Identifying epithelial-mesenchymal transition-related genes as prognostic biomarkers and therapeutic targets of hepatocellular carcinoma by integrated analysis of single-cell and bulk-RNA sequencing data
Highlight box
Key findings
• Combined analysis of single-cell RNA sequencing (scRNA-seq) and bulk-RNA sequencing (bulk RNA-seq) data revealed prognostic values of epithelial-mesenchymal transition-related genes (ERGs) in hepatocellular carcinoma patients.
What is known and what is new?
• With the advancement of microarray and high-throughput sequencing techniques, numerous prognostic markers for tumors have been reported through the utilization of Gene Expression Omnibus and The Cancer Genome Atlas databases.
• We integrate scRNA-seq and bulk RNA-seq data based on machine learning methods.
What is the implication, and what should change now?
• Eight differentially expressed ERGs (HSP90AA1, CIRBP, CCR7, S100A9, ADAM17, ENG, PGF, and INPP4B) displayed distinct immune infiltration states and showed different correlations with immune checkpoint genes. Nevertheless, performing in vivo and in vitro experiments remains a crucial next step.
Introduction
Primary liver cancer stands as one of the most prevalent and lethal malignancies worldwide, posing significant threats to human health and well-being (1). Hepatocellular carcinoma (HCC) constitutes the predominant subtype, encompassing approximately 75–85% of primary liver cancer cases (2). Despite advancements in medical sciences, early surgical intervention remains paramount for mitigating mortality among HCC patients (3). Moreover, emerging therapeutic modalities such as interventional procedures, precision therapies, and immunomodulation offer promising avenues (4). Nonetheless, HCC patients continue to confront with grim prognoses, characterized by a dismal 5-year survival rate (3). The adverse outlook in HCC stems from multifaceted factors, including the insidious onset and heterogeneous nature of tumors, posing challenges in therapeutic stratification. Hence, there is an exigent imperative to delineate potential biomarkers that can accurately prognosticate diagnostic and prognostic trajectories in HCC patients.
Epithelial-mesenchymal transition (EMT) denotes a dynamic biological phenomenon wherein epithelial cells undergo a phenotypic shift from their polarized state, acquiring mesenchymal characteristics typified by enhanced migratory capacity, invasiveness, and resistance to apoptosis (5). Within selected cohorts afflicted by cancer, malignant cells have been shown to acquire stem-like properties, relinquishing epithelial polarity, diminishing intercellular adhesion, and augmenting metastatic propensity through pathways intricately linked with EMT (6). An accumulating body of evidence underscores the intricate interplay between the EMT cascade and various regulatory elements, encompassing transcriptional modulators such as Snail, Slug, Twist, ZEB, alongside the activation of pivotal signaling cascades including Wnt/β-catenin, TGF-β/Smad, and the Hedgehog pathway (7).
Single-cell RNA sequencing (scRNA-seq) represents a groundbreaking methodology facilitating the comprehensive interrogation of gene expression patterns across the genome at the level of individual cells. This innovative approach holds immense promise in elucidating cellular heterogeneity, intracellular signaling dynamics, and the intricate interplay of cellular pathways (8). In recent years, the application of single-cell genomics has been extensively leveraged, leading to unprecedented insights into the intricacies of HCC biology and its etiological underpinnings.
In the present investigation, we harnessed scRNA-seq data to discern differentially expressed EMT-related genes (ERGs) at the cellular level, elucidate the expression profiles of differentially expressed EMT-related genes (DE-ERGs) across diverse cell clusters, and conduct in-depth analyses pertaining to cell-cell communication and cellular trajectories. By integrating bulk RNA sequencing data, we devised a prognostic model predicated on an ERG signature comprising HSP90AA1, CIRBP, CCR7, S100A9, ADAM17, ENG, PGF, and INPP4B. The robustness of our findings was substantiated through validation using datasets sourced from the International Cancer Genome Consortium (ICGC). Our study endeavors to unveil novel biomarkers and bolster the accuracy of prognostic predictions in HCC. We present this article in accordance with the TRIPOD reporting checklist (available at https://tcr.amegroups.com/article/view/10.21037/tcr-24-521/rc).
Methods
Data collection and process
This study was conducted in accordance with the Declaration of Helsinki (as revised in 2013). We extracted HCC data of 424 patients with analyzable clinical information from The Cancer Genome Atlas (TCGA) website (https://portal.gdc.cancer.gov/), and RNA-seq of HCC patients from the ICGC portal (https://docs.icgc-argo.org/docs/data-access/icgc-25k-data) was used as the validation cohort. The gene expression profiling datasets [GSE14520 (9) and GSE76427 (10)] were obtained from the Gene Expression Omnibus (GEO) database (https://www.ncbi.nlm.nih.gov/geo). ERGs were attained from the Epithelial-Mesenchymal Transition Gene Database (https://bioinfo-minzhao.org/dbemt/download.cgi) (11). scRNA-seq data acquisition and processing was carried out using datasets from GSE202642 (12) in the GEO database. Moreover, the Human Protein Atlas (HPA) (https://www.proteinatlas.org/) repository employs transcriptomic and proteomic methodologies for investigating protein expression across various tissues and organs at both RNA and protein tiers (13).
Quality control at the single-cell level and annotation of cell types
Genes within cells were chosen based on cytoplasmic control criteria, necessitating counts exceeding 200 yet not surpassing 8,000, alongside unique molecular identifiers (UMIs) surpassing 200 through utilizing the Seurat package. Furthermore, mitochondrial content was mandated to remain below 20%. Identification of highly variable genes ensued, followed by application of “SCTransform” (14) for normalization, while addressing batch effects via harmony. Dimensionality reduction techniques included uniform manifold approximation and projection (UMAP), t-distributed stochastic neighbor embedding (t-SNE), and implementation of the Louvian clustering algorithm within Seurat. The method FindAllMarkers was utilized to identify genes that differed between cell types or clusters, with parameters set at a P value below 0.05 and a log2 fold change (FC) exceeding 0.25. Furthermore, the expression ratio was required to exceed 0.1. We used signatures from previous publications as well as CellMarker for annotation (15).
Cell-cell communication
To analyze cellular communication at the molecular level, we utilized the “Cellchat R package” (16). We quantified and visually represented the count of receptor-ligand pairs.
Identification of epithelial subtypes
In order to delve deeper into the subpopulations of tumor cells in HCC, we isolated all epithelial cells from the single-cell data of HCC. Subsequently, we re-executed the process of dimensional reduction and clustering utilizing Seurat software.
Establishment and validation of the prognostic risk model
Utilizing DE-ERGs, we performed univariate Cox analysis to identify genes associated with prognosis, considering a significance threshold of P<0.05. These identified genes were integrated into a robust and accurate model using our machine learning-based integration algorithm. Within the TCGA dataset, we constructed 77 prediction models employing the leave-one-out cross-validation (LOOCV) technique. Subsequently, we evaluated the concordance index (C-index) of each model across all validation datasets. The model exhibiting the highest average C-index was selected as the optimal one. Then we calculated each patient a risk score based on the following formula: risk score = gene exp1 × β1 + gene exp2 × β2 + … + gene expression n × βn (gene expression refers to the value of gene expression, while β represents the corresponding coefficient in the multivariate Cox model). Survival curves and risk maps for patients were graphically represented using the R software, incorporating the “survival” and “survminer” (17). To evaluate the predictive performance of risk scores for overall survival (OS) at 1, 3, and 5 years in HCC patients, receiver operating characteristic (ROC) curves were generated employing the “timeROC” (18) package. Additionally, the prognostic model’s validity was confirmed through analysis of external ICGC datasets.
Gene set enrichment analysis (GSEA) enrichment analysis
Enrichment analysis via GSEA (19) was conducted utilizing the “clusterProfiler” package (20) to scrutinize functional disparities and associated pathways among high- and low-risk groups in TCGA, encompassing all genes. To delineate these distinctions, a compilation of 50 human cancer marker pathway genes was acquired from the Molecular Signature Database (MSigDB) (https://www.gsea-msigdb.org/gsea/index.jsp). Additionally, gene set variation analysis (GSVA) (21) enrichment analysis was executed on gene samples from high- and low-risk groups, and discrepancies in GSVA scores were assessed utilizing the “limma” package (22).
Independent prognostic analysis
Univariate analysis was utilized to assess the risk model and clinical parameters. Next, multivariate Cox analysis was employed to identify independent risk factors crucial for predicting the OS of HCC patients. After identifying independent prognostic factors through multivariate Cox analysis, we constructed the nomogram model using the “cph” function in R. This model was designed to visualize the prediction and estimate potential survival rates at 1, 3, and 5 years for patients. Calibration curves were subsequently utilized to assess the accuracy of the presented information.
Analysis of immune cell infiltration
For every HCC specimen, we computed the immunological score using the “ESTIMATE” package, and subsequently compared differences in immune scores among clusters. To evaluate immune-related pathway activity and the infiltration of immune cells, we employed single-sample gene set enrichment analysis (ssGSEA) algorithms. Moreover, the “CIBERSORT” tool was utilized to calculate the relative proportions of immune cells within heterogeneous cell clusters. Subsequently, samples were screened based on a significance threshold of P<0.05. Additionally, we explored the association between hub genes and immune cell infiltration, as well as their correlation with immune checkpoints. Finally, we retrieved the expression levels of four immune checkpoint markers in HCC. Mutational differences between high- and low-risk groups were investigated employing the “maftools” package (23).
Chemotherapy drug sensitivity analysis
To delve deeper into the potential utility of risk scores in guiding chemotherapy, this study utilized the “oncoPredict” package (24) to acquire the half maximal inhibitory concentration (IC50) values of drugs from the Genomics of Drug Sensitivity in Cancer (GDSC) database.
Statistical analysis
All data processing, statistical analysis and plots were performed by R (version 4.3.2). Pearson’s correlation coefficients were employed to evaluate the associations between two continuous variables. To assess the statistical significance of normally distributed variables in comparing two groups with continuous variables, the independent t-test was employed. For non-normally distributed variables, analyses between the two groups were conducted utilizing the Wilcoxon test. The threshold for significance was defined as P<0.05.
Results
Identification of HCC cell subtypes
Utilizing an scRNA-seq methodology, we aimed to delineate the transcriptional profile of a considerable cell population within primary tumors. Post meticulous filtration, a total of 73,159 cells met the criteria for subsequent examination. Subsequent to normalizing gene expression, we executed dimensionality reduction and clustering procedures employing principal component analysis and UMAP, correspondingly. The cellular classification revealed the presence of 7 discernible cell phenotypes (Figure 1A) using known marker genes: epithelial cells (marked with EPCAM, ALDH1A1 and ALB); B cells (marked with MS4A1 and CD79A); T cells (marked with CD3D and CD3E); natural killer (NK) cells (marked with FGFBP2 and FCG3RA); monocytes or macrophages (marked with CD68, CD163, and CD14); dendritic cells (marked with ITGAX); fibroblasts (marked with ACTA2 and COL1A2); and endothelial cells (marked with PECAM1 and vWF). Observing variations in the cellular composition across individual samples, we found disparities in the proportions of cells within each sample while examining the distributions of the seven distinct cell clusters (Figure 1B). Upon analyzing all seven cell distributions, we discovered that the fraction of cells varied across all samples. In two samples (p5 and p7), the largest proportion belonged to epithelial cells, whereas in three samples (p1, p2, and p4), the highest percentage belonged to T cells. The dot plots displaying differentially expressed genes (DEGs) and marker genes further validated the precision of cell characterization (Figure 1C,1D).
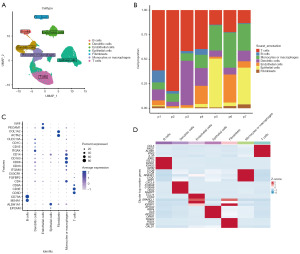
Identification of DE-ERGs
In order to elucidate the association between ERGs and various cell clusters, we conducted an intersection analysis between 5,144 differentially expressed cell markers and 1,024 MRGs (table available at https://cdn.amegroups.cn/static/public/TCR-24-521-1.xlsx). This analysis yielded 421 DE-ERGs, as illustrated in Figure 2A. Subsequent Gene Ontology (GO) and Kyoto Encyclopedia of Genes and Genomes (KEGG) analyses were carried out to further characterize the DE-ERGs. GO results suggested that DE-ERGs were mainly involved in epithelial to mesenchymal transition, Wnt signaling pathway, Ras protein signal transduction, SMAD protein signal transduction, ERBB signaling pathway, Toll-like receptor binding, epithelial cell migration, Notch signaling pathway (Figure S1). KEGG results suggested that DE-ERGs were mainly associated with AGE-RAGE signaling pathway in diabetic complications, PI3K-Akt signaling pathway, ECM-receptor interaction, HIF-1 signaling pathway, EGFR tyrosine kinase inhibitor resistance, MAPK signaling pathway, ERBB signaling pathway, TGF-β signaling pathway, NF-kappa B signaling pathway, TNF signaling pathway, Toll-like receptor signaling pathway, JAK-STAT signaling pathway, Ras signaling pathway, VEGF signaling pathway, Wnt signaling pathway, AMPK signaling pathway (Figure 2B).
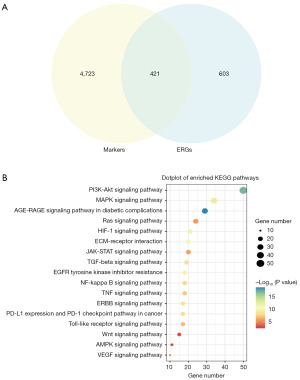
Cell-cell communication analysis
CellChat was employed to illustrate complex networks of cell-to-cell communication. Within HCC samples, there was an observed augmentation in the interaction between epithelial cells and fibroblasts, endothelial cells, as well as monocytes or macrophages (Figure 3A). Hierarchical plot of the inferred TGF-β, EGF, MIF, NOTCH, VEGF and VTN signaling network identified that possible ligands between several cells and the main source (Figure 3B). Analysis was conducted on the network of interactions between ligands and receptors involving epithelial cells and various other cell types. Results indicated a notable increase in MIF-(CD74 + CXCR4), MIF-(CD74 + CD44), and VTN-(ITGAV + ITGB1) within HCC (Figure 3C). Further analysis of cell-cell communication among subgroups also revealed that multiple pairs of ligand-receptor pairs were activated in HCC tissues, such as MIF-(CD74 + CXCR4) and MIF-(CD74 + CD44) (Figure 3D). In general, communication patterns among cells within tumor tissues deviate from those observed in normal cells, a phenomenon potentially linked to the onset and progression of HCC.
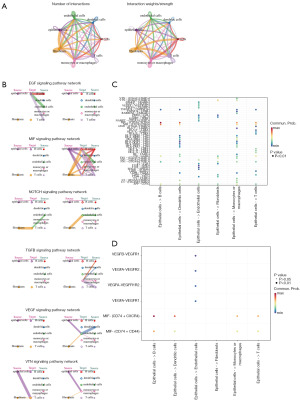
Analysis of epithelial subtypes
For a more comprehensive investigation of the involvement of epithelial cells in HCC, we employed the “Seurat” software to downscale the epithelial cells within the HCC single-cell transcriptional profile, resulting in the identification of 10 distinct subsets of epithelial cells (Epi1–Epi10, Figure 4A). Utilizing the “FindAllMarkers” function, we identified the cellular signature genes within the 10 epithelial cell subsets. Subsequently, we employed the “scRNAtoolVis” software to visualize the volcano plots depicting the signature genes, showcasing the top 5 upregulated and downregulated genes within each respective cell subset (Figure 4B). Furthermore, GSEA enrichment analysis of the marker genes within the Epi1–Epi10 subsets was conducted separately (Figure 4C). The upregulated genes in Epi5 are mainly enriched in the cytokine-cytokine receptor interaction and T cell receptor signaling pathway. The upregulated genes in Epi7 are mainly enriched in the PPAR signaling pathway and that in Epi8 are mainly enriched in the PI3K-Akt signaling pathway.
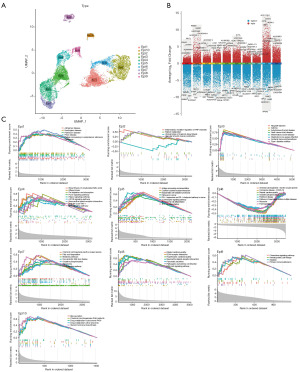
Construction and validation of model based on eight feature genes
Conducting univariate Cox regression analysis, we identified 112 ERGs whose expression significantly correlated with OS among the DE-ERGs. Following this, the ERGs were integrated into an ensemble framework to construct a prognostic model. Within the TCGA cohort, we developed uniform models utilizing 77 different algorithmic combinations. Subsequently, the average C-index was computed for every model across all cohorts to evaluate their predictive capacity (Figure 5A, Table S1). Analysis of these 77 models revealed that the combination of least absolute shrinkage and selection operator (LASSO) and random survival forest (RSF) algorithms consistently retained the highest mean C-index, thereby serving as the foundation for constructing the ultimate model. Moreover, the multivariate Cox algorithm facilitated the identification of the most valuable model, which was characterized by the incorporation of 8 pivotal hub genes (Figure 5B-5D). The coefficients associated with each gene in the multiple regression model were calculated to assess their contributions (Figure 5E). Then, we calculated the risk score per sample for all cohorts: risk score = expression of HSP90AA1 × 0.2885370 + expression of CIRBP × (−0.4929703) + expression of CCR7 × (−0.4914148) + expression of S100A9 × 0.1492653 + expression of ADAM17 × 0.7450708 + expression of ENG × (−0.2721958) + expression of PGF × 0.5476513 + expression of INPP4B × (−0.9619075). In the TCGA and ICGC cohorts, individuals with high-risk scores had lower survival time (Figure 5F). ROC curves were built with an area under the curve (AUC) of 0.785 at 1-year, 0.778 at 3-year, and 0.79 at 5-year, demonstrating the superior OS prediction ability of our prognostic model. Our prognostic model concludes with good prediction efficiency in HCC (Figure 5G).
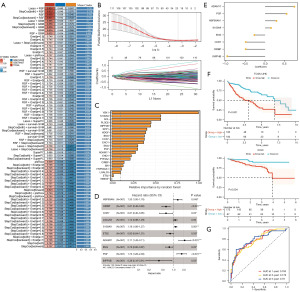
Screening for independent prognostic variables and nomogram creation
In order to identify independent prognostic determinants, both clinical attributes and risk scores underwent univariate and multivariate Cox analyses. Our findings highlighted the risk score as a standalone prognosticator among patients (Figure 6A,6B). This independent prognostic determinant was integrated into the nomogram model (Figure 6C). Additionally, the calibration plot illustrated the significant predictive capability of the model (Figure 6D). Hence, our outcomes imply that the risk score could serve as an independent prognostic factor, and the nomogram exhibits a certain predictive performance in estimating the OS of patients with HCC.
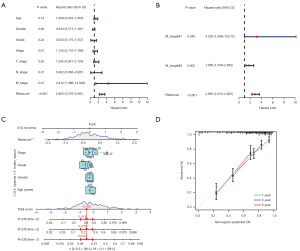
Analysis of risk scores and different clinical characteristics
It illustrates the heatmap depicting the risk model alongside clinical attributes (Figure 7A). In order to investigate the correlation between risk score expression and clinical attributes, we conducted separate assessments of patient risk score variations across different clinical characteristic groups. Our findings indicated significant disparities in risk scores across T-stage, grade, and OS status categories (Figure 7B-7I). The risk score rises in proportion to the tumor’s advancement. In general, our prognostic model, based on eight distinctive genes, exhibited remarkable prognostic efficacy.
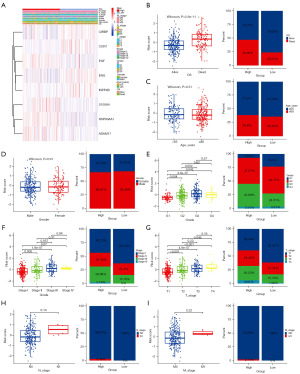
Analysis of GSEA between high and low risk groups
To evaluate the influence of high- and low-risk subcategories on cancer progression, we performed GSEA to identify the pathways showing substantial differences in enrichment between the high- and low-risk subgroups. Our analysis revealed a significant enrichment of epithelial mesenchymal transition, PI3K-Akt-mTOR signaling, and Wnt β-catenin signaling pathways within the high-risk subgroup (Figure 8A). HALLMARK showed that low-risk group was enriched in metabolic functions such as bile acid metabolism, and fatty acid metabolism (Figure 8B). Additionally, we conducted GSVA analysis to reveal PI3K-Akt-mTOR signaling, G2M checkpoint, and DNA repair was activated in the high-risk group. As the low-risk group displayed activation in marker entries related to myogenesis, peroxisome function, bile acid metabolism, KRAS signaling, and other associated marker entries (Figure S2A,S2B).
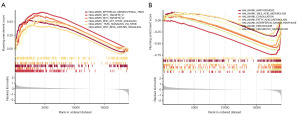
Evaluation of the possibility of HCC immunotherapy
Utilizing the CIBERSORT methodology, we generated 22 distinct immune cell profiles in patients with HCC. We assessed the proportions of immune subtypes infiltrating the tumor to establish the connection between the risk score and the immunological aspect (Figure 9A,9B). The low-risk group exhibited higher levels of immunological infiltration in terms of CD8 T cells, monocytes, and M1 macrophages. On the other hand, the high-risk group had greater levels of immune infiltration in terms of M0 macrophages and regulatory T cells. Utilizing ssGSEA, we evaluated the infiltration scores of 28 distinct immune cell types across different risk categories. Our examination uncovered notable differences in the levels of infiltration among activated CD4 T cells, activated CD8 T cells, and NK T cells (Figure 9C). The Pearson correlation assessment unveiled a significant correlation linking infiltrating immune cells with both prognostic genes and risk assessments (Figure 9D). Box plot analysis depicted discernible dissimilarities in the expression levels of PD-L1 between the high- and low-risk groups (Figure 9E). Our investigation disclosed a prevalence of missense mutations and single nucleotide polymorphisms (SNPs) among patients with HCC (Figure 10A). Comparing mutation patterns between high- and low-risk cohorts indicated a predominance of missense mutations in both groups. The TP53 mutation rate of 42% in the high-risk group and 15% in the low-risk group was displayed in the waterfall plot. Notably, the high-risk cohort exhibited a higher mutation frequency compared to the low-risk cohort, with an overall increase in the mutation load index TMB index observed in the high-risk group relative to the low-risk group (Figure 10B). In summary, our findings suggest promising prospects for the advancement of HCC treatment through immunotherapy.
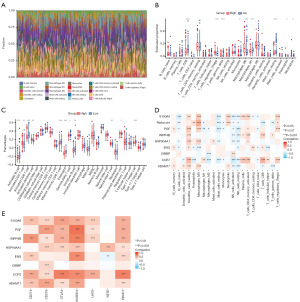
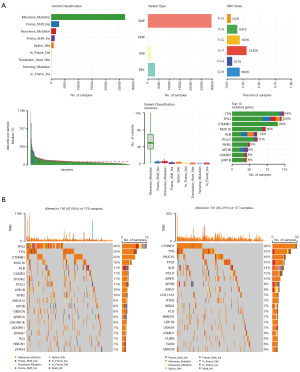
Prediction of chemotherapy effects by risk score
To explore the predictive capacity of the risk score in determining the response to chemotherapy, we initially obtained data from the GDSC database. Our findings revealed significant discrepancies in the efficacy of several common HCC chemotherapy drugs among high-risk groups (P<0.05). Notably, sorafenib, a prevalent chemotherapy option for HCC, exhibited substantially lower IC50 values in the low-risk group compared to the high-risk group (P<0.05), indicating a potentially higher efficacy of sorafenib in the low-risk population (Figure 11).
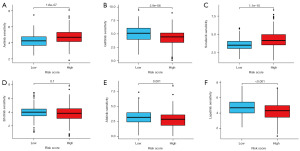
RNA and protein expression level of ERGs
The expression of S100A9 was found to be down-regulated in the eight ERGs utilized to construct the risk model from the TCGA cohort, but HSP90AA1, CIRBP, ADAM17, PGF, and INPP4B expression was up-regulated (Figure 12A). Subsequently, we delved deeper into the expressions of HSP90AA1, CCR7, S100A9, ADAM17, ENG, and INPP4B using immunohistochemistry methods from the HPA database. Protein levels of CCR7, ADAM17, ENG, and INPP4B were elevated in HCC tissues compared to paracarcinoma tissues, while no notable distinction was observed in the protein levels of HSP90AA1 and S100A9 between paracarcinoma and HCC tissues (Figure 12B).
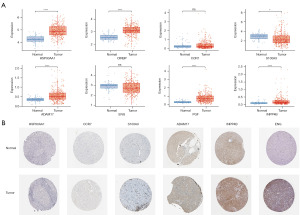
Discussion
HCC stands as a formidable global health challenge, characterized by pronounced recurrence rates and mortality. The selection of optimal therapeutic strategies constitutes a pivotal aspect of clinical decision-making for individuals afflicted with HCC. Consequently, there exists an urgent imperative to unearth novel prognostic biomarkers and therapeutic targets tailored to address this pressing clinical need. To date, EMT is recognized as a pre-metastatic cellular event that facilitates tumor cell invasion and metastasis. This process similarly plays a pivotal role in HCC metastasis (25). Consequently, genes associated with EMT hold significant promise as biomarkers for monitoring liver cancer progression and predicting patient prognosis. Within the purview of this investigation, predictive models were meticulously constructed based on the distinct expression profiles of eight ERGs, leveraging data gleaned from the TCGA cohort. Robust validation endeavors were undertaken utilizing the ICGC cohort, yielding congruent outcomes. Remarkably, patients stratified with elevated risk scores evinced diminished prognostic outlooks and heightened mortality rates relative to their counterparts with lower risk scores. Noteworthy associations were discerned between escalated risk scores and augmented Phase T, grade, and clinical stage parameters. These findings underscore the utility of risk scores derived from the aforementioned eight ERGs as formidable independent prognosticators for individuals afflicted with HCC.
Regarding methodology, our research employs a unique way. Machine learning performs better at handling complicated data structures and interactions than traditional statistical methods, which makes it easier to identify probable features and patterns from a variety of clinical and gene expression data. Furthermore, by using LASSO and RSF for gene characterization and optimization methods, we were able to determine the most pertinent markers for the prediction of HCC patients. By using this technique, the feature space’s dimensionality is successfully decreased, and the model’s predictive capabilities are enhanced. Crucially, our research takes advantage of machine learning’s generalizability to evaluate the accuracy and dependability of models using a variety of datasets from several sources, such as TCGA and GEO. Initially, scRNA-seq was conducted on seven HCC specimens, facilitating the delineation of distinct cell clusters comprising epithelial cells, B cells, T cells, NK cells, monocytes or macrophages, dendritic cells, and endothelial cells. Subsequent GO and KEGG analyses of DE-ERGs sourced from TCGA revealed predominant enrichment in critical signaling pathways such as the PI3K-Akt and MAPK pathways, implicated in the proliferation and progression of HCC. Further, an 8-gene prognostic model was devised through a rigorous analytical framework encompassing univariate Cox regression analysis, machine learning algorithms, and multivariate Cox regression analysis, incorporating the genes HSP90AA1, CIRBP, CCR7, S100A9, ADAM17, ENG, PGF, and INPP4B.
Indeed, the prognostic model includes eight ERGs that have demonstrated associations with EMT processes across various malignant tumors, each fulfilling distinct roles within the tumor microenvironment (TME). Specifically, the HSP90AA1 gene encodes heat shock protein α (HSP90α), which, upon activation, functions to stabilize downstream proteins like mutant P53, EGFR, BRAF, and hTERT, consequently fostering tumor progression indirectly. Investigations into colorectal, ovarian, and renal cancers have elucidated HSP90α’s capability to modulate the EMT pathway, leading to the downregulation of epithelial markers E-cadherin and Vimentin, thereby facilitating lymph node metastasis in tumors (25). CCR7 is a G-coupled cheekiness receptor that has been identified as a mediator of Epstein-Barr virus (EBV) effects on B lymphocytes. Thus, CCR7 is mainly involved in the migration/trafficking of immune cells. Regarding metastasis, recent studies have found that high expression of CCR7 correlates with lymph node metastasis and promotes cell invasion and migration processes through the Akt signaling pathway in breast cancer (26-28). S100A9 exhibits affinity for Ca2+, Zn2+, RAGE, TLR4, and MMPs in a highly selective manner. It assumes regulatory roles both intracellularly and extracellularly, participating in cellular differentiation, signal transduction, migration, and adhesion processes (27). Numerous prior investigations have demonstrated the potential of S100A9 as a biomarker for various tumors, including HCC (29,30), gastric cancer, and neuroblastoma (31). Moreover, S100A9 facilitates HCC growth and metastasis via RAGE-mediated activation of ERK1/2 and P58 MAPK pathways (30). Increased serum levels of S100A9 expression are indicative of an unfavorable prognosis for patients following radical resection for HCC (31,32). Belonging to the ADAMs family, ADAM17 facilitates the shedding and maturation of multiple membrane proteins thus playing a role in cancer progression. Specifically, ADAM17 is involved in the cleavage of E-cadherin, promoting EMT and cellular motility (33,34). Within our investigation, we observed elevated levels of both RNA and protein for CCR7, ADAM17, and INPP4B in HCC. However, the protein levels of CIRBP and PGF were not confirmed. Further exploration is warranted to elucidate the specific mechanisms and functions of these genes.
Moreover, the samples underwent categorization into low-risk and high-risk cohorts determined by computed risk scores. Through studying the relationship between riskscore and clinical pathological features, we found that the risks were higher in G2 than G1, higher in G3 than G1, higher in G4 than G1, T2 than T1, higher in T3 than T1, higher in T4 than T1, higher in clinical stage II than clinical stage I, and higher in clinical stage III to clinical stage I. In terms of clinical relevance, the risk score was correlated with TNM stage, grade, and clinical stage suggesting a potentially higher risk score in patients with advanced HCC. Utilizing GSEA, it emerged that the high-risk cohort exhibited pronounced enrichment in pathways closely associated with tumorigenesis, notably encompassing EMT, PI3K-Akt-mTOR signaling, TNFα signaling via NF-kappa B, and Wnt/β-catenin signaling. This delineation underscores the heightened aggressiveness inherent in tumors within this high-risk stratum. Intriguingly, these findings mirror the clinical profile typifying the high-risk cohort, characterized by advanced pathological and clinical staging, dismal prognostic outlooks, and elevated mortality rates. In stark contrast, pathways enriched in the low-risk subgroup predominantly center on the liver’s physiological metabolic processes, including bile acid, fatty acid, and xenobiotic metabolism. Given the liver’s pivotal role as a nexus for fatty acid metabolism, encompassing β-oxidation, ω-oxidation, and fatty acid synthesis, among others, a more favorable clinical trajectory is anticipated relative to their high-risk counterparts. Furthermore, the liver plays a pivotal role in primary bile acid biosynthesis and histidine metabolism, further underscoring its multifaceted metabolic significance. HCC demonstrates considerable resistance to multiple chemotherapeutic agents, either alone or when combined. Hence, the selection of suitable chemotherapeutic agents for patients with varying risk levels becomes crucial. Utilizing oncoPredict, we found individuals categorized as high-risk exhibited heightened responsiveness to sorafenib, while gefitinib might yield more favorable outcomes in low-risk patients.
The TME denotes the intricate cellular milieu enveloping a neoplasm, encompassing not only the tumor per se but also proximal vascular structures, the extracellular matrix, neighboring normal cells, and associated signaling molecules (35). Among the most prevalent tumor-infiltrating immune entities within the TME, tumor-associated macrophages (TAMs) occupy a central position (36). TAMs have been delineated as pivotal accelerators of HCC progression, owing to their secretion of an array of cytokines that incite EMT and potentiate tumor proliferation, invasion, and migration (37). Specifically, IL-6 secretion by TAMs has been documented to foster tumor dissemination via activation of the downstream JAK/STAT3 signaling axis, concurrent with the downregulation of E-cadherin and upregulation of EMT-associated transcription factors such as vimentin, snail, and twist (38). Moreover, TAMs are implicated in the release of exosomes and S100A9, which, in turn, modulate the stemness attributes of tumor cells. Our study’s findings delineate a discernible correlation between the 8-gene prognostic model and the infiltration of immune cells, alongside immune subtypes. We posit that the discernible prognostic disparity between high- and low-risk cohorts may, in part, be attributed to variances in the immune landscape of patients.
The dissemination of cancer cells through invasion constitutes a pivotal determinant of malignancy, exerting a profound impact on patient prognosis. Consequently, EMT has garnered considerable attention as a putative facilitator of tumor metastasis. Initially, our investigation focused on evaluating the expression levels of eight genes in HCC. Nonetheless, further refinements are requisite to enhance the robustness of our findings. The formulation and validation of our prognostic model, comprising eight genes, relied exclusively on data sourced from two databases, underscoring the necessity for additional experimental validation utilizing cellular or animal models to elucidate the precise regulatory mechanisms governing prognostic attributes. Furthermore, it is imperative to underscore that our conclusions predominantly emanate from transcriptomic profiling, and the prognostic model delineated for ERGs is yet to be translated into clinical practice or widely adopted.
Conclusions
Leveraging both scRNA-seq and RNA-seq datasets, we harnessed diverse machine learning methodologies to devise a novel prognostic framework aiming at predicting OS in HCC patients. This model represents an advancement, facilitating the estimation of survival probabilities among individuals grappling with HCC. Our discernments afford augmented insight into the pivotal prognostic determinants within the realm of HCC, thereby envisaging their prospective utility as diagnostic and therapeutic biomarkers.
Acknowledgments
Funding: This work was funded by
Footnote
Reporting Checklist: The authors have completed the TRIPOD reporting checklist. Available at https://tcr.amegroups.com/article/view/10.21037/tcr-24-521/rc
Peer Review File: Available at https://tcr.amegroups.com/article/view/10.21037/tcr-24-521/prf
Conflicts of Interest: All authors have completed the ICMJE uniform disclosure form (available at https://tcr.amegroups.com/article/view/10.21037/tcr-24-521/coif). The authors have no conflicts of interest to declare.
Ethical Statement: The authors are accountable for all aspects of the work in ensuring that questions related to the accuracy or integrity of any part of the work are appropriately investigated and resolved. The study was conducted in accordance with the Declaration of Helsinki (as revised in 2013).
Open Access Statement: This is an Open Access article distributed in accordance with the Creative Commons Attribution-NonCommercial-NoDerivs 4.0 International License (CC BY-NC-ND 4.0), which permits the non-commercial replication and distribution of the article with the strict proviso that no changes or edits are made and the original work is properly cited (including links to both the formal publication through the relevant DOI and the license). See: https://creativecommons.org/licenses/by-nc-nd/4.0/.
References
- Bray F, Ferlay J, Soerjomataram I, et al. Global cancer statistics 2018: GLOBOCAN estimates of incidence and mortality worldwide for 36 cancers in 185 countries. CA Cancer J Clin 2018;68:394-424. [Crossref] [PubMed]
- Wang G, Ding B, Sun L, et al. Construction and Validation of a Necroptosis-Related Signature Associated With the Immune Microenvironment in Liver Hepatocellular Carcinoma. Front Genet 2022;13:859544. [Crossref] [PubMed]
- Forner A, Reig M, Bruix J. Hepatocellular carcinoma. Lancet 2018;391:1301-14. [Crossref] [PubMed]
- Fang C, Liu S, Feng K, et al. Ferroptosis-related lncRNA signature predicts the prognosis and immune microenvironment of hepatocellular carcinoma. Sci Rep 2022;12:6642. [Crossref] [PubMed]
- Shi D, Wu F, Mu S, et al. Correction to: LncRNA AFAP1-AS1 promotes tumorigenesis and epithelial-mesenchymal transition of osteosarcoma through RhoC/ROCK1/p38MAPK/Twist1 signaling pathway. J Exp Clin Cancer Res 2020;39:72. [Crossref] [PubMed]
- Pastushenko I, Blanpain C. EMT Transition States during Tumor Progression and Metastasis. Trends Cell Biol 2019;29:212-26. [Crossref] [PubMed]
- Kong H, Yu W, Chen Z, et al. Correction to: CCR9 initiates epithelial-mesenchymal transition by activating Wnt/β-catenin pathways to promote osteosarcoma metastasis. Cancer Cell Int 2022;22:152. [Crossref] [PubMed]
- Yu X, Zheng H, Tse G, et al. Long non-coding RNAs in melanoma. Cell Prolif 2018;51:e12457. [Crossref] [PubMed]
- Qu X, Meng LC, Lu X, et al. Prognostic and metabolic characteristics of a novel cuproptosis-related signature in patients with hepatocellular carcinoma. Heliyon 2023;10:e23686. [Crossref] [PubMed]
- Chen D, Aierken A, Li H, et al. Identification of subclusters and prognostic genes based on glycolysis/gluconeogenesis in hepatocellular carcinoma. Front Immunol 2023;14:1232390. [Crossref] [PubMed]
- Gong H, Tao Y, Xiao S, et al. Identification of an EMT-related gene-based prognostic signature in osteosarcoma. Cancer Med 2023;12:12912-28. [Crossref] [PubMed]
- Tao Z, Huang J, Li J. Comprehensive intratumoral heterogeneity landscaping of liver hepatocellular carcinoma and discerning of APLP2 in cancer progression. Environ Toxicol 2024;39:612-25. [Crossref] [PubMed]
- Uhlen M, Zhang C, Lee S, et al. A pathology atlas of the human cancer transcriptome. Science 2017;357:eaan2507. [Crossref] [PubMed]
- Mu Y, Zheng D, Peng Q, et al. Integration of single-cell and bulk RNA-sequencing to analyze the heterogeneity of hepatocellular carcinoma and establish a prognostic model. Cancer Rep (Hoboken) 2024;7:e1935. [Crossref] [PubMed]
- Zhu GQ, Tang Z, Huang R, et al. CD36(+) cancer-associated fibroblasts provide immunosuppressive microenvironment for hepatocellular carcinoma via secretion of macrophage migration inhibitory factor. Cell Discov 2023;9:25. [Crossref] [PubMed]
- Jin S, Guerrero-Juarez CF, Zhang L, et al. Inference and analysis of cell-cell communication using CellChat. Nat Commun 2021;12:1088. [Crossref] [PubMed]
- Pravallika G, Rajasekaran R. Stage II oesophageal carcinoma: peril in disguise associated with cellular reprogramming and oncogenesis regulated by pseudogenes. BMC Genomics 2024;25:135. [Crossref] [PubMed]
- Ding M, Ran X, Qian S, et al. Clinical and therapeutical significances of the cluster and signature based on oxidative stress for osteosarcoma. Aging (Albany NY) 2023;15:15360-81. [Crossref] [PubMed]
- Zeng S, Xu Z, Liu Y, et al. CRABP2 reduces the sensitivity of Olaparib in ovarian cancer by downregulating Caspase-8 and decreasing the production of reactive oxygen species. Chem Biol Interact 2024;393:110958. [Crossref] [PubMed]
- Dai S, Gu Y, Zhan Y, et al. The potential mechanism of Aidi injection against neuroblastoma-an investigation based on network pharmacology analysis. Front Pharmacol 2024;15:1310009. [Crossref] [PubMed]
- Jiang B, Ye X, Wang W, et al. Comprehensive assessment of regulatory T-cells-related scoring system for predicting the prognosis, immune microenvironment and therapeutic response in hepatocellular carcinoma. Aging (Albany NY) 2024;16:5288-310. [Crossref] [PubMed]
- Wu J, Duan C, Han C, et al. Identification of CXC Chemokine Receptor 2 (CXCR2) as a Novel Eosinophils-Independent Diagnostic Biomarker of Pediatric Eosinophilic Esophagitis by Integrated Bioinformatic and Machine-Learning Analysis. Immunotargets Ther 2024;13:55-74. [Crossref] [PubMed]
- Liu Y, Zhao Y, Zhang S, et al. Developing a prognosis and chemotherapy evaluating model for colon adenocarcinoma based on mitotic catastrophe-related genes. Sci Rep 2024;14:1655. [Crossref] [PubMed]
- Yang X, Wei M, Huang Y, et al. ITGA11, a Prognostic Factor Associated with Immunity in Gastric Adenocarcinoma. Int J Gen Med 2024;17:471-83. [Crossref] [PubMed]
- Yuan K, Xie K, Lan T, et al. TXNDC12 promotes EMT and metastasis of hepatocellular carcinoma cells via activation of β-catenin. Cell Death Differ 2020;27:1355-68. [Crossref] [PubMed]
- Xu B, Zhou M, Qiu W, et al. CCR7 mediates human breast cancer cell invasion, migration by inducing epithelial-mesenchymal transition and suppressing apoptosis through AKT pathway. Cancer Med 2017;6:1062-71. [Crossref] [PubMed]
- Ma H, Gao L, Li S, et al. CCR7 enhances TGF-β1-induced epithelial-mesenchymal transition and is associated with lymph node metastasis and poor overall survival in gastric cancer. Oncotarget 2015;6:24348-60. [Crossref] [PubMed]
- Hayasaka H, Yoshida J, Kuroda Y, et al. CXCL12 promotes CCR7 ligand-mediated breast cancer cell invasion and migration toward lymphatic vessels. Cancer Sci 2022;113:1338-51. [Crossref] [PubMed]
- Shin JM, Chang IK, Lee YH, et al. Potential Role of S100A8 in Cutaneous Squamous Cell Carcinoma Differentiation. Ann Dermatol 2016;28:179-85. [Crossref] [PubMed]
- Huang CH, Kuo CJ, Liang SS, et al. Onco-proteogenomics identifies urinary S100A9 and GRN as potential combinatorial biomarkers for early diagnosis of hepatocellular carcinoma. BBA Clin 2015;3:205-13. [Crossref] [PubMed]
- Chen X, Xue Y, Feng J, et al. Identification S100A9 as a potential biomarker in neuroblastoma. Mol Biol Rep 2021;48:7743-53. [Crossref] [PubMed]
- Wu R, Duan L, Cui F, et al. S100A9 promotes human hepatocellular carcinoma cell growth and invasion through RAGE-mediated ERK1/2 and p38 MAPK pathways. Exp Cell Res 2015;334:228-38. [Crossref] [PubMed]
- Meng J, Gu F, Fang H, et al. Elevated Serum S100A9 Indicated Poor Prognosis in Hepatocellular Carcinoma after Curative Resection. J Cancer 2019;10:408-15. [Crossref] [PubMed]
- Ma Z, Gao Y, Liu W, et al. CD82 Suppresses ADAM17-Dependent E-Cadherin Cleavage and Cell Migration in Prostate Cancer. Dis Markers 2020;2020:8899924. [Crossref] [PubMed]
- Quail DF, Joyce JA. Microenvironmental regulation of tumor progression and metastasis. Nat Med 2013;19:1423-37. [Crossref] [PubMed]
- DeNardo DG, Ruffell B. Macrophages as regulators of tumour immunity and immunotherapy. Nat Rev Immunol 2019;19:369-82. [Crossref] [PubMed]
- Xiao P, Long X, Zhang L, et al. Neurotensin/IL-8 pathway orchestrates local inflammatory response and tumor invasion by inducing M2 polarization of Tumor-Associated macrophages and epithelial-mesenchymal transition of hepatocellular carcinoma cells. Oncoimmunology 2018;7:e1440166. [Crossref] [PubMed]
- Wu J, Zhang J, Shen B, et al. Long noncoding RNA lncTCF7, induced by IL-6/STAT3 transactivation, promotes hepatocellular carcinoma aggressiveness through epithelial-mesenchymal transition. J Exp Clin Cancer Res 2015;34:116. [Crossref] [PubMed]