of recurrence and a 74% lower risk of death than low CD8+ tumor patients in lymph node-positive patients. Zhou et al. (25) also found that a high density of CD163+ cells around the tumor was associated with poorer overall survival after adjusting for tumor stage, relapse, and lymph node metastasis. These findings highlight different patterns of tumor-related immune cell penetration in LC, with the density and location of tumor-related immune cell penetration being related to the clinicopathological characteristics of LC. In summary, the functions of ATP6V1B1 can be delineated as follows: The tumor microenvironment typically exhibits low oxygen and low pH conditions. V-ATPase, encoded by the ATP6V1B1 gene, may influence tumor cell growth and invasion by modulating the acid-base balance within this environment (26). Tumor cells display unique metabolic characteristics, including enhanced glycolysis and active glutamine metabolism. The tumor microenvironment hosts various immune cells, such as T cells and NK cells, which exert anti-tumor effects through cytokine secretion and direct cytotoxicity. V-ATPase can indirectly affect immune cell activity and function by regulating lysosomal acidification, which impacts antigen presentation and cytotoxic efficacy (27,28). Additionally, the growth and metastasis of tumors are heavily reliant on angiogenesis, a process in which V-ATPase plays a crucial role (29,30). ATP6V1B1 may regulate V-ATPase activity, thereby influencing blood vessel formation and remodeling in the tumor microenvironment, facilitating tumor growth and metastasis. Furthermore, V-ATPase is integral to intracellular metabolism, participating in amino acid transport and metabolite excretion (31). Consequently, ATP6V1B1 affects tumor cell metabolic pathways and survival by modulating V-ATPase activity. Combining these research results, it appears that ATP6V1B1 may promote LC recurrence by weakening the immune phenotype of LC.
A total of 83 patients with LC were ultimately included in the present study. The patients had a recurrence rate of 19.3% (16/83). The immunohistochemistry results suggest that ATP6V1B1 is highly expressed in patients with recurrent LC, which aligns with the results of the bioinformatics analysis. A review of the clinical data of these LC patients revealed that T stage and ATP6V1B1 expression were risk factors for LC recurrence. Specifically, the risk of recurrence increased significantly in T3 and T4 stage patients, as well as in patients with high ATP6V1B1 expression. Therefore, close follow up is recommended for such LC patients after surgery.
A meta-analysis of 2007 patients with LC found that postoperative adjuvant radiotherapy reduces the composite risk ratio, is linked with better disease-free survival and local control rates, and thus improves the survival of patients with locally advanced LC undergoing surgery (32). For patients with LC, surgery can remove most tumor cells, while postoperative adjuvant radiotherapy can effectively eradicate subclinical cancer foci. Thus, the use of radiotherapy after LC surgery can help reduce the recurrence rate. However, due to the heterogeneity of LC patients, the decision to combine surgery with radiotherapy should be carefully considered, weighing its pros and cons.
Conclusions
In summary, the occurrence and recurrence of LC may involve the regulation of multiple factors. The key genes and related signaling pathways identified through our screening contribute to a deeper understanding of the molecular mechanisms of LC. Based on these findings, targeted treatment strategies for LC can be developed in the following aspects:
- Targeted therapy: specific therapeutic drugs can be designed for the key genes identified in our study. These drugs can directly target these genes or their products, inhibiting the proliferation and invasion of tumor cells, thereby slowing or preventing cancer progression.
- Immunotherapy: our study underscores the importance of immune regulation in LC recurrence. Immunotherapies, such as CAR-T cell therapy and PD-1/PD-L1 inhibitors, can be considered to enhance the patient’s immune system’s ability to recognize and eradicate tumor cells.
- Personalized treatment: by integrating the patient’s gene expression profile with clinical information, personalized treatment plans can be formulated. Assessing the expression levels of key genes in the patient’s body allows for predicting their response to various treatment strategies, enabling the selection of the most appropriate therapeutic approach.
- Early diagnosis and recurrence monitoring: Our research findings can be utilized to develop biomarkers for early diagnosis and monitoring of recurrence. By detecting changes in the expression of these key genes, it is possible to identify signs of cancer recurrence earlier and implement timely intervention measures. However, the current study also had some limitations, including the absence of relevant in vitro and in vivo experimental validations and clinical data verification. Future research, including multicenter, large-sample, prospective studies, should aim to further validate and explore these results.
Acknowledgments
Funding: None.
Footnote
Reporting Checklist: The authors have completed the STREGA reporting checklist. Available at https://tcr.amegroups.com/article/view/10.21037/tcr-24-1015/rc
Data Sharing Statement: Available at https://tcr.amegroups.com/article/view/10.21037/tcr-24-1015/dss
Peer Review File: Available at https://tcr.amegroups.com/article/view/10.21037/tcr-24-1015/prf
Conflicts of Interest: Both authors have completed the ICMJE uniform disclosure form (available at https://tcr.amegroups.com/article/view/10.21037/tcr-24-1015/coif). The authors have no conflicts of interest to declare.
Ethical Statement: The authors are accountable for all aspects of the work in ensuring that questions related to the accuracy or integrity of any part of the work are appropriately investigated and resolved. The study was conducted in accordance with the Declaration of Helsinki (as revised in 2013). The study was approved by the Ethics Committee of the First Affiliated Hospital of Wannan Medical College (No. LLSC-2020-003) and informed consent was taken from all the patients.
Open Access Statement: This is an Open Access article distributed in accordance with the Creative Commons Attribution-NonCommercial-NoDerivs 4.0 International License (CC BY-NC-ND 4.0), which permits the non-commercial replication and distribution of the article with the strict proviso that no changes or edits are made and the original work is properly cited (including links to both the formal publication through the relevant DOI and the license). See: https://creativecommons.org/licenses/by-nc-nd/4.0/.
References
- Cao ZZ, Bao YY, Chen Z, et al. Fibroblast-epithelial metabolic coupling in laryngeal cancer. Pathol Res Pract 2022;240:154177. [Crossref] [PubMed]
- Xiao H, Su QS, Li CQ. Identification of prognostic immune genes in laryngeal cancer. J Int Med Res 2020;48:300060520964662. [Crossref] [PubMed]
- Yi H, Chen C, Zhou S, et al. Comparison of three nutritional assessment methods associated with the prognostic impact of laryngeal cancer. Support Care Cancer 2023;31:737. [Crossref] [PubMed]
- Wang K, Tang J, Liu X, et al. UBR5 regulates proliferation and radiosensitivity in human laryngeal carcinoma via the p38/MAPK signaling pathway. Oncol Rep 2020;44:685-97. [Crossref] [PubMed]
- Huynh E, Boyle S, Campbell J, et al. Technical note: Toward implementation of MR-guided radiation therapy for laryngeal cancer with healthy volunteer imaging and a custom MR-CT larynx phantom. Med Phys 2022;49:1814-21. [Crossref] [PubMed]
- Wang G, Li G, Wu J, et al. Analysis of prognostic factors for Tis-2N0M0 early glottic cancer with different treatment methods. Braz J Otorhinolaryngol 2022;88:375-80. [Crossref] [PubMed]
- Hoffmann TK. Total Laryngectomy-Still Cutting-Edge? Cancers (Basel) 2021;13:1405. [Crossref] [PubMed]
- Sun M, Chen S, Fu M. Model establishment of prognostic-related immune genes in laryngeal squamous cell carcinoma. Medicine (Baltimore) 2021;100:e24263. [Crossref] [PubMed]
- Liu Y, Gao Z, Peng C, et al. Construction of a 10-gene prognostic score model of predicting recurrence for laryngeal cancer. Eur J Med Res 2022;27:249. [Crossref] [PubMed]
- Cui J, Wang L, Zhong W, et al. Identification and validation of methylation-driven genes prognostic signature for recurrence of laryngeal squamous cell carcinoma by integrated bioinformatics analysis. Cancer Cell Int 2020;20:472. [Crossref] [PubMed]
- Li P, Hu W, Zhu Y, et al. Treatment and predictive factors in patients with recurrent laryngeal carcinoma: A retrospective study. Oncol Lett 2015;10:3145-52. [Crossref] [PubMed]
- Hay A, Simo R, Hall G, et al. Outcomes of salvage surgery for the oropharynx and larynx: a contemporary experience in a UK Cancer Centre. Eur Arch Otorhinolaryngol 2019;276:1153-9. [Crossref] [PubMed]
- Đokanović D, Gajanin R, Gojković Z, et al. Clinicopathological Characteristics, Treatment Patterns, and Outcomes in Patients with Laryngeal Cancer. Curr Oncol 2023;30:4289-300. [Crossref] [PubMed]
- Li J, Cao H, Yang J, et al. IGF2BP2-m6A-circMMP9 axis recruits ETS1 to promote TRIM59 transcription in laryngeal squamous cell carcinoma. Sci Rep 2024;14:3014. [Crossref] [PubMed]
- Baek MW, Cho HS, Kim SH, et al. Ascorbic Acid Induces Necrosis in Human Laryngeal Squamous Cell Carcinoma via ROS, PKC, and Calcium Signaling. J Cell Physiol 2017;232:417-25. [Crossref] [PubMed]
- Min JW, Kim KI, Kim HA, et al. INPP4B-mediated tumor resistance is associated with modulation of glucose metabolism via hexokinase 2 regulation in laryngeal cancer cells. Biochem Biophys Res Commun 2013;440:137-42. [Crossref] [PubMed]
- Wang L, Sun J, Gao P, et al. Wnt1-inducible signaling protein 1 regulates laryngeal squamous cell carcinoma glycolysis and chemoresistance via the YAP1/TEAD1/GLUT1 pathway. J Cell Physiol 2019;234:15941-50. [Crossref] [PubMed]
- Zhong JT, Zhou SH. Warburg effect, hexokinase-II, and radioresistance of laryngeal carcinoma. Oncotarget 2017;8:14133-46. [Crossref] [PubMed]
- Saxena V, Arregui S, Zhang S, et al. Generation of Atp6v1g3-Cre mice for investigation of intercalated cells and the collecting duct. Am J Physiol Renal Physiol 2023;325:F770-8. [Crossref] [PubMed]
- Vasanthakumar T, Rubinstein JL. Structure and Roles of V-type ATPases. Trends Biochem Sci 2020;45:295-307. [Crossref] [PubMed]
- Han GH, Yun H, Chung JY, et al. High ATP6V1B1 expression is associated with poor prognosis and platinum-based chemotherapy resistance in epithelial ovarian cancer. Oncol Rep 2023;49:102. [Crossref] [PubMed]
- Nishie M, Suzuki E, Hattori M, et al. Downregulated ATP6V1B1 expression acidifies the intracellular environment of cancer cells leading to resistance to antibody-dependent cellular cytotoxicity. Cancer Immunol Immunother 2021;70:817-30. [Crossref] [PubMed]
- Gong XY, Chen HB, Zhang LQ, et al. NOTCH1 mutation associates with impaired immune response and decreased relapse-free survival in patients with resected T1-2N0 laryngeal cancer. Front Immunol 2022;13:920253. [Crossref] [PubMed]
- Chatzopoulos K, Kotoula V, Manoussou K, et al. Tumor Infiltrating Lymphocytes and CD8+ T Cell Subsets as Prognostic Markers in Patients with Surgically Treated Laryngeal Squamous Cell Carcinoma. Head Neck Pathol 2020;14:689-700. [Crossref] [PubMed]
- Zhou L, Li Y, Gao W, et al. Assessment of tumor-associated immune cells in laryngeal squamous cell carcinoma. J Cancer Res Clin Oncol 2019;145:1761-72. [Crossref] [PubMed]
- Ikeuchi M, Inoue M, Miyahara H, et al. A pH imbalance is linked to autophagic dysregulation of inner ear hair cells in Atp6v1ba-deficient zebrafish. Biochem Biophys Res Commun 2024;699:149551. [Crossref] [PubMed]
- Quick JD, Silva C, Wong JH, et al. Lysosomal acidification dysfunction in microglia: an emerging pathogenic mechanism of neuroinflammation and neurodegeneration. J Neuroinflammation 2023;20:185. [Crossref] [PubMed]
- Ibrahim SA, Katara GK, Kulshrestha A, et al. Breast cancer associated a2 isoform vacuolar ATPase immunomodulates neutrophils: potential role in tumor progression. Oncotarget 2015;6:33033-45. [Crossref] [PubMed]
- Ibrahim SA, Kulshrestha A, Katara GK, et al. Cancer-associated V-ATPase induces delayed apoptosis of protumorigenic neutrophils. Mol Oncol 2020;14:590-610. [Crossref] [PubMed]
- Pamarthy S, Kulshrestha A, Katara GK, et al. The curious case of vacuolar ATPase: regulation of signaling pathways. Mol Cancer 2018;17:41. [Crossref] [PubMed]
- Wang N, Ren L, Danser AHJ, Vacuolar H. ATPase in Diabetes, Hypertension, and Atherosclerosis. Microcirculation 2024;31:e12855. [Crossref] [PubMed]
- Li M, Zhang T, Tan B, et al. Role of postoperative adjuvant radiotherapy for locally advanced laryngeal cancer: a meta-analysis. Acta Otolaryngol 2019;139:172-7. [Crossref] [PubMed]