Identification and validation of ferroptosis-related prognostic gene signature in patients with cervical cancer
Highlight box
Key findings
• We have established a novel ferroptosis-related prognostic genes model (SLC2A1, CA9, and DUOX1) in cervical cancer (CC) using public databases and validated it in clinical specimens.
What is known and what is new?
• Ferroptosis plays an important role in the biological process of various cancers.
• In this study, the features of ferroptosis-related genes in CC were pictured, a novel prognostic model was constructed, and the interaction between ferroptosis and immune microenvironment was explored.
What is the implication, and what should change now?
• Our findings implicated that targeting ferroptosis may be a new reliable biomarker and a promising therapy in CC, and inspired further investigation into the regulatory mechanisms.
Introduction
Cervical cancer (CC) is the leading cause of cancer death in women. In 2020 alone, more than 600,000 women were newly diagnosed with CC, and 342,000 mortalities were reported worldwide (1). Persistent human papilloma virus (HPV) infection is the main cause of CC (2). However, only a minority of high-risk HPV carriers develop CC with an array of genetic and epigenetic modifications, making the prediction of outcomes a challenge (3,4). Current treatments, such as radiation, surgery, immunotherapy, and chemotherapy are exciting, but drug resistance, recurrence, and metastasis obstruct the clinical management and survival in a subset of patients. Thus, it is necessary to explore the mechanisms of carcinogenesis and progression of CC which might lead to finding new reliable biomarkers, developing the prognostic model, and optimizing management.
Selective induction of cancer cell death is the most effective antitumor therapy. Ferroptosis, a new term coined in 2012, is an iron-dependent form of regulated cell death driven by an overload of lipid peroxides on cellular membranes (5). Different from other types of regulated cell death such as apoptotic and necrotic cell death, ferroptosis has its special features of morphology, biochemistry, genetics, and mechanism. Ferroptosis reflects a confrontation between prerequisites for ferroptosis and ferroptosis defense systems. It is distinguished by a rise in intracellular iron, continuous escalation in free radical reactions primarily driven by labile and other forms of iron, a decline in glutathione production, the suppression of glutathione peroxidase (GPX4) activity, the accumulation of lipid peroxides on cell membranes, and an autophagic cell death process dependent on iron (6-9).
Compared with normal cells, tumor cells show a stronger desire for iron in the process of growing. This dependence on iron makes tumor cells more susceptible to ferroptosis (6,10). Mounting evidence shows that the role of ferroptosis in tumor is complex and context-dependent with both promotion and suppression effects in tumorigenesis (11). Several tumor-suppressors have been shown to promote ferroptosis, whereas some oncogenic signaling pathways evolve mechanisms to escape from ferroptosis (9,12). These suggest that ferroptosis holds great potential in tumor prognosis and therapies. However, the association between ferroptosis-related genes (FRGs) and prognosis in CC remains to be elucidated.
In this study, we analyzed the differentially expressed FRGs between CC and tumor-adjacent tissues, identified survival-associated signatures, constructed a ferroptosis-related prognostic model to predict the outcome of patients with CC, and explored the interaction between ferroptosis and immune microenvironment. The results were verified in clinical CC specimens and tumor-adjacent tissues. We present this article in accordance with the TRIPOD reporting checklist (available at https://tcr.amegroups.com/article/view/10.21037/tcr-23-2402/rc).
Methods
The analysis procedure is shown in Figure S1.
Collection and preprocessing of raw data
The transcriptome profiling data including the RNA sequencing data of 306 samples (CC solid tumor: 303; tumor-adjacent: 3) and corresponding clinical data were obtained from The Cancer Genome Atlas (TCGA) database (https://portal.gdc.cancer.gov/) in April 2023. All data were quantile normalized via log2-scale transformation to ensure standardization. The baseline clinical characteristics of the CC patients in the TCGA database are summarized in Table S1.
Identification of significantly different FRGs
The FerrDb database is dedicated to the study of ferroptosis regulatory factors and disease associations. The FRGs obtained from the FerrDb database were experimentally validated. FRGs were identified from the FerrDb database (13) (http://www.zhounan.org/ferrdb/current/). The significantly different FRGs between tumor and tumor-adjacent tissues were identified using the “limma” R package with the Wilcoxon test. The cut-off values were determined according to the following parameters: P<0.05; false discovery rate (FDR) <0.05; and |log2 fold change (FC)| ≥2. Then the “heatmap” and “ggplot2” R packages were applied to paint the heatmap and Volcano to visualize these different FRGs.
Identification of hub genes associated with ferroptosis
An interaction network of significantly different FRGs was performed at the Search Tool for the Retrieval of Interacting Genes (STRING) website (http://string-db.org/cgi/input.pl), and visualized in Cytoscape software (14). Hub genes (highly connected genes) were determined by calculating the degree value (number of lines connecting the genes) with a cutoff of ≥3 (15).
Identification of FRGs associated with prognosis
The “survival” R package was used for Cox regression analysis of hub genes to construct survival prognostic model. Firstly, univariate Cox analysis of overall survival (OS) was performed to identify the survival-related hub genes with a significant prognosis value (both of P and Z <0.05). Then, hub genes with significant statistical significance in univariate Cox regression were selected for multivariate Cox regression to identify the independent prognostic risk factors. Finally, the risk score of patients was calculated according to the normalized expression level of each gene and the corresponding regression coefficient in the model. CC patients were divided into the high-risk group and the low-risk group based on the median value of the risk scores. Kaplan-Meier survival curves were used to distinguish the difference in OS and progression-free interval (PFI) between the different risk groups. Time-dependent receiver operating characteristic (ROC) curve was created with “timeROC” R package, and the area under the curve (AUC) was used to evaluate the efficiency of the prognostic signature.
Verification by clinical specimens
Quantitative real-time polymerase chain reaction (qRT-PCR) and western blot were performed on 10 pairs of CC tumor and tumor-adjacent normal tissues (Biological Resource Center, The Second Affiliated Hospital of Guangzhou University of Chinese Medicine) to validate the ferroptosis-related prognostic genes (FRPGs) expression profiles. Ten CC patients who underwent radical hysterectomy from November 2018 to August 2020 in Guangdong Provincial Hospital (The Second Affiliated Hospital of Guangzhou University of Chinese Medicine) were included. This study was approved by the Ethics Committee of Guangdong Provincial Hospital of Chinese Medicine (Ethical Approval Numbers: B2015-102-01, and ZF2023-004-01). The study was conducted in accordance with the Declaration of Helsinki (as revised in 2013). In addition, for investigations involving human subjects, informed consent has been obtained from the patients involved. The baseline clinical characteristics of the CC patients in the validated clinical cohort are summarized in Table S1.
qRT-PCR
Total RNA was extracted from the sample tissues via RNAiso Plus (Accurate biology, China), followed by reverse transcription into complementary DNA. qRT-PCR was carried out using the TB Green R Premix Ex TaqTM II kit (Accurate biology). Glyceraldehyde-3-phosphate dehydrogenase (GAPDH) served as an internal control. The relative expression levels were quantified by the Ct (2−ΔΔCt) method. The primer sequences are listed in Table S2.
Western blot
Total protein was extracted from sample tissues by cutting tissues into chips, dissociating with lysis buffer, then replenishing with protease inhibitors and phosphatase inhibitors. Subsequently, the proteins were fractionated by sodium dodecyl sulfate-polyacrylamide gel electrophoresis (SDS-PAGE) and transferred to polyvinylidene fluoride membranes. The membranes were blocked with 5% evaporated milk for 2 h at room temperature, then incubated with primary antibodies against solute carrier family 2 member 1 (SLC2A1) (SAB, USA), carbonic anhydrases IX (CA9) (SAB), dual oxidase 1 (DUOX1) (BIOSS, China), and GAPDH (Abcam, USA) with rocking overnight at 4 ℃. The membranes were then incubated with the secondary antibodies (Cell Signaling Technology, USA) for 2 h at room temperature. The protein bands were visualized using the enhanced chemiluminescence detection reagents (Tanon, China) with a chemiluminometer (Tanon) and then quantified and digitized by Image J software.
Assessment of the immune microenvironment
CIBERSORT algorithm and the LM22 signature matrix were applied to calculate the proportion of tumor-infiltrating immune cells in the CC microenvironment based on the gene expression data (16). Boxplots were constructed using the “ggpubr” R package to compare the composition fraction of infiltrating immune cells between different risk groups. Moreover, the relationships between immune cells and prognostic genes were explored through TIMER 2.0 database (http://timer.cistrome.org/).
Functional enrichment analysis of FRPGs
The FRPGs were uploaded to an online biological information database, the Database for Annotation, Visualization, and Integrated Discovery (DAVID) (https://david.ncifcrf.gov/), for the functional and pathway enrichment analysis. In this study, the DAVID database was applied to study Gene Ontology (GO) and Kyoto Encyclopedia of Genes and Genomes (KEGG) pathways of FRPGs, and they were visualized through the online website (http://www.bioinformatics.com.cn) (17).
Statistical analysis
Data analyses were performed and visualized by using RStudio or GraphPad Prism software. The Student t-test and Wilcoxon test were applied to estimate the different expressions between groups. Statistical significance was determined with a P value less than 0.05 for all analyses.
Results
Differentially expressed ferroptosis signatures in CC
In total, 20,530 gene expression profiles were retrieved from TCGA dataset. The Venn diagram revealed that 344 FRGs, including driver, suppressor, and marker intersected between TCGA and FerrDb database (Figure 1A). Following the differential gene analysis, 61 dysregulated FRGs were obtained, with 33 genes showing upregulation and 28 showing downregulation in CC tumor tissues (Figure 1B,1C, Table S3).
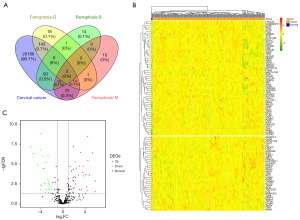
To determine the interaction relationship between the significantly different FRGs in CC, the interaction network was constructed. The analysis showed that a total of 48 genes were found interacting with each other. Among them, 24 genes were regarded as hub genes with a degree ≥3 (Figure 2).
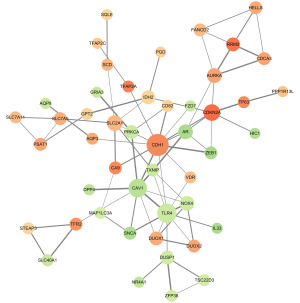
The establishment of a ferroptosis-related prognostic model
By performing the univariate Cox regression analysis in the 24 hub genes, we identified five FRPGs which were significantly associated with OS: SLC2A1 (P=0.03), solute carrier family 7 member 5 (SLC7A5) (P=0.02), CA9 (P=0.004), DUOX1 (P=0.006) and lymphoid-specific helicase (HELLS) (P=0.01) (Figure 3A). SLC2A1, CA9, and DUOX1 were subsequently recognized as independent FRPGs in a multivariate Cox regression (P=0.007, P=0.02, P<0.001, respectively) (Figure 3B). As a result, a prognostic model based on multivariate Cox regression was established, and a risk score for each patient was generated as follows: 1.29 × expression of SLC2A1 + 1.12 × expression of CA9 + 0.77 × expression of DUOX1. Then, based on the median risk score, a high-risk group and a low-risk group were stratified (Figure 3C). The likelihood of death for CC patients rises with a higher risk score and their survival time declines (Figure 3D). The risk heatmap clearly showed CA9 and SLC2A1 were up-regulated in the high-risk group, whereas DUOX1 was up-regulated in the low-risk group (Figure 3E). The OS (P<0.001) and PFI (P=0.009) in the high-risk group were considerably poorer than in the low-risk group, according to Kaplan-Meier survival curves (Figure 3F,3G). The efficiency of the signature-based risk score for OS prognostic prediction was evaluated using time-dependent ROC curves. For 1-, 3-, and 5-year, the AUC was 0.76, 0.62, and 0.65, respectively (Figure 3H). Beyond that, for the stratified analysis, the risk score showed a good predictive effect in clinical stages [both stage I + II (P=0.004) and III + IV (P=0.04)], N0 (P=0.04) and primary tumor stages [both T1 + T2 (P=0.002) and T3 + T4 (P=0.03)] (Figure S2).
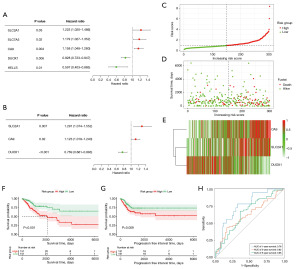
Verification of the expression profile of FRPGs
To verify the expression profile of three independent FRPGs, we carried out qRT-PCR and western blot experiments. In messenger RNA (mRNA) level, both SLC2A1 and DUOX1 were significantly higher in the tumor than in the tumor-adjacent normal tissues (P<0.05). However, there was no statistical significance of the transcription of CA9 (P>0.05) (Figure 4A). In protein level, SLC2A1 (P=0.03), CA9 (P=0.04), and DUOX1 (P=0.03) were all significantly higher in tumor tissues (Figure 4B).
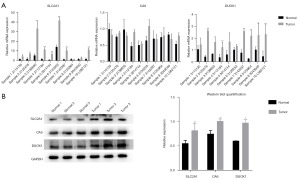
The evaluation of immune microenvironment
To investigate the interaction between immune infiltration and gene expression, we first used the CIBERSORT algorithm to calculate the proportion of 22 immune cells in each sample (Figure 5A). Macrophages (including the macrophages M0, M1, and M2 subsets), accounted for a large proportion of infiltrating immune cells. Then, we analyzed the connection between risk groups and immune cells. Heatmap showed there was a significant difference in the proportion of tumor-infiltrating immune cells between the low- and high-risk group (Figure 5B). Compared with the low-risk group, the high-risk group had high fractions of T regulatory cells (Tregs), M0 macrophages, and M2 macrophages, whereas low fractions of CD8+ T cells, naive CD4+ T cells, resting CD4+ memory T cells, activated CD4+ memory T cells, follicular helper T cells, gamma delta T cells, activated natural killer (NK) cells, naive B cells, memory B cells, monocytes, M1 macrophages, resting dendritic cells, activated mast cells, eosinophils, neutrophils (all of the above: P<0.001) and resting mast cells (P<0.05) (Figure 5C). We also computed the correlation coefficients between the prognostic FRGs expression and immune cell infiltration levels. The expression levels of SLC2A1, CA9, and DUOX1 were all negatively correlated with macrophage (cor =−0.37, −0.16, −0.16, P<0.001) (Figure 5D-5F).
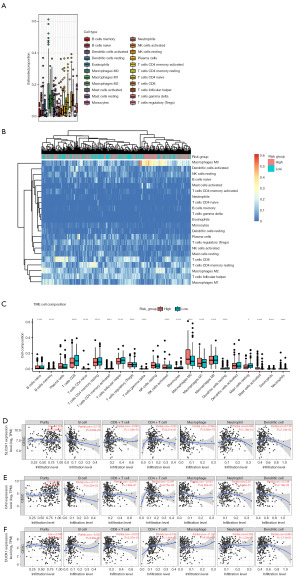
Functional enrichment analysis
To elucidate the biological functions and pathways of the FRPGs, the genes were used for functional enrichment analysis. GO-based analysis showed that the FRPGs were significantly enriched in seven signaling pathways, including biological process (xanthine dehydrogenase activity, cellular response to glucose starvation, response to hypoxia), cellular component (basolateral plasma membrane, apical plasma membrane, microvillus membrane), molecular function (transmembrane transporter activity). KEGG-based analysis showed that the FRPGs were significantly enriched in central carbon metabolism in cancer (Figure 6A,6B).
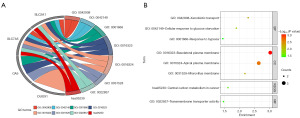
Discussion
CC is the major contributor to cancer-related mortality in women worldwide (18). Current treatments bring a favorable prognosis in early-stage CC patients. However, recurrence and metastasis affect the survival time of patients. Thus, it will be helpful to identify predictive biomarkers and carry out risk stratification management to optimize the therapy for CC patients. Nowadays, increasing evidence has shown that ferroptosis plays an important role in tumor proliferation and metastasis (6,10), and becomes a promising therapeutic strategy for drug-resistant tumors in the near future. In this study, we established a novel prognostic model with FRGs in CC using public databases and validated it in clinical specimens.
Firstly, we systematically investigated the FRGs expression profiles in CC, 61 FRGs were expressed differently between the CC and normal tissues, and 24 genes were closely interacting with each other as hub genes. Hub genes are closely associated with key biological processes and pathways in CC.
Secondly, we constructed a novel prognostic model via Cox regressions and identified SLC2A1, CA9, and DUOX1 as independent prognostic signatures. All of these three genes showed upregulation in CC tumor tissues, and the high expressions of SLC2A1 and CA9 were associated with higher risk and worse prognosis. While, paradoxically, the high expression of DUOX1 represented a lower risk and better prognosis. In line with the TCGA database, our experiments confirmed the relationship of those risk genes with CC tumorigenesis at the mRNA and protein levels. Future investigations utilizing larger independent cohorts should be performed to verify our model. SLC2A1, also known as glucose transporter 1 (GLUT1), promotes cell glycolysis metabolism and proliferation (19,20). Glycolysis is the major metabolic pathway that mammalian cells synthesize adenosine triphosphate (ATP). Glucose is transported into cells via the cell membrane glucose transporters, particularly SLC2A1, which is overexpressed in many types of tumor cells (21,22). SLC2A1 mediates glucose uptake and promotes glycolysis, thus, stimulating fatty acid synthesis, finally causing facilitating lipid peroxidation-dependent ferroptosis (23). CA9 belongs to the carbonic anhydrase family, which catalyzes reversible carbon dioxide hydration to maintain intracellular pH homeostasis (24). In malignant mesothelioma cells, CA9 inhibition under hypoxia results in iron overload in lysosomes and mitochondria, inducing oxidative stress, triggering lipid peroxidation, and leading to ferroptosis (25). Besides, both SLC2A1 and CA9 are the downstream targets of the hypoxia-inducible factor (HIF) 1α that are specific and significantly overexpressed in various tumor cells in response to hypoxia (26,27). Hypoxia is a critical carcinogenic mechanism because it contributes a more aggressive phenotype with increased invasiveness and proliferation, metastasis formation, and a worse prognosis (27,28). Previous research has demonstrated that hypoxia stimulates HIF1α transcriptional activity, thus enhancing ferroptosis resistance in CC cells (29). Some studies have found that high-risk HPVs induce the overexpression of SLC2A1 and CA9 in turn to facilitate the metabolic reprogramming in CC (30,31). So far, no conclusions about the specific roles of SLC2A1 and CA9 have been drawn from the CC. Our study suggested that the overexpression of SLC2A1 and CA9 under hypoxia may enhance the high-risk HPV infected cervical squamous cells to acquire aggressive phenotypes to evade ferroptosis.
DUOX1 is a crucial member of the nicotinamide adenine dinucleotide phosphate (NADPH) oxidases that catalyze reactive oxygen species (ROS) production (32). Consistent with our findings, a recent study showed the expression of DUOX1 was dramatically increased in CC, whereas a low level of DUOX1 expression was correlated with poor prognosis (33). Several studies have found that DUOX1 can promote or restrict tumor growth depending on its tissue expression and the type of malignancy. In thyroid carcinomas, DUOX1 induces cellular proliferation, migration, and angiogenesis by generating H2O2 (34). While in lung cancer and liver cancer, DUOX1 is frequently suppressed by DNA hypermethylation in the promoter region (35,36). DUOX1 silencing enhances lung epithelial cells’ epithelial-to-mesenchymal transition (EMT) features and promotes tumorigenic properties (37).
Thirdly, we estimated the correlation between ferroptosis and CC tumor microenvironment (TME). TME plays a key role in the occurrence and development of CC. Some immunotherapies have entered clinical trials and shown promising antitumor effectiveness in CC (38-40). However, the TME comprises not only tumor cells but also immune cells, such as T cells and macrophages, which show similar growth signals and metabolic processes to tumor cells. This characteristic leads to the similar susceptibility of immune and tumor cells to immunotherapies, which may damage immune cell function (41). Despite studies demonstrating that ferroptosis has importance with immunity in tumorigenesis and development (2), it is still unclear whether it plays the same significant role in CC. CD8+ T cells and CD4+ T cells are crucial in antitumor immunity, while Tregs are essential for maintaining immunological tolerance and suppressing antitumor immunity. In our study, samples with higher infiltrated CD8+ T cells and CD4+ T cells, whereas lower infiltrated Tregs conferred a better survival outcome. CD8+ T cells and CD4+ T cells are metabolic susceptibilities to ferroptosis. Depletion of GPX4 induces lipid peroxidation and, consequently, ferroptosis in CD8+ T cells and CD4+ T cells. In contrast, the overexpression of GPX4 can protect CD8+ T cells and CD4+ T cells from ferroptosis and recover the cells’ cytotoxic cytokine production, which enhances tumor control (42,43). Conversely, GPX4-deficient Tregs retain homeostatic survival and proliferation by obstructing the suppression of T helper 1 (TH1) and TH17 responses in a tissue-context-dependent manner (44,45).
Macrophages are an important component of TME. When they receive and integrate environmental signals, macrophages can polarize their functions along a continuum between two extremes—M1 versus M2 (46). Evidence indicates that in high-stage intraepithelial neoplasia (premalignant lesion of CC) tissue, pro-inflammatory and anti-tumor M1 macrophages are the dominant cell subpopulations, which are also suppressed by Tregs. On the contrary, CC tumor tissue has more infiltration of anti-inflammatory and tumor promoting M2 macrophages, which will further depress the anti-tumor ability of cytotoxic CD8+ T cells. Researchers also pointed out that therapies targeting the macrophage polarization may be effective in preventing the lesion from high-stage intraepithelial neoplasia to tumor (47). A current study showed that autophagy-dependent ferroptosis causes macrophages to switch to an M2-like pro-tumor phenotype via the release and uptake of oncogenic KRAS protein during oxidative stress in pancreatic ductal adenocarcinoma (48). Based on a ferroptosis-related prognostic model, our study reported that the high-risk group showed higher fractions of M2 macrophages and lower fractions of M1 macrophages than the low-risk group in CC, but the potential mechanisms remain the further investigation.
Furthermore, we conducted GO and KEGG enrichment analyses of the FRPGs. Xanthine dehydrogenase activity (GO:0042908) is implicated in purine metabolism, the dysregulation of which may lead to oxidative stress and iron overload, potentially inducing ferroptosis (49). Cellular response to glucose deprivation (GO:0042149) reflects how tumor cells adjust their metabolism to cope with energetic stress, which may impact intracellular iron metabolism and levels of ROS, thereby influencing ferroptosis (50). Response to hypoxia (GO:0001666) is a common feature in the TME and might affect ferroptosis through alterations in iron metabolism and ROS production (27,28). Basal lateral plasma membrane (GO:0016323), apical plasma membrane (GO:0016324), and microvillar membrane (GO:0031528) represent distinct regions and structures of the cell membrane, where iron transport proteins related to iron metabolism may reside, with potential functional implications for ferroptosis. Transmembrane transporter activity (GO:0022857) involves the import and export of iron ions within tumor cells, likely affecting intracellular iron levels and subsequently influencing ferroptosis. Central carbon metabolism in cancer (KEGG hsa05230) reflects how tumor cells reprogram their energy and biosynthetic metabolism to accommodate rapid growth demands (51). This metabolic reprogramming might impact iron metabolism and ferroptosis since iron serves as a cofactor for many metabolic enzymes. Overall, these pathways may modulate iron metabolism, ROS generation, and cell death signaling, as well as the metabolic reprogramming of CC cells, which collectively could influence the process of ferroptosis.
Compared to previous researches, the highlights of our study include the exploration of FRGs utilizing the FerrDb database, considerable improvements in the biological importance and robustness of the prognostic model through the construction of Hub genes, and validation using clinical specimens. However, our study also has its limitations. We only utilized 10 pairs of clinical specimens to validate the FRPGs model. Future studies should employ a larger sample size for validation to ensure more reliable results. Moreover, the mechanism by which ferroptosis influences the occurrence and development of CC requires further in-depth investigation in the future.
Conclusions
In conclusion, we have developed a novel FRPGs model capable of independently identifying patients with a high risk of poor survival in CC. Our results showed that ferroptosis has a particular significance in the development and progression of CC, and has deepened the understanding of CC tumorigenesis. Our findings implicated that targeting ferroptosis may be a new reliable biomarker and a promising therapy in CC, and inspired further investigation into the regulatory mechanisms.
Acknowledgments
We acknowledge Run-Gao Li (South China University of Technology, Guangzhou, China) for his valuable help with statistical analyses.
Funding: This study was funded by
Footnote
Reporting Checklist: The authors have completed the TRIPOD reporting checklist. Available at https://tcr.amegroups.com/article/view/10.21037/tcr-23-2402/rc
Data Sharing Statement: Available at https://tcr.amegroups.com/article/view/10.21037/tcr-23-2402/dss
Peer Review File: Available at https://tcr.amegroups.com/article/view/10.21037/tcr-23-2402/prf
Conflicts of Interest: All authors have completed the ICMJE uniform disclosure form (available at https://tcr.amegroups.com/article/view/10.21037/tcr-23-2402/coif). X.F.R. reports that this study was funded by the Specific Research Fund for Traditional Chinese Medicine Science and Technology at Guangdong Provincial Hospital of Chinese Medicine (No. YN2022QN10, to X.F.R.), and the Research Fund of Traditional Chinese Medicine Bureau of Guangdong Province (No. 20231096, to X.F.R.). D.T.W. reports that this study was funded by the Specific Research Fund for Traditional Chinese Medicine Science and Technology at Guangdong Provincial Hospital of Chinese Medicine (No. ZY2022YL25, to D.T.W.). J.X. reports that this study was funded by the Research Fund of Guangzhou Municipal Science and Technology Bureau (No. 202102010285, to J.X.). The other authors have no conflicts of interest to declare.
Ethical Statement:
Open Access Statement: This is an Open Access article distributed in accordance with the Creative Commons Attribution-NonCommercial-NoDerivs 4.0 International License (CC BY-NC-ND 4.0), which permits the non-commercial replication and distribution of the article with the strict proviso that no changes or edits are made and the original work is properly cited (including links to both the formal publication through the relevant DOI and the license). See: https://creativecommons.org/licenses/by-nc-nd/4.0/.
References
- Feldman S, Alimena S. Cervical Cancer Prevention and Cost for Women Older Than 65 Years in the US-Are We Spending Too Much or Too Little? JAMA Intern Med 2023;183:20-1. [Crossref] [PubMed]
- Rahangdale L, Mungo C, O'Connor S, et al. Human papillomavirus vaccination and cervical cancer risk. BMJ 2022;379:e070115. [Crossref] [PubMed]
- Sawaya GF, Smith-McCune K, Kuppermann M. Cervical Cancer Screening: More Choices in 2019. JAMA 2019;321:2018-9. [Crossref] [PubMed]
- Albulescu A, Plesa A, Fudulu A, et al. Epigenetic approaches for cervical neoplasia screening Exp Ther Med 2021;22:1481. (Review). [Crossref] [PubMed]
- Dixon SJ, Lemberg KM, Lamprecht MR, et al. Ferroptosis: an iron-dependent form of nonapoptotic cell death. Cell 2012;149:1060-72. [Crossref] [PubMed]
- Stockwell BR. Ferroptosis turns 10: Emerging mechanisms, physiological functions, and therapeutic applications. Cell 2022;185:2401-21. [Crossref] [PubMed]
- Stockwell BR, Friedmann Angeli JP, Bayir H, et al. Ferroptosis: A Regulated Cell Death Nexus Linking Metabolism, Redox Biology, and Disease. Cell 2017;171:273-85. [Crossref] [PubMed]
- Soula M, Weber RA, Zilka O, et al. Metabolic determinants of cancer cell sensitivity to canonical ferroptosis inducers. Nat Chem Biol 2020;16:1351-60. [Crossref] [PubMed]
- Lei G, Zhuang L, Gan B. Targeting ferroptosis as a vulnerability in cancer. Nat Rev Cancer 2022;22:381-96. [Crossref] [PubMed]
- Hassannia B, Vandenabeele P, Vanden Berghe T. Targeting Ferroptosis to Iron Out Cancer. Cancer Cell 2019;35:830-49. [Crossref] [PubMed]
- Chen X, Kang R, Kroemer G, et al. Broadening horizons: the role of ferroptosis in cancer. Nat Rev Clin Oncol 2021;18:280-96. [Crossref] [PubMed]
- Yi J, Zhu J, Wu J, et al. Oncogenic activation of PI3K-AKT-mTOR signaling suppresses ferroptosis via SREBP-mediated lipogenesis. Proc Natl Acad Sci U S A 2020;117:31189-97. [Crossref] [PubMed]
- Zhou N, Yuan X, Du Q, et al. FerrDb V2: update of the manually curated database of ferroptosis regulators and ferroptosis-disease associations. Nucleic Acids Res 2023;51:D571-82. [Crossref] [PubMed]
- Tang S, Jing H, Huang Z, et al. Identification of key candidate genes in neuropathic pain by integrated bioinformatic analysis. J Cell Biochem 2020;121:1635-48. [Crossref] [PubMed]
- Wang WL, Batzorig U, Hung CS, et al. Aldehyde Dehydrogenase 2 Family Member (ALDH2) Is a Therapeutic Index for Oxaliplatin Response on Colorectal Cancer Therapy with Dysfunction p53. Biomed Res Int 2022;2022:1322788. [Crossref] [PubMed]
- Song Y, Jiang Y, Shi L, et al. Comprehensive analysis of key m5C modification-related genes in type 2 diabetes. Front Genet 2022;13:1015879. [Crossref] [PubMed]
- Mall R, Saad M, Roelands J, et al. Network-based identification of key master regulators associated with an immune-silent cancer phenotype. Brief Bioinform 2021;22:bbab168. [Crossref] [PubMed]
- Sung H, Ferlay J, Siegel RL, et al. Global Cancer Statistics 2020: GLOBOCAN Estimates of Incidence and Mortality Worldwide for 36 Cancers in 185 Countries. CA Cancer J Clin 2021;71:209-49. [Crossref] [PubMed]
- Shen C, Xuan B, Yan T, et al. m(6)A-dependent glycolysis enhances colorectal cancer progression. Mol Cancer 2020;19:72. [Crossref] [PubMed]
- Liu XS, Yang JW, Zeng J, et al. SLC2A1 is a Diagnostic Biomarker Involved in Immune Infiltration of Colorectal Cancer and Associated With m6A Modification and ceRNA. Front Cell Dev Biol 2022;10:853596. [Crossref] [PubMed]
- Vander Heiden MG, DeBerardinis RJ. Understanding the Intersections between Metabolism and Cancer Biology. Cell 2017;168:657-69. [Crossref] [PubMed]
- Iessi E, Vona R, Cittadini C, et al. Targeting the Interplay between Cancer Metabolic Reprogramming and Cell Death Pathways as a Viable Therapeutic Path. Biomedicines 2021;9:1942. [Crossref] [PubMed]
- Song X, Liu J, Kuang F, et al. PDK4 dictates metabolic resistance to ferroptosis by suppressing pyruvate oxidation and fatty acid synthesis. Cell Rep 2021;34:108767. [Crossref] [PubMed]
- Zhang C, Lu X, Liu X, et al. Carbonic Anhydrase IX Controls Vulnerability to Ferroptosis in Gefitinib-Resistant Lung Cancer. Oxid Med Cell Longev 2023;2023:1367938. [Crossref] [PubMed]
- Li Z, Jiang L, Chew SH, et al. Carbonic anhydrase 9 confers resistance to ferroptosis/apoptosis in malignant mesothelioma under hypoxia. Redox Biol 2019;26:101297. [Crossref] [PubMed]
- Gaber G, El Achy S, Khedr GA, et al. Impact of p53, HIF1a, Ki-67, CA-9, and GLUT1 Expression on Treatment Outcomes in Locally Advanced Cervical Cancer Patients Treated With Definitive Chemoradiation Therapy. Am J Clin Oncol 2021;44:58-67. [Crossref] [PubMed]
- Seeber LM, Horrée N, Vooijs MA, et al. The role of hypoxia inducible factor-1alpha in gynecological cancer. Crit Rev Oncol Hematol 2011;78:173-84. [Crossref] [PubMed]
- Jing X, Yang F, Shao C, et al. Role of hypoxia in cancer therapy by regulating the tumor microenvironment. Mol Cancer 2019;18:157. [Crossref] [PubMed]
- Xiong J, Nie M, Fu C, et al. Hypoxia Enhances HIF1α Transcription Activity by Upregulating KDM4A and Mediating H3K9me3, Thus Inducing Ferroptosis Resistance in Cervical Cancer Cells. Stem Cells Int 2022;2022:1608806. [Crossref] [PubMed]
- Priego-Hernández VD, Arizmendi-Izazaga A, Soto-Flores DG, et al. Expression of HIF-1α and Genes Involved in Glucose Metabolism Is Increased in Cervical Cancer and HPV-16-Positive Cell Lines. Pathogens 2022;12:33. [Crossref] [PubMed]
- Reyna-Hernández MA, Alarcón-Romero LDC, Ortiz-Ortiz J, et al. GLUT1, LDHA, and MCT4 Expression Is Deregulated in Cervical Cancer and Precursor Lesions. J Histochem Cytochem 2022;70:437-46. [Crossref] [PubMed]
- Ashtiwi NM, Sarr D, Rada B. DUOX1 in mammalian disease pathophysiology. J Mol Med (Berl) 2021;99:743-54. [Crossref] [PubMed]
- Cho SY, Kim S, Son MJ, et al. Dual oxidase 1 and NADPH oxidase 2 exert favorable effects in cervical cancer patients by activating immune response. BMC Cancer 2019;19:1078. [Crossref] [PubMed]
- Ameziane El Hassani R, Buffet C, Leboulleux S, et al. Oxidative stress in thyroid carcinomas: biological and clinical significance. Endocr Relat Cancer 2019;26:R131-43. [Crossref] [PubMed]
- Ling Q, Shi W, Huang C, et al. Epigenetic silencing of dual oxidase 1 by promoter hypermethylation in human hepatocellular carcinoma. Am J Cancer Res 2014;4:508-17. [PubMed]
- Luxen S, Belinsky SA, Knaus UG. Silencing of DUOX NADPH oxidases by promoter hypermethylation in lung cancer. Cancer Res 2008;68:1037-45. [Crossref] [PubMed]
- Little AC, Sham D, Hristova M, et al. DUOX1 silencing in lung cancer promotes EMT, cancer stem cell characteristics and invasive properties. Oncogenesis 2016;5:e261. [Crossref] [PubMed]
- Kang Y, Huang J, Liu Y, et al. Integrated Analysis of Immune Infiltration Features for Cervical Carcinoma and Their Associated Immunotherapeutic Responses. Front Cell Dev Biol 2021;9:573497. [Crossref] [PubMed]
- Ji H, Zhang JA, Liu H, et al. Comprehensive characterization of tumor microenvironment and m6A RNA methylation regulators and its effects on PD-L1 and immune infiltrates in cervical cancer. Front Immunol 2022;13:976107. [Crossref] [PubMed]
- Orbegoso C, Murali K, Banerjee S. The current status of immunotherapy for cervical cancer. Rep Pract Oncol Radiother 2018;23:580-8. [Crossref] [PubMed]
- Tang D, Chen X, Kang R, et al. Ferroptosis: molecular mechanisms and health implications. Cell Res 2021;31:107-25. [Crossref] [PubMed]
- Drijvers JM, Gillis JE, Muijlwijk T, et al. Pharmacologic Screening Identifies Metabolic Vulnerabilities of CD8(+) T Cells. Cancer Immunol Res 2021;9:184-99. [Crossref] [PubMed]
- Matsushita M, Freigang S, Schneider C, et al. T cell lipid peroxidation induces ferroptosis and prevents immunity to infection. J Exp Med 2015;212:555-68. [Crossref] [PubMed]
- Li C, Jiang P, Wei S, et al. Regulatory T cells in tumor microenvironment: new mechanisms, potential therapeutic strategies and future prospects. Mol Cancer 2020;19:116. [Crossref] [PubMed]
- Xu C, Sun S, Johnson T, et al. The glutathione peroxidase Gpx4 prevents lipid peroxidation and ferroptosis to sustain Treg cell activation and suppression of antitumor immunity. Cell Rep 2021;35:109235. [Crossref] [PubMed]
- Chen X, Kang R, Kroemer G, et al. Ferroptosis in infection, inflammation, and immunity. J Exp Med 2021;218:e20210518. [Crossref] [PubMed]
- Li C, Hua K. Dissecting the Single-Cell Transcriptome Network of Immune Environment Underlying Cervical Premalignant Lesion, Cervical Cancer and Metastatic Lymph Nodes. Front Immunol 2022;13:897366. [Crossref] [PubMed]
- Dai E, Han L, Liu J, et al. Autophagy-dependent ferroptosis drives tumor-associated macrophage polarization via release and uptake of oncogenic KRAS protein. Autophagy 2020;16:2069-83. [Crossref] [PubMed]
- Matsuoka M, Yamaguchi J, Kinoshita K. Clinical Significance of Elevated Xanthine Dehydrogenase Levels and Hyperuricemia in Patients with Sepsis. Int J Mol Sci 2023;24:13857. [Crossref] [PubMed]
- Meng Q, Xu J, Liang C, et al. GPx1 is involved in the induction of protective autophagy in pancreatic cancer cells in response to glucose deprivation. Cell Death Dis 2018;9:1187. [Crossref] [PubMed]
- Xia H, Huang Z, Xu Y, et al. Reprogramming of central carbon metabolism in hepatocellular carcinoma. Biomed Pharmacother 2022;153:113485. [Crossref] [PubMed]