Predictive model of gene expression regulating invasion and migration of M2 macrophages in breast cancer: clinical prognosis and therapeutic implications
Highlight box
Key findings
• This study identified 14 prognosis-related genes by integrating differential genes associated with the invasion and metastasis of breast cancer (BRCA) and genes related to M2 macrophages. We constructed a prognosis model, which holds significant clinical value in assessing prognosis and making treatment decisions for BRCA.
What is known and what is new?
• Macrophages can promote the migration and invasion of BRCA cells, thereby facilitating the metastasis of BRCA. Therefore, it is crucial to find the targets through which they exert their effects.
• We have identified differentially expressed genes associated with BRCA invasion and migration as well as M2 macrophages, which may serve as potential targets in the process of tumor metastasis and invasion.
What is the implication, and what should change now?
• This research can assist doctors in conducting better clinical prognostic assessments, facilitate the development of novel immunotherapy strategies, and guide drug selection through the analysis of drug sensitivity.
• Further research is needed to elucidate the role of immune cells, especially macrophages, in BRCA.
Introduction
In women, the prevalence of breast cancer (BRCA) can reach 24.2%, which has surpassed lung cancer to become the highest incidence of malignant tumor in female population, and is one of the top cancer-related deaths in female population (1,2). Unchecked multiplication of abnormal cells in the breast tissue is one of the characteristics of BRCA (3). As a complex and heterogeneous disease, treatments and clinical symptoms of BRCA vary widely. Understanding of the biology of BRCA has changed over time and more and more people have recognized that tumor progression and available treatment options are significantly influenced by the tumor microenvironment (TME) (4).
Tumor cells are surrounded by and interact with a variety of cellular and non-cellular elements which comprise the microenvironment of the tumor. It is significant for the development, invasion, metastasis, and genesis of tumors as well as the response to treatment (5). TME includes tumor-associated macrophages (TAMs), endothelial cells, cancer-associated fibroblasts (CAFs), immune cells, extracellular matrix (ECM), growth factors, cytokines, and other signaling molecules (6). The complex interactions among these components influence tumor behavior, angiogenesis, immune evasion, and treatment resistance. TME is a dynamic complex network that is constantly remodeled, leading to tumor heterogeneity and plasticity.
TME influences tumor occurrence and development, which creates an atmosphere that is conducive to the growth and survival of tumors (5). BRCA’s start, development, and metastasis depend on the TME (7). Making over half of the cells in the TME, TAMs are the most significant element because they can promote the invasion and migration of tumor cells (8). TAMs are often classified into two phenotypes, M1 and M2, and can display a variety of functional traits. Most people agree that M1 TAMs have the ability to stimulate inflammation and demonstrate an anti-tumor growth response, while M2 TAMs have anti-inflammatory properties and are associated with immunosuppression, promotion of tumor growth, invasion, migration, and enhancement of angiogenesis (9-11). Based on many indications that M2 TAMs may boost BRCA cells’ invasion and migration, we developed an M2 macrophage prognostic model concerning the BRCA cells’ invasion and migration.
In this work, we examined the molecular network mechanisms and prognostic models of M2 macrophages linked to BRCA invasion and migration using a variety of datasets. Clinical information and expression profiles of BRCA were obtained from a publicly accessible database [The Cancer Genome Atlas (TCGA)/Gene Expression Omnibus (GEO)]. Differential expression analysis was performed for genes associated with BRCA invasion and migration, and genes with differential expression were then chosen and an analysis of pathway enrichment was carried out. M2 macrophages in BRCA were quantified, and a prognostic model for M2 macrophages linked to invasion and migration was created using the CIBERSORT algorithm. Through univariate and multivariate studies, the model’s stability was confirmed using external datasets, and its significance to additional clinical features was evaluated. The risk score model, immunological traits, immune checkpoints, immune regulatory variables, and medication sensitivity were all analyzed to see whether there were any relationships. Furthermore, patients were divided into high- and low-risk groups and had their immunotherapy responses predicted. The main steps of this study are outlined in Figure 1. We present this article in accordance with the TRIPOD reporting checklist (available at https://tcr.amegroups.com/article/view/10.21037/tcr-24-29/rc).
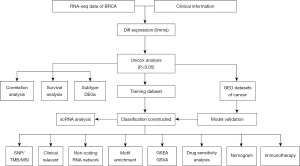
Methods
Data acquisition
The Series Matrix File of GSE20685 was retrieved from the National Center for Biotechnology Information (NCBI) GEO public database, and the annotation platform used was GPL570. For a total of 327 BRCA patients, an extensive expression profile and survival information were obtained. There were two groups: 190 people with lymph node metastases and 137 people with lymph node non-metastasis. There were for distant metastases (n=319) or distant metastases (n=8). To find the differential genes between the two groups, the limma program was utilized; P value <0.05 & |log2fold change (FC)| >0.585 were the screening conditions for differential genes. The TCGA database (https://portal.gdc.cancer.gov/), the greatest source of information on cancer genes, includes details on DNA methylation, single nucleotide polymorphisms (SNPs), copy number variation, gene expression, and microRNA (miRNA) expression, among other things. It was possible to retrieve the original, processed BRCA messenger RNA (mRNA) expression data, which comprised the tumor group (n=1,118) and the control group (n=113). The Series Matrix File data file for GSE58812 was acquired, and the annotation platform utilized was GPL570. Complete expression profiles and survival data for 107 BRCA patient records were obtained for the external validation dataset used in the subsequent model formulation. The study was conducted in accordance with the Declaration of Helsinki (as revised in 2013).
Function analysis of Gene Ontology (GO) and Kyoto Encyclopedia of Genes and Genomes (KEGG)
To completely investigate the functional connection between these genes and the variations, functional annotation of differential genes was done using the R program “ClusterProfiler”. The relevant functional categories were assessed using the GO and the KEGG. Pathways having P and q values less than 0.05 were considered to be significant GO and KEGG enrichment pathways.
Model construction and prognosis
After the prognostic-related genes were chosen, the prognostic-related model was further built using the least absolute shrinkage and selection operator (LASSO) regression. The expression values of each individual gene were taken into consideration while creating a risk score algorithm for each patient. The expected regression coefficient from the LASSO regression analysis was then used to weigh this formula. Using the risk scoring system as a guide, the patients were divided into high- and low-risk groups, with the median risk score being the divisional point. The study used Kaplan-Meier and log-rank statistical approaches to assess and compare the variations in survival between the two groups. This study used both stratified and LASSO regression analysis to examine how risk score affects patient prognosis prediction. The receiver operating characteristic (ROC) curve was used to assess the model’s prediction accuracy.
Analysis of immune cell infiltration
A popular technique for assessing immune cell types in a microenvironment is the CIBERSORT approach. The support vector regression method was used to deconvolutionally analyze the expression matrix of the immune cell subtypes. Twenty-two phenotypes of human immune cells, including T cells, B cells, plasma cells, and subsets of myeloid cells, were identified by its 547 biomarkers. The CIBERSORT algorithm was used in this study to evaluate patient data and calculate the relative abundance of 22 distinct immune infiltrating cell types. The content of immune cells and gene expression were then linked.
Drug sensitivity analysis
We utilized a genomes database based on the most common drug (the GDSC cancer drugs sensitivity genomics database; https://www.cancerrxgene.org/) in conjunction with the R software package “pRRophetic” to forecast each tumor sample’s reaction to chemotherapy. Using the genomics of drug sensitivity in cancer (GDSC) training set, ten cross-validations were conducted to confirm the accuracy of the regression and prediction of the half maximal inhibitory concentration (IC50) estimations for each specific chemotherapeutic agent. Every setting, including the elimination of batch effects and the “combat” option for averaging repetitive gene expression, was left to its default state.
Gene set variation analysis (GSVA)
To evaluate transcriptome gene set enrichment, GSVA, an unsupervised, nonparametric method, was employed. GSVA translated gene-level alterations into pathway-level modifications using a comprehensive grading system for the gene set of interest. The biological function of the sample was then evaluated. Gene sets were obtained for this study from the Molecular Signatures database (v7.0 version). Each gene set was thoroughly assessed using the GSVA algorithm to determine potential biological functional variations in different samples.
Gene set enrichment analysis (GSEA)
After the patients were categorized into high- and low-risk groups by the model, differences in their signaling pathways were further investigated using GSEA. As background gene sets, annotated gene sets of subtype pathways were obtained from the MsigDB database. Differential expression analysis was used to examine pathways across subtypes, and gene sets that showed substantial enrichment (adjusted P value less than 0.05) were ranked according to consistency scores. Exploring the close relationship between tumor type and biological importance is a common use of GSEA analysis.
Nomogram model construction
Regression analysis is the foundation of the nomogram. It is predicated on the model’s risk score and clinical symptoms. To illustrate how the variables in the prediction model related to one another, scaled line segments were drawn on the same plane in a certain scale. A multi-factor regression model was created, and the predicted value was obtained by adding the total score of all the influencing factors to the magnitude of the regression coefficients, which indicated the extent to which each model impact factor affected the end variable.
Regulatory network analysis of key genes
The R package “RcisTarget” was applied in this research to forecast transcription factors. RcisTarget bases all of its computations on motif. The total number of motifs in the database determines the normalized enrichment score (NES) for motifs. We deduced additional annotated files based on gene sequences and motif similarities, in addition to the themes the original data annotated. The area under the curve (AUC) for each pair of motif-motif pairs is the first step in measuring the overexpression of each motif on the gene set. The recovery curve of the sequence is used to ascertain this based on the gene set. The sequence’s recovery curve is utilized to ascertain this based on the gene set. based on the gene set’s overall motifs’ AUC distribution, the NES for each motif was computed. For the Gene-motif rankings database, we utilize rcistarge.hg19.motifdb.cisbpont.500bp database.
Statistical analysis
The Kaplan-Meier method was utilized to produce survival curves, and log-rank was utilized as a comparison. For multivariate analysis, the Cox proportional risk model was employed. For all statistical studies, R language version 4.2 was utilized, with P<0.05 denoting statistical significance.
Results
Differential expression analysis related to invasion and migration of BRCA
The annotation platform was GPL570 and GSE20685’s Series Matrix File was acquired via the NCBI GEO public database. A comprehensive expression profile and survival data were retrieved for a total of 327 BRCA individuals. There were two groups: lymph node metastasis (n=190) and lymph node non-metastasis (n=137). The limma program was used to identify the genes that differed between the two groups (n=319) and the distant metastasis group (n=8), respectively, with P value <0.05 & |log2FC| >0.585. The non-metastatic lymph node group and the lymph node metastasis group were examined for a total of 38 differential genes, of which 28 were up-regulated. Ten genes that were down-regulated were identified (Figure 2A,2B); 548 up-regulated and 503 down-regulated genes were among the 1,051 differential genes that were screened in both the distant transfer group and the non-distant transfer group (Figure 2C,2D).
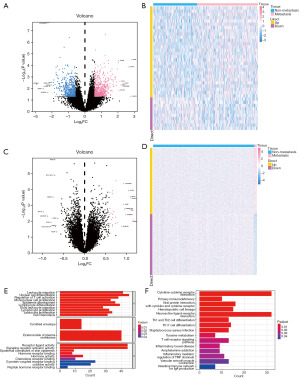
Functional enrichment of differential genes and acquisition of prognostic-related genes
There were 1,002 differential genes obtained by combining the two groups of differential genes. They were then examined using GO and KEGG enrichment analysis. The regulation of T cell activation pathways and mononuclear cell differentiation were the two primary areas of enrichment for differential genes, according to the GO data (Figure 2E). According to KEGG data, the majority of the enriched pathways were associated with Th17 cell development and cytokine-cytokine receptor interaction (Figure 2F). We used the CIBERSORT algorithm to quantify M2 macrophages and looked into the co-expression network of these macrophages according to the expression profiles of BRCA patients. There was a 0.3 correlation coefficient and a 0.05 P value.
It was discovered that the expression of macrophages M2 was substantially correlated with 293 different genes. Then, we intersected 1,002 differential genes with these 293 genes to obtain 85 intersection genes in total (Figure 3A). We employed the TCGA database to gather clinical data on BRCA patients and Cox univariate regression to screen prognostic genes in BRCA in order to further find prognostic genes in intersection genes. Based on Cox univariate regression (P value <0.05), 37 prognostic genes were chosen, according to the results (Figure 3B).
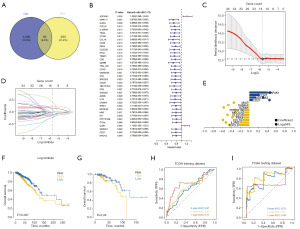
Prognostic model was constructed by prognostic-related genes
We gathered clinical data from patients with BRCA and used the LASSO regression feature selection technique to filter out the genes that are indicative of BRCA (Figure 3C-3E). This made it possible for us to pinpoint the crucial genes in the prognostic gene list in more detail.
The patients were divided into training and validation sets at random in a 4:1 ratio. Following LASSO regression analysis, for each sample, the ideal risk score value was found in order to facilitate additional analysis [risk score = CXCL9 × (−0.231546665) + IL2RG × (−0.222383446) + CCL19 × (−0.157240118) + PBX4 × (−0.135133863) + CLEC2D × (−0.119494616) + MCOLN2 × (−0.10589281) + PPP1R16B × (−0.103951182) + GZMM × (−0.025098373) + CAMK4 × (−0.022468457) + MIAT × (−0.020804683) + CD27 × 0.078044303 + ADORA3 × 0.151317409 + CCR7 × 0.22096329 + MAP4K1 × 0.414214868]. Based on risk scores, patients were categorized into high- and low-risk groups, and Kaplan-Meier curve analysis was performed. In comparison to the low-risk group, the high-risk group’s OS was much lower in both the training and test sets (Figure 3F,3G). Furthermore, the training and test sets’ ROC curve findings showed that the model had a high validation efficiency (Figure 3H,3I).
External dataset verified the robustness of the prognostic model
The GEO database (GSE58812) provided the survival data for BRCA patients, which were downloaded. The model was then used to forecast the BRCA patients’ clinical categorization in the GEO database. Using Kaplan-Meier analysis, the survival difference between the two groups was assessed in order to assess the stability of the prediction model. The findings demonstrated that the overall survival (OS) of the high-risk group in the GEO external validation set was considerably lower than that of the low-risk group (Figure 4A). Using external datasets, ROC curve analysis was conducted on the model to confirm its correctness. The findings demonstrated the model’s strong predictive efficacy in forecasting patients’ prognoses (Figure 4B).
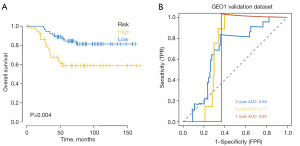
Relationship between prognostic model and immune microenvironment
After quantifying the immune cell composition using CIBERSORT, the bar plot of immune cell abundance is shown in Figure S1. By examining the association between risk scores and tumor immune infiltration percentages of immune cells in high- and low-risk groups, we further investigated the potential biological mechanisms through which risk scores promote cancer progression (Figure 5A). The results of this study, which also assessed the immune cell differences between the high- and low-risk groups, demonstrated a substantial decrease in B cells, T cells with CD8, and T cells with follicular helper within the high-risk categories. The number of resting mast cells and M2 macrophages increased noticeably (Figure 5B). Furthermore, we looked into the connection between the risk score and neutrophils, macrophages, and other cells. The outcome demonstrated a strong favorable correlation with M2 macrophages. It showed a significant inverse relationship between follicular helper cells and CD4 memory-activated T cells (Figure 5C). The chemokines, immunosuppressants, immunostimulators, immunological receptors, and other immune-related genes that differ in expression between high- and low-risk groups are displayed in Figure 6 of his study, which involved additional analysis of immunoregulatory genes.
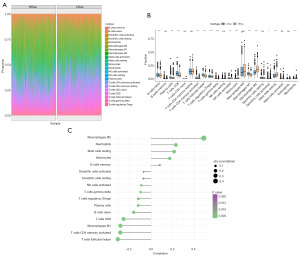
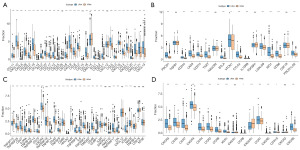
Investigating the model’s clinical prognostic value through a multi-omics research
The combined benefit of early BRCA surgery and chemotherapy treatment is evident. To ascertain each tumor sample’s sensitivity to chemotherapy, our work utilized the R packet “pRRophetic” to further assess the risk score and sensitivity of popular chemotherapy medicines based on the drug sensitivity data of the GDSC database. The findings demonstrated a significantly correlation between the risk score and the patient’s sensitivity to CCT007093, AP.24534, CEP.701, ABT.888, and roscovitine (Figure 7A). Next, we explore the possible molecular processes by which risk scores influence tumor progression by examining the particular signaling pathways that are part of the high-low risk correlation model.
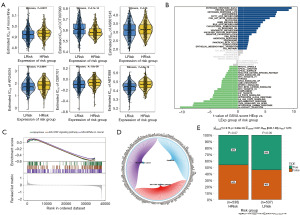
According to the GSVA results, the two groups’ distinct pathways were primarily enriched in the PI3K AKT MTOR SIGNALING and FATTY ACID METABOLISM pathways (Figure 7B). The JAK-STAT signaling pathway, miRNAs in cancer, and apoptosis were the pathways involved, according to the GSEA data (Figure 7C). Figure 7D illustrates the network of molecular interactions that exist between the pathways. The group with high risk, which did not respond well to high-expression immunotherapy, was subsequently predicted to be sensitive to anti-tumor immunotherapy (Figure 7E).
Risk and independent prognosis analysis
The samples were divided into groups according to their level of risk using the median risk score, and a column graph representing the regression analysis’s findings was displayed. The results of the logistic regression analysis indicated that risk score significantly influenced how each sample’s nomogram prediction model was scored (Figure 8A). In parallel, we performed a prediction analysis (Figure 8B-8D) for BRCA at 1 and 3 years, respectively, and discovered that the outcomes of the predictions agreed with one another. Furthermore, risk score was an independent predictor of prognosis for patients with BRCA, according to univariate and multivariate analyses (Figure 9A,9B).
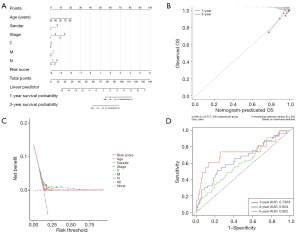
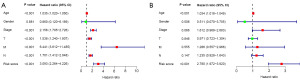
Correlation analysis between morbidity risk and multiple clinical indicators
The risk score corresponding to the sample was split into several groups based on the size of the clinical indicator value, and the outcomes of each clinical indicator group were shown as a boxplot (Figure 10). Furthermore, the rank sum test revealed that the distribution of risk score values for the clinical variables of age, gender, fustat, stage, T, N, and M differed significantly between the groups (P value <0.05). It demonstrates how well the risk score derived from modeling analysis can be used to the categorization of BRCA samples.
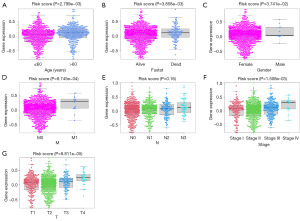
Motif analysis
When we applied model genes to the gene sets analyzed in this study, we discovered that shared regulatory mechanisms, like several transcription factors, were in place. For this reason, cumulative recovery curves were employed to improve these transcription factors (Figure 11). The analysis’s findings indicated that cisbp M2952 is the MOTIF annotation. This motif was found to be enriched in three model genes. 5.58 was the NES. We displayed every major gene’s enriched motif together with its matching transcription factor.
The recovery curve of the current motif is shown in blue, the mean value ± standard deviation (SD) is shown in green, and the mean value of the recovery curve for each of the four motifs with the highest AUC is shown in red. The largest separation (mean ± SD) between the green curve and the current motif.
Discussion
Several different molecular processes are participating in the invasion and migration of BRCA. Matrix metalloproteinases (MMPs), for instance, are secreted by BRCA cells and possess the capacity to degrade ECM, encourage the migration and invasion of additional cells (12-14). BRCA cells obtain sufficient nutrients to support their growth and migration by inducing new angiogenesis and releasing vascular endothelial growth factor (VEGF) (15). And TAMs can interact with BRCA cells to promote their invasion and migration (16). BRCA cells can exhibit invasion and migration by entering distant organs through the lymphatic system or blood circulation, forming circulating tumor cells (CTCs) (17).
In BRCA, the most prevalent immune cell components seen in tumor tissues are TAMs, and their number can reach 50% of the total number of cells in tumor tissues (18). TAMs and BRCA cells have a variety of intricate interactions. In the first place, TAMs have the potential to accelerate tumor growth, lymphatic angiogenesis, invasion and metastasis by releasing a variety of cytokines such as VEGF and regulating ECM decomposition enzymes (19,20). In the second place, BRCA cells can also recruit and activate TAMs by secreting various factors and chemicals (21).
A deeper understanding of this interaction between TAMs and BRCA cells is important for revealing the mechanisms of BRCA invasion and migration and for developing therapeutic strategies that target these processes. To gain a deeper comprehension of the relationship between M2 TAMs and BRCA cells, we built an invasion, migration, and prognosis model. We also demonstrated how crucial it is to build a prognosis model for BRCA treatment.
When immune cells are present and functioning in tumor tissue, it is referred to as immunoinfiltration. The investigation of immune infiltration in BRCA is gaining importance. The microenvironment of BRCA tumors contains several immune cell types, such as natural killer (NK) cells, dendritic cells, T cells, B cells, and macrophages (22). By identifying and eliminating tumor cells, triggering immunological reactions, and controlling inflammatory reactions, these immune cells can influence the development and growth of tumors (23).
Research has demonstrated that BRCA patients who have immune infiltration have a better prognosis. Higher levels of immune cell infiltration are often associated with lower recurrence rates, longer survival, and better treatment response (24).
Overall, immune infiltration contributes significantly to BRCA. Establishing a model of immune invasion is therefore crucial in order to better assess prognosis and uncover the connection between immune invasion and BRCA.
CCL19 and CXCL9 have been reported to be potential prognostic biomarkers of BRCA (25,26). In BRCA, CXCL9 can migrate immune cells to BRCA tissues. Through the chemotaxis of immune cells like T cells and NK cells. At the same time, CXCL9 has a role in controlling the BRCA TME, impacting how surrounding tissues and cells interact with tumor cells. Petty et al.’s study showed that CXCL9 is a ligand of CXCR3 and important for the recruitment of CD8-positive T cells (27). The human IL2RG chain gene, or IL2RG is an essential factor in the onset and progression of BRCA. IL2RG may influence the development of BRCA by affecting the function of immune cells and immune regulatory pathways, as it is associated with immune system function and regulation (28). The expression level of IL2RG in BRCA tissue may be associated with the malignancy and prognosis of the tumor (29). CCR7 is a chemokine receptor that is often expressed at elevated levels in BRCA tissues. The migration of BRCA cells to the distant location is considered to be one of the most significant stages in their metastasis, which is facilitated by CCR7’s binding to its ligand CCL21 (30). The activation of CCR7 can also attract immune cells (such as dendritic cells and T lymphocytes) to migrate to BRCA tissues and enhance anti-tumor immune response, and tumor cells can also use the signaling pathway of CCR7 to evade immune surveillance and destruction, thus promoting tumor growth and metastasis (31). Therefore, tumor metastasis and the immune system are two areas in which CCR7 is crucial. CCR7 has also been reported to serve as potential prognostic biomarkers of gastric cancer respectively (32). Mitogen-activated protein kinase 1, or MAP4K1, is a type of kinase that is a part of the MAPK signaling pathway (33). MAP4K1 regulates cell growth by activating cell cycle regulators and apoptosis-inhibiting pathways. Secondly, MAP4K1 contributes to the growth of BRCA by limiting the capacity of BRCA cells to migrate and invade (34). In addition, MAP4K1 is also associated with the stimulation of intracellular pathways, including the epithelial-mesenchymal transition and the Wnt/beta-catenin pathway (35). MAP4K1 has also been reported to serve as potential prognostic biomarkers of acute myeloid leukemia (AML) respectively (36).
BRCA is thought to be caused and progressed by the PI3K/AKT/mTOR signaling pathway, the p53 signaling pathway, and fatty acid metabolism. Energy balance and cell survival are significantly impacted by fatty acid metabolism in the course of BRCA development. Increased synthesis and buildup of generated unsaturated (like oleic acid) and saturated (like stearic acid) fatty acids might result from abnormal fatty acid metabolism. It has been discovered that these metabolites encourage the development and invasion of BRCA cells (37). The regulation of metabolism, cell growth, and survival are all significantly impacted by the PI3K/AKT/mTOR signaling pathway. The signaling pathway PI3K/AKT/mTOR is frequently aberrantly activated in BRCA and can stimulate the growth, invasion, and angiogenesis of tumor cells (38). The tumor suppressor gene p53 is significant because it regulates DNA damage and aberrant cell growth. In BRCA, mutations and loss of function of p53 are very common, disrupting normal cell cycle regulation and DNA repair processes. This may lead to the disturbance of BRCA cell cycle, enhanced anti-apoptotic ability, infinite proliferation potential and invasion and metastasis (39). The development and response to treatment of BRCA may be significantly influenced by abnormal fatty acid metabolism, activation of the PI3K/AKT/mTOR signaling network, and the p53 signaling system. These pathways regulate crucial processes like invasion, proliferation, anti-apoptosis, and metabolism of BRCA cells.
In order to confirm the prognostic model’s accuracy, ROC curve analysis was performed on external datasets. The external GEO validation set demonstrated that the high-risk group’s OS was much lower than the low-risk group’s, and the results showed that the model had a strong precision in forecasting the prognosis of patients. The prediction model for different survival time periods in the verification set had average accuracy and diagnostic value, as seen in the training set where the AUC values in the 1-, 2-, and 3-year survival times were 0.71, 0.63, and 0.61 respectively, and were generally 0.6, which was greater than 0.5 and less than 1. The AUC values in the verification set for the 1-, 2-, and 3-year survival times were 0.69, 0.8, and 0.76, respectively. These values were generally 0.7, and since they were greater than 0.5 and less than 1, they suggest that the prediction model performs well in the training set’s various survival times. They also suggested that the diagnostic value of the model was good. It is discovered that although the prediction model’s accuracy was generally higher, it performed better in the verification training set than it did in the training set.
We constructed a drug sensitivity model for several common antitumor drugs. The higher the IC50, the more resistant the model, the high-risk group had a significantly higher rate of medicine resistance than the low-risk group, and the prognosis was not good. Here are the studies of these common antitumor drugs in BRCA. Roscovitine is a protein kinase inhibitor, which can cause cell apoptosis and prevent BRCA cells from proliferating and surviving (40). By meddling with the cell cycle’s regulation, it prevents the growth and multiplication of cancerous cells. Furthermore, by influencing the activity of MMPs and cell adhesion, roscovitine can lessen the propensity of BRCA to metastasize and inhibit the BRCA cells’ ability to invade and migrate (41). CCT007093 is a dual inhibitor of PI3K and mTOR. CCT007093 can decrease the cell cycle process, trigger cell apoptosis, and inhibit the PI3K/AKT/mTOR pathway to prevent BRCA cells from proliferating and surviving. Second, CCT007093 can lessen the propensity for tumor metastasis and block the capacity of BRCA cells to invade and migrate (42,43). AS601245 has the ability to suppress MAP4K1 activity, stop the cell cycle from progressing, prevent BRCA cells from proliferating and invading other cells, and lower the chance of tumor metastasis (44,45). Tyrosine kinase inhibitor AP24534 (ponatinib) impacts the invasion, metastasis, and proliferation of BRCA cells by interfering with the signaling pathways of VEGFR, PDGFR, and SRC kinase family (46-48). A multi-kinase inhibitor, CEP701 (also called lestaurtinib) mainly targets protein kinases such FLT3, JAK2, and TrkA. Through the inhibition of FLT3 receptor kinase activity, CEP701 may decrease the capacity of BRCA cells to proliferate and invade, consequently disrupting associated signaling pathways like JAK/STAT, PI3K/AKT, and RAS/RAF/MEK/ERK (49). ABT-888 (veliparib) is a PARP inhibitor, and studies on BRCA mostly concentrate on its use in patients with triple-negative, HER2-positive, and BRCA gene-mutant forms of the disease (50,51). DNA repair mechanisms are disrupted by mutations in the BRCA gene, making tumor cells more sensitive to PARP inhibitors. ABT-888 interferes with the DNA repair process by inhibiting PARP enzyme activity, causing the DNA damage of tumor cells to be unable to be repaired and resulting in cell death (52,53).
In summary, we have constructed and validated a prognostic signature containing 14 prognostic-related invasion and migration as well as M2 macrophage genes for predicting prognosis in BRCA patients. In addition, our study suggests that risk scores generated by the 14 genetic prognostic traits are separate risk factors for BRCA patients’ bad outcomes.
Conclusions
This study conducted differential expression analysis of invasion and migration-related genes in BRCA patients using the NCBI GEO and TCGA databases, identifying differential genes associated with lymph node metastasis and distant metastasis. Through GO and KEGG enrichment analysis, these genes were found to primarily participate in pathways such as monocyte differentiation, T cell activation, and cytokine-cytokine receptor interaction. Utilizing the CIBERSORT algorithm to quantify M2 macrophages in BRCA, genes associated with them were screened, and 14 prognosis-related genes were identified through a combination of differential gene analysis and Cox univariate regression analysis. A prognosis model was constructed and its robustness was validated in the GEO dataset. This model holds significant clinical value for prognosis assessment and treatment decision-making in BRCA.
Acknowledgments
We are grateful to the anonymous reviewers for their constructive comments on the original manuscript.
Funding: This work was supported by
Footnote
Reporting Checklist: The authors have completed the TRIPOD reporting checklist. Available at https://tcr.amegroups.com/article/view/10.21037/tcr-24-29/rc
Peer Review File: Available at https://tcr.amegroups.com/article/view/10.21037/tcr-24-29/prf
Conflicts of Interest: All authors have completed the ICMJE uniform disclosure form (available at https://tcr.amegroups.com/article/view/10.21037/tcr-24-29/coif). The authors have no conflicts of interest to declare.
Ethical Statement: The authors are accountable for all aspects of the work in ensuring that questions related to the accuracy or integrity of any part of the work are appropriately investigated and resolved. The study was conducted in accordance with the Declaration of Helsinki (as revised in 2013).
Open Access Statement: This is an Open Access article distributed in accordance with the Creative Commons Attribution-NonCommercial-NoDerivs 4.0 International License (CC BY-NC-ND 4.0), which permits the non-commercial replication and distribution of the article with the strict proviso that no changes or edits are made and the original work is properly cited (including links to both the formal publication through the relevant DOI and the license). See: https://creativecommons.org/licenses/by-nc-nd/4.0/.
References
- Xiao K, Li K, Long S, et al. Potential Molecular Mechanisms of Chaihu-Shugan-San in Treatment of Breast Cancer Based on Network Pharmacology. Evid Based Complement Alternat Med 2020;2020:3670309. [Crossref] [PubMed]
- Lenga L, Bernatz S, Martin SS, et al. Iodine Map Radiomics in Breast Cancer: Prediction of Metastatic Status. Cancers (Basel) 2021;13:2431. [Crossref] [PubMed]
- Liu H, Ye H. Screening of the prognostic targets for breast cancer based co-expression modules analysis. Mol Med Rep 2017;16:4038-44. [Crossref] [PubMed]
- Chalmin F, Bruchard M, Vegran F, et al. Regulation of T cell antitumor immune response by tumor induced metabolic stress. Cell Stress 2018;3:9-18. [Crossref] [PubMed]
- Yoshida A, Takata T, Kanda T, et al. Impact of endoscopic submucosal dissection and epithelial cell sheet engraftment on systemic cytokine dynamics in patients with oesophageal cancer. Sci Rep 2021;11:15282. [Crossref] [PubMed]
- Shaashua L, Mayer S, Lior C, et al. Stromal Expression of the Core Clock Gene Period 2 Is Essential for Tumor Initiation and Metastatic Colonization. Front Cell Dev Biol 2020;8:587697. [Crossref] [PubMed]
- Carron EC, Homra S, Rosenberg J, et al. Macrophages promote the progression of premalignant mammary lesions to invasive cancer. Oncotarget 2017;8:50731-46. [Crossref] [PubMed]
- Zhou J, Tang Z, Gao S, et al. Tumor-Associated Macrophages: Recent Insights and Therapies. Front Oncol 2020;10:188. [Crossref] [PubMed]
- Wang G, Chen JJ, Deng WY, et al. CTRP12 ameliorates atherosclerosis by promoting cholesterol efflux and inhibiting inflammatory response via the miR-155-5p/LXRα pathway. Cell Death Dis 2021;12:254. [Crossref] [PubMed]
- Mao Y, Chen L, Wang F, et al. Cancer cell-expressed B7-H3 regulates the differentiation of tumor-associated macrophages in human colorectal carcinoma. Oncol Lett 2017;14:6177-83. [Crossref] [PubMed]
- Aras S, Zaidi MR. TAMeless traitors: macrophages in cancer progression and metastasis. Br J Cancer 2017;117:1583-91. [Crossref] [PubMed]
- Zeng XY, Jiang XY, Yong JH, et al. lncRNA ABHD11-AS1, regulated by the EGFR pathway, contributes to the ovarian cancer tumorigenesis by epigenetically suppressing TIMP2. Cancer Med 2019;8:7074-85. [Crossref] [PubMed]
- Cheng HL, Hsieh MJ, Yang JS, et al. Nobiletin inhibits human osteosarcoma cells metastasis by blocking ERK and JNK-mediated MMPs expression. Oncotarget 2016;7:35208-23. [Crossref] [PubMed]
- Mitsiogianni M, Koutsidis G, Mavroudis N, et al. The Role of Isothiocyanates as Cancer Chemo-Preventive, Chemo-Therapeutic and Anti-Melanoma Agents. Antioxidants (Basel) 2019;8:106. [Crossref] [PubMed]
- Saoudi González N, Castet F, Élez E, et al. Current and emerging anti-angiogenic therapies in gastrointestinal and hepatobiliary cancers. Front Oncol 2022;12:1021772. [Crossref] [PubMed]
- Li X, Wang M, Gong T, et al. A S100A14-CCL2/CXCL5 signaling axis drives breast cancer metastasis. Theranostics 2020;10:5687-703. [Crossref] [PubMed]
- Tsai WS, Hung TF, Chen JY, et al. Early Detection and Dynamic Changes of Circulating Tumor Cells in Transgenic NeuN Transgenic (NTTg) Mice with Spontaneous Breast Tumor Development. Cancers (Basel) 2021;13:3294. [Crossref] [PubMed]
- Yi B, Cheng Y, Chang R, et al. Prognostic significance of tumor-associated macrophages polarization markers in lung cancer: a pooled analysis of 5105 patients. Biosci Rep 2023;43:BSR20221659. [Crossref] [PubMed]
- Zhou CH, Wan YY, Chu XH, et al. Urotensin II contributes to the formation of lung adenocarcinoma inflammatory microenvironment through the NF-κB pathway in tumor-bearing nude mice. Oncol Lett 2012;4:1259-63. [Crossref] [PubMed]
- Sotoodehnejadnematalahi F, Burke B. Human activated macrophages and hypoxia: a comprehensive review of the literature. Iran J Basic Med Sci 2014;17:820-30. [PubMed]
- Janssen LME, Ramsay EE, Logsdon CD, et al. The immune system in cancer metastasis: friend or foe? J Immunother Cancer 2017;5:79. [Crossref] [PubMed]
- Li F, Li J, Yin K, et al. CS1003, a novel human and mouse cross-reactive PD-1 monoclonal antibody for cancer therapy. Acta Pharmacol Sin 2021;42:142-8. [Crossref] [PubMed]
- Wang S, Xu X. An Immune-Related Gene Pairs Signature for Predicting Survival in Glioblastoma. Front Oncol 2021;11:564960. [Crossref] [PubMed]
- Chen H, Chong W, Yang X, et al. Age-related mutational signature negatively associated with immune activity and survival outcome in triple-negative breast cancer. Oncoimmunology 2020;9:1788252. [Crossref] [PubMed]
- Wang J, Qin D, Ye L, et al. CCL19 has potential to be a potential prognostic biomarker and a modulator of tumor immune microenvironment (TIME) of breast cancer: a comprehensive analysis based on TCGA database. Aging (Albany NY) 2022;14:4158-75. [Crossref] [PubMed]
- Liang YK, Deng ZK, Chen MT, et al. CXCL9 Is a Potential Biomarker of Immune Infiltration Associated With Favorable Prognosis in ER-Negative Breast Cancer. Front Oncol 2021;11:710286. [Crossref] [PubMed]
- Petty AJ, Li A, Wang X, et al. Hedgehog signaling promotes tumor-associated macrophage polarization to suppress intratumoral CD8+ T cell recruitment. J Clin Invest 2019;129:5151-62. [Crossref] [PubMed]
- Liu X, Jin G, Qian J, et al. Digital gene expression profiling analysis and its application in the identification of genes associated with improved response to neoadjuvant chemotherapy in breast cancer. World J Surg Oncol 2018;16:82. [Crossref] [PubMed]
- Lv W, Zhao C, Tan Y, et al. Identification of an Aging-Related Gene Signature in Predicting Prognosis and Indicating Tumor Immune Microenvironment in Breast Cancer. Front Oncol 2021;11:796555. [Crossref] [PubMed]
- Rizeq B, Malki MI. The Role of CCL21/CCR7 Chemokine Axis in Breast Cancer Progression. Cancers (Basel) 2020;12:1036. [Crossref] [PubMed]
- Aspord C, Pedroza-Gonzalez A, Gallegos M, et al. Breast cancer instructs dendritic cells to prime interleukin 13-secreting CD4+ T cells that facilitate tumor development. J Exp Med 2007;204:1037-47. [Crossref] [PubMed]
- Mashino K, Sadanaga N, Yamaguchi H, et al. Expression of chemokine receptor CCR7 is associated with lymph node metastasis of gastric carcinoma. Cancer Res 2002;62:2937-41. [PubMed]
- Ruan X, Chen T, Wang X, et al. Suxiao Jiuxin Pill protects cardiomyocytes against mitochondrial injury and alters gene expression during ischemic injury. Exp Ther Med 2017;14:3523-32. [Crossref] [PubMed]
- Yang HS, Matthews CP, Clair T, et al. Tumorigenesis suppressor Pdcd4 down-regulates mitogen-activated protein kinase kinase kinase kinase 1 expression to suppress colon carcinoma cell invasion. Mol Cell Biol 2006;26:1297-306. [Crossref] [PubMed]
- Bai Z, Yao Q, Sun Z, et al. Prognostic Value of mRNA Expression of MAP4K Family in Acute Myeloid Leukemia. Technol Cancer Res Treat 2019;18:1533033819873927. [Crossref] [PubMed]
- Knight TE, Edwards H, Taub JW, et al. MAP4K1 expression is a novel resistance mechanism and independent prognostic marker in AML-but can be overcome via targeted inhibition. EBioMedicine 2021;70:103488. [Crossref] [PubMed]
- Menendez JA, Vellon L, Colomer R, et al. Oleic acid, the main monounsaturated fatty acid of olive oil, suppresses Her-2/neu (erbB-2) expression and synergistically enhances the growth inhibitory effects of trastuzumab (Herceptin) in breast cancer cells with Her-2/neu oncogene amplification. Ann Oncol 2005;16:359-71. [Crossref] [PubMed]
- Le T, Jerel D, Bryan LJ. Update on the role of copanlisib in hematologic malignancies. Ther Adv Hematol 2021;12:20406207211006027. [Crossref] [PubMed]
- Kim MP, Zhang Y, Lozano G. Mutant p53: Multiple Mechanisms Define Biologic Activity in Cancer. Front Oncol 2015;5:249. [Crossref] [PubMed]
- Montagnoli A, Valsasina B, Croci V, et al. A Cdc7 kinase inhibitor restricts initiation of DNA replication and has antitumor activity. Nat Chem Biol 2008;4:357-65. [Crossref] [PubMed]
- Goodyear S, Sharma MC. Roscovitine regulates invasive breast cancer cell (MDA-MB231) proliferation and survival through cell cycle regulatory protein cdk5. Exp Mol Pathol 2007;82:25-32. [Crossref] [PubMed]
- Wang J, He G, Li H, et al. Discovery of novel PARP/PI3K dual inhibitors with high efficiency against BRCA-proficient triple negative breast cancer. Eur J Med Chem 2021;213:113054. [Crossref] [PubMed]
- Mandl MM, Zhang S, Ulrich M, et al. Inhibition of Cdk5 induces cell death of tumor-initiating cells. Br J Cancer 2017;116:912-22. [Crossref] [PubMed]
- Kort A, van Hoppe S, Sparidans RW, et al. Brain Accumulation of Ponatinib and Its Active Metabolite, N-Desmethyl Ponatinib, Is Limited by P-Glycoprotein (P-GP/ABCB1) and Breast Cancer Resistance Protein (BCRP/ABCG2). Mol Pharm 2017;14:3258-68. [Crossref] [PubMed]
- Carboni S, Boschert U, Gaillard P, et al. AS601245, a c-Jun NH2-terminal kinase (JNK) inhibitor, reduces axon/dendrite damage and cognitive deficits after global cerebral ischaemia in gerbils. Br J Pharmacol 2008;153:157-63. [Crossref] [PubMed]
- Roskoski R Jr. Src protein-tyrosine kinase structure, mechanism, and small molecule inhibitors. Pharmacol Res 2015;94:9-25. [Crossref] [PubMed]
- Shao W, Li S, Li L, et al. Chemical genomics reveals inhibition of breast cancer lung metastasis by Ponatinib via c-Jun. Protein Cell 2019;10:161-77. [Crossref] [PubMed]
- Gao Y, Ding Y, Tai XR, et al. Ponatinib: An update on its drug targets, therapeutic potential and safety. Biochim Biophys Acta Rev Cancer 2023;1878:188949. [Crossref] [PubMed]
- Diaz T, Navarro A, Ferrer G, et al. Lestaurtinib inhibition of the Jak/STAT signaling pathway in hodgkin lymphoma inhibits proliferation and induces apoptosis. PLoS One 2011;6:e18856. [Crossref] [PubMed]
- Isakoff SJ, Puhalla S, Domchek SM, et al. A randomized Phase II study of veliparib with temozolomide or carboplatin/paclitaxel versus placebo with carboplatin/paclitaxel in BRCA1/2 metastatic breast cancer: design and rationale. Future Oncol 2017;13:307-20. [Crossref] [PubMed]
- Lin KK, Harrell MI, Oza AM, et al. BRCA Reversion Mutations in Circulating Tumor DNA Predict Primary and Acquired Resistance to the PARP Inhibitor Rucaparib in High-Grade Ovarian Carcinoma. Cancer Discov 2019;9:210-9. [Crossref] [PubMed]
- LoRusso PM, Li J, Burger A, et al. Phase I Safety, Pharmacokinetic, and Pharmacodynamic Study of the Poly(ADP-ribose) Polymerase (PARP) Inhibitor Veliparib (ABT-888) in Combination with Irinotecan in Patients with Advanced Solid Tumors. Clin Cancer Res 2016;22:3227-37. [Crossref] [PubMed]
- Isakoff SJ, Mayer EL, He L, et al. TBCRC009: A Multicenter Phase II Clinical Trial of Platinum Monotherapy With Biomarker Assessment in Metastatic Triple-Negative Breast Cancer. J Clin Oncol 2015;33:1902-9. [Crossref] [PubMed]