Novel mitochondrial-related gene signature predicts prognosis and immunological status in glioma
Highlight box
Key findings
• The novel mitochondrial-related gene signatures (TYMP, TSFM, MGME1, BOLA3, TRMT5, NDUFA9) can predict prognosis and immunological status in glioma.
What is known and what is new?
• Mitochondria are key organelles critical for fulfilling the bioenergetic and biosynthetic needs of the cellular system. The relationship between mitochondria and diseases has also been studied for a long time.
• The prognostic model built from mitochondrial-related gene signatures can successfully predict the prognosis and reflect clinicopathological characteristics.
What is the implication, and what should change now?
• This study could better predict patient prognosis and inspire further research on mitochondria-related functions in glioma.
Introduction
Glioma is the most common primary intracranial malignancy. The average survival rate is approximately 15 months even if the patient undergoes surgical resection combined with chemotherapy and radiotherapy, and an average of 15,944 deaths per year are caused by malignant brain and other central nervous system tumors (1). Although there has been important progress in understanding the molecular pathogenesis and biology of glioblastoma, this has not translated into significantly improved outcomes for patients (2). How to address tumor heterogeneity, blood-brain barrier, stem cells, immune suppression, and DNA damage repair mechanisms in glioma is an obstacle in the process of transferring basic medical research to clinical practice (3).
Mitochondria are key organelles critical for fulfilling the bioenergetic and biosynthetic needs of the cellular system. The relationship between mitochondria and diseases has also been studied for a long time. Generally, nuclear-mitochondrial disease can be classified into four distinct groups: (I) disorders resulting from a reduction in mitochondrial DNA (mtDNA) stability; (II) disorders resulting from mutations in nuclear-encoded components or assembly factors of the oxidative phosphorylation (OXPHOS) system; (III) disorders resulting from mutations affecting mitochondrial translation and (IV) disorders due to defects in genes controlling mitochondrial network dynamics (4). In view of the complex consequences of mitochondrial dysfunction, attention is also turning to the study of the relationship between mitochondria and tumor, and mitochondrial metabolism is also a potential target for cancer therapy (5). Many by-products of mitochondrial metabolism due to mutations in enzyme genes, such as (R)-2-hydroxyglutarate (2-HG), succinate, and fumarate have been proved as promoters of tumor growth and progression (6). Besides, inadequate energy supply to immune cells and abnormal internal signal transduction due to mitochondrial dysfunction have also been shown to be mechanisms of tumor immune escape (7). Therefore, therapeutic strategies with mechanisms of action that target mitochondria could be candidates for use in the treatment of difficult-to-treat cancers like glioma (8). Selt et al. utilized BH3 mimetics to reduce metabolic activity and induce mitochondrial apoptosis in senescent pilocytic astrocytoma cells at only nano-molar concentrations (9). The inhibition of mitochondrial biogenesis has been proved as one of antitumor mechanisms in glioma for gamitrinib (10).
Currently, the prognostic role of mitochondrial-related genes (MRGs) in patients with glioma and their biological effects are still unclear. Therefore, we identified some MRGs and evaluated their prognostic effects based on publicly accessible glioma datasets. Then we constructed a prognostic model with these genes and validated it in The Cancer Genome Atlas (TCGA) and Chinese Glioma Genome Atlas (CGGA) datasets. On this basis, we further explored the clinical features and biological functions associated with these genes. We hope that our study could better predict patient prognosis and inspire further research on mitochondria-related functions in glioma. We present this article in accordance with the TRIPOD reporting checklist (available at https://tcr.amegroups.com/article/view/10.21037/tcr-23-2072/rc).
Methods
The workflow of data collection and analysis in this study is shown in Figure 1. The study was conducted in accordance with the Declaration of Helsinki (as revised in 2013).
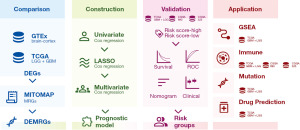
Data acquisition and processing
All gene expression profile and relevant clinical information (when available) in this study were obtained from three public databases: the Genotype-Tissue Expression project (GTEx, https://www.gtexportal.org/), TCGA (https://portal.gdc.cancer.gov/), and CGGA (http://www.cgga.org.cn). The comprehensive list of 147 mitochondrial genes was downloaded from the Human Mitochondrial Genome Database (MITOMAP, https://www.mitomap.org/MITOMAP) (table available at https://cdn.amegroups.cn/static/public/tcr-23-2072-1.xlsx). We filtered five sub-datasets for further analysis, including normal brain cortex data (n=255) from GTEx, TCGA-low grade glioma (LGG) (n=514) and TCGA-glioblastoma multiforme (GBM) (n=161) from TCGA, CGGA_325 (11-13) (n=325) and CGGA_693 (11,14,15) (n=693) from CGGA. Among these sub-datasets, TCGA was selected as training cohort to analyze differentially expressed MRGs (DEMRGs) and construct prognostic model; CGGA sub-datasets were chosen as validation cohort. In addition, an extended clinical information of TCGA patients based on bioinformatics analysis provided by Ceccarelli et al. (16) was selected to cross-validate the clinicopathological characteristics of patients in the TCGA and CGGA datasets (table available at https://cdn.amegroups.cn/static/public/tcr-23-2072-2.xlsx). The expression data from TCGA and GTEx were in the form of read counts when analyzing differentially expressed genes (DEGs) while all other analysis involving RNA-seq were in the form of transcripts per kilobase million (TPM).
Identification of DEGs
The expression data from TCGA-LGG and TCGA-GBM were integrated to compare with the normal tissue data from GTEx. After normalizing with the “limma” R package, Wilcoxon signed rank test was used for large samples differential expression analysis to obtain more accurate results (17). DEGs were identified with adjusted P<0.05 and |log2fold change (FC)| >1. After taking the intersection of DEGs and 147 mitochondrial genes, DEMRGs were then identified. These genes, categories and log2FC value were presented via “circlize” R package.
For further exhibiting the association between DEMRGs, spearman correlation was conducted and visualized by “reshape2” and “corrplot” R package respectively. Additionally, protein-protein interaction (PPI) analysis was also performed based on STRING database and visualized by “Cytoscape” software on Windows. Proteins were ranked in Cytoscape using cytoHubba with the Matthews correlation coefficient (MCC) algorithm.
Construction of prognostic model
To evaluate the prognostic value of different DEMRGs, the expression data of DEMRGs and associated survival information of patients from TCGA training cohort were combined for various Cox regression. Univariate Cox regression was utilized to screen prognostic related genes. Those genes with P<0.05 were chosen for applying the Least Absolute Shrinkage and Selection Operator (LASSO) Cox regression model provided from the “glmnet” R package. Next, to construct more precise prognostic model, multivariate Cox regression on the genes screened by LASSO were then performed. Finally, those genes with P<0.05 and non-zero coefficients were decided as gene signature to construct prognostic model with the following formula:
In this formula, n means all screened genes by multivariate Cox regression, and Ci and Ei mean the coefficient value and expression level of gene I, respectively.
Validation of prognostic model
The risk scores of patients from training cohort were calculated, and patients were divided into high-risk and low-risk groups using the median risk scores as the cutoff value. The principal components analysis (PCA) and the t-distributed stochastic neighbor embedding (t-SNE) for two groups were performed via the “ggpolt2” and the “Rtsne” R packages to ensure that the two groups were differentiated. The Kaplan-Meier curve was generated using the R package “survminer” to compare the overall survival (OS) between the high- and low-risk groups. If OS were statistically significant, the time-dependent receiver operating characteristic (ROC) curve was utilized to evaluate the prediction accuracy of prognostic model via the “timeROC” R package. The validation method above was applied to the validation cohorts as well.
Development and validation of nomogram
The clinicopathological characteristics of patients were combined with risk scores for univariate and multivariate regression analyses, just like the way of genes screening above, and plotted the nomogram from the multivariate regression results using the “RMS” package in R. The prediction performance of monogram for 1-, 3-, and 5-year survival was assessed by calibration plots.
Gene Set Enrichment Analysis (GSEA)
GSEA was conducted on the TCGA cohort for exploring differences in biological functions and pathways between the two groups. Similarly, the two groups of patients in TCGA were analyzed for DEGs using the method described above. Patients in the low-risk group were considered as the control group. Gene symbols and log2FC values were analyzed via “clusterProfiler” package. The “gmt” files were downloaded in GSEA website (http://www.gsea-msigdb.org/gsea/msigdb/collections.jsp), including hallmark gene sets and Kyoto Encyclopedia of Genes and Genomes (KEGG) subset of canonical pathways. Results were visualized via “ggplot2” package.
Analysis of tumor immune status
In this section, we investigate the association of prognostic models with the tumor immune microenvironment (TIME) of glioma in TCGA and CGGA cohorts. ESTIMATE package in R was used to calculate parameters related to the tumor microenvironment (18). “CIBERSORT” R script (19) was used to assess the level of immune cell infiltration. “GSVA” package was used to analyze the enrichment degree of immune-related pathways. The gene set of immune-related pathways was cited from a review by Bindea et al. (20) (table available at https://cdn.amegroups.cn/static/public/tcr-23-2072-3.xlsx). To investigate the association between MRGs and immune checkpoints, nine immune checkpoints from the literature that have been proved to be associated with glioma (21) were selected and their gene expression profiles analyzed, including PD-1 (PDCD1), LAG-3, CTLA-4, CD73 (NT5E), CD161 (KLRB1), IDO1, CD47, CD276 and CD39 (ENTPD1).
Analysis of somatic mutation
To demonstrate the landscape of somatic mutation in high- and low-risk groups, “TCGAbiolinks” package in R was utilized to download copy number variation (CNV) data from TCGA and integrate them with patients in different risk groups. Then the mutation profiles of risk groups were analyzed and visualized using “maftools” package in R. The genes with mutation rates greater than 5% in each group were taken as a concatenation, and the pathways enriched in mutated genes in each group were taken as an intersection, which were all screened using the fisher exact test for significantly different mutated genes and associated pathways.
Prediction for chemotherapeutic agents
The drug prediction was performed using “oncoPredict” package (22). The training data of cell lines expression and drug response information for 545 drugs came from The Cancer Therapeutics Response Portal (CTRP) (23-25). These data, together with the gene expression data of TCGA, were processed integratedly to get sensitivity scores that predict the half-maximal inhibitory concentration (IC50) of drugs for glioma patients. After that, the top 4 Food and Drug Administration (FDA) approved drugs and top 2 FDA-experimental drugs that were positively and negatively correlated with risk scores would be selected as sensitive drugs.
Statistical analysis and visualization
All statistical analyses and figures except for PPI analysis were all completed by the R software (4.2.0 Build 485). P<0.05 was considered statistically significant differences. Most figures were tuned in Adobe Illustrator 2022 before publication for better viewing experience.
Results
Identification of 29 DEMRGs between normal and tumor tissues
A total of 675 cases of glioma tissues from TCGA-LGG and TCGA-GBM and 255 cases of normal tissues from GTEx were integrated to analysis. The differences in RNA-seq data of 9,425 genes between normal and tumor were statistically significant (table available at https://cdn.amegroups.cn/static/public/tcr-23-2072-4.xlsx), and subsequently 29 DEMRGs were identified from these genes. Among them, 17 DEMRGs were downregulated while 12 DEMRGs upregulated. Noteworthy, according to the definition of mitochondrial genes categories from MITOMAP, DEGs belonging to structural nuclear genes were all downregulated while DEGs belonging to MtDNA maintenance and coenzyme Q10 biogenesis were all upregulated (Figure 2A) The PPI network of these DEMRGs indicated that NDUFA12, NDUFA11, NDUFS7 and COX6A1 are hub genes (Figure 2B), while ATP8A2 does not interact with this network.
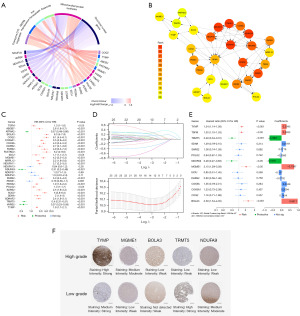
Construction of prognostic model with DEMRGs
To evaluate the prognostic value of each DEMRGs, univariate Cox regression analysis was applied to identify prognostic genes. The results showed that four genes (NDUFA13, NDUFS7, NDUFV2, PDSS1) were excluded for P>0.05. Among the remaining 25 genes, four genes (ATP8A2, NDUFA9, TRMT5, VARS2) had an improved effect for prognosis, while the remaining 21 genes had the opposite effect (Figure 2C). Subsequently, these prognostic genes were enrolled in the LASSO Cox regression analysis for narrowing down candidates according to the minimum penalty parameter (Lambda), finally identifying 14 genes (TYMP, TSFM, TRMT5, SDHA, SARS2, POLG2, NDUFA9, MGME1, ISCU, DARS2, COX8A, COX6A1, COQ2, BOLA3) for next multivariate Cox regression (Figure 2D) Eventually, six genes, including four risk genes (TYMP, TSFM, MGME1, BOLA3) and two protective genes (TRMT5, NDUFA9) were identified with non-zero coefficients (Figure 2E) to construct MRGs prognostic model as following:
Furthermore, to verify the expression of these genes in real-world tumor tissues, we found immunostaining images of five DEMRGs other than TSFM in high- and low-grade gliomas in the Human Protein Atlas (HPA) database (Figure 2F).
Validation of prognostic model built from MRGs
The robustness of the prognostic model built from MRGs was validated in both training cohort and validation cohort. The results of PCA (Figure S1A-S1C) and t-SNE (Figure S1D-S1F) indicated this prognostic model can well distinguish the two groups. The distribution of the risk score, expression level of 6 genes and prognostic status between high- and low-risk groups is shown in Figure S1G-S1I. Kaplan-Meier survival curves (Figure 3A-3C) indicted that patients in high-risk were more likely to display a significantly worse prognosis in TCGA, CGGA_325 and CGGA_693 datasets. The ROC curve, which reflects a combination of sensitivity and specificity in a predictive model, also showed that the model had good predictive performance. For example, the area under the curve (AUC) of the model in CGGA_325 was 0.76 at 1 year, 0.82 at 3 years, 0.85 at 5 years (Figure 3D). The TCGA cohort (Figure 3E) and the CGGA_693 cohort (Figure 3F) showed a similar pattern to the CGGA_325.
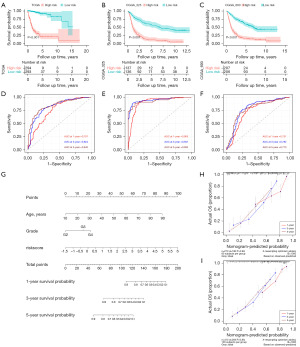
Next, the risk score was considered as independent factors to perform univariate and multivariate regression analysis together with other clinical features of the patients. In TCGA, eventually, age, grade and risk score were identified as statistically significant prognostic factors (Figure S2A,S2B) While grade, isocitrate dehydrogenase (IDH) mutation status, 1p/19q codeletion status and risk score were identified as statistically significant prognostic factors in both CGGA_325 (Figure S2C,S2D) and CGGA_693 (Figure S2E,S2F). The nomogram built from prognostic factors in TCGA is shown in Figure 3G, and the calibration curves of the nomogram validated in TCGA (Figure S2G), CGGA_325 (Figure 3H) and CGGA_693 (Figure 3I) also manifested a favorable consistence between observational and predictive values for OS.
Additionally, the association between risk scores and some clinicopathological features in CGGA_693 (Figure 4A) and CGGA_325 (Figure 4B) showed that higher risk scores were more likely to be associated with higher World Health Organization (WHO) grade, 1p/19q codeletion, IDH mutation and O6-methylguanine-DNA methyltransferase (MGMT) methylation. The results of TCGA (Figure S2H) were also similar. However, it is worth noting that this clinicopathological data was derived from bioinformatics analysis and the results contained not available (NA) values, which may be biased compared to the actual results.
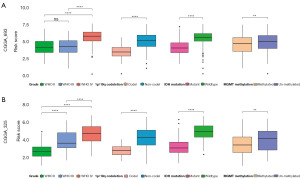
GSEA
Biological functional analysis between risk groups was performed based on DEGs and their log2FC value. The GSEA results for KEGG database (Figure 5A) showed that tumors in high-risk group were more involved in immune pathways [systemic lupus erythematosus, CC chemokine receptor (CCR) interaction, etc.], tumor formation (cell cycle, hematopoietic cell lineage, focal adhesion, etc.) and various signal pathways (Toll-like receptors, P53, chemokine, etc.). For tumors in low-risk group some different signal pathways involved (ERBB, phosphatidylinositol, calcium). In addition to these, long term potentiation, neuroactive ligand receptor interaction, gap junction, tight junction, etc. were involved. The GSEA results for HALLMARK database (Figure 5B) also mentioned many new signaling pathways that were enriched in high-risk group, like JAK-STAT3, m-TOR, KRAS, and new immune pathways, such as tumor necrosis factor (TNF)-α, interferon (IFN)-γ. In summary, tumorigenesis in the two groups involved many distinct biological mechanisms.
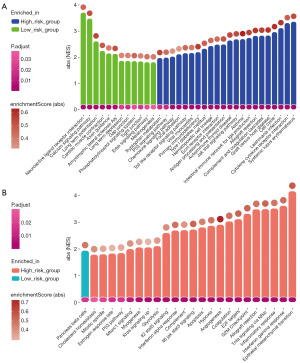
Prognostic model significantly correlated with immune status
Immune analyses were performed to explore the correlation between risk scores and immune status and immune differences between different risk groups. ESTIMATE results in TCGA cohorts revealed that risk scores are positively correlated with the presence of stroma (R=0.67; Figure 6A), the infiltration of immune cells (R=0.72; Figure 6B) and negatively correlated with tumor purity (R=−0.71; Figure 6C). And patients in the high-risk group showed a higher correlation for all three than in the low-risk group (0.45 vs. 0.2, 0.51 vs. 0.18, −0.49 vs. −0.19). These results imply higher tumor heterogeneity and a more complex immune environment in patients of high-risk group. Analysis of immune cell infiltration in TCGA (Figure 6D) and CGGA (Figure S3A,S3B) demonstrated that tumor in the low-risk group had higher infiltration of B memory cells, monocytes, and CD4 T naive cells, while tumor in the high-risk group had more infiltration of B naïve cells, macrophages, CD4 T memory cells, γδ T cells, and T reg cells. Further, the analysis between the prognostic model and immune checkpoints revealed that the risk score was correlated with immunotherapy checkpoints other than CD73 (NT5E). And the correlation between some immune checkpoints and risk score was high, such as PD-1 (PDCD1, R=0.6), IDO1 (R=0.68), CD276 (R=0.71) (Figure 6E), which suggest that patients in the high-risk group would suffer more for tumor immunosuppression related with these immune checkpoints. The situation was similar in CGGA (Figure S3C,S3D). Logically, tumor cells in the high-risk group would be more likely to be enriched in various immune pathways for immune escape, and the degree of enrichment was positively correlated with the risk score (Figure 6F). Some highly associated immune pathways and immune processes are of particular interest, such as JAK-STAT pathway (RIL6 JAK−STAT3 signaling =0.74, RJAK-STAT signaling pathway =0.72) and para-inflammation (R=0.8). Taken together, the results above demonstrated that prognostic models built from MRGs are associated with tumor heterogeneity, immune suppression and immune checkpoints.
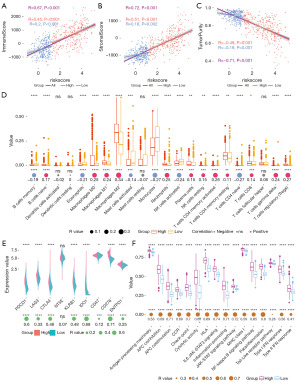
Analysis of somatic mutation
Somatic mutation analysis showed that the mutation rate of all MRGs genes did not exceed 1% (Figure S4A), suggesting that MRGs are generally stable in the development of glioma. However, the distribution of somatic mutations was dramatically different between the two groups (Figure 7A,7B). Fisher’s exact test showed that genes like TTN, EGFR, PTEN were more likely to be mutated in high-risk group while genes like ATRX, CIC, IDH1/2, FUBP1 were more likely to be mutated in low-risk group (Figure 7C). The pathways where these significantly mutated genes are located were also analyzed for high-risk (Figure S4B) and low-risk groups (Figure S4C). Fisher’s exact test showed that WNT, RTK-RAS, PI3K and cell cycle pathways were significantly affected by mutated genes (Figure 7D-7G) in high-risk group (Figure 7H).
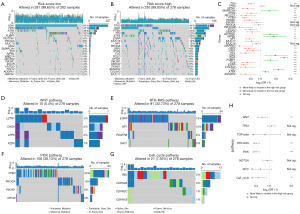
Chemotherapeutic agents prediction for different groups
Twelve chemotherapeutic agents with strongly correlated sensitivity and risk scores were selected among the 545 agents (Figure 8). Drugs sensitive to tumors in the high-risk group included olaparib, pevonedistat, cyclophosphamide, fulvestrant, abiraterone, and bleomycin A2, with olaparib and pevonedistat not yet FDA-approved. Drugs sensitive to tumors in the low-risk group included nilotinib, itraconazole, canertinib, lovastatin, sitagliptin, and pitstop2, with canertinib and pitstop2 not yet FDA-approved. The targets or activities of selected compounds, and the full result of prediction are shown in table available at https://cdn.amegroups.cn/static/public/tcr-23-2072-5.xlsx.
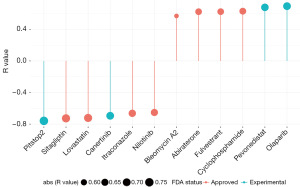
Discussion
It has been a consensus that mitochondria, as the center of cellular metabolism, have an important driving function in tumor. The tumor microenvironment with scarce resources makes the mitochondria in tumor cells often defective and mitochondria can promote tumor growth (26). Nowadays, most studies tend to focus on the effect of individual mitochondria-related gene, pathway, or component in glioma. The effects of all MRGs on glioma progress and prognosis are still unclear. In this study, we identified 29 MRGs that were aberrantly expressed in glioma tissues, and after analyzing their prognostic value, we constructed a prognostic model using six of them and validated its robustness. Compared to the low-risk group, patients in the high-risk group had worse OS and corresponding clinicopathological characteristics. GSEA and immunological analysis showed that patients in the high-risk group had higher tumor heterogeneity and significant immunosuppressive effects. In addition, we gave six potential therapeutic agents for each of the high- and low-risk groups.
Observing all DEMRGs, it is intriguing to find that genes belong to coenzyme Q10 biogenesis and mtDNA maintenance were all upregulated while genes belong to nuclear structure were all downregulated. Coenzyme Q10 (CoQ10) is an essential component of the mitochondrial respiratory chain for modulating gene expression, mitochondrial function and even cell death (27,28). Yen et al. experimentally explored that low level of CoQ10 predicted more malignancy and PDSS2 was significantly upregulated in astrocytoma (29), which is consistent with our data analysis, but the effect of PDSS1 and COQ2 had not been clarified due to technical reasons. Literature has reported a close association between the mutations of PDSS1 and COQ2 and eye diseases caused by primary CoQ10 deficiency syndrome (30). Research has also found that PDSS1 can regulate the cell cycle to induce cancer (31). In the field of gliomas, further research is still needed to elucidate the specific roles of these two genes. The results combined with our data analysis imply that upregulation of these three genes leads to decreased intracellular CoQ10. MtDNA, also known as mitochondrial genome, cannot replicate, repair itself and synthesize nucleotides without proteins used to maintain its integrity (32). The genes encoding these proteins are categorized as mtDNA maintenance. In this category, TYMP is responsible for nucleotide metabolism by thymidine phosphorylase synthesis. Excessive thymidine produced by highly expressed TYMP can imbalance mitochondrial deoxynucleotide pools and further lead to DNA mutations (33). MGME1 protein is a mitochondrial nuclease. Many studies have demonstrated that lacking MGME1 causes mutations and various mitochondrial-related diseases (34-36), but little is known about the role that high expression of MGME1 plays in tumor. Structural nuclear genes in mitochondria are closely related to cellular OXPHOS activities (37). These differential genes, especially for hub genes, display lower expression in gliomas and may imply abnormalities in OXPHOS function. Abnormal OXPHOS function promotes aerobic glycolysis rather than pyruvate oxidation, as known as Warburg effect, driving the aggressive character of tumors (38). Attempts have been made to restore oxidative phosphorylation function in glioblastoma by other means to stimulate tumor immunity (39). BOLA3 is an important gene involved in Fe-S cluster biosynthesis, which is a complex, stepwise process, and its dysfunction leads to a deficiency of mitochondrial respiratory complexes and impaired function of lipoic-acid-dependent enzymes. The role of Fe-S biogenesis in carcinogenesis is currently unclear (40,41). However, as this gene is a key prognostic gene in glioma, it could be a future research direction. The effect of TSFM and TRMT5 expression on tumor has not yet been reported in the literature, and more attention has been paid to the effects of their mutations on disease.
Although MRGs mutations are not the primary cause of glioma, mitochondrial function and gene mutations often interact with each other (33,42). Generally, IDH-mutated glioma exhibits a favorable disease outcome compared with its wild-type counterpart. One reason is that the imbalance of redox status in glioma cells can be rebalanced by the proline produced by the IDH mutation, thus maintaining cellular metabolism in mitochondria (43). It has been shown that gliomas with IDH1 mutations are also frequently accompanied by mutations in CIC or FUBP1, which is linked to codeletion of the 1p/19q arms where these genes reside (44). The data analysis in our study also came to this conclusion.
Immune status analysis and GSEA results similarly implied a profound influence of mitochondria on the TIME. JAK-STAT signaling pathway (45,46), Toll-like receptor pathway (47) and IFN response (48) all have immunosuppressive effects on gliomas via PD-L1. These may be an explanation for the downregulation of naïve T cells and upregulation of PD-1 expression in the high-risk group. Recently, new nanoparticles called IR-LND@Alb has successfully inhibited PD-L1 in tumor cells by targeting aberrant OXPHOS activities in mitochondria (49). In addition to PD-1/PDL1, some immune checkpoints with high correlation with mitochondria-related prognostic model, such as IDO1, CD276 and KLRB1, might be promising targets for combination of mitochondria-targeted drugs to jointly improve the prognosis of high-risk patients.
Some predicted chemotherapeutic agents had been tested in other studies. Olaparib is a mitochondrial complex I inhibitor. It was the most positively correlated drug in the results and has been proved to be effective in vitro against temozolomide-resistant human glioblastoma cells, particularly those with lower OXPHOS activities (50). Pevonedistat can upregulate PD-L1 expression in glioblastoma (51). Canertinib is sensitive to tumor cells in the low-risk group according to prediction results, probably because it is an ERBB pathway inhibitor that is significantly activated in low-risk tumors. And experiments have proved that it has a reduced effect on glioma cells with STAT3 activated (52). Therefore, the effect is better for tumor cells in the low-risk group with insignificant STAT3 activation.
It is glad to see parts of our results have been proven before, which shows the reliability of our analytical approaches. Undoubtedly, many of our conclusions are only derived from some public databases, and they still need to be demonstrated experimentally. The feasibility and utility of this prognostic model still need to be tested in future clinical practice. However, our study has initially elucidated the important role of MRGs in glioma prognosis and immune function. We hope our study will provide a new pathway for assessing patient prognosis and inspire further research regarding glioma mitochondria-related functions.
Conclusions
The novel MRG signatures (TYMP, TSFM, MGME1, BOLA3, TRMT5, NDUFA9) can predict prognosis and immunological status in glioma.
Acknowledgments
Funding: This work was supported by
Footnote
Reporting Checklist: The authors have completed the TRIPOD reporting checklist. Available at https://tcr.amegroups.com/article/view/10.21037/tcr-23-2072/rc
Peer Review File: Available at https://tcr.amegroups.com/article/view/10.21037/tcr-23-2072/prf
Conflicts of Interest: All authors have completed the ICMJE uniform disclosure form (available at https://tcr.amegroups.com/article/view/10.21037/tcr-23-2072/coif). The authors have no conflicts of interest to declare.
Ethical Statement: The authors are accountable for all aspects of the work in ensuring that questions related to the accuracy or integrity of any part of the work are appropriately investigated and resolved. The study was conducted in accordance with the Declaration of Helsinki (as revised in 2013).
Open Access Statement: This is an Open Access article distributed in accordance with the Creative Commons Attribution-NonCommercial-NoDerivs 4.0 International License (CC BY-NC-ND 4.0), which permits the non-commercial replication and distribution of the article with the strict proviso that no changes or edits are made and the original work is properly cited (including links to both the formal publication through the relevant DOI and the license). See: https://creativecommons.org/licenses/by-nc-nd/4.0/.
References
- Ostrom QT, Cioffi G, Gittleman H, et al. CBTRUS Statistical Report: Primary Brain and Other Central Nervous System Tumors Diagnosed in the United States in 2012-2016. Neuro Oncol 2019;21:v1-v100. [Crossref] [PubMed]
- Wen PY, Weller M, Lee EQ, et al. Glioblastoma in adults: a Society for Neuro-Oncology (SNO) and European Society of Neuro-Oncology (EANO) consensus review on current management and future directions. Neuro Oncol 2020;22:1073-113. [Crossref] [PubMed]
- Liu D, Dai X, Ye L, et al. Nanotechnology meets glioblastoma multiforme: Emerging therapeutic strategies. Wiley Interdiscip Rev Nanomed Nanobiotechnol 2023;15:e1838. [Crossref] [PubMed]
- Chinnery PF, Hudson G. Mitochondrial genetics. Br Med Bull 2013;106:135-59. [Crossref] [PubMed]
- Welch DR, Foster C, Rigoutsos I. Roles of mitochondrial genetics in cancer metastasis. Trends Cancer 2022;8:1002-18. [Crossref] [PubMed]
- Inigo JR, Chandra D. The mitochondrial unfolded protein response (UPRmt): shielding against toxicity to mitochondria in cancer. J Hematol Oncol 2022;15:98. [Crossref] [PubMed]
- Zhang L, Zhang W, Li Z, et al. Mitochondria dysfunction in CD8+ T cells as an important contributing factor for cancer development and a potential target for cancer treatment: a review. J Exp Clin Cancer Res 2022;41:227. [Crossref] [PubMed]
- Yamada Y, Sato Y, Nakamura T, et al. Innovative cancer nanomedicine based on immunology, gene editing, intracellular trafficking control. J Control Release 2022;348:357-69. [Crossref] [PubMed]
- Selt F, Sigaud R, Valinciute G, et al. BH3 mimetics targeting BCL-XL impact the senescent compartment of pilocytic astrocytoma. Neuro Oncol 2023;25:735-47. [Crossref] [PubMed]
- Wei S, Yin D, Yu S, et al. Antitumor Activity of a Mitochondrial-Targeted HSP90 Inhibitor in Gliomas. Clin Cancer Res 2022;28:2180-95. [Crossref] [PubMed]
- Zhao Z, Zhang KN, Wang Q, et al. Chinese Glioma Genome Atlas (CGGA): A Comprehensive Resource with Functional Genomic Data from Chinese Glioma Patients. Genomics Proteomics Bioinformatics 2021;19:1-12. [Crossref] [PubMed]
- Bao ZS, Chen HM, Yang MY, et al. RNA-seq of 272 gliomas revealed a novel, recurrent PTPRZ1-MET fusion transcript in secondary glioblastomas. Genome Res 2014;24:1765-73. [Crossref] [PubMed]
- Zhao Z, Meng F, Wang W, et al. Comprehensive RNA-seq transcriptomic profiling in the malignant progression of gliomas. Sci Data 2017;4:170024. [Crossref] [PubMed]
- Liu X, Li Y, Qian Z, et al. A radiomic signature as a non-invasive predictor of progression-free survival in patients with lower-grade gliomas. Neuroimage Clin 2018;20:1070-7. [Crossref] [PubMed]
- Wang Y, Qian T, You G, et al. Localizing seizure-susceptible brain regions associated with low-grade gliomas using voxel-based lesion-symptom mapping. Neuro Oncol 2015;17:282-8. [Crossref] [PubMed]
- Ceccarelli M, Barthel FP, Malta TM, et al. Molecular Profiling Reveals Biologically Discrete Subsets and Pathways of Progression in Diffuse Glioma. Cell 2016;164:550-63. [Crossref] [PubMed]
- Li Y, Ge X, Peng F, et al. Exaggerated false positives by popular differential expression methods when analyzing human population samples. Genome Biol 2022;23:79. [Crossref] [PubMed]
- Yoshihara K, Shahmoradgoli M, Martínez E, et al. Inferring tumour purity and stromal and immune cell admixture from expression data. Nat Commun 2013;4:2612. [Crossref] [PubMed]
- Chen B, Khodadoust MS, Liu CL, et al. Profiling Tumor Infiltrating Immune Cells with CIBERSORT. Methods Mol Biol 2018;1711:243-59. [Crossref] [PubMed]
- Bindea G, Mlecnik B, Tosolini M, et al. Spatiotemporal dynamics of intratumoral immune cells reveal the immune landscape in human cancer. Immunity 2013;39:782-95. [Crossref] [PubMed]
- Yang K, Wu Z, Zhang H, et al. Glioma targeted therapy: insight into future of molecular approaches. Mol Cancer 2022;21:39. [Crossref] [PubMed]
- Maeser D, Gruener RF, Huang RS. oncoPredict: an R package for predicting in vivo or cancer patient drug response and biomarkers from cell line screening data. Brief Bioinform 2021;22:bbab260. [Crossref] [PubMed]
- Rees MG, Seashore-Ludlow B, Cheah JH, et al. Correlating chemical sensitivity and basal gene expression reveals mechanism of action. Nat Chem Biol 2016;12:109-16. [Crossref] [PubMed]
- Seashore-Ludlow B, Rees MG, Cheah JH, et al. Harnessing Connectivity in a Large-Scale Small-Molecule Sensitivity Dataset. Cancer Discov 2015;5:1210-23. [Crossref] [PubMed]
- Basu A, Bodycombe NE, Cheah JH, et al. An interactive resource to identify cancer genetic and lineage dependencies targeted by small molecules. Cell 2013;154:1151-61. [Crossref] [PubMed]
- Altieri DC. Mitochondria in cancer: clean windmills or stressed tinkerers? Trends Cell Biol 2023;33:293-9. [Crossref] [PubMed]
- Wang Y, Hekimi S. The efficacy of coenzyme Q10 treatment in alleviating the symptoms of primary coenzyme Q10 deficiency: A systematic review. J Cell Mol Med 2022;26:4635-44. [Crossref] [PubMed]
- Cirilli I, Damiani E, Dludla PV, et al. Role of Coenzyme Q10 in Health and Disease: An Update on the Last 10 Years (2010-2020). Antioxidants (Basel) 2021;10:1325. [Crossref] [PubMed]
- Yen HC, Chen BS, Yang SL, et al. Levels of Coenzyme Q10 and Several COQ Proteins in Human Astrocytoma Tissues Are Inversely Correlated with Malignancy. Biomolecules 2022;12:336. [Crossref] [PubMed]
- Stallworth JY, Blair DR, Slavotinek A, et al. Retinopathy and optic atrophy in a case of COQ2-related primary coenzyme Q(10) deficiency. Ophthalmic Genet 2023;44:486-90. [Crossref] [PubMed]
- Rao Z, Li H, Yao W, et al. A novel HCC prognosis predictor PDSS1 affects the cell cycle through the STAT3 signaling pathway in HCC. Front Oncol 2022;12:927468. [Crossref] [PubMed]
- Almannai M, El-Hattab AW, Azamian MS, et al. Mitochondrial DNA maintenance defects: potential therapeutic strategies. Mol Genet Metab 2022;137:40-8. [Crossref] [PubMed]
- Copeland WC. Defects in mitochondrial DNA replication and human disease. Crit Rev Biochem Mol Biol 2012;47:64-74. [Crossref] [PubMed]
- Matic S, Jiang M, Nicholls TJ, et al. Mice lacking the mitochondrial exonuclease MGME1 accumulate mtDNA deletions without developing progeria. Nat Commun 2018;9:1202. [Crossref] [PubMed]
- Nicholls TJ, Zsurka G, Peeva V, et al. Linear mtDNA fragments and unusual mtDNA rearrangements associated with pathological deficiency of MGME1 exonuclease. Hum Mol Genet 2014;23:6147-62. [Crossref] [PubMed]
- Kornblum C, Nicholls TJ, Haack TB, et al. Loss-of-function mutations in MGME1 impair mtDNA replication and cause multisystemic mitochondrial disease. Nat Genet 2013;45:214-9. [Crossref] [PubMed]
- Fernandez-Vizarra E, Zeviani M. Mitochondrial disorders of the OXPHOS system. FEBS Lett 2021;595:1062-106. [Crossref] [PubMed]
- Velpula KK, Bhasin A, Asuthkar S, et al. Combined targeting of PDK1 and EGFR triggers regression of glioblastoma by reversing the Warburg effect. Cancer Res 2013;73:7277-89. [Crossref] [PubMed]
- Hasan MN, Luo L, Ding D, et al. Blocking NHE1 stimulates glioma tumor immunity by restoring OXPHOS function of myeloid cells. Theranostics 2021;11:1295-309. [Crossref] [PubMed]
- Wachnowsky C, Rao B, Sen S, et al. Reconstitution, characterization, and [2Fe-2S] cluster exchange reactivity of a holo human BOLA3 homodimer. J Biol Inorg Chem 2019;24:1035-45. [Crossref] [PubMed]
- Petronek MS, Spitz DR, Allen BG. Iron-Sulfur Cluster Biogenesis as a Critical Target in Cancer. Antioxidants (Basel) 2021;10:1458. [Crossref] [PubMed]
- Baran N, Lodi A, Dhungana Y, et al. Inhibition of mitochondrial complex I reverses NOTCH1-driven metabolic reprogramming in T-cell acute lymphoblastic leukemia. Nat Commun 2022;13:2801. [Crossref] [PubMed]
- Han S, Liu Y, Cai SJ, et al. IDH mutation in glioma: molecular mechanisms and potential therapeutic targets. Br J Cancer 2020;122:1580-9. [Crossref] [PubMed]
- Borodovsky A, Meeker AK, Kirkness EF, et al. A model of a patient-derived IDH1 mutant anaplastic astrocytoma with alternative lengthening of telomeres. J Neurooncol 2015;121:479-87. [Crossref] [PubMed]
- Ravi VM, Neidert N, Will P, et al. T-cell dysfunction in the glioblastoma microenvironment is mediated by myeloid cells releasing interleukin-10. Nat Commun 2022;13:925. [Crossref] [PubMed]
- Henrik Heiland D, Ravi VM, Behringer SP, et al. Tumor-associated reactive astrocytes aid the evolution of immunosuppressive environment in glioblastoma. Nat Commun 2019;10:2541. [Crossref] [PubMed]
- Akira S, Takeda K. Toll-like receptor signalling. Nat Rev Immunol 2004;4:499-511. [Crossref] [PubMed]
- Qian J, Wang C, Wang B, et al. The IFN-γ/PD-L1 axis between T cells and tumor microenvironment: hints for glioma anti-PD-1/PD-L1 therapy. J Neuroinflammation 2018;15:290. [Crossref] [PubMed]
- Liu Y, Zhou Z, Hou J, et al. Tumor Selective Metabolic Reprogramming as a Prospective PD-L1 Depression Strategy to Reactivate Immunotherapy. Adv Mater 2022;34:e2206121. [Crossref] [PubMed]
- Zampieri LX, Sboarina M, Cacace A, et al. Olaparib Is a Mitochondrial Complex I Inhibitor That Kills Temozolomide-Resistant Human Glioblastoma Cells. Int J Mol Sci 2021;22:11938. [Crossref] [PubMed]
- Zhou S, Zhao X, Yang Z, et al. Neddylation inhibition upregulates PD-L1 expression and enhances the efficacy of immune checkpoint blockade in glioblastoma. Int J Cancer 2019;145:763-74. [Crossref] [PubMed]
- von Manstein V, Groner B. Tumor cell resistance against targeted therapeutics: the density of cultured glioma tumor cells enhances Stat3 activity and offers protection against the tyrosine kinase inhibitor canertinib. Medchemcomm 2016;8:96-102. [Crossref] [PubMed]