Exploring the effects of matrix metalloproteinase-13 on the malignant biological behavior of tongue squamous cell carcinoma via the TNF signaling pathway based on bioinformatics methods
Highlight box
Key findings
• The key findings of this study are the identification of matrix metalloproteinase-13 (MMP-13) as a potential biomarker that may influence the malignant biological behavior of tongue squamous cell carcinoma (TSCC) through the tumor necrosis factor (TNF) signaling pathway.
What is known and what is new?
• Previously, it was known that MMPs play crucial roles in cancer progression, invasion, and metastasis. However, the specific role of MMP-13 in TSCC, and its association with the TNF signaling pathway, remained unclear.
• This manuscript adds new insights into the molecular mechanisms underlying TSCC by utilizing bioinformatics analysis of publicly available gene expression datasets. Through comprehensive analysis of gene expression profiles, pathway enrichment, and protein-protein interaction networks, we were able to identify MMP-13 as a novel candidate gene that may serve as a potential target for therapeutic intervention.
What is the implication, and what should change now?
• The implications of this finding are significant. First, MMP-13 may serve as a biomarker for early diagnosis and prognosis of TSCC. Second, targeting MMP-13 or the TNF signaling pathway may offer novel therapeutic approaches for TSCC. Finally, this study highlights the importance of bioinformatics in identifying novel biomarkers and therapeutic targets in cancer research. As a result, future research should focus on validating the role of MMP-13 in TSCC and exploring potential therapeutic strategies targeting this pathway.
Introduction
Oral cancer is a malignancy with a high incidence rate (1,2). Tongue squamous cell carcinoma (TSCC) is a prevalent form of oral cancer with a poor prognosis (3,4). TSCC exhibits aggressive biological behaviors, and has high rates of lymphatic and distant metastasis (5). Currently, due to environmental and genetic factors, the incidence of TSCC worldwide continues to rise. Early stage TSCC patients have a 5-year survival rate of up to 50%; however, most patients receive advanced-stage diagnoses, leading to poor prognosis (6) and potential adverse sequelae, such as chewing, speech, and swallowing dysfunctions (7). Despite significant advances in surgery and radiochemotherapy techniques, and the limited efficacy of some tumor-targeted therapies, there has been no substantial improvement in the prognosis of TSCC patients in recent years (8). Therefore, identifying the etiology, molecular mechanisms, and carcinogenic pathways of TSCC is crucial for developing new diagnostic and therapeutic strategies.
Machine learning has been widely applied in medical research and has shown good performance. It can be used for feature extraction, feature selection, model construction (9). In this study, we utilized the Gene Expression Omnibus (GEO), a public chip database, this study employed bioinformatics methods to examine the differentially expressed genes (DEGs) of TSCC. It also examined the related signaling pathways and protein-protein interaction (PPI) networks. The study sought to identify genes that could potentially have a major effect on the malignant biological behavior of TSCC at the molecular level. Ultimately, this research aimed to provide a theoretical foundation for uncovering the molecular mechanisms behind the occurrence of TSCC. We present this article in accordance with the STREGA reporting checklist (available at https://tcr.amegroups.com/article/view/10.21037/tcr-24-1016/rc).
Methods
Data source
The GSE13601 and GSE34106 data sets of gene chips were acquired from the GEO database (https://www.ncbi.nlm.nih.gov/geo/). The data sets comprised data for both TSCC tumor patients and normal control subjects. To be included in the study, each selected data set had to meet the following inclusion criteria: (I) represent whole-genome messenger RNA expression profiles; (II) include samples from both TSCC tumor patients and normal control subjects; (III) use original data sets that had been normalized; (IV) include more than three samples. The study was conducted in accordance with the Declaration of Helsinki (as revised in 2013).
Processing of data and selection of DEGs
A principal component analysis (PCA) was performed on the specimens from the two data sets using R software (https://www.bioconductor.org/) to examine the distribution of grouping within each dataset group. The GEO2R online tool (https://www.ncbi.nlm.nih.gov/geo/geo2r/) was used to conduct the analysis of the DEGs in these data sets. The selection criteria were as follows: |log fold change| >1 and P<0.05. From the two chips, several common DEGs were identified. Directly perform Venn plot intersection of all co expressed DEGs and conduct bioinformatics analysis of related pathways heatmaps and volcano plots were generated using R language to analyze the DEGs from both data sets. An intersection analysis was conducted using a Venn diagram to identify the consistently upregulated DEGs in relation to TSCC from both data sets.
Gene Ontology (GO) and Kyoto Encyclopedia of Genes and Genomes (KEGG) pathway enrichment analyses, and the gene set enrichment analysis (GSEA)
For this study, GO and KEGG pathway analyses were conducted to further investigate the physiological roles and possible molecular causes of the DEGs in TSCC. The expression differences of these genes could reveal key biological characteristics and pathological processes of the disease. A GO analysis is a powerful bioinformatics tool that helps us understand the functions of genes and gene products at the molecular level, as well as their location and roles in cells or organisms. This analysis maps DEGs to three main categories: (I) biological processes (BPs), which describes the roles of genes or gene sets in biological functions; (II) cellular components (CCs), which describes the location of genes within cells; and (III) molecular functions (MFs), which describes the activities of genes at the molecular level. Additionally, a GSEA was conducted to further identify the biological pathways and networks associated with the DEGs. The GSEA was conducted to provide insights into the potential functions that these genes might have in the pathophysiological process of TSCC, and in their interactions in specific pathways and networks.
PPI network analysis of common DEGs
Protein interaction network analysis on common DEGs was performed using the online database STRING (https://string-db.org/). The results were imported into Cytoscape software (https://cytoscape.org/) to visualize and analyze any correlations. The Molecular Complex Detection (MCODE) plugin was used to identify essential protein expression molecules through protein complex clustering algorithms.
Screening for core genes associated with TSCC
This study initially used the MalaCards (https://www.malacards.org/) database, an integrated resource encompassing a vast collection of human disease-related genes. We conducted a search using the keyword “TSCC” to obtain gene information related to TSCC. The MalaCards database is a reliable and widely used resource and provides a wealth of disease-related gene information, and thus allowed us to undertake a comprehensive exploration of the genes associated with TSCC. Subsequently, we conducted a MCODE analysis using a network topology-based algorithm designed to find densely connected regions, or protein complexes. The application of MCODE enabled us to identify the key protein molecules associated with TSCC. The advantage of this method is that it not only discovers dense subgraphs in a network but also identifies potential functional modules or protein complexes through the subgraphs. Finally, Venn diagrams were used to compare the TSCC-related genes obtained from the MalaCards database with the crucial protein molecules identified through the MCODE investigation. Venn diagrams are a common tool for comparing sets, and are used to visually represent the intersection and difference between two or more sets. By comparing these two data sets, we identified elements present in both data sets; that is, the key genes and protein molecules associated with TSCC.
Feature gene-directed chemical or targeted drug prediction
We entered our key genes into the search bar of the Comparative Toxicogenomics Database (CTD; https://ctdbase.org), and the search engine of the database returned chemicals or targeted drugs related to the input genes that may have a direct or indirect effect on these genes. These effects could be mediated by mechanisms such as altering gene expression, gene mutation, or gene splicing. The results were then filtered to identify chemicals or targeted drugs that may be relevant for the treatment of TSCC. This filtering process included considering the mechanisms of action of the drugs and whether they had already been applied in the treatment of TSCC or had shown potential therapeutic effects in related clinical trials.
Statistical analysis
The software used for the graphical depiction and statistical analysis was GraphPad Prism 7.0 (https://www.graphpad-prism.cn/). The data were presented as the mean ± standard deviation. For the DEG analysis, a t-test was used to calculate the P values and adjusted P values. The P values were adjusted using the false discovery rate method. A P value less than 0.05 was considered statistically significant.
Results
Selection of DEGs in TSCC
The GEO database was used to select the gene chip data sets (i.e., GSE13601 and GSE34106) that included tumors from TSCC patients and normal control subjects. Volcano plots, PCA plots, sample normalization box plots, and heatmaps were generated for the DEGs according to the selection criteria (Figure 1A,1B). Subsequently, the upregulated DEGs from both data sets were intersected, leading to the creation of a Venn diagram for the two data sets. Ultimately, 100 upregulated DEGs were identified (Figure 1C, Table 1).
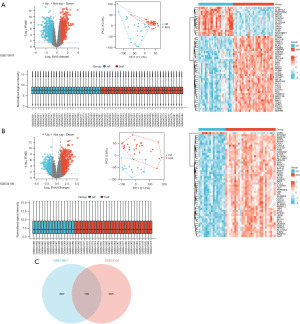
Table 1
MMP1, RBP1, MMP12, GBP1, PLAUR, LY6E, CD27, TRIM22, NFE2L3, IRF1, MMP10, CXCL11, POSTN, KIF23, DKK1, CFB, RAC2, FAT1, SKIL, CD3D, MMP13, PTHLH, APOL1, BST2, COL4A6, IFIT1, LST1, TNFRSF4, ESM1, SH2D2A, MMP3, ISG15, IL24, SCG5, OAS1, CA2, IFITM1, MDK, SPP1, CDKN3, AIM2, TAP1, TNFSF10, PLA2G7, IFI35, FYB, RNASE6, MMP11, TYMP, TNC, PLAU, SOCS1, GZMA, CDKN2A, LMNB1, SKAP2, ITK, LILRB4, LAMC2, KRT75, LAMA3, TAP2, GNLY, FOLR3, HLA-F, REL, APOC1, ADAM10, CXCL10, MYO1B, GPR183, HIF1A, IFI44, PLSCR1, SP110, IL17RA, INPP4B, TLR2, IFI27, RSAD2, MICB, IFIT3, MMP7, IFI44L, UBE2L6, SEL1L3, ODC1, SP100, MMP9, LAMP3, CXCL13, CXCL1, DLGAP5, NELL2, FN1, FCGR3A, HOXC6, CCL5 |
DEGs, differentially expressed genes.
GO and KEGG enrichment analyses
Using the DAVID online database (https://david.ncifcrf.gov/), an enrichment analysis was conducted on the chosen set of DEGs, resulting in the acquisition of enrichment information related to the GO of the DEGs. The upregulated DEGs were primarily enriched in BPs, including T-cell chemotaxis, the immune response regulating signaling pathway, leukocyte chemotaxis, the regulation of extracellular matrix (ECM) adhesion, the negative regulation of cell adhesion, the cellular response to lipopolysaccharide, and the positive regulation of mitochondrial cytochrome c release (Figure 2A). The DEGs were primarily enriched in various CCs, including the extracellular side of the plasma membrane, endocytic vesicle, endoplasmic reticulum lumen, specific granules, tertiary granules, and laminin complex (Figure 2B). As for the MFs, the analysis indicated that the DEGs were linked with endopeptidase activity, G-protein coupled receptor binding, enzyme inhibitor activity, the ECM structural constituent, receptor-ligand activity, and peptide transmembrane transporter activity (Figure 2C). The enrichment analysis of the KEGG signaling pathways revealed that the upregulated DEGs were mainly related to the Toll-like receptor signaling pathway, interleukin (IL)-17 signaling pathway, cytokine-cytokine receptor interaction, tumor necrosis factor (TNF) signaling pathway, chemokine signaling pathway, ECM-receptor interaction, and natural killer cell-mediated cytotoxicity (Figure 2D). The top five results are presented in Table 2.
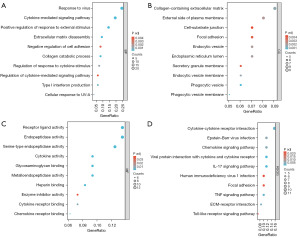
Table 2
Ontology | ID | Description | Gene ratio | Bg ratio | P value | P adjusted value |
---|---|---|---|---|---|---|
BP | GO:0009615 | Response to virus | 24/98 | 392/18,800 | 2.54e−19 | 5.5e−16 |
GO:0051607 | Defense response to virus | 19/98 | 290/18,800 | 6.06e−16 | 4.67e−13 | |
GO:0140546 | Defense response to symbiont | 19/98 | 291/18,800 | 6.46e−16 | 4.67e−13 | |
GO:0019221 | Cytokine-mediated signaling pathway | 19/98 | 486/18,800 | 6.37e−12 | 3.45e−09 | |
GO:0022617 | Extracellular matrix disassembly | 9/98 | 62/18,800 | 3.15e−11 | 1.37e−08 | |
CC | GO:0030670 | Phagocytic vesicle membrane | 5/99 | 77/19,594 | 4.41e−05 | 0.006 |
GO:0045335 | Phagocytic vesicle | 6/99 | 138/19,594 | 7.17e−05 | 0.006 | |
GO:0062023 | Collagen-containing extracellular matrix | 9/99 | 429/19,594 | <0.001 | 0.02 | |
GO:0034364 | High-density lipoprotein particle | 3/99 | 27/19,594 | <0.001 | 0.02 | |
GO:0030666 | Endocytic vesicle membrane | 6/99 | 194/19,594 | <0.001 | 0.02 | |
MF | GO:0004252 | Serine-type endopeptidase activity | 12/96 | 174/18,410 | 1.09e−10 | 2.72e−08 |
GO:0008236 | Serine-type peptidase activity | 12/96 | 191/18,410 | 3.21e−10 | 3.4e−08 | |
GO:0017171 | Serine hydrolase activity | 12/96 | 195/18,410 | 4.08e−10 | 3.4e−08 | |
GO:0004222 | Metalloendopeptidase activity | 9/96 | 111/18,410 | 6.35e−09 | 3.97e−07 | |
GO:0008009 | Chemokine activity | 6/96 | 49/18,410 | 2e−07 | 1e−05 | |
KEGG | hsa04657 | IL-17 signaling pathway | 8/70 | 94/8,164 | 1.2e−06 | <0.001 |
hsa04061 | Viral protein interaction with cytokine and cytokine receptor | 8/70 | 100/8,164 | 1.93e−06 | <0.001 | |
hsa04060 | Cytokine-cytokine receptor interaction | 11/70 | 295/8,164 | 3.67e−05 | 0.002 | |
hsa04668 | TNF signaling pathway | 7/70 | 112/8,164 | 4.46e−05 | 0.002 | |
hsa04512 | ECM-receptor interaction | 6/70 | 88/8,164 | 9.96e−05 | 0.003 |
GO, Gene Ontology; KEGG, Kyoto Encyclopedia of Genes and Genomes; Bg, background; BP, biological process; CC, cellular component; MF, molecular function; IL, interleukin; TNF, tumor necrosis factor; ECM, extracellular matrix.
Construction of the PPI network and module analysis of the common DEGs, and core gene selection
The STRING database was used to create a PPI network of the shared DEGs. The obtained data were subsequently analyzed using Cytoscape software to visually and analytically filter the PPI network. The Network Analyzer tool in Cytoscape was used to determine undirected scores for each node in the PPI network, resulting in the degree values for each node. The degree values were represented using the node size, while neighborhood connectivity was indicated by a color gradient transitioning from red to green, indicating high to low values, respectively. The thickness of the edges indicated the combined_score values. An attribute circle layout was applied to arrange all the protein nodes, with nodes with a degree ≥4 placed in the inner layer (Figure 3). Using the default settings of a node score cut-off value of 0.2, a K-core value of 2, and a maximum depth of 100, the MCODE plugin was used to perform a clustering analysis of these significant protein molecules. This produced five clusters with high scores (Figure 4A). Subsequently, the relevant genes for TSCC were retrieved from the MalaCards database, and a Venn diagram was used to find intersections between those genes and a MCODE analysis was performed to find the important protein molecules. Ultimately, we identified three key genes; that is, matrix metalloproteinase-1 (MMP1), MMP9, and MMP13 (Figure 4B).
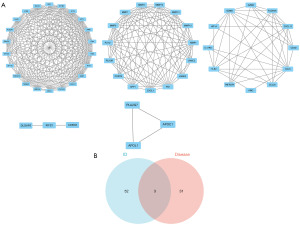
Chemical or targeted drug prediction based on feature genes in the CTD
In the CTD, we searched for therapeutic drugs related to the key gene MMP13 across various types of cancer, and identified seven related drugs specifically classified under tongue tumors (Figure 5).
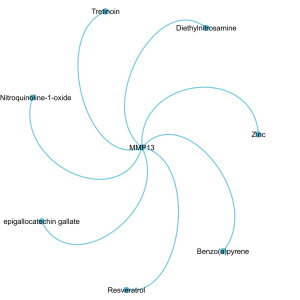
Discussion
Currently, the primary treatment modalities for TSCC include surgery, radiotherapy, chemotherapy, and targeted therapy. However, given the elevated aggressiveness of TSCC, patient prognosis remains unfavorable despite systemic treatment, and the possibility of recurrence is high. As a result, the long-term monitoring and supervision of patients are necessary (10). In addition, the targeting of TSCC is limited, and primarily focuses on the epidermal growth factor receptor (EGFR) and EGFR/AKT (AKT Serine/Threonine Kinase) (11). Hence, it is crucial that specific therapeutic targets for TSCC be identified.
Gene chips have been widely used in investigations of TSCC in recent years (12). In this study, using TSCC-related data sets from the GEO database and bioinformatics techniques, we identified 100 upregulated common DEGs. The enriched KEGG pathways were mainly related to the Toll-like receptor signaling pathway, IL-17 signaling pathway, cytokine-cytokine receptor interaction, TNF signaling pathway, chemokine signaling pathway, ECM-receptor interaction, and natural killer cell-mediated cytotoxicity. These mechanisms have also been reported in related TSCC studies. For example, Shigeoka et al. (13) found that CD163-positive macrophages play a role in the invasiveness of oral squamous cell carcinoma. Huang et al. (14) proposed that long non-coding RNAs interacting with NF-κB (Nuclear Factor Kappa B Subunit) play a crucial role in the migration and infiltration of TSCC. Leung et al. (15) identified numerous DEGs and networks resulting from ARG1 (Arginase 1) overexpression using RNA sequencing. These included natural killer cell signaling pathways, hypoxia-inducible factor alpha signaling, and interferon signaling. Their research also established a foundation for understanding the mechanism of impaired metabolism of arginine in oral TSCC. This indirectly reflects the accuracy and research value of our study.
During this investigation, a PPI network was constructed, and a module analysis of the common DEGs was performed. We ultimately identified five clusters and 55 candidate genes. An intersection analysis of the related genes in the MalaCards database yielded three key genes; that is, MMP1, MMP9, and MMP13. Matrix metalloproteinases (MMPs) belong to the zinc-dependent endopeptidase family and play roles in multiple physiological mechanisms, including organ development, tissue remodeling, inflammation regulation, and tumor diseases (16-18). MMPs play a critical role in tumor invasion and metastasis by breaking down almost all of the protein components of the ECM (19-21), and thus damaging the histological barriers that prevent tumor cells from invasively forming. As a result, interest in their function in tumor infiltration and metastasis has increased (22,23).
Recent studies have also documented the participation of the above-mentioned three critical genes in TSCC. For example, Jia et al. (24) found that by targeting MMP9 and MMP14, miR-34a may prevent TSCC from migrating and invading. Liu et al. (25) suggested that manganese superoxide dismutase promotes TSCC migration and invasion through H2O2-dependent Snail signaling, enhancing the protein levels of MMP1. There has not yet ben a study on the cellular mechanisms of MMP13 in TSCC specifically. However, MMP13 has been extensively studied in the context of infiltration and metastasis in other cancers. It is often overexpressed in various cancers and can predict poor prognosis, such as lung adenocarcinoma (26), colorectal cancer (27), ovarian cancer (28), gastric cancer (29), and prostate cancer (30). Based on the above research, we can conclude that there is a close relationship between MMP13 and the tumor microenvironment (TME). MMP13 may play the following regulatory roles in it: (I) ECM degradation and remodeling; (II) angiogenesis; (III) immune regulation.
The CTD database provides information on interactions between chemicals and genes/proteins, as well as diseases. Given the multiple roles of MMP13 in the TME, it may influence the sensitivity of tumor cells to drugs. MMP13 can enhance drug penetration into tumors by degrading ECM components, potentially increasing drug efficacy. However, it may also reduce the accumulation of drugs at the tumor site by degrading drugs or their carriers, thereby decreasing therapeutic efficacy. Thus, the expression level of MMP13 could be a potential indicator for predicting the sensitivity of TSCC patients to specific drugs. Using this approach, our study identified seven potential compounds for treating tongue tumors. Given the results mentioned above, we boldly hypothesize that MMP13 may influence the malignant biological behavior of TSCC through the TNF signaling pathway.
Conclusions
In summary, the occurrence of malignant biological events in TSCC involves several aspects, including ECM degradation and remodeling, signal pathway regulation, and immune escape. Pathologically, MMP13 primarily promotes tumor invasion and metastasis, angiogenesis, and affecting patient prognosis evaluation. The study identified essential genes and signaling pathways that enhance our knowledge of the molecular mechanisms involved. Based on these findings, we can develop treatment strategies for TSCC from the following aspects: (I) guiding the selection of treatment targets: MMP13 as a treatment target or TNF signaling pathway related target; (II) optimize personalized treatment plans: patient stratification or combination treatment plans; (III) promote new drug research and development: drug screening or clinical trial design; (IV) improve the accuracy of prognostic assessment. However, this research had a number of limitations, including a lack of related in vitro and in vivo experiments, and clinical data validation. In the future, multicenter, large-sample, and prospective research studies should be conducted to further validate, explore, solidify, and extend these findings.
Acknowledgments
Funding: None.
Footnote
Reporting Checklist: The authors have completed the STREGA reporting checklist. Available at https://tcr.amegroups.com/article/view/10.21037/tcr-24-1016/rc
Peer Review File: Available at https://tcr.amegroups.com/article/view/10.21037/tcr-24-1016/prf
Conflicts of Interest: All authors have completed the ICMJE uniform disclosure form (available at https://tcr.amegroups.com/article/view/10.21037/tcr-24-1016/coif). The authors have no conflicts of interest to declare.
Ethical Statement: The authors are accountable for all aspects of the work in ensuring that questions related to the accuracy or integrity of any part of the work are appropriately investigated and resolved. The study was conducted in accordance with the Declaration of Helsinki (as revised in 2013).
Open Access Statement: This is an Open Access article distributed in accordance with the Creative Commons Attribution-NonCommercial-NoDerivs 4.0 International License (CC BY-NC-ND 4.0), which permits the non-commercial replication and distribution of the article with the strict proviso that no changes or edits are made and the original work is properly cited (including links to both the formal publication through the relevant DOI and the license). See: https://creativecommons.org/licenses/by-nc-nd/4.0/.
References
- Qin L, Wu J. Targeting anticancer immunity in oral cancer: Drugs, products, and nanoparticles. Environ Res 2023;239:116751. [Crossref] [PubMed]
- Paduano F, Altomare E, Marrelli B, et al. Translational aspects of the modern genetics in head and neck cancers. BIOCELL 2022;46:1827-35. [Crossref]
- Wang R, Zhou X, Wang H, et al. Integrative analysis of gene expression profiles reveals distinct molecular characteristics in oral tongue squamous cell carcinoma. Oncol Lett 2019;17:2377-87. [PubMed]
- Zhong P, Liu L, Shen A, et al. Five extracellular matrix-associated genes upregulated in oral tongue squamous cell carcinoma: An integrated bioinformatics analysis. Oncol Lett 2019;18:5959-67. [Crossref] [PubMed]
- Vidiri A, Marzi S, Piludu F, et al. Magnetic resonance imaging-based prediction models for tumor stage and cervical lymph node metastasis of tongue squamous cell carcinoma. Comput Struct Biotechnol J 2023;21:4277-87. [Crossref] [PubMed]
- Vimal J, George NA, Kumar RR, et al. Identification of salivary metabolic biomarker signatures for oral tongue squamous cell carcinoma. Arch Oral Biol 2023;155:105780. [Crossref] [PubMed]
- Massé R, Duvernay J, Korbi S, et al. Oral carcinoma cuniculatum, a rare variant of squamous cell carcinoma. J Stomatol Oral Maxillofac Surg 2023; Epub ahead of print. [Crossref] [PubMed]
- Hu H, Wang Y, Li Z, et al. Overexpression of suppressor of zest 12 is associated with cervical node metastasis and unfavorable prognosis in tongue squamous cell carcinoma. Cancer Cell Int 2017;17:26. [Crossref] [PubMed]
- Lin Y, Tang M, Liu Y, et al. A narrative review on machine learning in diagnosis and prognosis prediction for tongue squamous cell carcinoma. Transl Cancer Res 2022;11:4409-15. [Crossref] [PubMed]
- Vered M, Shnaiderman-Shapiro A, Zlotogorski-Hurvitz A, et al. Cancer-associated fibroblasts in the tumor microenvironment of tongue carcinoma is a heterogeneous cell population. Acta Histochem 2019;121:151446. [Crossref] [PubMed]
- Batta N, Pandey M. Mutational spectrum of tobacco associated oral squamous carcinoma and its therapeutic significance. World J Surg Oncol 2019;17:198. [Crossref] [PubMed]
- Zhang H, Song Y, Du Z, et al. Exome sequencing identifies new somatic alterations and mutation patterns of tongue squamous cell carcinoma in a Chinese population. J Pathol 2020;251:353-64. [Crossref] [PubMed]
- Shigeoka M, Koma YI, Kodama T, et al. Tongue Cancer Cell-Derived CCL20 Induced by Interaction With Macrophages Promotes CD163 Expression on Macrophages. Front Oncol 2021;11:667174. [Crossref] [PubMed]
- Huang W, Cui X, Chen J, et al. Long non-coding RNA NKILA inhibits migration and invasion of tongue squamous cell carcinoma cells via suppressing epithelial-mesenchymal transition. Oncotarget 2016;7:62520-32. [Crossref] [PubMed]
- Leung LL, Lau NCH, Liu J, et al. The Role of Arginine Metabolism in Oral Tongue Squamous Cell Carcinoma. Cancers (Basel) 2021;13:6068. [Crossref] [PubMed]
- Bäck M, Ketelhuth DF, Agewall S. Matrix metalloproteinases in atherothrombosis. Prog Cardiovasc Dis 2010;52:410-28. [Crossref] [PubMed]
- Li X, Wu JF. Recent developments in patent anti-cancer agents targeting the matrix metalloproteinases (MMPs). Recent Pat Anticancer Drug Discov 2010;5:109-41. [Crossref] [PubMed]
- Chen YE. MMP-12, an old enzyme plays a new role in the pathogenesis of rheumatoid arthritis? Am J Pathol 2004;165:1069-70. [Crossref] [PubMed]
- Egeblad M, Werb Z. New functions for the matrix metalloproteinases in cancer progression. Nat Rev Cancer 2002;2:161-74. [Crossref] [PubMed]
- Tao L, Li Z, Lin L, et al. MMP1, 2, 3, 7, and 9 gene polymorphisms and urinary cancer risk: a meta-analysis. Genet Test Mol Biomarkers 2015;19:548-55. [Crossref] [PubMed]
- Visse R, Nagase H. Matrix metalloproteinases and tissue inhibitors of metalloproteinases: structure, function, and biochemistry. Circ Res 2003;92:827-39. [Crossref] [PubMed]
- Wang Q, Wang K, Tan X, et al. Immunomodulatory role of metalloproteases in cancers: Current progress and future trends. Front Immunol 2022;13:1064033. [Crossref] [PubMed]
- Liu M, Huang L, Liu Y, et al. Identification of the MMP family as therapeutic targets and prognostic biomarkers in the microenvironment of head and neck squamous cell carcinoma. J Transl Med 2023;21:208. [Crossref] [PubMed]
- Jia LF, Wei SB, Mitchelson K, et al. miR-34a inhibits migration and invasion of tongue squamous cell carcinoma via targeting MMP9 and MMP14. PLoS One 2014;9:e108435. [Crossref] [PubMed]
- Liu Z, Li S, Cai Y, et al. Manganese superoxide dismutase induces migration and invasion of tongue squamous cell carcinoma via H2O2-dependent Snail signaling. Free Radic Biol Med 2012;53:44-50. [Crossref] [PubMed]
- Shih DJH, Nayyar N, Bihun I, et al. Genomic characterization of human brain metastases identifies drivers of metastatic lung adenocarcinoma. Nat Genet 2020;52:371-7. [Crossref] [PubMed]
- Eiro N, Carrión JF, Cid S, et al. Toll-Like Receptor 4 and Matrix Metalloproteases 11 and 13 as Predictors of Tumor Recurrence and Survival in Stage II Colorectal Cancer. Pathol Oncol Res 2019;25:1589-97. [Crossref] [PubMed]
- Zhang H, Yang Q, Lian X, et al. Hypoxia-Inducible Factor-1α (HIF-1α) Promotes Hypoxia-Induced Invasion and Metastasis in Ovarian Cancer by Targeting Matrix Metallopeptidase 13 (MMP13). Med Sci Monit 2019;25:7202-8. [Crossref] [PubMed]
- Zhang S, Yang Y, Huang S, et al. SIRT1 inhibits gastric cancer proliferation and metastasis via STAT3/MMP-13 signaling. J Cell Physiol 2019;234:15395-406. [Crossref] [PubMed]
- Orive-Ramos A, Seoane S, Ocaña A, et al. Regulation of the prometastatic neuregulin-MMP13 axis by SRC family kinases: therapeutic implications. Mol Oncol 2017;11:1788-805. [Crossref] [PubMed]
(English Language Editor: L. Huleatt)