Integrated analysis of the lncRNA-miRNA-mRNA ceRNA network in nasopharyngeal carcinoma
Highlight box
Key findings
• We have identified key biomarkers for nasopharyngeal carcinoma (NPC) through the integration of multi-omics data.
What is known and what is new?
• Some long non-coding RNA have a close association with NPC.
• This is the first application of the competing endogenous RNA network in NPC.
What is the implication, and what should change now?
• Our study provides a direction for subsequent basic validation and clinical testing of novel NPC treatments, which could allow us to perform more targeted scientific trials.
Introduction
Nasopharyngeal carcinoma (NPC) originates from the epithelial cells of the nasopharynx and is classified as an epithelial cancer (1). In 2020, approximately 133,354 new cases of NPC were reported globally, accounting for 0.7% of all cancer cases. In addition, there were 80,008 deaths related to NPC, accounting for 0.8% of all cancer-related deaths (2). NPC is prevalent in several regions of East and Southeast Asia, particularly in Southern China, including Guangxi and Guangdong (3). According to data from 2020, the incidence of NPC in Guangxi was 10.71 per 100,000, with a mortality rate of 5.15 per 100,000 (4).
Owing to its particular anatomical location and high response to radiation, NPC is mainly treated by radiotherapy and chemotherapy. The treatment of NPC has been improved by the development of advanced radiotherapy, concurrent chemotherapy, and precise cancer staging systems (5). However, the outlook for patients diagnosed with metastatic NPC remains unfavorable, with recurrence rates as high as 82%, even after treatment with combined radiotherapy and chemotherapy (6). Therefore, it is imperative to study the mechanisms involved in the progression of NPC to improve treatment strategies for this disease.
Bioinformatics analysis of microarray gene expression data facilitates identification of dysregulated genes and provides insights into the biological processes (BPs) involved in disease development (7). For example, Li and Zhang discovered LINC01385, a new long non-coding RNA (lncRNA; non-coding RNA molecules with a length exceeding 200 nucleotides) implicated in the development of NPC. Functional analysis revealed that LINC01385 is a potential target for therapeutic intervention in NPC (8). It has been suggested that lncRNA H19 controls the expression of EZH2 by interacting with miR-630 and enhances cell invasion in NPC (9). In contrast, lncRNA FAM225A was found to promote NPC tumorigenesis by absorbing miR-590-3p/miR-1275 and increasing ITGB3 expression (10). Earlier studies have significant shortcomings because they focus on exploring the functions of only one lncRNA-microRNA (miRNA)-messenger RNA (mRNA) pathway. Therefore, our understanding of the exact molecular mechanisms and BPs underlying NPC remains limited.
Salmena et al. introduced the competing endogenous RNA (ceRNA) hypothesis in 2011 (11), which has been supported by various lines of evidence (12-14). They point out that any RNA transcript containing miRNA recognition elements (MREs) can compete with other transcripts that have the same MREs, leading to the sequestration of miRNAs and consequent alteration of miRNA target gene expression. To date, no specific ceRNA network has been described for NPC. Thus, it is of utmost importance to explore the impact of ceRNA networks on the unfavorable NPC prognosis. Understanding the roles of lncRNAs in NPC development may provide solutions to the most critical hurdles in managing this disease.
In this study, we aimed to utilize the Gene Expression Omnibus (GEO) database to acquire mRNA, miRNA, and lncRNA expression profiles in NPC-affected and normal tissues and perform a comprehensive analysis to construct a ceRNA network specific to NPC (a flow diagram of the study is shown in Figure 1). This study provides valuable insights regarding therapeutic targets for NPC, the manipulation of which could prolong patient survival, and lay the foundation for better understanding of the mechanisms underlying NPC development. We present this article in accordance with the MDAR reporting checklist (available at https://tcr.amegroups.com/article/view/10.21037/tcr-24-263/rc).
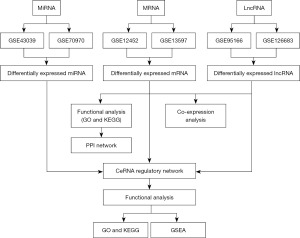
Methods
Data downloading and preprocessing
We downloaded the data from the GEO database (https://www.ncbi.nlm.nih.gov/geo/), a reliable source of NPC gene expression profiles, using R software package GEO query (version 4.0.3, http://r-project.org/) (15). The mRNA expression profiles were obtained from GSE12452 (16) and GSE13597 (17); miRNA expression profiles were obtained from GSE43039 (18) and GSE70970 (19); and lncRNA expression profiles were derived from GSE95166 (20) and GSE126683 (10). The sample and platform information for each dataset is listed in Table 1. All samples were analyzed using the limma package (21) for standardized data processing. The study was conducted in accordance with the Declaration of Helsinki (as revised in 2013).
Table 1
Type | ID | Platform | Sample | Experiment type |
---|---|---|---|---|
MRNA | GSE12452 | GPL570 | 41 | Expression profiling by array |
GSE13597 | GPL96 | 28 | Expression profiling by array | |
MiRNA | GSE70970 | GPL20699 | 263 | Non-coding RNA profiling by array |
GSE43039 | GPL16414 | 40 | Non-coding RNA profiling by array | |
LncRNA | GSE126683 | GPL16956 | 6 | Non-coding RNA profiling by array |
GSE95166 | GPL15314 | 8 | Non-coding RNA profiling by array |
MRNA, messenger RNA; miRNA, microRNA; lncRNA, long non-coding RNA.
Screening of differentially expressed genes (DEGs)
The limma package was used to identify mRNAs, miRNAs, and lncRNAs that were differentially expressed in tumor and normal samples from various datasets by using the following criteria: mRNAs, |log2fold change (FC)| >1; miRNAs, |log2FC| >0.5; lncRNAs, |log2FC| >0.5 (P<0.05 in all cases). Subsequently, the intersection of differentially expressed mRNA, miRNAs, and lncRNAs was performed to obtain more accurate results. The results of the analysis of DEGs were presented using heat, volcano, and Circos maps to show the chromosomal position of DEGs and correlations of their expression levels.
Functional analysis of differentially expressed mRNAs
Functional annotation analysis using Gene Ontology (GO) is a widely employed technique for conducting extensive studies on gene functional enrichment, encompassing molecular function (MF), BP, and cellular component (CC) (22). The Kyoto Encyclopedia of Genes and Genomes (KEGG) is a widely used database that contains comprehensive information on genomes, biological pathways, diseases, and drugs (23). The clusterProfiler package was used for GO and KEGG enrichment analyses of DEGs and effects were considered statistically significant if the adjusted P value <0.05. In addition, this package was used to further analyze the GO and KEGG enrichment of key mRNA molecules in the ceRNA network and predict the potential regulatory functions of ceRNA networks.
Construction of the protein-protein interaction (PPI) network
The PPI network analysis of the differentially expressed RNAs was conducted using the STRING database. Cytoscape is an open-source bioinformatics software that facilitates visualization of molecular interaction networks (24). We visualized the PPI network and hub genes using Cytoscape (ver. 3.8.0) and CytoHubba plug-in (25). Hub genes were screened according to the maximum correlation standard.
ceRNA regulatory network construction and functional analysis
To delve deeper into the potential correlations between mRNAs and different types of ncRNAs, a ceRNA regulatory network was created. First, to predict the interactions between miRNAs and mRNAs, we used TargetScan (http://www.targetscan.org) (26), miRTarBase (https://mirtarbase.cuhk.edu.cn/) (27), and miRDB (http://www.mirdb.org/) (28). The interaction between lncRNA and miRNA was predicted using the miRcode tool (http://www.mircode.org/), which is an extensive resource of putative miRNA target sites in the long non-coding transcriptome (29). Cytoscape 3.8.0 software was utilized to construct and visualize the ceRNA network and its core network to build and display the lncRNA-miRNA-mRNA network (25). The ggplot2 package was used to generate a Sankey diagram that visualized the regulatory relationships between lncRNAs, miRNAs, and mRNA.
Gene set enrichment analysis (GSEA)
To determine the impact of a predefined set of genes on the phenotype, the GSEA was used to evaluate their distribution trend in the order of phenotypic relevance (30). Using the GSEA, we examined the pathways and employed clusterProfiler to identify each operational cluster (31). Our cut-off thresholds included a false discovery rate <0.1 and P<0.05.
Cell culture
The NPC cell line C666 and nasopharyngeal epithelial cell line NP69 (Guangxi Medical University, Nanning, China) were cultured in RPMI-1640 medium (Asia-Vector, Shanghai, China) containing 10% fetal bovine serum (Asia-Vector) and 1% penicillin/streptomycin (Asia-Vector). The cells were cultured in an incubator at 37 ℃ in an atmosphere of 95% air and 5% CO2. After adherent growth was achieved, trypsin was used to detach the cells. Only cells in the logarithmic growth phase were chosen for further analysis.
Quantitative real-time polymerase chain reaction (qRT-PCR)
RNA was extracted according to the TRIzol reagent protocol (Invitrogen, Carlsbad, CA, USA) and reverse-transcribed into complementary DNA (cDNA) using the Fast Quant RT Kit (TaKaRa, Otsu, Shiga, Japan). This cDNA served as template for the qRT-PCR, which was performed using the Roche Light Cycler® 480 System and Light Cycler® 480 SYBR Green I Master Mix (Roche, Pleasanton, CA, USA). The experimental data were normalized to the expression level of the GAPDH gene and presented as 2−ΔΔCt values. The primer information is shown in Table 2.
Table 2
RNA | Forward | Reverse |
---|---|---|
ENST00000478301 | 5'-GCAGAAACATCTGGTCGGTT-3' | 5'-CCATTCTCTCCTGCTGCTCTA-3' |
ENST00000451496 | 5'-AAGAGCTGACACAAGAGTGGA-3' | 5'-ACCACCTACAGGTTGTGTTCT-3' |
ENST00000544214 | 5'-CGGGTCACACACACCTGATT-3' | 5'-GGGTCCTGCGGAAGTTCATT-3' |
ENST00000505694 | 5'-CATTCTGGTAGAGGGCGAACC-3' | 5'-GTGACCCTGCTGCCTTCAAC-3' |
ENST00000544214 | 5'-CGGGTCACACACACCTGATT-3' | 5'-GGGTCCTGCGGAAGTTCATT-3' |
Cell transfection
Small interfering RNAs (siRNAs) to knockdown lncRNAs were synthesized by GenePharma (Suzhou, China). Once the cells reached 50–80% confluence, they were transfected with siRNA using Lipofectamine 2000 (Invitrogen) following the manufacturer’s instructions.
Cell counting kit-8 (CCK-8) assay
In 48 h after transfection, the cells were harvested using trypsin and seeded into 96-well plates at a density of 1,000 cells per well. Cell proliferation was assessed using the CCK-8 assay (Dojindo, Kumamoto, Japan) by measuring the absorbance at 450 nm at 0, 12, 24, 48, and 72 h after reseeding, according to the manufacturer’s specifications.
Statistical analysis
The experimental data were statistically analyzed using SPSS (version 26.0; IBM, Armonk, NY, USA) and GraphPad Prism 5 software (GraphPad, La Jolla, CA, USA). Differences between groups were determined using the two-tailed Student’s t-test. Pearson’s correlation analysis was used to explore the correlation between lncRNAs and miRNAs. Effects were considered statistically significant if P<0.05.
Results
Data collection and preprocessing
To identify DEGs in NPC samples, we used the limma package to analyze mRNA, lncRNA, and miRNA expression profiles. We found that 3,510 mRNAs, 16,520 lncRNAs, and 323 miRNAs were expressed at significantly different levels (|log2FC| ≥1, adjusted P<0.01) in the NPC and normal tissues. Our analysis showed that dataset GSE12452 contained 2,493 differentially expressed mRNAs, including 1,265 upregulated and 1,228 downregulated mRNAs. Dataset GSE13597 contained 1,017 differentially expressed mRNAs, including 565 upregulated and 452 downregulated mRNAs. Classification heatmaps and volcano plots of DEGs were drawn using data grouping (Figure 2A-2D). Dataset GSE43039 contained 199 differentially expressed miRNAs, including 96 upregulated and 103 downregulated molecules. A total of 124 differentially expressed miRNAs were obtained from dataset GSE70970, including 70 upregulated and 54 downregulated miRNAs (Figure 2E-2H). In dataset GSE95166, we found 9,193 differentially expressed lncRNAs, including 4,547 upregulated and 4,646 downregulated lncRNAs. In total, 7,327 differentially expressed lncRNAs were identified in dataset GSE126683, including 3,891 upregulated and 3,436 downregulated lncRNAs (Figure 2I-2L).
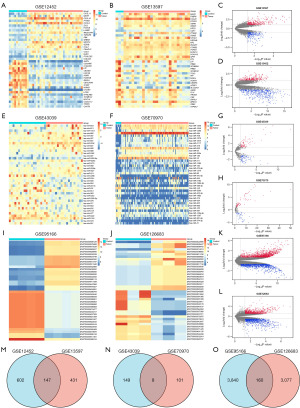
The overlapping differentially expressed RNA molecules are displayed using Venn diagrams (Figure 2M-2O). The chromosomal positions of the common differentially expressed mRNAs, miRNAs, and lncRNAs were determined using the RCircos package (Figure 3A-3C).
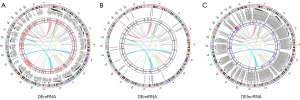
Functional enrichment analysis
To assess the functional significance and potential BPs involving proteins encoded by mRNAs from the ceRNA network, we performed GO annotation (Figure 4A-4C) and KEGG (Figure 4D) pathway enrichment analyses. Our results revealed that the DEGs were significantly enriched in both GO and KEGG terms. Specifically, GO analysis revealed a notable enrichment of the following BPs among the DEGs: nuclear division, mitotic nuclear division, and organelle fission. The chromosomal region, chromosomes, centromeric region, and kinetochore were the most significantly enriched CCs. In addition, the following MFs were enriched: tubulin binding, microtubule binding, ATPase activity, and DNA replication origin binding. According to the KEGG pathway analysis, the dysregulated mRNAs in NPC were mainly enriched in cell cycle regulation, DNA replication, and the p53 signaling pathway. A GO functional interaction network of the differentially expressed mRNAs was constructed (Figure 4E). These findings imply that chromosomal abnormalities may have a crucial impact on NPC progression.
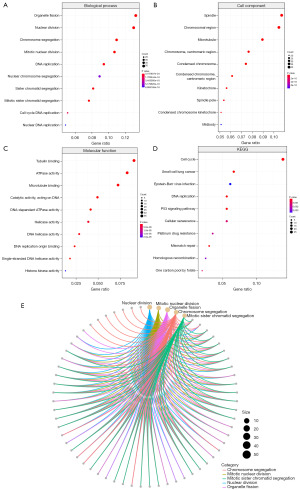
PPI network construction
To gain a deeper understanding of the interactions between mRNAs dysregulated in NPC, a PPI network was constructed using Cytoscape, which consisted of 146 nodes and 1,345 edges (Figure 5A). The distribution of node degrees in the PPI network was analyzed, and the top 30 mRNAs with the highest degrees are shown in Figure 5B. The mRNAs of the top 10 hub genes (CDK1, CDC20, NUSAP1, KIF11, KIF20A, DLGAP5, CCNA2, BUB1B, TOP2A, and ASPM) were screened from the differentially expressed mRNAs (Figure 5C). These genes may aid in exploration of the biological mechanisms underlying NPC development and reveal potential therapeutic targets.
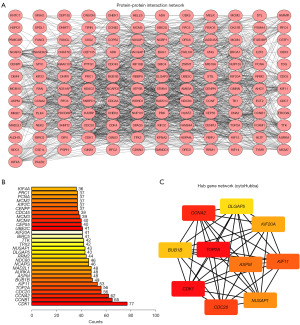
ceRNA network construction and lncRNA-mRNA co-expression analysis
To investigate the roles of various types of RNA in NPC, we analyzed correlations between lncRNA and mRNA expression levels as well as correlations between expression levels of miRNAs and those of their corresponding target mRNAs and lncRNAs (Figure 6A,6B). We found that lncRNAs such as ENST00000220514 and ENST00000301633 were significantly positively correlated with mRNAs, whereas ENST000000330915 and ENST00000332503 were significantly negatively correlated with mRNAs. Based on the expression profiles of the differentially expressed miRNAs, lncRNAs, and mRNAs in patients with NPC, we constructed a ceRNA network consisting of 7 miRNA nodes, 18 mRNA nodes, and 10 lncRNA nodes. Furthermore, we evaluated the topological significance of each gene within the ceRNA network by calculating its degree of connectivity (Figure 7A,7B).
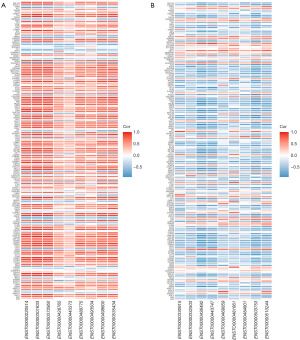
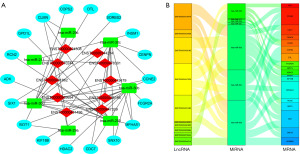
ceRNA core module screening and functional analysis
By applying the degree and maximum correlation standard algorithm in CytoHubba, we discovered five hub miRNAs, four lncRNAs, and one mRNA as a core module (Figure 8A). The ceRNA regulatory network suggested that CCNE2 may play a key role as an effector. Biological analyses were performed using clusterProfiler to examine CCNE2 protein functions. We found that CCNE2 is significantly enriched in both GO and KEGG terms (Figure 8B,8C). GO analysis revealed that CCNE2 mRNA was notably enriched in BPs such as fungiform papillary morphogenesis, tongue morphogenesis, and ectodermal placode formation. According to the KEGG analysis, CCNE2 demonstrated significant enrichment in various pathways, such as the cell cycle, neutrophil extracellular trap formation, and transcriptional misregulation in cancer. We then explored potential regulatory pathways using GSEA and found that the results of GSEA were also mainly related to cell cycle-related functions (Figure 8D-8M).
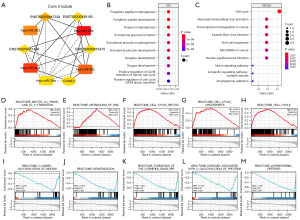
ENST00000451496 was highly expressed in C666 cells and required for their proliferation
To validate expression profiles of five lncRNAs in NPC cells, qRT-PCR was performed in C666 and NP69 cells. The C666 cells had elevated expression levels of ENST00000478301 and ENST00000451496, whereas lncRNAs ENST00000510244, ENST00000505694, and ENST00000544214 were expressed at relatively lower levels in the C666 cells compared to those in the NP69 cells. These findings suggest potential roles for ENST00000478301 and ENST00000451496 in NPC pathogenesis and warrant further investigation of their molecular mechanisms of action. To further elucidate the functional roles of these two lncRNAs, the C666 cells were transfected with corresponding siRNAs and a negative control siRNA. qPCR analysis revealed that ENST00000451496-siRNA1/4 and ENST00000478301-siRNA-3/4 had superior efficacy and were therefore selected for subsequent experiments. The CCK-8 assay indicated that ENST00000451496 knockdown led to a more pronounced inhibition of C666 cell proliferation compared to that afforded by ENST00000478301 knockdown at 12, 24, 48, and 72 h post-treatment, prompting us to focus on ENST00000451496 in the subsequent analysis (Figure 9).
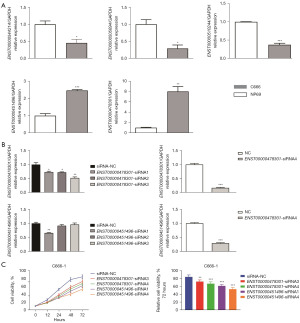
Discussion
NPC is a malignant tumor of the head and neck, and the resistance to treatment of NPC distant metastases is the major cause of patient mortality. Despite the adoption of various treatment strategies, such as radiation therapy and chemotherapy, the prognosis of NPC patients who receive these treatments remains unsatisfactory (32). Understanding the molecular mechanisms and processes that contribute to NPC is crucial for identifying novel therapeutic targets and improving clinical outcomes of patients with this disease. According to the ceRNA hypothesis, lncRNAs are considered miRNA sponges because of their ability to bind and neutralize miRNAs, thereby having a regulatory impact (33,34). Therefore, a comprehensive understanding of lncRNA-miRNA-mRNA interactions will enhance our understanding of the onset and progression of NPC.
In this study, we identified 3,510 mRNAs, 16,520 lncRNAs, and 323 miRNAs that were differentially expressed in patients with NPC. We then used these DEGs to establish a ceRNA network linked to lncRNAs, enabling us to investigate the regulatory mechanisms of ceRNAs. This ceRNA network included 20 lncRNAs, 30 miRNAs, and 30 mRNAs that were differentially expressed specifically in NPC. Among them, four lncRNAs (ENST00000438195, ENST00000415479, ENST00000451496, and ENST00000447329), one mRNA (CCNE2) and five miRNAs (hsa-miR-203, hsa-miR-30b, hsa-miR-30d, hsa-miR-30c, and hsa-miR-29c) were identified as hub genes. Among the prognostic differentially expressed lncRNAs in the ceRNA network, the lncRNA ENST00000447329 (also known as LINC01358) has the highest degree of connectivity (5). Thus, we concluded that it could have a significant impact on NPC pathogenesis. In the hub miRNAs, hsa-miR-29c plays a crucial role in NPC. In vitro experiments showed that the overexpression of hsa-miR-29c inhibited the migration and invasion of NPC cells (35). Decreased miR-29c expression is associated with increased resistance to therapy in patients with NPC. This is because miR-29c inhibits expression of the anti-apoptotic factors Mcl-1 and Bcl-2, leading to an increased sensitivity of NPC cells to ionizing radiation and cisplatin treatment (36). Additionally, according to Yu et al., the upregulation of miR-203 inhibits the G1/S transition in cells infected with Epstein-Barr virus and hinders tumor growth in vivo, suggesting that miR-203 could serve as a potential biomarker for the diagnosis and treatment of Epstein-Barr virus-associated NPC (37). Based on our findings, we hypothesized that the hsa-miR-203 and hsa-miR-29c axes play significant roles in the development of NPC and could serve as promising targets for therapeutic interventions in this cancer.
Among the prognostic DEGs, CCNE2 has the highest degree of connectivity (4) among other differentially expressed mRNAs. CCNE2 binds to and activates CDK2, forming a kinase complex that participates in the G1/S phase transition, thereby regulating cell proliferation and tumor development (38-40). According to a study by Xie et al. performed on 308 samples of ovarian cancer tissues, high CCNE2 expression is linked to reduced overall survival (41). Similarly, a study of prostate cancer has indicated that CCNE2 is substantially upregulated in patients with this condition and is regarded as a protein that promotes tumor growth (42). Our GO analysis showed that differentially expressed mRNAs were mainly associated with the morphogenesis of the fungiform papilla, tongue, and ectodermal placode. The KEGG pathway analysis demonstrated that the differentially expressed mRNAs were significantly enriched in several pathways, including the cell cycle, formation of neutrophil extracellular traps, transcriptional misregulation in cancer, Epstein-Barr virus infection, and viral carcinogenesis, which are all closely associated with cancer development. This finding not only indicates the reliability of the constructed ceRNA regulatory network but also supports the necessity of studying lncRNAs as ceRNA regulators, which could serve as therapeutic targets in NPC.
Although we developed an NPC-specific ceRNA regulatory network and identified potential prognostic biomarkers, our study had several limitations. First, we relied solely on gene expression data obtained from the GEO database. Further prospective studies involving larger patient populations from different centers are required to validate our results. Second, the bioinformatics analysis results require extensive experimental verification before they can be implemented in clinical practice. Future research should focus on the functional and mechanistic validation of genes dysregulated in NPC to ensure practical application of our findings.
Conclusions
In this study, we constructed a novel ceRNA network that provided several mechanistic clues regarding the interactions between genes dysregulated in NPC. Our results not only provide theoretical support for revealing the regulatory relationship between lncRNAs, miRNAs, and mRNAs in NPC but also offer a more substantiated approach for exploring clinical diagnostic markers and potential therapeutic targets in NPC. Furthermore, our study provides a direction for subsequent basic validation and clinical testing of novel NPC treatments, which could allow us to perform more targeted scientific trials, thereby sparing valuable research resources.
Acknowledgments
Funding: This work was supported by
Footnote
Reporting Checklist: The authors have completed the MDAR reporting checklist. Available at https://tcr.amegroups.com/article/view/10.21037/tcr-24-263/rc
Data Sharing Statement: Available at https://tcr.amegroups.com/article/view/10.21037/tcr-24-263/dss
Peer Review File: Available at https://tcr.amegroups.com/article/view/10.21037/tcr-24-263/prf
Conflicts of Interest: All authors have completed the ICMJE uniform disclosure form (available at https://tcr.amegroups.com/article/view/10.21037/tcr-24-263/coif). The authors have no conflicts of interest to declare.
Ethical Statement: The authors are accountable for all aspects of the work in ensuring that questions related to the accuracy or integrity of any part of the work are appropriately investigated and resolved. This study was conducted in accordance with the Declaration of Helsinki (as revised in 2013).
Open Access Statement: This is an Open Access article distributed in accordance with the Creative Commons Attribution-NonCommercial-NoDerivs 4.0 International License (CC BY-NC-ND 4.0), which permits the non-commercial replication and distribution of the article with the strict proviso that no changes or edits are made and the original work is properly cited (including links to both the formal publication through the relevant DOI and the license). See: https://creativecommons.org/licenses/by-nc-nd/4.0/.
References
- Chua MLK, Wee JTS, Hui EP, et al. Nasopharyngeal carcinoma. Lancet 2016;387:1012-24. [Crossref] [PubMed]
- Sung H, Ferlay J, Siegel RL, et al. Global Cancer Statistics 2020: GLOBOCAN Estimates of Incidence and Mortality Worldwide for 36 Cancers in 185 Countries. CA Cancer J Clin 2021;71:209-49. [Crossref] [PubMed]
- Chang ET, Ye W, Zeng YX, et al. The Evolving Epidemiology of Nasopharyngeal Carcinoma. Cancer Epidemiol Biomarkers Prev 2021;30:1035-47. [Crossref] [PubMed]
- Niu Y, Zhang F, Chen D, et al. A comparison of Chinese multicenter breast cancer database and SEER database. Sci Rep 2022;12:10395. [Crossref] [PubMed]
- Chen YP, Chan ATC, Le QT, et al. Nasopharyngeal carcinoma. Lancet 2019;394:64-80. [Crossref] [PubMed]
- Prawira A, Oosting SF, Chen TW, et al. Systemic therapies for recurrent or metastatic nasopharyngeal carcinoma: a systematic review. Br J Cancer 2017;117:1743-52. [Crossref] [PubMed]
- Behzadi P, Ranjbar R. DNA microarray technology and bioinformatic web services. Acta Microbiol Immunol Hung 2019;66:19-30. [Crossref] [PubMed]
- Li L, Zhang F. Novel long noncoding RNA LINC01385 promotes nasopharyngeal carcinoma proliferation via the miR-140-3p/Twist1 signaling pathway. Cell Cycle 2020;19:1352-62. [Crossref] [PubMed]
- Ng A, Tang JP, Goh CH, et al. Regulation of the H19 imprinting gene expression in human nasopharyngeal carcinoma by methylation. Int J Cancer 2003;104:179-87. [Crossref] [PubMed]
- Zheng ZQ, Li ZX, Zhou GQ, et al. Long Noncoding RNA FAM225A Promotes Nasopharyngeal Carcinoma Tumorigenesis and Metastasis by Acting as ceRNA to Sponge miR-590-3p/miR-1275 and Upregulate ITGB3. Cancer Res 2019;79:4612-26. [Crossref] [PubMed]
- Salmena L, Poliseno L, Tay Y, et al. A ceRNA hypothesis: the Rosetta Stone of a hidden RNA language? Cell 2011;146:353-8. [Crossref] [PubMed]
- Ebert MS, Neilson JR, Sharp PA. MicroRNA sponges: competitive inhibitors of small RNAs in mammalian cells. Nat Methods 2007;4:721-6. [Crossref] [PubMed]
- Poliseno L, Salmena L, Zhang J, et al. A coding-independent function of gene and pseudogene mRNAs regulates tumour biology. Nature 2010;465:1033-8. [Crossref] [PubMed]
- Wang J, Liu X, Wu H, et al. CREB up-regulates long non-coding RNA, HULC expression through interaction with microRNA-372 in liver cancer. Nucleic Acids Res 2010;38:5366-83. [Crossref] [PubMed]
- Davis S, Meltzer PS. GEOquery: a bridge between the Gene Expression Omnibus (GEO) and BioConductor. Bioinformatics 2007;23:1846-7. [Crossref] [PubMed]
- Dodd LE, Sengupta S, Chen IH, et al. Genes involved in DNA repair and nitrosamine metabolism and those located on chromosome 14q32 are dysregulated in nasopharyngeal carcinoma. Cancer Epidemiol Biomarkers Prev 2006;15:2216-25. [Crossref] [PubMed]
- Bose S, Yap LF, Fung M, et al. The ATM tumour suppressor gene is down-regulated in EBV-associated nasopharyngeal carcinoma. J Pathol 2009;217:345-52. [Crossref] [PubMed]
- Lyu X, Fang W, Cai L, et al. TGFβR2 is a major target of miR-93 in nasopharyngeal carcinoma aggressiveness. Mol Cancer 2014;13:51. [Crossref] [PubMed]
- Bruce JP, Hui AB, Shi W, et al. Identification of a microRNA signature associated with risk of distant metastasis in nasopharyngeal carcinoma. Oncotarget 2015;6:4537-50. [Crossref] [PubMed]
- Xu Y, Huang X, Ye W, et al. Comprehensive analysis of key genes associated with ceRNA networks in nasopharyngeal carcinoma based on bioinformatics analysis. Cancer Cell Int 2020;20:408. [Crossref] [PubMed]
- Ritchie ME, Phipson B, Wu D, et al. limma powers differential expression analyses for RNA-sequencing and microarray studies. Nucleic Acids Res 2015;43:e47. [Crossref] [PubMed]
- Ashburner M, Ball CA, Blake JA, et al. Gene ontology: tool for the unification of biology. The Gene Ontology Consortium. Nat Genet 2000;25:25-9. [Crossref] [PubMed]
- Ogata H, Goto S, Sato K, et al. KEGG: Kyoto Encyclopedia of Genes and Genomes. Nucleic Acids Res 1999;27:29-34. [Crossref] [PubMed]
- Shannon P, Markiel A, Ozier O, et al. Cytoscape: a software environment for integrated models of biomolecular interaction networks. Genome Res 2003;13:2498-504. [Crossref] [PubMed]
- Assenov Y, Ramírez F, Schelhorn SE, et al. Computing topological parameters of biological networks. Bioinformatics 2008;24:282-4. [Crossref] [PubMed]
- Agarwal V, Bell GW, Nam JW, et al. Predicting effective microRNA target sites in mammalian mRNAs. Elife 2015;4:e05005. [Crossref] [PubMed]
- Huang HY, Lin YC, Li J, et al. miRTarBase 2020: updates to the experimentally validated microRNA-target interaction database. Nucleic Acids Res 2020;48:D148-54. [PubMed]
- Chen Y, Wang X. miRDB: an online database for prediction of functional microRNA targets. Nucleic Acids Res 2020;48:D127-31. [Crossref] [PubMed]
- Jeggari A, Marks DS, Larsson E. miRcode: a map of putative microRNA target sites in the long non-coding transcriptome. Bioinformatics 2012;28:2062-3. [Crossref] [PubMed]
- Subramanian A, Tamayo P, Mootha VK, et al. Gene set enrichment analysis: a knowledge-based approach for interpreting genome-wide expression profiles. Proc Natl Acad Sci U S A 2005;102:15545-50. [Crossref] [PubMed]
- Yu G, Wang LG, Han Y, et al. clusterProfiler: an R package for comparing biological themes among gene clusters. OMICS 2012;16:284-7. [Crossref] [PubMed]
- Lee HM, Okuda KS, González FE, et al. Current Perspectives on Nasopharyngeal Carcinoma. Adv Exp Med Biol 2019;1164:11-34. [Crossref] [PubMed]
- Tan JY, Sirey T, Honti F, et al. Extensive microRNA-mediated crosstalk between lncRNAs and mRNAs in mouse embryonic stem cells. Genome Res 2015;25:655-66. [Crossref] [PubMed]
- Duan X, Wu Y, Zhang Z, et al. Identification and analysis of dysregulated lncRNA and associated ceRNA in the pathogenesis of keloid. Ann Transl Med 2020;8:222. [Crossref] [PubMed]
- Liu N, Tang LL, Sun Y, et al. MiR-29c suppresses invasion and metastasis by targeting TIAM1 in nasopharyngeal carcinoma. Cancer Lett 2013;329:181-8. [Crossref] [PubMed]
- Zhang JX, Qian D, Wang FW, et al. MicroRNA-29c enhances the sensitivities of human nasopharyngeal carcinoma to cisplatin-based chemotherapy and radiotherapy. Cancer Lett 2013;329:91-8. [Crossref] [PubMed]
- Yu H, Lu J, Zuo L, et al. Epstein-Barr virus downregulates microRNA 203 through the oncoprotein latent membrane protein 1: a contribution to increased tumor incidence in epithelial cells. J Virol 2012;86:3088-99. [Crossref] [PubMed]
- Lauper N, Beck AR, Cariou S, et al. Cyclin E2: a novel CDK2 partner in the late G1 and S phases of the mammalian cell cycle. Oncogene 1998;17:2637-43. [Crossref] [PubMed]
- Gudas JM, Payton M, Thukral S, et al. Cyclin E2, a novel G1 cyclin that binds Cdk2 and is aberrantly expressed in human cancers. Mol Cell Biol 1999;19:612-22. [Crossref] [PubMed]
- Aleem E, Kiyokawa H, Kaldis P. Cdc2-cyclin E complexes regulate the G1/S phase transition. Nat Cell Biol 2005;7:831-6. [Crossref] [PubMed]
- Xie L, Li T, Yang LH. E2F2 induces MCM4, CCNE2 and WHSC1 upregulation in ovarian cancer and predicts poor overall survival. Eur Rev Med Pharmacol Sci 2017;21:2150-6. [PubMed]
- Liu B, Qian D, Zhou W, et al. A Novel Androgen-Induced lncRNA FAM83H-AS1 Promotes Prostate Cancer Progression via the miR-15a/CCNE2 Axis. Front Oncol 2021;10:620306. [Crossref] [PubMed]