Characterization and validation of a prognostic model for the N6-methyladenosine-associated ferroptosis gene in colon adenocarcinoma
Highlight box
Key findings
• This study developed a prognostic model using ferroptosis related genes (Ferr-RGs) and N6-methyladenosine (m6A) regulators, and evaluated its effectiveness in predicting the prognosis of patients with colon adenocarcinoma (COAD).
What is known and what is new?
• Targeting m6A to induce ferroptosis may be a promising strategy for ferroptosis-based therapy.
• Through a series of bioinformatics techniques, screening prognostic genes associated with ferroptosis and m6A for COAD may provide new targets for its treatment.
What is the implication, and what should change now?
• The outcomes of this study contribute to the advancement of personalized medicine for COAD. The prognostic model based on differentially expressed m6A-Ferr-RGs (DE-m6A-Ferr-RGs) can potentially aid in patient stratification, treatment decision-making, and prognosis prediction. The findings open avenues for further research exploring the underlying molecular mechanisms of DE-m6A-Ferr-RGs in COAD progression and may inspire the development of targeted therapeutic interventions.
Introduction
According to statistics, colon cancer ranks third in global incidence and second in mortality among all cancers, with both incidence and mortality increasing year by year. A previous study (1) has shown that about 20% of colon cancer patients have metastases by the time they are diagnosed, and up to 50% of patients with initial localized disease will develop metastases. Although surgery, chemoradiotherapy, and targeted therapy have extended the survival time of colon cancer patients to some extent, the recurrence rate is high, and cancer drug resistance increases the treatment failure rate (2). Therefore, it is of great significance to develop personalized treatment plan for each patient and effectively predict prognosis level in colon cancer treatment. N6-methyladenosine (m6A) modification is one of the most abundant modifications affecting the fate of RNA among all the ways of post-transcriptional modification of biological RNA (3). Roles of m6A in various cancers have been reported recently, and m6A methylation modification regulatory factors play a role in promoting cancer through a variety of tumor-related molecules or signaling pathways, such as MYC, Wnt/β-catenin, PI3K/AKT/mTOR, p53, BCL-2, etc. M6A regulatory factors such as methyltransferase-like 3 (METTL3), methyltransferase-like 14 (METTL14), YTH domain family (YTHDF), and heterogeneous nuclear ribonucleoprotein C-like 2 recombinant protein (hnRNPCL2) are involved in colorectal tumorigenesis (4). Through sequencing and biological function tests, Chen et al. (5) found that METTL3 induced GLUTI signal in a m6A-dependent manner, further affecting glucose uptake and utilization, and ultimately promoting the progression of colorectal tumors, while mTROC1 inhibition enhanced the anti-tumor effect of METTL3 silencing.
Ferroptosis is a reactive oxygen species (ROS)-dependent form of cell death associated with two major biochemical characteristics: iron accumulation and lipid peroxidation (6). Ferroptosis has been implicated in a broad set of biological contexts, from development to aging, immunity, and cancer. Additionally, mounting evidence has demonstrated that under specific biological contexts, multiple signaling pathways can dictate the susceptibility of cells to ferroptosis (7). Recently, ferroptosis has been associated with a variety of diseases and functions as a tumor suppressor mechanism (8-10). Ferroptosis induction is a promising method for the treatment of many diseases including neoplastic diseases. TP53-induced glycolysis and apoptosis regulator (TIGAR) is a potential regulator of iron-resistant death in the development of colon cancer. Its knockout significantly increases Erastin-induced ferroptosis in SW620 and HCT116 cells (11). Abnormal levels of m6A modification have been detected during the progression of ferroptosis in several diseases (12). Researchers have provided new insights into the molecular mechanism of dihydroartemisinin (DHA) induced ferroptosis, thus suggesting that m6A modified-dependent ferroptosis is a potential target for the treatment of liver fibrosis. The potential regulatory relationship between the m6A modification and ferroptosis and their role in the pathogenesis and progression of cancer are attracting more and more attention. However, the role of m6A in altering cancer prognosis and treatment by regulating ferroptosis has never been systematically evaluated in colon adenocarcinoma (COAD).
Hence, this study used bioinformatics technology to explore the biological functions and potential mechanisms of m6A related ferroptosis genes in the occurrence, development and prognosis of COAD, identify related biomarkers, and establish related prognosis models, so as to provide certain references for the clinical diagnosis and treatment of COAD. We present this article in accordance with the TRIPOD reporting checklist (available at https://tcr.amegroups.com/article/view/10.21037/tcr-24-88/rc).
Methods
Data sources
The transcriptomic data and clinical data of 450 COAD and 41 paracancerous samples were downloaded from The Cancer Genome Atlas (TCGA) database (https://portal.gdc.cancer.gov/) (table available at https://cdn.amegroups.cn/static/public/tcr-24-88-1.xlsx). The data with both gene expression data and survival time and survival status were combined, then, a total of 450 COAD samples served as training set. Transcriptome sequencing data and clinical data of GSE17538 (containing 232 COAD samples, Caucasian) and GSE41258 (containing 185 COAD and 54 paracancerous samples) datasets were downloaded from the Gene Expression Omnibus (GEO) database (https://www.ncbi.nlm.nih.gov/geo/) for validation sets (tables available at https://cdn.amegroups.cn/static/public/tcr-24-88-2.xls, https://cdn.amegroups.cn/static/public/tcr-24-88-3.xls). There were 624 ferroptosis related genes (Ferr-RGs) obtained from the FerrDb database (http://www.zhounan.org/ferrdb/current/), and 23 m6A regulators were obtained from the literature (13).
Survival analysis
Survminer (14) (version 0.4.8) was used to determine the optimal threshold for genes based on patient survival time and survival status, thus patients were divided into high and low gene expression groups. Then, survival of the two groups was concluded using survival (version 3.2-3) (14) to analyze the correlation between each gene and the survival of COAD patients. Spearman correlation analysis was performed for prognosis-related m6A regulators, and correlation heat map was visualized using ggplot2 (version 3.3.2) (15).
Screening of differentially expressed m6A Ferr-RGs (DE-m6A-Ferr-RGs)
The expression data of Ferr-RGs were collected from the TCGA-COAD expression matrix and correlated with prognostic m6A genes for correlation analysis. Candidate m6A-Ferr-RGs were identified based on the criterion |Cor| >0.3, P<0.05. The same operation was performed in the GSE17538 and GSE41258 datasets, respectively. The limma package (version 3.44.3) (16) was used to mine the differentially expressed genes (DEGs) between COAD and normal groups (tumor vs. normal), with the screening condition of |log2 fold change (FC)| >1 and adjusted P value <0.05. The intersection of DEGs with candidate m6A-Ferr-RGs was taken to obtain DE-m6A-Ferr-RGs, using Venn (version 1.11) to create Venn diagrams. The heat map was drawn using pheatmap (version 0.7.7) to visualize the expression patterns of genes.
Cox regression analysis
The expression data of DE-m6A-Ferr-RGs were extracted from the training set, and then combined with the overall survival (OS) and other clinical data to obtain the clinical expression data of COAD samples. The “survival” package (version 3.2-3) (14) was used for univariate Cox and multivariate Cox regression analysis. The forest maps were drawn for visualization of the analysis result.
Construction and validation of prognostic model
COAD patients were scored by the expression of the model genes and risk coefficients obtained from multivariate Cox, and the median of risk score was used as the boundary to divide patients into high- and low-risk groups. The risk curves were plotted based on risk scores. Survival curves were plotted for OS in high- and low-risk groups using “survminer” (version 0.4.8) (14). The receiver operating characteristic (ROC) curves were plotted using the “pROC” package (version 1.0.3) (17) with 1–5 years as the survival time point, and the area under the curve (AUC) area was calculated to assess the accuracy of the model.
Correlation analysis between risk score and clinical characteristics
A trilinear table was applied to show the results of chi-square tests to compare the number of patients in different clinical subgroups between high- and low-risk groups. Heat map of the gene expression in different clinical subgroups was drawn using the pheatmap package (version 0.7.7) (18). The rank sum test was deployed to compare whether there were significant differences in risk scores among stage staging and tumor node metastasis (TNM) staging subgroup. Stratified survival was performed in different clinical subgroups to further investigate the survival significance of risk score.
Independent prognostic analysis
For the COAD samples in training set (N=450), clinicopathological factors such as age, gender, height, weight, stage, and TNM stage were included in the risk model along with the risk score for univariate Cox analysis. The factors with P<0.05 were then included in the multivariate Cox analysis. The R package rms (version 6.2-0) (19) was used to construct the nomogram to predict 1-, 3- and 5-year survival in COAD patients. Calibration curves were plotted based on the regplot (version 1.1) (20).
Functional enrichment analysis
The limma package was used to perform differential analysis of genes between high- and low-risk groups on the basis of |log2FC| >0.5 and P<0.05. The R language “cluster Profiler” (version 3.18.1) (21) was used to perform functional enrichment analysis of differential genes between high- and low-risk groups. The bubble plots were plotted using enrichplot.
Immune cells, immunotherapy and drug sensitivity analysis
Using the R language immunedeconv package (version 2.0.4) (22), the proportion of each immune cell in each sample was calculated by the maximum class probability (MCP)-counter algorithm. The differences in immune cells between high- and low-risk groups were compared using the rank sum test, and the box line plots were plotted using the R language ggplot2 and ggpubr packages. Then, the Spearman correlation analysis was performed and correlation heat maps were drawn. Tumor immune dysfunction and exclusion (TIDE) scores were calculated for each TCGA-COAD sample using TIDE database (http://tide.dfci.harvard.edu/), differences in TIDE scores between high- and low-risk groups were compared. The Genomics of Drug Sensitivity in Cancer (GDSC) database (https://www.cancerrxgene.org/) contained 138 chemotherapeutic drugs that were routinely utilized in oncology treatment. The half maximal inhibitory concentration (IC50) values of each drug for each sample was calculated using pRRophetic (version 0.5) (23) based on TCGA-COAD gene expression data. Spearman correlation analysis of risk scores with IC50 values of drugs was performed.
RNA isolation and quantitative reverse transcription polymerase chain reaction (qRT-PCR)
Twenty tissues (resected cancerous tissue and adjacent tissue from the surgical removal of COAD in 10 patients) were lysed with TRIzol reagent and total RNA was isolated following the manufacturer’s instructions. The concentration of RNA was measured with a NanoPhotometer N50. Then, RNA was reverse transcribed into complementary DNA (cDNA) using the SureScript First strand cDNA synthesis kit (Servicebio, Wuhan, China). The qRT-PCR reaction consisted of 3 µL of reverse transcription product, 5 µL of 2× Universal Blue SYBR Green qPCR Master Mix, and 1 µL each of forward and reverse primer. All primer sequence information is shown in Table 1. The GAPDH gene served as an internal control, and the relative expression of genes was determined using the 2−ΔΔCt method (24). Graphpad Prism 5 was used to make the graph and calculate the P value. The study was conducted in accordance with the Declaration of Helsinki (as revised in 2013). Approval was granted by the Ethics Committee of The China-Japan Union Hospital of Jilin University (approval ID No. 2023121301). Written inform consent was waived due to the retrospective nature of this study.
Table 1
Primer | Sequence |
---|---|
HSD17B11 | |
Forward | TGAAGGCAGAAATTGGAGATGT |
Reverse | CCAGTAAGAAGGGGACCGAGAC |
VEGFA | |
Forward | CTGTCTTGGGTGCATTGGAG |
Reverse | CGATTGGATGGCAGTAGCTG |
CXCL2 | |
Forward | ACGGCAGGGAAATGTATGTGT |
Reverse | CTGCTCTAACACAGAGGGAAAC |
ASNS | |
Forward | GGAAGACAGCCCCGATTTACT |
Reverse | AGCACGAACTGTTGTAATGTCA |
FABP4 | |
Forward | GGAATGCGTCATGAAAGGCG |
Reverse | TTCAGTCCAGGTCAACGTCC |
GPX2 | |
Forward | AATGTGAGGTGAATGGGCAGA |
Reverse | GGTCATGAGGGAAAATGGGTC |
GAPDH | |
Forward | CGAAGGTGGAGTCAACGGATTT |
Reverse | ATGGGTGGAATCATATTGGAAC |
Statistical analysis
All bioinformatics analyses were undertaken in R language. The rank sum test was employed to contrast the data from different groups.
Results
Survival analysis of m6A regulators in COAD
The results of survival analysis showed that 16 m6A regulators were significantly associated with the survival of COAD patients (Figure S1). There was mainly a positive correlation between prognosis-related m6A regulatory genes (Figure S2).
Screening of DE-m6A-Ferr-RGs in COAD
A total of 1,641 DEGs existed between COAD and normal samples, of which 745 genes were up-regulated and 896 genes were down-regulated in COAD samples (Figure 1A, table available at https://cdn.amegroups.cn/static/public/tcr-24-88-4.xls). The expression data of Ferr-RGs were correlated with prognosis-related m6A regulators to obtain 298 candidate m6A-Ferr-RGs (https://cdn.amegroups.cn/static/public/tcr-24-88-5.xls). The correlation between Ferr-RGs and prognosis-related m6A regulators in the GSE17538 and GSE41258 datasets, respectively, is shown in tables available at https://cdn.amegroups.cn/static/public/tcr-24-88-6.xls, https://cdn.amegroups.cn/static/public/tcr-24-88-7.xls. Then, 47 intersected genes were obtained by intersecting the DEGs with the candidate m6A-Ferr-RGs, which were recorded as DE-m6A-Ferr-RGs (Figure 1B,1C) and incorporated into subsequent analysis.
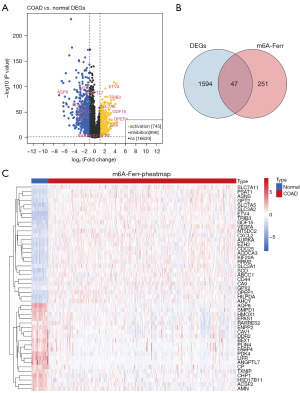
The risk model based on DE-m6A-Ferr-RGs associated with prognosis in COAD
To investigate whether DE-m6A-Ferr-RGs were associated with the prognosis of COAD patients, Cox regression analysis was performed. A total of 8 survival-related genes were obtained after univariate Cox (Figure 2A).
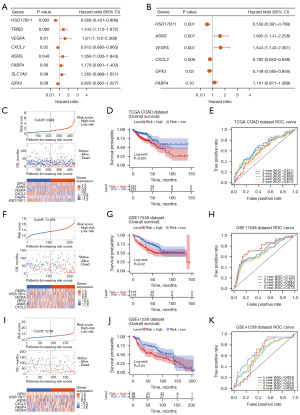
And 3 genes (HSD17B11, CXCL2, and GPX2) were protective factors [hazard ratio (HR) <1], 5 genes (TRIB3, VEGFA, ASNS, FABP4, and SLC7A5) were risk factors (HR >1). Next, a model consisting 6 model genes (HSD17B11, VEGFA, CXCL2, ASNS, FABP4, and GPX2) was obtained by multivariate Cox analysis (Figure 2B, Table 2). Risk curve was plotted based on risk scores, and COAD patients were divided into high- and low-risk groups (Figure 2C). There was a significant difference in patient survival between the high- and low-risk groups (P<0.001), and patients in the high-risk group had a lower survival rate (Figure 2D). Moreover, the AUC of the ROC curve in the training set was greater than 0.6, indicating a decent efficacy of the risk model (Figure 2E). Similarly, the prognosis were worse in the high-risk group in the validation sets GSE17538 (P=0.02) and GSE41258 (P=0.04), and the AUC at the 1–5 year node in the ROC curves were greater than 0.6 (Figure 2F-2K).
Table 2
Genes | Coef | Exp(coef) | SE(coef) | Z | P |
---|---|---|---|---|---|
HSD17B11 | −0.58749 | 0.55572 | 0.17906 | −3.281 | 0.001 |
VEGFA | 0.49623 | 1.64251 | 0.1852 | 2.679 | 0.007 |
CXCL2 | −0.24534 | 0.78244 | 0.09254 | −2.651 | 0.008 |
ASNS | 0.473 | 1.6048 | 0.17422 | 2.715 | 0.007 |
FABP4 | 0.14964 | 1.16141 | 0.09141 | 1.637 | 0.10 |
GPX2 | −0.29024 | 0.74808 | 0.12511 | −2.32 | 0.02 |
The correlation between risk score and clinical factors
First, the number of high- and low-risk patients with different clinical subtypes was compared. As shown in Table 3, there were significant correlation between risk grouping and weight, survival status, stage, and TNM stage. The heat map of the model genes expression in different clinical subgroups is shown in Figure 3A. And then there were significant difference in risk scores among stage and TNM stage subgroups (Figure 3B). In addition, in stage III + stage IV, T3 + T4, M0, and N1 + N2 subgroups, there were significant differences in survival between patients in both high- and low-risk groups (Figure 3C).
Table 3
Clinical features | Total | Risk | P value | |
---|---|---|---|---|
High | Low | |||
Age (years) | 67.0±13.0 | 66.5±13.5 | 67.5±12.5 | 0.55 |
Gender | 0.51 | |||
Female | 212 (47.1) | 110 (48.9) | 102 (45.3) | |
Male | 238 (52.9) | 115 (51.1) | 123 (54.7) | 0.24 |
Height (cm) | 168.4±12.3 | 167.5±13.0 | 169.7±11.1 | |
Weight (kg) | 80.9±20.8 | 78.8±21.8 | 83.9±18.9 | |
Vital | <0.001 | |||
Alive | 349 (77.6) | 159 (70.7) | 190 (84.4) | |
Dead | 101 (22.4) | 66 (29.3) | 35 (15.6) | 0.10 |
OS (months) | 850.3±765.4 | 809.7±758.8 | 890.9±771.4 | |
Stage | <0.001 | |||
Stage I | 75 (17.1) | 24 (11.0) | 51 (23.2) | |
Stage II | 175 (39.9) | 79 (36.1) | 96 (43.6) | |
Stage III | 126 (28.7) | 73 (33.3) | 53 (24.1) | |
Stage IV | 63 (14.4) | 43 (19.6) | 20 (9.1) | |
pT | <0.001 | |||
T1 | 11 (2.4) | 3 (1.3) | 8 (3.6) | |
T2 | 77 (17.1) | 27 (12.0) | 50 (22.3) | |
T3 | 306 (68.2) | 154 (68.4) | 152 (67.9) | |
T4 | 55 (12.2) | 41 (18.2) | 14 (6.3) | |
pN | <0.001 | |||
N0 | 265 (58.9) | 113 (50.2) | 152 (67.6) | |
N1 | 103 (22.9) | 58 (25.8) | 45 (20.0) | |
N2 | 82 (18.2) | 54 (24.0) | 28 (12.4) | |
pM | 0.002 | |||
M0 | 330 (84.0) | 154 (78.2) | 176 (89.8) | |
M1 | 63 (16.0) | 43 (21.8) | 20 (10.2) |
Data are presented as mean ± standard deviation or number (percentage). TCGA, The Cancer Genome Atlas; COAD, colon adenocarcinoma; OS, overall survival.
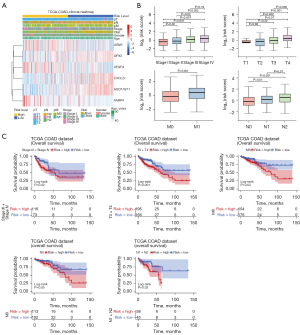
Independent prognostic analysis and nomogram model creation
To further investigate the prognosis value of clinicopathological characteristics with the risk model, clinicopathological factors along with the risk score were included in independent prognostic analysis. The results indicated that the risk score, age, and pT were independent prognostic factors in the univariate and multivariate Cox analysis (Figure 4A,4B). Hence, the nomogram was plotted based on the risk score, age and pT to predict 1-, 3-, and 5-year survival of patients with COAD. The concordance index (C-index) of the nomogram was 0.707, indicating that the prediction of the model was satisfactory (Figure 4C). Calibration curves showed that the risk model had a decent accuracy in predicting the survival rate of patients at 1, 3, and 5 years (Figure 4D).
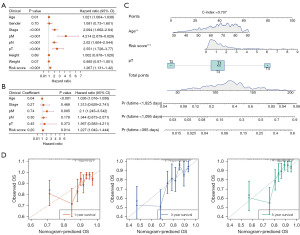
Functional differences between high- and low-risk groups
First, 111 DEGs between high- and low-risk groups were obtained (Figure 5A). Then the functional enrichment analysis was performed on them. Gene Ontology (GO) functional enrichment analysis resulted in a total of 104 items, including 39 biological process (BP) items (neutrophil migration, neutrophil chemotaxis, and chemokine response, etc.), 25 cellular component (CC) items (zymogen granule membrane, zymogen granules, and specific granule lumen, etc.), and 40 molecular function (MF) items (CXCR chemokine receptor binding, chemokine activity, and cytokine activity, etc.), and Figure 5B showed the top 10 ranked items under each GO classification. The results of Kyoto Encyclopedia of Genes and Genomes (KEGG) showed that a total of 30 pathways were enriched, the top 15 enriched pathways [tumor necrosis factor (TNF) signaling pathway, chemokine signaling pathway, cytokine-cytokine receptor interaction, and IL17 signaling pathway, etc.] are shown in Figure 5C.
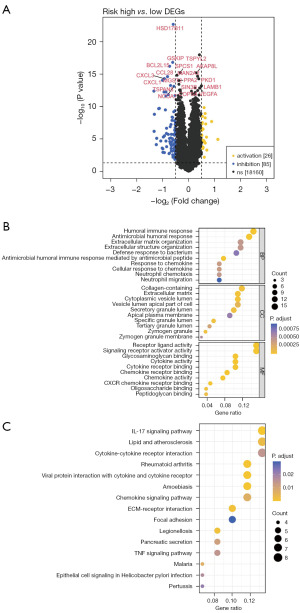
Infiltration analysis of immune/stromal cells
The proportion of each immune/stromal cells in each sample was calculated by the MCP counter algorithm. The NK cells, myeloid dendritic cells, cancer-associated fibroblasts (CAFs), endothelial cells, and neutrophils were significantly different between high- and low-risk groups (Figure 6A). The model genes were significantly positively/negatively correlated with CAF (Figure 6B).
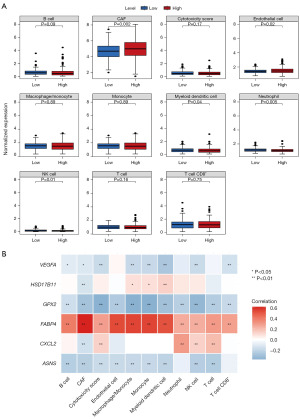
Immunotherapy and drug sensitivity analysis
TIDE scores were higher in the high-risk group compared with the low-risk group (Figure 7A). The correlation analysis between risk score and TIDE score showed a significant positive correlation (Cor =0.23, P<0.001) (Figure 7A). In addition, there was a significant difference in the number of immune responding/non-responding patients between high- and low-risk groups (P<0.001), and comparison of risk scores between patients revealed that risk scores were higher in non-responding patients (P<0.001) (Figure 7B). The correlation analysis between risk score and IC50 values of drugs from GDSC showed that Nilotinib and PD.173074 were negatively correlated with risk score (correlation coefficients less than −0.3). Meanwhile, the IC50 values of BMS.708163, lapatinib, sorafenib, and PF.4708671 were positively correlated with risk score (correlation coefficients were all greater than 0.3) (Figure 7C). Finally, the IC50 of the six drugs (|Cor| >0.3) were compared, in which the IC50 of BMS.708163, lapatinib, sorafenib, and PF.4708671 were significantly higher in high-risk group, while the IC50 of nilotinib and PD.173074 were significantly lower in high-risk group (Figure 7D).
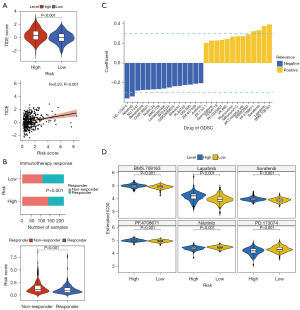
Expression validation of 6 model genes
In this study, 10 pairs of COAD and para-cancer tissue samples were collected and qRT-PCR was performed to elucidate the changes in expression of model genes in the COAD and normal groups. The expression levels of VEGFA, CXCL2 and ASNS were significantly lower in COAD samples than in paraneoplastic tissues, while the opposite was true for HSD17B11 and FABP4 (Figures 8,9), which was consistent with results from public database (table available at https://cdn.amegroups.cn/static/public/tcr-24-88-1.xlsx). In addition, although GPX2 expression was not significantly different in COAD and paraneoplastic tissues (Figures 8,9), the expression trends were consistent with public database (Figures 8,9, table available at https://cdn.amegroups.cn/static/public/tcr-24-88-1.xlsx). Finally, in order to better understand the expression of the six model genes in patients at various phases of pathogenesis, we conducted additional analysis, which revealed that CXCL2 expression was considerably raised in both the M0 and N0 stages, among others (Figure S3).
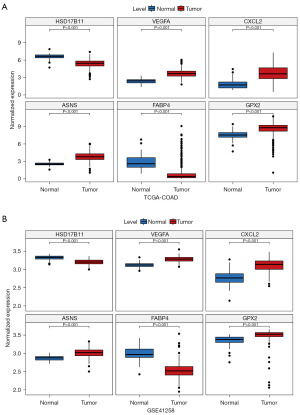
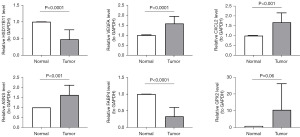
Discussion
COAD remains one of the deadliest malignancies in the world, with a poor prognosis. The high mortality rate for COAD is expected to continue in the coming decades. Therefore, further studies are needed to identify new biomarkers for the early diagnosis and effective prognosis prediction of COAD. However, there have been no studies on the correlation of m6A modified ferroptosis in colon cancer. We used the transcriptome and clinical data of COAD from public databases to construct the m6A-Ferr-related prognostic model, which may have potential significance in guiding immunotherapy and chemotherapy. Here, the prognostic value of m6A related iron deposition gene in COAD was investigated by bioinformatics analysis. We ended up with a six-gene model and a nomogram for predicting COAD patient survival.
FABP4 has been shown to be a fat-derived cytokine, which can be released into the circulation (25,26) and is involved in cancer cell growth and metastasis in a variety of malignancies (25,27). Mukherjee et al. have suggested that the regulation of FABP4 is crucial for cancer cells to adapt to lipid-rich microenvironments. Additionally, targeting FABP4 within cancer cells not only reduces their migratory capacity to the retina but also enhances tumor cell sensitivity to platinum-based chemotherapy (28). A study on colon cancer found that FABP4 protein was highly expressed in colon cancer tissues, and was positively correlated with TNM stage, differentiation degree and lymph node metastasis of colon cancer. The expression level of FABP4 in colon cancer tissues correlates with the expression of E-cadherin and Snail, suggesting that FABP4 promotes colon cancer progression associated with epithelial-mesenchymal transition (EMT) (29). This is consistent with our findings that FABP4 is a risk factor for patients’ prognosis and disease progression.
Chemokines are a class of small cytokines with a molecular weight of about 10 kDa (30), that play an integral role in various physiological and pathological processes. Chemokines are known to be expressed in a variety of cell types, including tumor cells, CAF, and tumor-associated macrophages (TAM) (31,32). In recent years, studies have identified VEGFA and CXC chemokines as important players in angiogenesis, especially tumor angiogenesis (33-35). VEGFA/kinase insertion domain receptor (KDR or VEGFR2) is a signaling pathway involved in tumor angiogenesis (36), among which VEGFA is a key driver of angiogenesis, secreted from many types of cells (including malignant cells) by stimulating the migration, invasion and proliferation of VEC (37,38). B7-h3-rich exosomes promote colon cancer angiogenesis and metastasis by activating the VEGFA/VEGFR2 and AKTl/mTOR signaling pathways. CXCL2 was also found to induce angiogenesis through neutrophil VEGFA release in a colon cancer liver metastasis model, thereby promoting cell migration and tumor growth. Our study demonstrated that both CXCL2 and VEGFA are prognostically relevant biomarkers in COAD patients, with CXCL2 as a protective factor and VEGFA as a risk factor.
GPX2 is a selenase with antioxidant properties mainly found in the gastrointestinal tract. It works by removing ROS as antioxidants (39). It has been found to be involved in tumor progression and metastasis in a variety of cancers, including colon, stomach, bladder, and lung cancers (40). Interestingly, Emmink et al. found that mice with GPX2 knocked out had higher tumor multiple, possibly due to more severe inflammation, but significantly smaller tumor volume. This finding suggests that GPX2 prevents colon tumor formation by limiting inflammation. In addition, in an induced mouse model of colon cancer liver metastasis, GPX2 depleted cells were found to show a significantly reduced ability to metastasize (41). This is consistent with our findings that GPX2 is a protective factor for patient survival and disease progression.
One of the mechanisms by which mutant KRAS promotes tumor progression in colorectal cancer (CRC) is induced by the PI3K-AKT-mTOR pathway, which causes the proto-carcinogenic signaling cascade (42). In the downstream of KRAS, activation of transcription factor 4 (ATF4) and nuclear factor erythroid-derived 2-like 2 (NRF2) can up-regulate ASNS expression (43). Notably, tumor growth in KRAS mutant CRC cells was significantly inhibited after ASNS knockdown. P53 inhibits asparagine synthesis through transcriptional down-regulation of ASNS expression and disrupts asparagine homeostasis, leading to in vivo and in vitro lymphoma and colon tumor growth and inhibition (44). High levels of ASNS maintain cell survival and promote tumor cell proliferation by inhibiting AMPK-mediated p53 activation. The surprise was that ASNase treatment or ASNS knockdown mutually activated p53 to induce cell cycle arrest and protect cells from apoptosis. Therefore, we believe that ASNS, as a biomarker associated with prognosis in COAD patients, is a risk factor. This gives us a new way to treat cancer. It is concluded that asparagine biosynthesis plays a key role in accelerating cancer growth and metastasis.
HSD17B11 is a subtype of 17-hydroxysteroid dehydrogenase, also known as DHRS8 and Pan1b. It is highly expressed in human tissues such as lung, eye, liver, pancreas, intestine, kidney, kidney, adrenal gland, heart, testis, ovary, placenta and sebaceous glands (45). It is important in a variety of tumors. HSD17B11 expression levels are high in human prostate cancer tissues, but relatively low in normal prostate tissues. Furthermore, in esophageal cancer, a study (46) has shown that the reading protein YTHDF1 may regulate tumor lipid metabolism by reducing the translation efficiency of the target gene HSD17B11. To date, no relevant reports of HSD17B11 have been found in colon cancer, and we have found for the first time that HSD17B11 gene was associated with the survival of COAD patients and affected the progression of the disease as a protective factor. Therefore, we believe that HSD17B11 is a favorable factor for patient prognosis and disease progression.
In Hallmarks of cancer: the next generation (47) article puts forward that the patient’s immune system is important for identification of prognostic markers, and predicting response to chemotherapy and radiotherapy markers is of great significance. Over the past decade, immunotherapy has generated great excitement due to its success in achieving long lasting responses in previously difficult-to-treat solid tumors, such as melanoma and lung cancer (48). In this study, we further studied the infiltration ratio of 10 kinds of immune/stromal cells in the high-low risk group and found that 5 immune cells were significantly different between the high-low risk group. Correlation analysis showed that risk model genes were significantly positively/negatively correlated with CAF, endothelial cell, myeloid dendritic cell, B cell, and natural killer (NK) cell. Again, this strongly demonstrates that the amount, type, and location of tumor immune infiltration are important for predicting the outcome of immune checkpoint blockade (ICB) therapy (49). TIDE was used to assess the difference in immunotherapy in the high- and low-risk groups, which showed greater immune resistance and more non-immune responses in the high-risk group. According to the characteristics of the high- and low-risk group, drug sensitivity analysis showed that six drugs were significantly correlated with the risk score, and there were significant differences between the high- and low-risk groups. This may help achieve a better overall outcome.
The study has some limitations. First, risk profiles were created using public data and lack new clinical samples and data. In addition, due to insufficient samples, qRT-PCR was performed on only 10 pairs of clinical samples to verify the expression of 6 risk model genes. Therefore, the function of this signature must be verified in clinical studies. In addition, the reproducibility of ferroptosis and m6A related genes obtained from the data set requires further verification. Further large-scale basic studies can confirm the conclusions of this study.
In summary, we used the transcriptome data and clinical data of colon cancer from public databases to construct the m6A-Ferr related prognostic model, which may have potential significance in guiding immunotherapy and chemotherapy.
Conclusions
In this study, m6A-Ferr-related prognostic model for COAD was constructed using transcriptome data and clinical data of COAD in public database, which may have potential immunotherapy and chemotherapy guidance implications.
Acknowledgments
Funding: None.
Footnote
Reporting Checklist: The authors have completed the TRIPOD reporting checklist. Available at https://tcr.amegroups.com/article/view/10.21037/tcr-24-88/rc
Peer Review File: Available at https://tcr.amegroups.com/article/view/10.21037/tcr-24-88/prf
Conflicts of Interest: All authors have completed the ICMJE uniform disclosure form (available at https://tcr.amegroups.com/article/view/10.21037/tcr-24-88/coif). The authors have no conflicts of interest to declare.
Ethical Statement: The authors are accountable for all aspects of the work in ensuring that questions related to the accuracy or integrity of any part of the work are appropriately investigated and resolved. The study was conducted in accordance with the Declaration of Helsinki (as revised in 2013). Approval was granted by the Ethics Committee of The China-Japan Union Hospital of Jilin University (approval ID No. 2023121301). Written inform consent was waived due to the retrospective nature of this study.
Open Access Statement: This is an Open Access article distributed in accordance with the Creative Commons Attribution-NonCommercial-NoDerivs 4.0 International License (CC BY-NC-ND 4.0), which permits the non-commercial replication and distribution of the article with the strict proviso that no changes or edits are made and the original work is properly cited (including links to both the formal publication through the relevant DOI and the license). See: https://creativecommons.org/licenses/by-nc-nd/4.0/.
References
- Ciardiello F, Ciardiello D, Martini G, et al. Clinical management of metastatic colorectal cancer in the era of precision medicine. CA Cancer J Clin 2022;72:372-401. [Crossref] [PubMed]
- Hossain MS, Karuniawati H, Jairoun AA, et al. Colorectal Cancer: A Review of Carcinogenesis, Global Epidemiology, Current Challenges, Risk Factors, Preventive and Treatment Strategies. Cancers (Basel) 2022;14:1732. [Crossref] [PubMed]
- Wiener D, Schwartz S. The epitranscriptome beyond m(6)A. Nat Rev Genet 2021;22:119-31. [Crossref] [PubMed]
- Huang W, Chen TQ, Fang K, et al. N6-methyladenosine methyltransferases: functions, regulation, and clinical potential. J Hematol Oncol 2021;14:117. [Crossref] [PubMed]
- Chen H, Gao S, Liu W, et al. RNA N(6)-Methyladenosine Methyltransferase METTL3 Facilitates Colorectal Cancer by Activating the m(6)A-GLUT1-mTORC1 Axis and Is a Therapeutic Target. Gastroenterology 2021;160:1284-1300.e16. [Crossref] [PubMed]
- Tang D, Chen X, Kang R, et al. Ferroptosis: molecular mechanisms and health implications. Cell Res 2021;31:107-25. [Crossref] [PubMed]
- Jiang X, Stockwell BR, Conrad M. Ferroptosis: mechanisms, biology and role in disease. Nat Rev Mol Cell Biol 2021;22:266-82. [Crossref] [PubMed]
- Shen M, Li Y, Wang Y, et al. N(6)-methyladenosine modification regulates ferroptosis through autophagy signaling pathway in hepatic stellate cells. Redox Biol 2021;47:102151. [Crossref] [PubMed]
- Peng P, Ren Y, Wan F, et al. Sculponeatin A promotes the ETS1-SYVN1 interaction to induce SLC7A11/xCT-dependent ferroptosis in breast cancer. Phytomedicine 2023;117:154921. [Crossref] [PubMed]
- Nie J, Zhang P, Liang C, et al. ASCL1-mediated ferroptosis resistance enhances the progress of castration-resistant prostate cancer to neurosecretory prostate cancer. Free Radic Biol Med 2023;205:318-31. [Crossref] [PubMed]
- Liu MY, Li HM, Wang XY, et al. TIGAR drives colorectal cancer ferroptosis resistance through ROS/AMPK/SCD1 pathway. Free Radic Biol Med 2022;182:219-31. [Crossref] [PubMed]
- Zhi Y, Zhang S, Zi M, et al. Potential applications of N(6) -methyladenosine modification in the prognosis and treatment of cancers via modulating apoptosis, autophagy, and ferroptosis. Wiley Interdiscip Rev RNA 2022;13:e1719. [Crossref] [PubMed]
- Zhang X, Zhang S, Yan X, et al. m6A regulator-mediated RNA methylation modification patterns are involved in immune microenvironment regulation of periodontitis. J Cell Mol Med 2021;25:3634-45. [Crossref] [PubMed]
- Wang S, Su W, Zhong C, et al. An Eight-CircRNA Assessment Model for Predicting Biochemical Recurrence in Prostate Cancer. Front Cell Dev Biol 2020;8:599494. [Crossref] [PubMed]
- Ito K, Murphy D. Application of ggplot2 to Pharmacometric Graphics. CPT Pharmacometrics Syst Pharmacol 2013;2:e79. [Crossref] [PubMed]
- Ritchie ME, Phipson B, Wu D, et al. limma powers differential expression analyses for RNA-sequencing and microarray studies. Nucleic Acids Res 2015;43:e47. [Crossref] [PubMed]
- Park SH, Goo JM, Jo CH. Receiver operating characteristic (ROC) curve: practical review for radiologists. Korean J Radiol 2004;5:11-8. [Crossref] [PubMed]
- Ni J, Liu S, Qi F, et al. Screening TCGA database for prognostic genes in lower grade glioma microenvironment. Ann Transl Med 2020;8:209. [Crossref] [PubMed]
- Iasonos A, Schrag D, Raj GV, et al. How to build and interpret a nomogram for cancer prognosis. J Clin Oncol 2008;26:1364-70. [Crossref] [PubMed]
- Austin PC, Harrell FE Jr, van Klaveren D. Graphical calibration curves and the integrated calibration index (ICI) for survival models. Stat Med 2020;39:2714-42. [Crossref] [PubMed]
- Yu G, Wang LG, Han Y, et al. clusterProfiler: an R package for comparing biological themes among gene clusters. OMICS 2012;16:284-7. [Crossref] [PubMed]
- Sturm G, Finotello F, List M. Immunedeconv: An R Package for Unified Access to Computational Methods for Estimating Immune Cell Fractions from Bulk RNA-Sequencing Data. Methods Mol Biol 2020;2120:223-32. [Crossref] [PubMed]
- Geeleher P, Cox N, Huang RS. pRRophetic: an R package for prediction of clinical chemotherapeutic response from tumor gene expression levels. PLoS One 2014;9:e107468. [Crossref] [PubMed]
- Livak KJ, Schmittgen TD. Analysis of relative gene expression data using real-time quantitative PCR and the 2(-Delta Delta C(T)) Method. Methods 2001;25:402-8. [Crossref] [PubMed]
- Hotamisligil GS, Bernlohr DA. Metabolic functions of FABPs--mechanisms and therapeutic implications. Nat Rev Endocrinol 2015;11:592-605. [Crossref] [PubMed]
- Cao H, Sekiya M, Ertunc ME, et al. Adipocyte lipid chaperone AP2 is a secreted adipokine regulating hepatic glucose production. Cell Metab 2013;17:768-78. [Crossref] [PubMed]
- Uehara H, Takahashi T, Oha M, et al. Exogenous fatty acid binding protein 4 promotes human prostate cancer cell progression. Int J Cancer 2014;135:2558-68. [Crossref] [PubMed]
- Mukherjee A, Chiang CY, Daifotis HA, et al. Adipocyte-Induced FABP4 Expression in Ovarian Cancer Cells Promotes Metastasis and Mediates Carboplatin Resistance. Cancer Res 2020;80:1748-61. [Crossref] [PubMed]
- Zhang Y, Zhang W, Xia M, et al. High expression of FABP4 in colorectal cancer and its clinical significance. J Zhejiang Univ Sci B 2021;22:136-45. [Crossref] [PubMed]
- Gao A, Yan F, Zhou E, et al. Molecular characterization and expression analysis of chemokine (CXCL12) from Nile tilapia (Oreochromis niloticus). Fish Shellfish Immunol 2020;104:314-23. [Crossref] [PubMed]
- Liao Z, Tan ZW, Zhu P, et al. Cancer-associated fibroblasts in tumor microenvironment - Accomplices in tumor malignancy. Cell Immunol 2019;343:103729. [Crossref] [PubMed]
- Pathria P, Louis TL, Varner JA. Targeting Tumor-Associated Macrophages in Cancer. Trends Immunol 2019;40:310-27. [Crossref] [PubMed]
- Situ Y, Xu Q, Deng L, et al. System analysis of VEGFA in renal cell carcinoma: The expression, prognosis, gene regulation network and regulation targets. Int J Biol Markers 2022;37:90-101. [Crossref] [PubMed]
- Situ Y, Lu X, Cui Y, et al. Systematic Analysis of CXC Chemokine-Vascular Endothelial Growth Factor A Network in Colonic Adenocarcinoma from the Perspective of Angiogenesis. Biomed Res Int 2022;2022:5137301. [Crossref] [PubMed]
- Kumar A, Cherukumilli M, Mahmoudpour SH, et al. ShRNA-mediated knock-down of CXCL8 inhibits tumor growth in colorectal liver metastasis. Biochem Biophys Res Commun 2018;500:731-7. [Crossref] [PubMed]
- Apte RS, Chen DS, Ferrara N. VEGF in Signaling and Disease: Beyond Discovery and Development. Cell 2019;176:1248-64. [Crossref] [PubMed]
- Lai E, Cascinu S, Scartozzi M. Are All Anti-Angiogenic Drugs the Same in the Treatment of Second-Line Metastatic Colorectal Cancer? Expert Opinion on Clinical Practice. Front Oncol 2021;11:637823. [Crossref] [PubMed]
- Simon T, Gagliano T, Giamas G. Direct Effects of Anti-Angiogenic Therapies on Tumor Cells: VEGF Signaling. Trends Mol Med 2017;23:282-92. [Crossref] [PubMed]
- Brzozowa-Zasada M, Ianaro A, Piecuch A, et al. Immunohistochemical Expression of Glutathione Peroxidase-2 (Gpx-2) and Its Clinical Relevance in Colon Adenocarcinoma Patients. Int J Mol Sci 2023;24:14650. [Crossref] [PubMed]
- Naiki T, Naiki-Ito A, Iida K, et al. GPX2 promotes development of bladder cancer with squamous cell differentiation through the control of apoptosis. Oncotarget 2018;9:15847-59. [Crossref] [PubMed]
- Emmink BL, Laoukili J, Kipp AP, et al. GPx2 suppression of H2O2 stress links the formation of differentiated tumor mass to metastatic capacity in colorectal cancer. Cancer Res 2014;74:6717-30. [Crossref] [PubMed]
- Hua H, Kong Q, Zhang H, et al. Targeting mTOR for cancer therapy. J Hematol Oncol 2019;12:71. [Crossref] [PubMed]
- Krall AS, Mullen PJ, Surjono F, et al. Asparagine couples mitochondrial respiration to ATF4 activity and tumor growth. Cell Metab 2021;33:1013-1026.e6. [Crossref] [PubMed]
- Deng L, Yao P, Li L, et al. p53-mediated control of aspartate-asparagine homeostasis dictates LKB1 activity and modulates cell survival. Nat Commun 2020;11:1755. [Crossref] [PubMed]
- Brereton P, Suzuki T, Sasano H, et al. Pan1b (17betaHSD11)-enzymatic activity and distribution in the lung. Mol Cell Endocrinol 2001;171:111-7. [Crossref] [PubMed]
- Duan X, Yang L, Wang L, et al. m6A demethylase FTO promotes tumor progression via regulation of lipid metabolism in esophageal cancer. Cell Biosci 2022;12:60. [Crossref] [PubMed]
- Hanahan D, Weinberg RA. Hallmarks of cancer: the next generation. Cell 2011;144:646-74. [Crossref] [PubMed]
- Ghosn M, Tselikas L, Champiat S, et al. Intratumoral Immunotherapy: Is It Ready for Prime Time? Curr Oncol Rep 2023;25:857-67. [Crossref] [PubMed]
- Jochems C, Schlom J. Tumor-infiltrating immune cells and prognosis: the potential link between conventional cancer therapy and immunity. Exp Biol Med (Maywood) 2011;236:567-79. [Crossref] [PubMed]