Characteristics, clinical significance, and cancer immune interactions of lipid metabolism in prostate cancer
Highlight box
Key findings
• Lipid metabolism has a significant influence on immune response, immunotherapy, and medication guidance for patients with prostate cancer (PCa).
What is known and what is new?
• Lipid metabolism has a certain guiding significance for immunotherapy of PCa.
• This study collected the largest number of samples and groups, and comprehensively analyzed the impact of lipid metabolism on the immune response and immunotherapy of PCa.
What is the implication, and what should change now?
• A higher level of lipid metabolism may lead to reduced effectiveness of immunotherapy of PCa.
Introduction
Prostate cancer (PCa) is the most common cancer in terms of incidence and mortality among males in the urogenital system globally (1). For localized PCa, 10-year survival rate can be greater than 90% through radical prostatectomy or radiotherapy (2). Androgen deprivation therapy (ADT) is currently the primary approach for managing locally advanced and metastatic PCa (3,4). The majority of these patients will eventually progress to castration resistant PCa (CRPC) after undergoing ADT, and the combination of patient-specific immunotherapy and immune checkpoint inhibitors with conventional cytotoxic agents and therapies targeting the androgen receptor (AR) could be a viable option. Among them, the effectiveness of immunotherapy for CRPC has always been controversial in the past, however, as more mechanisms and targets are discovered, this approach has regained importance (5).
The exact causes of PCa remain unknown, but studies have suggested that individuals who are obese and on a high-fat diet have a higher risk (6). There was a positive correlation observed between the thickness of the prostate surrounding fat and the pathological staging and degree of invasion of PCa as revealed by prostate magnetic resonance imaging and transrectal prostate ultrasound examination (7). An elevated level of serum triglycerides was found to be linked with a higher likelihood of PCa recurrence (8). Studies have shown that PCa cells exhibit a higher rate of proliferation compared to normal cells, and have a greater demand for energy, with fat oxidation yielding significantly more adenosine triphosphate (ATP) than glucose (9,10). PCa cells have been found to rely more on fatty acid oxidation pathways for energy production, rather than glycolysis (11). In vitro study has demonstrated that upregulation of carnitine palmitoyltransferase 1A (CPT1A) in PCa cell lines LNCAP and C4-2 can enhance mitochondrial fatty acid oxidation and stimulate PCa cell growth (12). The transcriptomic and metabolomic data revealed a simultaneous increase in the expression of genes related to lipid metabolism and elevated levels of lipids in African-American PCa (13). These studies highlight the significance of lipid metabolism in the onset and progression of PCa.
Recent studies have found a close connection between lipid metabolism and the regulation of immune response (14-16). Additionally, lipid metabolism interventions have been discovered to hold promise as immunomodulatory agents and enhancers of anticancer immunotherapy (17). However, there has been no systematic study on the relationship between lipid metabolism, immune response, and immunotherapy in PCa. Here, we conducted a comprehensive analysis of the differences in lipid metabolism and associated genes between PCa tissues and normal tissues, as well as between PCa tissues and adjacent tissues. We analyzed the upstream transcription factors (TFs) and miRNAs that possibly target these associated genes, and then constructed the regulatory network of TF-gene-miRNA. Next, we assessed the correlations between these genes and immune cells, as well as immunotherapeutic effect. Furthermore, we obtained the score of lipid metabolism enrichment based on these genes, and analyzed the relationship with immune checkpoint, survival rate, recurrence rate, and drug sensitivity. This study is anticipated to make a valuable contribution towards the advancement of effective immunotherapies for PCa. We present this article in accordance with the TRIPOD reporting checklist (available at https://tcr.amegroups.com/article/view/10.21037/tcr-23-2140/rc).
Methods
Data acquisition
RNA sequencing (RNA-seq) data for PCa patients in The Cancer Genome Atlas (TCGA) and Genotype Tissue Expression (GTEx) were obtained from the UCSC Xena platform (https://xenabrowser.net/datapages/), which contains 496 PCa tissues, 52 adjacent non-tumor tissues and 100 normal tissues. And miRNA-seq data were downloaded from the TCGA data portal (https://tcga-data.nci.nih.gov/tcga/), which contains 496 PCa tissues and 52 adjacent non-tumor tissues. The RNA-seq data used in this study were publicly available and obtained in accordance with TCGA guidelines, therefore no additional ethical approval was required. The study was conducted in accordance with the Declaration of Helsinki (as revised in 2013).
Differentially expressed genes (DEGs) and enrichment analysis
In order to identify the DEGs, R software (v4.2.1) was used to compare the expression profiles of PCa tissues with those of normal tissues, and also to compare the expression profiles of PCa tissues with those of paracancerous tissues. Data analysis of DEGs was performed using package limma (v3.52.3) in R (18), with thresholds of fold change (FC) ≥2 and adjusted P value <0.05 [log2 FC ≥1 and adjusted P value <0.05]. In order to further understand the biological function of DEGs, both the up-regulated and down-regulated DEGs were input into a database for annotation, visualization, and integrated discovery with clusterProfiler (v3.16.1) package in R [20] for Gene Ontology (GO) analysis, which includes the enrichment of biological processes (BP), cellular components (CC), and molecular functions (MF), as well as for Kyoto Encyclopedia of Genes and Genomes (KEGG) pathway analysis enrichment. The ggplot2 (v3.3.5) and simplifyEnrichment (v1.6.1) package in R were used to create the visual GO and KEGG enrichment maps generated by the annotation analysis.
Validation of protein expressions of the up-regulated DEGs involved in lipid metabolism
The Human Protein Atlas (HPA, https://www.proteinatlas.org/) database was conducted to validate the protein expression of the up-regulated lipid metabolism related genes (LMRGs) between PCa tissues and normal prostate tissues via using immunohistochemistry (IHC) (19).
Regulatory network of TF-gene-miRNA
In order to comprehensively and accurately explore the regulatory relationship between up-regulated LMRGs in PCa tissues and the upstream TFs and miRNAs that may target these genes, the potential miRNAs were predicted by miRTarBase, starBase2, miRWalk, and miRNANet. The upstream regulatory TFs enriched in DEGs were analyzed using package RcisTarget (v1.8.0) in R. Then, we analyzed the Pearson’s correlation coefficient (r) of TF-target genes and miRNAs-target genes, and filtered those with absolute r value >0.1 to construct regulatory network of TF-gene-miRNA.
Gene set variation analysis (GSVA)
GSVA is a nonparametric and unsupervised method for evaluating enrichment of transcriptome gene set. Using these up-regulated LMRGs as a set of hallmark genes for high expression of lipid metabolism in PCa. Calculating GSVA enrichment scores for gene sets representing lipid metabolism expression and dividing the scores into high and low groups based on the median value. The hallmark gene sets were derived from gene expression profiles using the GSVA R package (version 1.36.3), which utilized the cluster-averaged log-transformed expression matrix.
Immune infiltration analysis
To analyze the correlation between the high and low GSVA enrichment scores and immune cells infiltration. The immune cells infiltration of TCGA PCa samples were download from the Tumor Immune Estimation Resource 2.0 (TIMER2.0) (http://timer.cistrome.org/) which analyzed using CIBERSORT. The correlations between the two groups and immune cells infiltration were analyzed using Hmisc R package (version 4.7.1).
Progression-free survival (PFS) and biochemical recurrence (BCR) analysis
To explore the correlation of the high and low GSVA enrichment scores and the survival and recurrence, we used survminer R package (v0.4.8) to perform PFS and BCR analysis.
Drug sensitivity analysis
To investigate the clinic performance of chemotherapy agents in patients, we computed the semi-inhibitory concentration (IC50) values of common drugs with package oncoPredict in R (20) based on Genomics of Drug Sensitivity in Cancer (GDSC, https://www.cancerrxgene.org/) database.
Statistical analysis
All statistical analyses were performed by R version 4.2.1 (https://www.r-project.org/). Student’s t-test and Wilcoxon rank-sum test were used in this study. Two-tailed P<0.05 was considered statistically significant. Data visualization was performed using the R package ggplot2 (v3.3.5). The heatmap was then plotted by the “pheatmap” package (v1.0.12) in R for visualization. Kaplan-Meier survival curves were created in each group, and log-rank tests were performed to compare the PFS and BCR between different groups. For correlation coefficients calculation, Pearson’s correlation coefficient analyses were performed.
Results
PCa and paracancerous tissues exhibit genetic and functional changes compared to normal tissues
To uncover the characteristic changes of PCa and paracancerous tissues relative to normal prostate tissues, genes with significant differences between PCa and normal tissues but not significant differences between PCa and paratumor tissues were filtered from 496 PCa samples, 52 paracancerous tissues and 100 normal samples from the UCSC Xena platform. A total of 5,533 DEGs (3,003 down-regulated, 2,530 up-regulated) were identified according to the fold change ≥2 and adjusted P value <0.05 cutoff criteria (Figure 1A). DEGs were then categorized to GO terms and KEGG pathways to discover biological functional changes in PCa and paracancerous tissues. The heatmap of GO enrichment results showed that phagocytosis, immunoglobulin, immunity, lipid, glycolipid, and homeostasis were highly enriched (Figure 1B). The top 20 most significant BP of GO terms showed that DEGs were enriched in phagocytosis, extracellular matrix organization, immune response, and lipid catabolic process (Figure 1C). The top 20 most significant KEGG pathways showed that protein digestion and absorption, inflammatory mediator regulation of TRP channels, and lipid metabolism related pathways (fatty acid metabolism, glycerophospholipid metabolism, steroid biosynthesis, fatty acid biosynthesis, glycerolipid metabolism) were highly enriched (Figure 1D). These results indicate that genes and biological functions, especially immune response and lipid metabolism have undergone significant changes in PCa and paracancerous tissues compared to normal tissues.
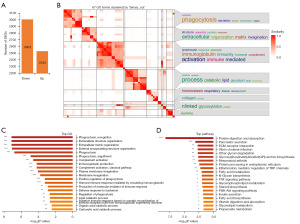
Lipid metabolism related biological functional and DEGs in PCa
Afterwards, we performed an DEGs analysis of PCa versus normal tissues and PCa versus paracancerous tissues, and obtained a total of 1,389 common DEGs (Figure 2A). GO enrichment analysis was performed according to the common DEGs. Up-regulated GO terms showed that lipid metabolism related BP (phospholipid catabolic, low-density lipoprotein particle remodeling, lipid catabolic process) were highly enriched (Figure 2B). Next, the up-regulated lipid metabolism related BP and genes were listed in detail (Figure 2C,2D). A total of 21 genes, including phospholipase A2 group IIA (PLA2G2A), phospholipase A1 member A (PLA1A), phospholipase A2 group VII (PLA2G7), arachidonate 15-lipoxygenase (ALOX15), twist family bHLH transcription factor 1 (TWIST1), angiopoietin like 3 (ANGPTL3), phospholipase A2 group IID (PLA2G2D), apolipoprotein C2 (APOC2), apolipoprotein C1 (APOC1), apolipoprotein E (APOE), glycerophosphodiester phosphodiesterase domain containing 1 (GDPD1), ELOVL fatty acid elongase 2 (ELOVL2), cytochrome P450 family 2 subfamily J member 2 (CYP2J2), nudix hydrolase 8 (NUDT8), TLC domain containing 1 (TLCD1), tribbles pseudokinase 3 (TRIB3), fatty acid synthase (FASN), acyl-CoA synthetase medium chain family member 1 (ACSM1), solute carrier family 27 member 2 (SLC27A2), sphingomyelin phosphodiesterase acid like 3B (SMPDL3B), and alpha-methylacyl-CoA racemase (AMACR) were differentially up-regulated in PCa, indicating that these DEGs might be critical for elevated lipid metabolism in PCa. Then, the volcano plots were visualized to show the expression pattern of the up-regulated DEGs involved in lipid metabolism between PCa and normal tissues (Figure 2E), as well as PCa and paracancerous tissues (Figure 2F).
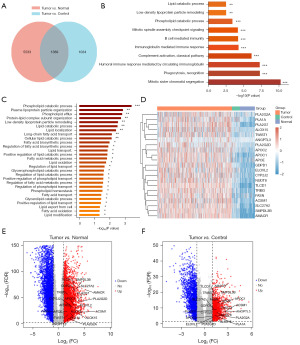
The protein expression levels of the up-regulated DEGs involved in lipid metabolism in PCa
To determine the differentially protein expression of the up-regulated DEGs involved in lipid metabolism in PCa, representative images for IHC of the up-regulated LMRGs in PCa and normal prostate tissues were obtained from the HPA database. The results showed that the protein expression levels of NUDT8, FASN, ACSM1, and AMACR were higher in PCa tissues than that in normal prostate tissues (Figure 3). These outcomes were in consistent with the RNA-seq results.
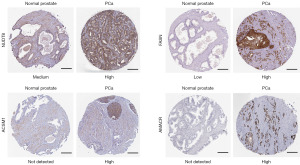
Construction of TF-gene-miRNA regulatory network
To explore the possible regulatory mechanism of these up-regulated LMRGs in PCa, we analyzed the upstream TFs and miRNAs that possibly target the DEGs, and then constructed the regulatory network of TF-gene-miRNA. The network showed that PLA2G7, TWIST1, and TRIB3 may be the key genes that elevated lipid metabolism in PCa. And the up-regulated TFs, including NK2 homeobox 2 (NKX2-2), NFE2 like bZIP transcription factor 3 (NFE2L3), as well as the down-regulated TFs, including GATA binding protein 5 (GATA5), MDS1 and EVI1 complex locus (MECOM), GATA binding protein 3 (GATA3), and retinoic acid receptor gamma (RARG) that regulated the three key genes, may play an important role in the regulation of lipid metabolism. Also, the predicted miRNAs (miR-205-5p, miR-1258, miR-204-5p) target TRIB3 were down-regulated, indicating that these miRNAs might be critical for lipid metabolism in PCa (Figure 4).
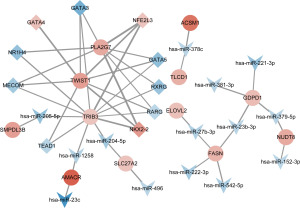
Correlations between up-regulated LMRGs and immunity
Lipid metabolism is closely related to immune responses in PCa (21). In view of this, we analyzed the correlations between up-regulated LMRGs and immune cells. As depicted in Figure 5A, the up-regulated LMRGs were positively associated with the infiltration of macrophage M0, macrophage M1, myeloid dendritic cell resting, and T cell regulatory (Tregs), while the opposite performance was noted in relationship with the up-regulated LMRGs and B cell plasma, T cell CD8+, and myeloid dendritic cell activated. We then divided the sample into high and low expression groups using the median of a single gene and analyzed the relationship between these two groups and immune cell. The results showed that the higher the expression of PLA2G7, ALOX15, PLA2G2D, and APOC1, the stronger the positive correlation with macrophage M1, myeloid dendritic cell resting, T cell regulatory (Tregs), and macrophage M2, respectively (Figure 5B). To explore whether these LMRGs are related to immunotherapy, the correlations between these genes and the biomarkers for immunotherapy were displayed in Figure 5C. It was demonstrated that most genes were negatively correlated with the expression of immunotherapy biomarkers, except for PLA2G2D, APOC1, APOC2, and APOE.
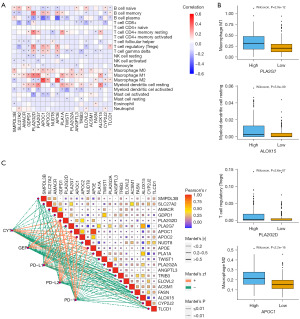
Association of the enrichment of lipid metabolism with immune cells and immune checkpoints
Subsequently, LMRGs were used as a marker gene set for high expression of lipid metabolism in PCa. And we calculated the GSVA enrichment score, which represents the score of lipid metabolism enrichment. Then we divided the sample into high and low groups based on the median score. The correlations between the two groups and immune cells infiltration were analyzed using Hmisc R package. The results showed that the high group had more infiltration of B cell memory, macrophage M0, macrophage M1, and myeloid dendritic cell resting, and the low group had more infiltration of B cell plasma, monocyte, myeloid dendritic cell activated, and mast cell resting (Figure 6A). Additionally, we assessed the relationship between the two groups and immune checkpoints. Figure 6B demonstrates that most checkpoint genes are highly expressed in the low group, such as CD274, ICOSLG, PDCD1LG2, TMIGD2, VTCN1, CD40, TNFRSF4, ENTPD1, LAG3, NT5E. Then, the gene expression levels of these checkpoints in different groups were displayed in a heatmap (Figure 6C).
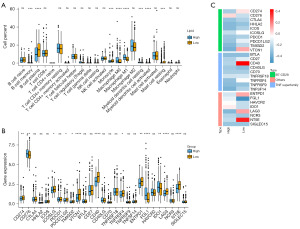
The impact of the enrichment of lipid metabolism on survival and recurrence
To explore the impact of the enrichment of lipid metabolism on survival and recurrence in PCa patients, we conducted PFS and BCR analysis across different groups. There was no significant difference in PFS and BCR between the high and low group (Figure 7A,7B).
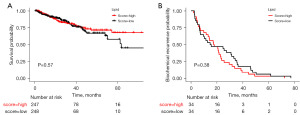
Drug sensitivity analysis
Drug therapy, such as chemotherapy, targeted therapy, and immunotherapy may limit PCa progression and improve patients’ prognoses (22). To identify the efficacy of the score of lipid metabolism enrichment as a biomarker to predict therapeutic response in PCa, we estimated the IC50 values of 160 drugs from GDSC dataset. We discovered that patients with high score of lipid metabolism enrichment may positively react to mitoxantrone, alisertib, topotecan, nelarabine, and foretinib, while patients with low score of lipid metabolism enrichment positively react to docetaxel, sorafenib, oxaliplatin, acetalax, and dabrafenib (Figure 8A-8J). These findings indicated that lipid metabolism was correlated with drug sensitivity.
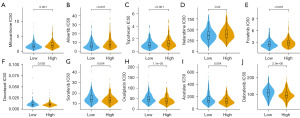
Discussion
In this study, we analyzed the TCGA and GTEx cohorts to determine the changes in transcription and expression of lipid metabolism and LMRGs, and further identified the relationship with immune infiltration, survival rate, recurrence rate and immunotherapy.
Emerging studies contributed to the discovery of lipid metabolism disorder in PCa. For instance, Li et al. collected PCa and adjacent nontumor (ANT) tissues and performed lipidomics and transcriptomics. They discovered significant disruptions in pathways related to the synthesis of lipids, the uptake of lipids, and the remodeling of phospholipids, which were associated with extensive lipid accumulation and alterations in lipid composition in PCa (23). The metabolism of cholesterol and lipids is significantly disrupted in PCa, with notable imbalances in the pathways responsible for fatty acid synthesis and oxidation (24). However, systematic analysis of PCa, adjacent and normal tissues remain largely undetermined, especially, the changes that occur in the adjacent tissues compared to normal tissues are not yet clearly explained.
Here, on the UCSC Xena platform, we identified 5,533 genes that exhibited significant differences between PCa and normal tissues, but not between PCa and paratumor tissues. These DEGs were found to be highly enriched in immune response and lipid metabolism, suggesting that significant alterations have occurred in these pathways in paracancerous tissues. Keeping track of these DEGs or related metabolic indicators can be highly important in terms of preventing and detecting issues at an early stage. Further analysis showed that the expression level of LMRGs, including PLA2G7, AMACR, TLCD1 and SLC27A2, gradually increased in normal, paracancerous, and PCa tissues. Consistently, a previous study has shown that PLA2G7 is associated with aggressive PCa and promotes PCa cell migration and invasion (25). However, the mechanism by which PLA2G7 regulates these phenotypes is still unclear. Our analysis revealed that TFs NKX2-2 and NFE2L3 may regulate PLA2G7 in PCa. Unfortunately, there were no further experimental data to verify it. Similarly, the expression level of AMACR was coincident with previous research (26,27).
Cao et al. provided evidence of increased FASN expression in PCa tissues, which correlated with higher Gleason scores and clinical stages. Furthermore, FASN emerged as an independent prognostic biomarker for shorter BCR-free survival, as revealed by both univariate and multivariate analysis (P<0.05) (28). Additionally, it was demonstrated that the activity of FASN promotes cell motility driven by hepatocyte growth factor (HGF) in PCa, thereby enhancing the migration of cancer cells (29). In this study, FASN was also found to be upregulated differentially in PCa. These findings suggested that FASN may serve as a potential biomarker for predicting the prognosis of PCa.
It was worth noting that, NUDT8, a novel CoA-degrading enzyme that localizes to the mitochondria (30), was identified to be LMRG and significantly up-regulated in PCa in our study. CoA played a crucial role in various metabolic pathways such as the tricarboxylic acid cycle, fatty acid β-oxidation, lipogenesis, acetylcholine synthesis, and ketone synthesis and oxidation (31,32). NUDT8 was more likely to downregulate lipid metabolism based on these results. In actuality, it is the reverse. This could be attributed to the fact that NUDT8 participates in the lipid metabolism of PCa through various pathways.
Lipid metabolism plays an important role in controlling the immune system in the tumor microenvironment (33). The reprogramming of lipid metabolism can have a significant impact on the fate and function of immune cells. Altered lipid metabolism may have an impact on the immune function of natural killer T cells (NKT) that is both dependent and non-dependent on NKT cells (34). Specific lipid mediators play a critical role in determining the M1 and M2 phenotypes of macrophages (35). In Zhang’s study, a six-gene signature related to lipid metabolism in PCa was developed to accurately predict prognosis and indicate the immune microenvironment status. They confirmed that patients in the high-risk subgroup had more infiltration of T cell CD4+ memory resting, Tregs, macrophage M0, and macrophage M1, while patients in the low-risk subgroup had more infiltration of monocyte and mast resting cell (36). Our finding was consistent with it. Additionally, we also demonstrated that the group with higher lipid metabolism showed a downtrend in the expression of immune checkpoint genes. This indicated that a higher level of lipid metabolism may lead to reduced effectiveness of immunotherapy. Finally, we also analyzed the correlation between lipid metabolism and drug sensitivity. Our data suggested that lipid metabolism can serve as a clinical guide for medication in PCa treatment, as patients with low lipid metabolism enrichment scores have shown a favorable response to docetaxel, which has traditionally been the primary chemotherapy drug used (37). These findings contribute to the identification of novel approaches for targeted therapy in PCa.
Lucarelli et al. reported novel genomic drivers of the lipid metabolism in PCa: PDHA1 and PDP1, which frequently exhibit amplification at both the gene and protein levels in primary PCa, particularly in high-grade tumors (38). Our analysis also revealed a significant upregulation of PDP1 in PCa, while no significant difference was observed in PDHA1 expression (the supplementary tables are available at https://cdn.amegroups.cn/static/public/tcr-23-2140-1.xlsx; https://cdn.amegroups.cn/static/public/tcr-23-2140-2.xlsx). This discrepancy may be attributed to the lack of tumor grading information for the PCa patients in our dataset, potentially introducing a statistical bias in gene expression levels.
There are several limitations to this study, including the retrospective nature of the data obtained from public databases, which may be subject to inherent selection bias. Additionally, the inclusion of additional clinical variables is necessary to fully explore the clinical significance of the lipid metabolism enrichment score. Furthermore, further prospective studies and in vivo and in vitro experimental studies are needed to validate our findings.
Conclusions
In conclusion, our thorough examination of lipid metabolism and associated genes reveals a comprehensive regulatory mechanism that has a significant impact on immune response, immunotherapy, and medication guidance for patients with PCa. This study highlights the crucial clinical relevance of lipid metabolism and provides a valuable foundation for future investigations into personalized therapy for PCa patients.
Acknowledgments
Funding: This work was supported by
Footnote
Reporting Checklist: The authors have completed the TRIPOD reporting checklist. Available at https://tcr.amegroups.com/article/view/10.21037/tcr-23-2140/rc
Peer Review File: Available at https://tcr.amegroups.com/article/view/10.21037/tcr-23-2140/prf
Conflicts of Interest: All authors have completed the ICMJE uniform disclosure form (available at https://tcr.amegroups.com/article/view/10.21037/tcr-23-2140/coif). The authors have no conflicts of interest to declare.
Ethical Statement: The authors are accountable for all aspects of the work, in including ensuring that issues related to the accuracy or integrity of any part of the work have been appropriately investigated and resolved. The study was conducted in accordance with the Declaration of Helsinki (as revised in 2013).
Open Access Statement: This is an Open Access article distributed in accordance with the Creative Commons Attribution-NonCommercial-NoDerivs 4.0 International License (CC BY-NC-ND 4.0), which permits the non-commercial replication and distribution of the article with the strict proviso that no changes or edits are made and the original work is properly cited (including links to both the formal publication through the relevant DOI and the license). See: https://creativecommons.org/licenses/by-nc-nd/4.0/.
References
- Bray F, Laversanne M, Sung H, et al. Global cancer statistics 2022: GLOBOCAN estimates of incidence and mortality worldwide for 36 cancers in 185 countries. CA Cancer J Clin 2024;74:229-63. [Crossref] [PubMed]
- Fidelito G, Watt MJ, Taylor RA. Personalized Medicine for Prostate Cancer: Is Targeting Metabolism a Reality? Front Oncol 2022;11:778761. [Crossref] [PubMed]
- Cui H, Hou C, Ma Q, et al. Effect and Mechanism of lncRNA-PCMF1/hsa-miR-137/Twist1 Axis Involved in the EMT Regulation of Prostate Cancer Cells. Mol Biotechnol 2023;65:1991-2003. [Crossref] [PubMed]
- DE Nunzio C. Androgen deprivation therapy and cardiovascular risk in prostate cancer. Minerva Urol Nephrol 2022;74:508-17. [PubMed]
- Cha HR, Lee JH, Ponnazhagan S. Revisiting Immunotherapy: A Focus on Prostate Cancer. Cancer Res 2020;80:1615-23. [Crossref] [PubMed]
- Li D, Zhou X, Xu W, et al. High-fat diet promotes prostate cancer metastasis via RPS27. Cancer Metab 2024;12:6. [Crossref] [PubMed]
- Bhindi B, Trottier G, Elharram M, et al. Measurement of peri-prostatic fat thickness using transrectal ultrasonography (TRUS): a new risk factor for prostate cancer. BJU Int 2012;110:980-6. [Crossref] [PubMed]
- Allott EH, Howard LE, Cooperberg MR, et al. Serum lipid profile and risk of prostate cancer recurrence: Results from the SEARCH database. Cancer Epidemiol Biomarkers Prev 2014;23:2349-56. [Crossref] [PubMed]
- Kuhajda FP. Fatty acid synthase and cancer: new application of an old pathway. Cancer Res 2006;66:5977-80. [Crossref] [PubMed]
- Morote J, Gómez-Caamaño A, Alvarez-Ossorio JL, et al. The metabolic syndrome and its components in patients with prostate cancer on androgen deprivation therapy. J Urol 2015;193:1963-9. [Crossref] [PubMed]
- Watt MJ, Clark AK, Selth LA, et al. Suppressing fatty acid uptake has therapeutic effects in preclinical models of prostate cancer. Sci Transl Med 2019;11:eaau5758. [Crossref] [PubMed]
- Joshi M, Kim J, D'Alessandro A, et al. CPT1A Over-Expression Increases Reactive Oxygen Species in the Mitochondria and Promotes Antioxidant Defenses in Prostate Cancer. Cancers (Basel) 2020;12:3431. [Crossref] [PubMed]
- Berchuck JE, Adib E, Abou Alaiwi S, et al. The Prostate Cancer Androgen Receptor Cistrome in African American Men Associates with Upregulation of Lipid Metabolism and Immune Response. Cancer Res 2022;82:2848-59. [Crossref] [PubMed]
- De Angulo A, Travis P, Galvan GC, et al. Obesity-Modified CD4+ T-Cells Promote an Epithelial-Mesenchymal Transition Phenotype in Prostate Cancer Cells. Nutr Cancer 2022;74:650-9. [Crossref] [PubMed]
- El-Kenawi A, Dominguez-Viqueira W, Liu M, et al. Macrophage-Derived Cholesterol Contributes to Therapeutic Resistance in Prostate Cancer. Cancer Res 2021;81:5477-90. [Crossref] [PubMed]
- Masetti M, Carriero R, Portale F, et al. Lipid-loaded tumor-associated macrophages sustain tumor growth and invasiveness in prostate cancer. J Exp Med 2022;219:e20210564. [Crossref] [PubMed]
- Bleve A, Durante B, Sica A, et al. Lipid Metabolism and Cancer Immunotherapy: Immunosuppressive Myeloid Cells at the Crossroad. Int J Mol Sci 2020;21:5845. [Crossref] [PubMed]
- Ritchie ME, Phipson B, Wu D, et al. limma powers differential expression analyses for RNA-sequencing and microarray studies. Nucleic Acids Res 2015;43:e47. [Crossref] [PubMed]
- Sjöstedt E, Zhong W, Fagerberg L, et al. An atlas of the protein-coding genes in the human, pig, and mouse brain. Science 2020;367:eaay5947. [Crossref] [PubMed]
- Maeser D, Gruener RF, Huang RS. oncoPredict: an R package for predicting in vivo or cancer patient drug response and biomarkers from cell line screening data. Brief Bioinform 2021;22:bbab260. [Crossref] [PubMed]
- Siltari A, Syvälä H, Lou YR, et al. Role of Lipids and Lipid Metabolism in Prostate Cancer Progression and the Tumor's Immune Environment. Cancers (Basel) 2022;14:4293. [Crossref] [PubMed]
- Nevedomskaya E, Baumgart SJ, Haendler B. Recent Advances in Prostate Cancer Treatment and Drug Discovery. Int J Mol Sci 2018;19:1359. [Crossref] [PubMed]
- Li J, Ren S, Piao HL, et al. Integration of lipidomics and transcriptomics unravels aberrant lipid metabolism and defines cholesteryl oleate as potential biomarker of prostate cancer. Sci Rep 2016;6:20984. [Crossref] [PubMed]
- Blomme A, Ford CA, Mui E, et al. 2,4-dienoyl-CoA reductase regulates lipid homeostasis in treatment-resistant prostate cancer. Nat Commun 2020;11:2508. [Crossref] [PubMed]
- Vainio P, Lehtinen L, Mirtti T, et al. Phospholipase PLA2G7, associated with aggressive prostate cancer, promotes prostate cancer cell migration and invasion and is inhibited by statins. Oncotarget 2011;2:1176-90. [Crossref] [PubMed]
- Kong G, Lee H, Tran Q, et al. Current Knowledge on the Function of α-Methyl Acyl-CoA Racemase in Human Diseases. Front Mol Biosci 2020;7:153. Erratum in: Front Mol Biosci 2021;8:639164. [Crossref] [PubMed]
- Woodman TJ, Lloyd MD. Analysis of enzyme reactions using NMR techniques: A case study with α-methylacyl-CoA racemase (AMACR). Methods Enzymol 2023;690:159-209. [Crossref] [PubMed]
- Cao Z, Xu Y, Guo F, et al. FASN Protein Overexpression Indicates Poor Biochemical Recurrence-Free Survival in Prostate Cancer. Dis Markers 2020;2020:3904947. [Crossref] [PubMed]
- De Piano M, Manuelli V, Zadra G, et al. Exploring a role for fatty acid synthase in prostate cancer cell migration. Small GTPases 2021;12:265-72. [Crossref] [PubMed]
- Kerr EW. Characterization of the Biochemical Properties of Nudt8, a Novel CoA-degrading Enzyme that Localizes to the Mitochondria. Graduate Theses, Dissertations, and Problem Reports 2020;7831.
- Choudhary C, Weinert BT, Nishida Y, et al. The growing landscape of lysine acetylation links metabolism and cell signalling. Nat Rev Mol Cell Biol 2014;15:536-50. [Crossref] [PubMed]
- Zhang Y, Bharathi SS, Rardin MJ, et al. Lysine desuccinylase SIRT5 binds to cardiolipin and regulates the electron transport chain. J Biol Chem 2017;292:10239-49. [Crossref] [PubMed]
- di Meo NA, Lasorsa F, Rutigliano M, et al. The dark side of lipid metabolism in prostate and renal carcinoma: novel insights into molecular diagnostic and biomarker discovery. Expert Rev Mol Diagn 2023;23:297-313. [Crossref] [PubMed]
- Fu S, He K, Tian C, et al. Impaired lipid biosynthesis hinders anti-tumor efficacy of intratumoral iNKT cells. Nat Commun 2020;11:438. [Crossref] [PubMed]
- Wang S, Liu R, Yu Q, et al. Metabolic reprogramming of macrophages during infections and cancer. Cancer Lett 2019;452:14-22. [Crossref] [PubMed]
- Zhang Y, Kong X, Xin S, et al. Discovery of Lipid Metabolism-Related Genes for Predicting Tumor Immune Microenvironment Status and Prognosis in Prostate Cancer. J Oncol 2022;2022:8227806. [Crossref] [PubMed]
- Nader R, El Amm J, Aragon-Ching JB. Role of chemotherapy in prostate cancer. Asian J Androl 2018;20:221-9. [Crossref] [PubMed]
- Lucarelli G, Loizzo D, Ferro M, et al. Metabolomic profiling for the identification of novel diagnostic markers and therapeutic targets in prostate cancer: an update. Expert Rev Mol Diagn 2019;19:377-87. [Crossref] [PubMed]