Integrating network pharmacology and computational biology to propose Yiqi Sanjie formula’s mechanisms in treating NSCLC: molecular docking, ADMET, and molecular dynamics simulation
Highlight box
Key findings
• The study successfully identified three stable drug-target combinations (CCND1-bifendate, CDK4-xambioona, and EGFR-hederagenin) through molecular docking, dynamic simulation, and absorption, distribution, metabolism, excretion, and toxicity analysis, highlighting potential anti-non-small cell lung cancer (NSCLC) effects of the Yiqi Sanjie formula.
• Molecular dynamic simulation with site-directed mutagenesis further explored the structure-activity relationship between Bifendate and key residue sites, providing new insights into NSCLC targeted therapy.
What is known and what is new?
• The therapeutic efficacy of traditional Chinese medicine (TCM), specifically Yiqi Sanjie formula, in alleviating NSCLC symptoms is recognized, yet its molecular mechanisms of action were not fully understood.
• This manuscript adds comprehensive computational insights into the potential mechanisms of Yiqi Sanjie formula in NSCLC treatment, identifying specific drug-target interactions and proposing new avenues for targeted therapy development.
What is the implication, and what should change now?
• The findings suggest a significant step forward in integrating TCM with modern computational biology approaches to unveil novel therapeutic targets and strategies for NSCLC treatment.
• These insights advocate for a shift towards more personalized and mechanistic-based approaches in the development of TCM therapies for cancer, emphasizing the need for further experimental validation and clinical trials to confirm these computational predictions and their therapeutic potential.
Introduction
In recent years, primary lung cancer has become a leading cause of cancer-related deaths worldwide (1), with high incidence and mortality rates. Statistics show that approximately 350 people die from lung cancer each day, which is nearly 2.5 times the number of deaths from the second leading cause of cancer-related deaths (colorectal cancer) (2). Lung cancer can be classified into two main types based on histopathology: small cell lung cancer (SCLC) and non-small cell lung cancer (NSCLC). NSCLC is the most common type of lung cancer in clinical practice, accounting for approximately 82% of all lung cancer cases (3). Patients with NSCLC have a poor prognosis, with a 5-year survival rate of only 26% (4). Although Western medicine has shown promising results in the treatment of lung cancer, it also incurs problems that undermine the benefits of treatment. These problems include drug toxicities and side effects, drug resistance, and tumor recurrence (5), which not only significantly weaken the effectiveness of anti-tumor therapies but also lead to a drastic decrease in patients’ quality of life. Therefore, the combination of modern and traditional medicine has become the best choice and an inevitable trend for the future.
Yiqi Sanjie formula is widely used in the clinical practice including the treatment of lung neoplasm (6). This formulation is regularly used in the Traditional Chinese Medicine (TCM) department of Shanxi Provincial Cancer Hospital. Its combined use with Changchun Ruibin and platinum has been widely recognized for its therapeutic effect (7). However, the molecular mechanisms of its action against NSCLC are not yet fully understood.
TCM network pharmacology (8) evaluates therapeutic potential of Chinese herbal compounds by screening for the potentially affected disease-related genes, predicting their targets and potential pharmacological effects and revealing the potential association between drug-gene-disease. Previous study has shown that TCM has the characteristics of “multiple components, multiple targets, and multiple pathways” (9). Computational methods are often used to propose the interplay between TCM compounds and human disease genes by prediction their mechanism of action (10-12). In this study, we utilized network pharmacology to identify potential targets of the active compounds in Yiqi Sanjie formula. Furthermore, in silico methods such as molecular docking, molecular dynamics (MD) simulations, absorption, distribution, metabolism, excretion, toxicity (ADMET) and chemical frequent hitter (ChemFH) predictive tools were employed to identify key compounds with the therapeutic potential. We present this article in accordance with the MDAR reporting checklist (available at https://tcr.amegroups.com/article/view/10.21037/tcr-24-972/rc).
Methods
Compounds retrieval and genes collection
The list of active ingredients present in the Yiqi Sanjie formula was systematically identified using the Traditional Chinese Medicine Systems Pharmacology (TCMSP) database (13). This database employs a hybrid approach, combining quantitative computational predictions with qualitative historical data on herbal components, to screen for compounds with significant pharmacological potential. For this study, compounds, listed in Supplementary l, were filtered based on a quantitative threshold for oral bioavailability (OB) ≥30% and drug-likeness (DL) ≥0.18, ensuring that only bioactive constituents with substantial potential for effective drug development were considered (14).
The GeneCards (https://www.genecards.org/) (15) and Online Mendelian Inheritance in Man (OMIM; https://omim.org/) (16) databases were searched with a relevance score of at least 20 to identify potential targets for NSCLC. The genes of targets associated with Yiqi Sanjie formula found in TCSMP and genes related to NSCLC as indicated in GeneCards were analyzed to identify overlapping targets between bioactive constituents and diseases, which allowed the prediction of the pharmacological effects of Yiqi Sanjie formula on NSCLC. Venn diagrams of the intersection were created using the network analysis software Venny 2.1.0 (https://bioinfogp.cnb.csic.es/tools/venny/), and the number of overlapping genes was computed automatically. The study was conducted in accordance with the Declaration of Helsinki (as revised in 2013).
Protein-protein interaction (PPI) network analysis
Potential target genes were imported into the Search Tool for the Retrieval of Interaction Genes/Proteins (STRING) database (https://cn.string-db.org/) (17) to construct a PPI network, which was analyzed using Cytoscape 3.7.2 (18) to calculate the degree values of the nodes. The node size and color were set according to their degree values, whereas the edge thickness and color were set based on the combined score. The CytoNCA plugin (19) was used to calculate betweenness centrality (BC) and adjust the network layout according to its value.
Gene ontology (GO) enrichment analysis
To establish a logical framework for gene functions and annotations (20), GO enrichment analysis was employed in this study. The Database for Annotation, Visualization, and Integrated Discovery (DAVID) database (https://david.ncifcrf.gov/) (21) was utilized with the “Homo sapiens” setting for the organism and a significance threshold of P<0.05 (22). Changes in genetic functions were observed in terms of molecular function (MF) and biological processes (BP), which respectively refer to genes that regulate molecular activity and biological procedures achieved through genetic programs (23). Additionally, the potential of Yiqi Sanjie formula for affecting the genetic function related to treating NSCLC was demonstrated by calculating the number of genes involved in each GO function.
Kyoto Encyclopedia of Genes and Genomes (KEGG) pathway enrichment analysis
The KEGG analysis could provide information on pathways of NSCLC and the pharmacological effects of Yiqi Sanjie formula. DAVID database (https://david.ncifcrf.gov/) was also used for KEGG enrichment (24) analysis and to merge KEGG pathways to construct a functional and efficient pathway network (25). The enrichment was performed in the organism setting to “Homo sapiens”, with a threshold value of P<0.05.
Molecular docking
Key protein targets critical to NSCLC pathogenesis, such as CCND1, CDK4, and EGFR, were selected for molecular docking based on their roles in cell cycle regulation and growth factor signaling. These targets were identified through integrated network pharmacology analyses, utilizing STRING for PPIs, KEGG for pathway insights, and GO for functional importance. The three-dimensional structures of proteins and corresponding natural compounds were sourced from UniProt (https://www.uniprot.org/) (26) and Pubchem (https://pubchem.ncbi.nlm.nih.gov/) (27), respectively. AutoDock Tools 1.5.2 (28). facilitated structure processing, which included the removal of natural ligands from the protein structures to prepare them for docking with the identified compounds from the Yiqi Sanjie formula. The GetBox Plugin.py (https://github.com/MengwuXiao/GetBox-PyMOLPlugin/blob/master/GetBox%20Plugin.py) calculated docking box parameters, ensuring targeted and efficient docking simulations. Docking was executed with AutoDock Vina 1.2.3 (29) using default settings (30), and the results were quantitatively evaluated and visually represented in a heat map. The most promising docking poses were visualized using PyMol 2.5.5 (https://pymol.org/2/) (31) and PLIP (32). Detailed information about key targets, PDB IDs, and docking box parameters can be found in Supplementary 2, providing a comprehensive resource for further verification and replication of the docking simulations. This systematic approach underscores a focused exploration of potential therapeutic interventions targeting crucial molecular interactions in NSCLC.
MD simulations
MD simulations were conducted using Desmond 2022-4 (https://www.deshawresearch.com, academic license) (33) to investigate protein-ligand interactions. Protein preparation wizard tool was used to prepare proteins for simulation by adding hydrogen atoms and setting protonation states of ionizable groups. The molecular systems for simulations were constructed using the System Builder module by assigning OPLS4 force field (34) parameters to the protein and ligand. The protein-ligand complexes was solvated into a cubic box with the size 10 Å larger that the protein-ligand complexes in all directions. The appropriate number of water molecules as well as Na+ and Cl− ions were added to neutralize the charges of systems. The water molecules were represented using TIP3P model. The simulation protocol included energy minimization, NVT simulation (constant temperature, constant volume) followed by NPT (constant temperature, constant pressure) simulation to equilibrate system, each for 50,000 time steps of 1 ps. A 500 ns production run MD simulation was then performed for each complex at 300 K and 1.0 atm, with energy and coordinate saving every 50 ps. The resulting trajectories were analysed using Maestro software (Schrödinger Release 2024-2: Maestro, Schrödinger, LLC, New York, NY, 2024).
ADME property assessment and lead filtering
Pharmacokinetic properties of compounds were evaluated using SwissADME (35) and ADMETlab 2.0 (36), which assessed absorption, distribution, metabolism, and excretion (ADME). The synthetic accessibility of compounds was determined using a graph attention-based assessment (GASA) tool (37). To filter out aggregators from potential lead molecules, ChemAGG (38) was utilized. In addition, ChemFluo (39) was used to filter out compounds that are known to interfere assays, including blue/green fluorescent compounds, aggregates, Fluc inhibitors, chaotic compounds, chemoreactive compounds, and other detection interference compounds.
Results
Identification of potential Yiqi Sanjie formula targets in NSCLC
The identification and analysis of potential therapeutic targets is a crucial step in drug discovery (40). In this study, the detailed analyses revealed 225 potential targets of the Yiqi Sanjie formula 225 and 495 NSCLC-related targets with 25 common targets (Figure 1A), such as CCND1 (PDB ID: 2w96), CDK4 (PDB ID: 7sj3), EGFR (PDB ID: 1m14) and MET (PDB ID: 1r0p) (Figure 1B).
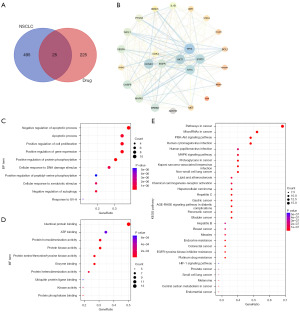
Interestingly, in the PPI network of 25 target genes, ADH1B was found isolated without edges that could indicate possible PPI with other targets in the set (Figure 1B). ADH1B encodes a protein belonging to the alcohol dehydrogenase family, which plays a crucial role in metabolizing various substrates including ethanol, retinol, and other alcohols, crucial for detoxifying lipid peroxides and aldehydes. This protein is composed of multiple isozymes formed by homologous α, β, and γ subunits, highly active in ethanol oxidation (41). Given the link between ethanol metabolism and increased risk of cancer development, ADH1B’s activity in ethanol catabolism may influence carcinogenic processes, potentially impacting NSCLC development (42). Recent findings underscore the utility of ADH1B expression levels as prognostic biomarkers in NSCLC, suggesting that higher expression correlates with more favorable outcomes. This correlation invites hypotheses that ADH1B’s metabolic roles might affect tumor microenvironment or influence tumor progression pathways in NSCLC (43). Further research is necessary to elucidate the specific mechanisms by which ADH1B impacts NSCLC pathology and to explore the potential of targeting ADH1B as a therapeutic approach. Such study could reveal novel strategies for improving NSCLC prognosis and treatment, affirming the importance of ADH1B not only in metabolic processes but also in cancer biology (44).
GO analysis of potential therapeutic targets in NSCLC
To gain insight into the potential biological functions of NSCLC-related targets, GO enrichment analysis and KEGG pathway analysis were performed. Results from the top ten items of BP and MF of GO analysis showed that the 25 overlapping targets were mainly involved in signal transduction, transcriptional regulation, and apoptosis, as shown in Figure 1C,1D.
Signal transduction, transcriptional regulation, and apoptosis play important roles in the development and progression of NSCLC (45). Aberrant signaling pathways can lead to abnormal proliferation, invasion, and metastasis of tumor cells (46), whereas alterations in transcription factors and their regulatory networks can result in aberrant gene expression (47). Apoptosis is a crucial self-protective mechanism that can eliminate abnormal cells (48). Unfortunately, the exact relationship between these three MFs and NSCLC is not fully established and requires further investigation. However, previous study has shown that PPIs and molecular structural changes significantly impact tumor cell proliferation, metastasis, and apoptosis (49). Further research could reveal the specific mechanisms by which these MFs contribute to NSCLC development, providing a new theoretical basis for the development of novel targeted therapies.
In KEGG analysis, as shown in Figure 1E, the top 20 pathways were ranked based on the P value, and the results revealed that the targets were mainly involved in the PI3K-Akt signaling pathway, PAPK signaling pathway, AGE-RAGE signaling pathway in diabetic complications, EGFR tyrosine kinase inhibitor resistance, and HIF-1 signaling pathway. These pathways are involved in critical cellular processes such as cell growth, survival, apoptosis, and metabolism, and their dysregulation can promote NSCLC pathogenesis. Targeting these pathways has been proposed as a promising strategy for the treatment of NSCLC. For example, inhibitors of the PI3K-Akt pathway have shown potential for use as anticancer agents in NSCLC treatment (50), whereas HIF-1 inhibitors have been shown to enhance the efficacy of chemotherapy and radiotherapy in NSCLC (51). Therefore, understanding the complex interplay between these pathways and NSCLC may provide new insights into the development of targeted therapies for this disease.
Molecular docking Yiqi Sanjie formula compounds against selected NSCLC target
Molecular docking plays a crucial role in the discovery process of potential drug candidates that have an affinity to drug targets. In this study, molecular docking was used to predict the potential binding affinities between Yiqi Sanjie formula’s active ingredients and 20 selected targets. To reduce computational costs while still enabling the convenient plotting of heatmaps, 20 targets were chosen instead of 25. This number strikes a balance between computational feasibility and sufficient coverage of relevant biological pathways. After excluding targets with single-segment α-helix structures, 18 potential targets were identified for further analysis. The top five targets for which ligands had the highest affinity were ADH1B, CCND1, CDK4, EGFR, and PTGS2, as shown in Figure 2A.
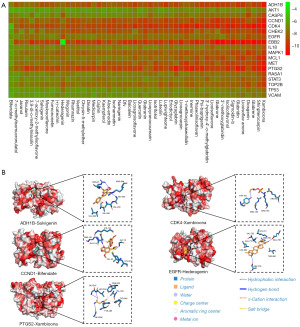
To better understand the interactions between the active ingredients and targets, the five complexes were visualized, as shown in Figure 2B and Table S1. It was found that hederagenin mainly interacted with EGFR through hydrophobic interactions, whereas xambioona interacted with CDK4 only through hydrogen bonds, forming a relatively simple set of intermolecular interactions. The remaining three complexes showed a more balanced interaction between the number of hydrogen bonds and hydrophobic interactions. Notably, a salt bridge was identified in the CCND1-bifendate complex, indicating potentially stronger binding stability.
MD simulation of top-scoring ligand-target complexes
Furthermore, MD simulations with the production run of 500 ns were performed for the most favorable complexes identified from the previous docking simulations, to assess the binding stability and duration. Stable and long-lasting binding would indicate the stability of the complexes and indirectly suggest the inhibitory potential of the small molecules (52). Root-mean-square deviation (RMSD) analysis, a critical tool for evaluating the stability of drug-target complex MD simulations, was performed on all five complexes. As shown in Figure 3, the ligand fit on protein RMSD of EGFR-hederagenin remained stable throughout the simulation, indicating a relatively stable binding between the two. However, ADH1B-Salvigenin, CCND1-bifendate, CDK4-xambioona, and PTGS2-xambioona showed varying degrees of fluctuations in their ligand fit on protein RMSD.
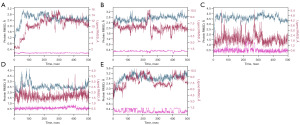
The RMSD analysis revealed that ADH1B-Salvigenin and PTGS2-xambioona displayed fluctuations beyond an acceptable range, indicating mobility of the ligand within the binding site and thus poor stability of these drug-target complexes. Moreover, as the KEGG analysis revealed ADH1B and PTGS2 were not involved in the relevant pathways studied, these two proteins were not further analyzed. However, CCND1-bifendate and CDK4-xambioona exhibited relatively stable protein-ligand complexes throughout the latter half of the simulation, as evidenced by smaller fluctuations in their RMSD and reaching stable plateaus.
As a new plateau was observed in the last 100 ns of the CCND1-bifendate simulation, the simulation was extended to 600 ns and there were no further significant changes in the RMSD (Figure S1). Additionally, stable hydrogen bonds were maintained between bifendate and CCND1 Ala-16 and Tyr-17 throughout the simulation, indicating that the conformational changes did not greatly affect the binding between them. The main difference between the 200–400 ns and 400–600 ns timeframes was the presence or absence of interactions with Lys-35 and Asp-158. As the simulation time was extended, the proportion of bifendate’s interactions with Lys-35 and Asp-158 gradually decreased to below 30% at the 400 ns time point. Thus, to improve the binding stability of bifendate derivatives to CCND1, future efforts could focus on enhancing interactions with these two residues. Further targeted mutations are detailed in Figure S2, and the results show that all four loci mutated to Gly have different degrees of effect on bifendate binding, with D158G and A16G having the most significant effect.
Overall, three Yiqi Sanjie components (bifendate, xambioona and hederagenin) in complex with their respective targets (CCND1, CDK4 and EGFR) were considered for further analysis.
Subsequently, MD simulations were performed on the three selected targets without ligands. Figure 4 shows the RMSD changes of the targets before and after ligand binding. The results indicated that the protein conformations were more stable after ligand binding than in the unbound state, as evidenced by the convergence of the RMSD changes to varying degrees. Therefore, it can be inferred that the three selected compounds enhanced the stability of the bound proteins to some extent.
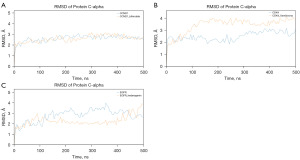
Residue mobility pre- and post-ligand delivery analysis
Assessing the dynamic behavior of ligand-target complexes was crucial to understanding how compounds interacted with their targets and enhanced stability. Therefore, we evaluated the root-mean-square fluctuation (RMSF) changes of the targets before and after ligand binding during MD simulations. Our findings, as shown in Figure 5, revealed that the compounds interacted differently with their respective targets and dynamically adjusted the RMSF of specific peptide segments.
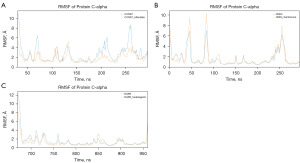
Bifendate binding to CCND1 upregulated the RMSF of the peptide segment 167–203, downregulated the RMSF of the peptide segments 98–123, and dynamically adjusted the RMSF of the peptide segments 9–65 and 215–238. Xambioona binding to CDK4 upregulated the RMSF of the peptide segments 78–85 and 278–281, downregulated the RMSF of the peptide segments 9–22 and 105–113, and dynamically adjusted the RMSF of the peptide segments 35–48 and 253–267 (partially upregulated, partially downregulated, and partially unmodified). Hederagenin binding to EGFR upregulated the RMSF of the regions 684–712, 728–738, and 756–764, and downregulated the RMSF of the region 848–862.
Based on the analysis presented earlier, it can be inferred that bifendate primarily targets the cyclin N-terminal of CCND1, although it remains unclear if it affects the key binding sites. Additional information, such as mutual interaction statistics, would be required for a more comprehensive analysis. Xambioona targets the protein kinase domain of CDK4, including binding sites 12–20 and Lys-35. However, the RMSF analysis did not reveal any impact on the active site Asp-140 of CDK4, suggesting a limited effect on its activity. Hederagenin targets the 688–704 region of EGFR, which is critical for dimerization, phosphorylation, and activation, as well as the protein kinase domain, including the active site Asp-837 and binding site Asp-855 (53).
Protein-ligands contact analysis of complexes
Estimation of protein interactions provides a measure of interaction power between the ligands and the target protein. These interactions can be categorized by type and summarized, as shown in the plot represented in Figure 6. In the case of CCND1-bifendate, as shown in Figure 6A,6B, the primary types of interactions were hydrogen bonds and water bridges, along with some hydrophobic interactions. Through the simulation trajectory, it was observed that bifendate maintained stable binding to the active pocket of CCND1 by forming hydrogen bonds with key residues, including Ala-16 and Tyr-17. These residues are known to be important constituents of the CCND1 pocket, which further confirms the specificity of bifendate in targeting CCND1. The 286–288 region (54-56) that produces activity or functional effects on CCND1 after mutation is located at the C-terminus, which is typically used for screening allosteric modulators. However, conventional inhibitors should still be screened based on the conventional druggable pockets. Therefore, it cannot be ruled out that bifendate may exert its inhibitory effect on CCND1 through a binding conformation in this pocket, given that multiple compound structures bound to the Ala-16 and Tyr-17 pocket have been resolved.
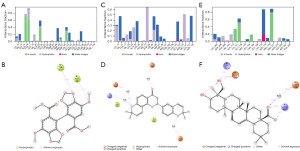
In the case of CDK4-xambioona, as shown in Figure 6C,6D, hydrophobic interactions and water bridges played a crucial role in maintaining a stable binding conformation between the compound and its target. Additionally, ionic interactions and hydrogen bonds also contributed to the interaction between the two. Water bridges were particularly essential for stabilizing the interaction between xambioona and specific residues throughout the simulation, indicating that xambioona may hinder the activity of CDK4 by blocking ATP binding to the protein.
For EGFR-hederagenin, as shown in Figure 6E,6F, hydrogen bonds and water bridges were the primary types of interactions, along with some hydrophobic and ionic interactions. Hederagenin interacted with specific residues through hydrogen bonds and water bridges, with nearly constant interaction with certain residues throughout the simulation. Similar to xambioona, hederagenin also impacted the adenosine triphosphate (ATP) binding site of EGFR, particularly Lys-721 (57), specifically by blocking the binding of ATP to the protein. This suggests that hederagenin may limit the activity of EGFR.
Evaluation of ADMET properties for bifendate as a drug candidate
Computational evaluation of ADMET properties is a crucial step in investigating the safety of drug candidates, as it helps to avoid drug adverse reactions and toxicity (52). This ADMET characterization is an essential part of the drug discovery process since it reduces costs and development times in clinical trials (58). The molecular properties of three compounds were calculated, such as molecular weight (MW) and volume. A radar chart was generated based on the theoretical range of drug-like compound properties, and compounds outside of this range were eliminated. The results, shown in Figure 7, indicate that only bifendate falls within the theoretical range of drug-like compounds. The log of the octanol/water partition coefficient (LogP) and log at physiological Ph 7.4 (LogD) of the other two compounds exceed the maximum theoretical value. Additionally, xambioona’s MaxRing, the number of atoms in the biggest ring, also exceeds the theoretical range, detailed in Table S2. Therefore, only bifendate, which conforms to drug-like compound properties, was selected for ADMET analysis.
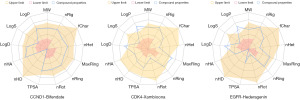
In the realm of medicinal chemistry, bifendate displays a promising potential for drug-like properties, as indicated by a high QED score of 0.672 and adherence to Lipinski and Pfizer rules (59). Furthermore, it possesses a low SA score of 2.808, suggesting relative ease of synthesis. Notably, it has not been classified as a PAINS compound.
Regarding absorption, bifendate exhibits favorable permeability in the Caco-2 intestinal permeability model with a value of −4.606, indicating good intestinal absorption. Its MDCK permeability is relatively high at 3.7e−05, implying passive permeability. The compound does not inhibit P-glycoprotein and can serve as a substrate for it, making it suitable for both Caco-2 and MDCK cell models.
Distribution analysis reveals that bifendate has a plasma protein binding (PPB) of 73.668%, which falls below the ideal range of 90%. This suggests a higher therapeutic index, as drugs with high protein binding may be immobilized and unable to reach their intended targets. Nonetheless, the compound has a blood-brain barrier (BBB) penetration value of less than 0.3, indicating a low potential for crossing the BBB and producing pharmacological effects in the central nervous system.
Excretion analysis shows that bifendate falls within the moderate range (5–15 mL/min/kg) for clearance rate (CL), with a value of 8.376 mL/min/kg. Its T1/2 value is 0.181, indicating that it likely has a half-life shorter than three hours. Metabolism analysis indicates that bifendate does not exhibit inhibitory effects on CYP2D6 and can undergo normal drug metabolism in vivo.
Toxicity analysis suggests that bifendate has a low probability of causing adverse effects, with a likelihood of less than 10% for toxic side effects such as Herg blockers, human hepatotoxicity (H-HT), AMES toxicity, and skin sensitization. Additionally, it satisfies the acute toxicity, genotoxic carcinogenicity, non-genotoxic carcinogenicity, skin sensitization, aquatic toxicity, non-biodegradable, SureChEMBL, and FAF-Drugs4 rules.
Discussion
NSCLC presents a formidable challenge in oncology (60), characterized by its heterogeneous nature and a complex molecular landscape. Despite the significant advancements in targeted therapies, the diverse genetic profiles of NSCLC tumors often result in variable responses to treatment and the development of resistance (61,62). In addressing these challenges, our study embarked on an integrative approach, combining bioinformatics, molecular docking, and dynamics simulations, to identify novel molecular targets and explore potential therapeutic strategies.
The initial phase of our study, centered on bioinformatics analysis, was pivotal in unraveling potential therapeutic targets (63). By meticulously analyzing the interactions between the components of Yiqi Sanjie formula and genes related to NSCLC, we identified several key proteins and pathways implicated in the disease. This analysis not only reinforced the known roles of proteins such as EGFR (64) and MET (65) in NSCLC but also brought to light the potential significance of ADH1B in the disease’s progression (66). These insights were instrumental in steering the direction of our subsequent drug discovery and target validation efforts.
Building on this foundation, the molecular docking analysis provided significant insights into the interactions between the identified targets and the active ingredients of Yiqi Sanjie formula (67). Notably, compounds such as hederagenin and xambioona exhibited high docking scores with critical NSCLC targets, namely EGFR and CDK4, suggesting strong binding affinities. These findings are particularly promising, hinting at the potential of these compounds to disrupt key signaling pathways involved in NSCLC tumor growth and progression.
Another crucial consideration in our study is the specificity of drug targeting on the identified proteins. Specificity is essential to minimize off-target effects and enhance the therapeutic efficacy of treatments (Latest Developed Strategies to Minimize the Off-Target Effects in CRISPR-Cas-Mediated Genome Editing). The molecular docking and dynamics simulations provided valuable insights into potential binding affinities, but further experimental validation is necessary to confirm the specificity of these interactions. Particularly for compounds like hederagenin, which shows potential for targeting EGFR, it is important to thoroughly investigate its specificity against other proteins to ensure precise targeting. Similarly, for xambioona and bifendate, further studies should explore their specificity to CDK4 and CCND1, respectively. This will help ascertain their therapeutic potential while minimizing unintended effects. Thus, specificity remains an essential factor in assessing the clinical viability of these compounds, and our future work will focus on validating these findings through comprehensive in vitro and in vivo studies.
The further dimension was added to our understanding through MD simulations, which offered a more nuanced view of the stability of drug-target interactions (68). The EGFR-hederagenin complex, for instance, maintained a stable binding throughout the simulation, indicative of a robust and potentially effective therapeutic interaction (69). In contrast, the variability observed in other drug–target interactions, such as that of ADH1B-Salvigenin, highlighted the necessity for more comprehensive investigations to fully ascertain their therapeutic viability.
In our investigation, we focused on predicted interactions of natural products with three proteins involving key NSCLC targets: hederagenin with EGFR, xambioona with CDK4, and bifendate with CCND1. Notably, the inhibitory potential of hederagenin and its derivatives on EGFR is supported by existing literature (70), highlighting the validity of this pairing as a therapeutic strategy in NSCLC. This confirmation aligns well with our findings and underscores the potential of the EGFR-hederagenin interaction in NSCLC treatment. Conversely, the interactions involving xambioona and CDK4, as well as bifendate and CCND1, although promising, have yet to be extensively validated in scientific literature. Xambioona’s targeting of CDK4, a key regulator of the cell cycle, suggests its potential to arrest tumor cell growth and induce apoptosis, directly addressing the dysregulation of the cell cycle observed in NSCLC (71). However, the optimization of xambioona’s pharmacological properties might be necessary to fully realize its therapeutic efficacy (72). Similarly, bifendate’s interaction with CCND1, coupled with its balanced ADMET profile, indicates its potential role in disrupting cell cycle progression in NSCLC, a critical aspect of the disease’s pathology (73).
Conclusions
These findings collectively highlight the significant potential application value of these interactions in NSCLC therapy. While the EGFR-hederagenin pairing is already supported by existing research, the other two combinations—xambioona with CDK4 and bifendate with CCND1—represent novel and promising areas for future investigation. Their exploration could lead to groundbreaking advancements in NSCLC treatment, particularly in targeting mechanisms that are currently underexplored in existing therapeutic strategies.
Acknowledgments
We thank Sinha and Alexandrow for the generous gift of reagents used in our work. The authors also appreciate the great support from Dr. Xiongbin Lu (Indiana University School of Medicine, USA) in improving the quality of this paper.
Funding: None.
Footnote
Reporting Checklist: The authors have completed the MDAR reporting checklist. Available at https://tcr.amegroups.com/article/view/10.21037/tcr-24-972/rc
Peer Review File: Available at https://tcr.amegroups.com/article/view/10.21037/tcr-24-972/prf
Conflicts of Interest: All authors have completed the ICMJE uniform disclosure form (available at https://tcr.amegroups.com/article/view/10.21037/tcr-24-972/coif). The authors have no conflicts of interest to declare.
Ethical Statement: The authors are accountable for all aspects of the work in ensuring that questions related to the accuracy or integrity of any part of the work are appropriately investigated and resolved. The study was conducted in accordance with the Declaration of Helsinki (as revised in 2013).
Open Access Statement: This is an Open Access article distributed in accordance with the Creative Commons Attribution-NonCommercial-NoDerivs 4.0 International License (CC BY-NC-ND 4.0), which permits the non-commercial replication and distribution of the article with the strict proviso that no changes or edits are made and the original work is properly cited (including links to both the formal publication through the relevant DOI and the license). See: https://creativecommons.org/licenses/by-nc-nd/4.0/.
References
- Kusumaningrum AE, Makaba S, Ali E, et al. A perspective on emerging therapies in metastatic colorectal cancer: Focusing on molecular medicine and drug resistance. Cell Biochem Funct 2024;42:e3906. [Crossref] [PubMed]
- Siegel RL, Miller KD, Wagle NS, et al. Cancer statistics, 2023. CA Cancer J Clin 2023;73:17-48. [Crossref] [PubMed]
- Mitchell CL, Zhang AL, Bruno DS, et al. NSCLC in the Era of Targeted and Immunotherapy: What Every Pulmonologist Must Know. Diagnostics (Basel) 2023;13:1117. [Crossref] [PubMed]
- Ganti AK, Klein AB, Cotarla I, et al. Update of Incidence, Prevalence, Survival, and Initial Treatment in Patients With Non-Small Cell Lung Cancer in the US. JAMA Oncol 2021;7:1824-32. [Crossref] [PubMed]
- Davodabadi F, Sajjadi SF, Sarhadi M, et al. Cancer chemotherapy resistance: Mechanisms and recent breakthrough in targeted drug delivery. Eur J Pharmacol 2023;958:176013. [Crossref] [PubMed]
- Zhou T, Liu J, Wang P, et al. Identification of Targets and Active Components of Yiqi SanJie Formula Against Lung Neoplasms Based on Network Pharmacology Analysis and Molecular Docking. Natural Product Communications 2021;16:1934578X21997677.
- Li W, Liu L, Lu Q, et al. Application effect of yiqi yangyin decoction combined with vinorelbine+cisplatin in patients with lung cancer. Oncology Progress 2022;23:2420-3.
- Li S, Zhang B. Traditional Chinese medicine network pharmacology: theory, methodology and application. Chin J Nat Med 2013;11:110-20. [Crossref] [PubMed]
- Li H, Zhao L, Zhang B, et al. A network pharmacology approach to determine active compounds and action mechanisms of ge-gen-qin-lian decoction for treatment of type 2 diabetes. Evid Based Complement Alternat Med 2014;2014:495840. [Crossref] [PubMed]
- Chen M, Zhu J, Kang J, et al. Exploration in the Mechanism of Action of Licorice by Network Pharmacology. Molecules 2019;24:2959. [Crossref] [PubMed]
- Lu L, Zhan S, Liu X, et al. Antitumor Effects and the Compatibility Mechanisms of Herb Pair Scleromitrion diffusum (Willd.) R. J. Wang-Sculellaria barbata D. Don. Front Pharmacol 2020;11:292. [Crossref] [PubMed]
- Du A, Zheng R, Disoma C, et al. Epigallocatechin-3-gallate, an active ingredient of Traditional Chinese Medicines, inhibits the 3CLpro activity of SARS-CoV-2. Int J Biol Macromol 2021;176:1-12. [Crossref] [PubMed]
- Ru J, Li P, Wang J, et al. TCMSP: a database of systems pharmacology for drug discovery from herbal medicines. J Cheminform 2014;6:13. [Crossref] [PubMed]
- Tao Y, Tian K, Chen J, et al. Network Pharmacology-Based Prediction of the Active Compounds, Potential Targets, and Signaling Pathways Involved in Danshiliuhao Granule for Treatment of Liver Fibrosis. Evid Based Complement Alternat Med 2019;2019:2630357. [Crossref] [PubMed]
- Stelzer G, Rosen N, Plaschkes I, et al. The GeneCards Suite: From Gene Data Mining to Disease Genome Sequence Analyses. Curr Protoc Bioinformatics 2016;54:1.30.1-1.30.33.
- Hamosh A, Amberger JS, Bocchini C, et al. Online Mendelian Inheritance in Man (OMIM®): Victor McKusick's magnum opus. Am J Med Genet A 2021;185:3259-65. [Crossref] [PubMed]
- Szklarczyk D, Kirsch R, Koutrouli M, et al. The STRING database in 2023: protein-protein association networks and functional enrichment analyses for any sequenced genome of interest. Nucleic Acids Res 2023;51:D638-46. [Crossref] [PubMed]
- Shannon P, Markiel A, Ozier O, et al. Cytoscape: a software environment for integrated models of biomolecular interaction networks. Genome Res 2003;13:2498-504. [Crossref] [PubMed]
- Tang Y, Li M, Wang J, et al. CytoNCA: a cytoscape plugin for centrality analysis and evaluation of protein interaction networks. Biosystems 2015;127:67-72. [Crossref] [PubMed]
- Xie G, Peng W, Li P, et al. A Network Pharmacology Analysis to Explore the Effect of Astragali Radix-Radix Angelica Sinensis on Traumatic Brain Injury. Biomed Res Int 2018;2018:3951783. [Crossref] [PubMed]
- Dennis G Jr, Sherman BT, Hosack DA, et al. DAVID: Database for Annotation, Visualization, and Integrated Discovery. Genome Biol 2003;4:3. [Crossref] [PubMed]
- Wang Y, Yu W, Shi C, et al. Network Pharmacology of Yougui Pill Combined with Buzhong Yiqi Decoction for the Treatment of Sexual Dysfunction. Evid Based Complement Alternat Med 2019;2019:1243743. [Crossref] [PubMed]
- Expansion of the Gene Ontology knowledgebase and resources. Nucleic Acids Res 2017;45:D331-8. [Crossref] [PubMed]
- Huang da W. Sherman BT, Lempicki RA. Systematic and integrative analysis of large gene lists using DAVID bioinformatics resources. Nat Protoc 2009;4:44-57. [Crossref] [PubMed]
- Palombo V, Milanesi M, Sferra G, et al. PANEV: an R package for a pathway-based network visualization. BMC Bioinformatics 2020;21:46. [Crossref] [PubMed]
- UniProt: the Universal Protein Knowledgebase in 2023. Nucleic Acids Res 2023;51:D523-31. [Crossref] [PubMed]
- Wang Y, Xiao J, Suzek TO, et al. PubChem's BioAssay Database. Nucleic Acids Res 2012;40:D400-12. [Crossref] [PubMed]
- Morris GM, Huey R, Lindstrom W, et al. AutoDock4 and AutoDockTools4: Automated docking with selective receptor flexibility. J Comput Chem 2009;30:2785-91. [Crossref] [PubMed]
- Trott O, Olson AJ. AutoDock Vina: improving the speed and accuracy of docking with a new scoring function, efficient optimization, and multithreading. J Comput Chem 2010;31:455-61. [Crossref] [PubMed]
- Eberhardt J, Santos-Martins D, Tillack AF, et al. AutoDock Vina 1.2.0: New Docking Methods, Expanded Force Field, and Python Bindings. J Chem Inf Model 2021;61:3891-8. [Crossref] [PubMed]
- DeLano WL. Pymol: An open-source molecular graphics tool. CCP4 Newsl Protein Crystallogr 2002;40:82-92.
- Adasme MF, Linnemann KL, Bolz SN, et al. PLIP 2021: expanding the scope of the protein-ligand interaction profiler to DNA and RNA. Nucleic Acids Res 2021;49:W530-4. [Crossref] [PubMed]
- Bowers KJ, Chow E, Xu H, et al., editors. Scalable algorithms for molecular dynamics simulations on commodity clusters. Proceedings of the 2006 ACM/IEEE Conference on Supercomputing; 2006, November 11-17
- Lu C, Wu C, Ghoreishi D, et al. OPLS4: Improving Force Field Accuracy on Challenging Regimes of Chemical Space. J Chem Theory Comput 2021;17:4291-300. [Crossref] [PubMed]
- Daina A, Michielin O, Zoete V. SwissADME: a free web tool to evaluate pharmacokinetics, drug-likeness and medicinal chemistry friendliness of small molecules. Sci Rep 2017;7:42717. [Crossref] [PubMed]
- Xiong G, Wu Z, Yi J, et al. ADMETlab 2.0: an integrated online platform for accurate and comprehensive predictions of ADMET properties. Nucleic Acids Res 2021;49:W5-W14. [Crossref] [PubMed]
- Yu J, Wang J, Zhao H, et al. Organic Compound Synthetic Accessibility Prediction Based on the Graph Attention Mechanism. J Chem Inf Model 2022;62:2973-86. [Crossref] [PubMed]
- Yang ZY, Yang ZJ, Dong J, et al. Structural Analysis and Identification of Colloidal Aggregators in Drug Discovery. J Chem Inf Model 2019;59:3714-26. [Crossref] [PubMed]
- Yang ZY, Dong J, Yang ZJ, et al. ChemFLuo: a web-server for structure analysis and identification of fluorescent compounds. Brief Bioinform 2021;22:bbaa282. [Crossref] [PubMed]
- Ziegler S, Pries V, Hedberg C, et al. Target identification for small bioactive molecules: finding the needle in the haystack. Angew Chem Int Ed Engl 2013;52:2744-92. [Crossref] [PubMed]
- Hurley TD, Edenberg HJ, Bosron WF. Expression and kinetic characterization of variants of human beta 1 beta 1 alcohol dehydrogenase containing substitutions at amino acid 47. J Biol Chem 1990;265:16366-72. [Crossref] [PubMed]
- Yasunami M, Kikuchi I, Sarapata D, et al. The human class I alcohol dehydrogenase gene cluster: three genes are tandemly organized in an 80-kb-long segment of the genome. Genomics 1990;7:152-8. [Crossref] [PubMed]
- Wang P, Zhang L, Huang C, et al. Distinct Prognostic Values of Alcohol Dehydrogenase Family Members for Non-Small Cell Lung Cancer. Med Sci Monit 2018;24:3578-90. [Crossref] [PubMed]
- Mootha VV, Kanoff JM, Shankardas J, et al. Marked reduction of alcohol dehydrogenase in keratoconus corneal fibroblasts. Mol Vis 2009;15:706-12. [PubMed]
- Zhang Y, Qian J, Gu C, et al. Alternative splicing and cancer: a systematic review. Signal Transduct Target Ther 2021;6:78. [Crossref] [PubMed]
- Liu X, Zhan T, Gao Y, et al. Benzophenone-1 induced aberrant proliferation and metastasis of ovarian cancer cells via activated ERα and Wnt/β-catenin signaling pathways. Environ Pollut 2022;292:118370. [Crossref] [PubMed]
- Doane AS, Elemento O. Alterations in transcriptional networks in cancer: the role of noncoding somatic driver mutations. Curr Opin Genet Dev 2022;75:101919. [Crossref] [PubMed]
- Heath MC. Apoptosis, programmed cell death and the hypersensitive response. European Journal of Plant Pathology 1998;104:117-24. [Crossref]
- Lu H, Zhou Q, He J, et al. Recent advances in the development of protein-protein interactions modulators: mechanisms and clinical trials. Signal Transduct Target Ther 2020;5:213. [Crossref] [PubMed]
- He Y, Sun MM, Zhang GG, et al. Targeting PI3K/Akt signal transduction for cancer therapy. Signal Transduct Target Ther 2021;6:425. [Crossref] [PubMed]
- Wei TT, Lin YT, Tang SP, et al. Metabolic targeting of HIF-1α potentiates the therapeutic efficacy of oxaliplatin in colorectal cancer. Oncogene 2020;39:414-27. [Crossref] [PubMed]
- Alturki NA, Mashraqi MM, Jalal K, et al. Therapeutic Target Identification and Inhibitor Screening against Riboflavin Synthase of Colorectal Cancer Associated Fusobacterium nucleatum. Cancers (Basel) 2022;14:6260. [Crossref] [PubMed]
- Yun CH, Boggon TJ, Li Y, et al. Structures of lung cancer-derived EGFR mutants and inhibitor complexes: mechanism of activation and insights into differential inhibitor sensitivity. Cancer Cell 2007;11:217-27. [Crossref] [PubMed]
- Santra MK, Wajapeyee N, Green MR. F-box protein FBXO31 mediates cyclin D1 degradation to induce G1 arrest after DNA damage. Nature 2009;459:722-5. [Crossref] [PubMed]
- Germain D, Russell A, Thompson A, et al. Ubiquitination of free cyclin D1 is independent of phosphorylation on threonine 286. J Biol Chem 2000;275:12074-9. [Crossref] [PubMed]
- Simoneschi D, Rona G, Zhou N, et al. CRL4(AMBRA1) is a master regulator of D-type cyclins. Nature 2021;592:789-93. [Crossref] [PubMed]
- Jura N, Endres NF, Engel K, et al. Mechanism for activation of the EGF receptor catalytic domain by the juxtamembrane segment. Cell 2009;137:1293-307. [Crossref] [PubMed]
- Basile AO, Yahi A, Tatonetti NP. Artificial Intelligence for Drug Toxicity and Safety. Trends Pharmacol Sci 2019;40:624-35. [Crossref] [PubMed]
- Lipinski CA, Lombardo F, Dominy BW, et al. Experimental and computational approaches to estimate solubility and permeability in drug discovery and development settings. Adv Drug Deliv Rev 2001;46:3-26. [Crossref] [PubMed]
- Yang F, Chen QF. A case of lung adenocarcinoma with MET∆ex14 mutation regressed after preoperative treatment with savolitinib, and successfully underwent radical resection. Anticancer Drugs 2023;34:302-5. [Crossref] [PubMed]
- Chen NY, Lin CW, Lai TY, et al. Increased expression of SSEA-4 on TKI-resistant non-small cell lung cancer with EGFR-T790M mutation. Proc Natl Acad Sci U S A 2024;121:e2313397121. [Crossref] [PubMed]
- Wang M, Herbst RS, Boshoff C. Toward personalized treatment approaches for non-small-cell lung cancer. Nat Med 2021;27:1345-56. [Crossref] [PubMed]
- Yanchao L, Sibin Z, Gareev I, et al. Bioinformatics analysis of potential therapeutic targets for COVID-19 infection in patients with carotid atherosclerosis. J Infect Public Health 2022;15:437-47. [Crossref] [PubMed]
- Yoneda K, Imanishi N, Ichiki Y, et al. Treatment of Non-small Cell Lung Cancer with EGFR-mutations. J UOEH 2019;41:153-63. [Crossref] [PubMed]
- Liang H, Wang M. MET Oncogene in Non-Small Cell Lung Cancer: Mechanism of MET Dysregulation and Agents Targeting the HGF/c-Met Axis. Onco Targets Ther 2020;13:2491-510. [Crossref] [PubMed]
- Polimanti R, Gelernter J. ADH1B: From alcoholism, natural selection, and cancer to the human phenome. Am J Med Genet B Neuropsychiatr Genet 2018;177:113-25. [Crossref] [PubMed]
- Agu PC, Afiukwa CA, Orji OU, et al. Molecular docking as a tool for the discovery of molecular targets of nutraceuticals in diseases management. Sci Rep 2023;13:13398. [Crossref] [PubMed]
- Filipe HAL, Loura LMS. Molecular Dynamics Simulations: Advances and Applications. Molecules 2022;27:2105. [Crossref] [PubMed]
- Majewski M, Ruiz-Carmona S, Barril X. An investigation of structural stability in protein-ligand complexes reveals the balance between order and disorder. Communications Chemistry 2019;2:110. [Crossref]
- Chen Z, Huang KY, Ling Y, et al. Discovery of an Oleanolic Acid/Hederagenin-Nitric Oxide Donor Hybrid as an EGFR Tyrosine Kinase Inhibitor for Non-Small-Cell Lung Cancer. J Nat Prod 2019;82:3065-73. [Crossref] [PubMed]
- Chen-Kiang S, Di Liberto M, Huang X, inventors; Cornell University, assignee. Targeting CDK4 and CDK6 in cancer therapy patent US 09259399. 2016 Feb 16 2016.
- Thiel C, Smit I, Baier V, et al. Using quantitative systems pharmacology to evaluate the drug efficacy of COX-2 and 5-LOX inhibitors in therapeutic situations. NPJ Syst Biol Appl 2018;4:28. [Crossref] [PubMed]
- Gautschi O, Ratschiller D, Gugger M, et al. Cyclin D1 in non-small cell lung cancer: a key driver of malignant transformation. Lung Cancer 2007;55:1-14. [Crossref] [PubMed]