Bioinformatics analysis of SH2D4A in glioblastoma multiforme to evaluate immune features and predict prognosis
Highlight box
Key findings
• The expression of SH2 domain containing 4A (SH2D4A) was upregulated in glioblastoma multiforme (GBM) patients, and the elevated expression of SH2D4A was also strongly associated with the grade of the tumor.
What is known, and what is new?
• SH2D4A plays different roles in different human cancers, and previous studies have validated the effect of SH2D4A as an oncogene.
• We systematically investigated the relationship between SH2D4A and GBM prognosis and validated it using different datasets.
What is the implication, and what should change now?
• We analyzed the prognosis of GBM patients based on public datasets and immune infiltration, and we found that SH2D4A could serve as a prognostic predictor and a potential immunotherapeutic target for GBM.
Introduction
Glioblastoma multiforme (GBM) is the most common and invasive primary brain tumor in adults, with limited choice of treatment, and a poor prognosis (1). Despite some progress in the standard treatment, which includes resection, radiation therapy, and chemotherapy, the median survival time of GBM patients is only about 15 months (2). Research has shown that the survival of GBM patients is affected by individual molecular biomarkers (3). Therefore, the identification of the key signaling molecules that contribute to GBM tumorigenesis is crucial to improve clinical treatment strategies and patient prognosis.
Tumor cell intrinsic genes, especially master transcription factors, dictate the initiation, progression, and evolution of GBM (4,5). The tumor microenvironment (TME) is made up of extracellular matrix (ECM) components, inflammatory mediators, mesenchymal cells, and endothelial cells (6,7). The two main subtypes of non-tumor components in the TME are stromal cells and immune cells, which have been shown to be useful for tumor prognostic and diagnostic evaluation (8). The TME also has a significant effect on gene expression in tumor tissue, and has been shown to influence patient prognosis overall, medication resistance, recurrence, and subtype classification (8). Immune-related risk factors can be used for treatment response monitoring and early diagnosis. Biomarkers and therapeutic targets linked to the advancement of GBM have been identified through extensive genomic and proteomic studies of the disease; however, these prospective targets need to be validated to generate novel clinical candidates and enhance the dismal prognosis of patients (9). Following developments in the fields of proteomics and genomics, there have been advances in precision medicine.
SH2 domain containing 4A (SH2D4A) (8p21.3 locus) encodes for SH(2)A (10). This protein has a SH2 structural domain that is very similar to that of the T cell-specific adapter protein and the adapter protein of unknown function, and has tyrosine residues with potential sites for the phosphorylation of lymphocyte-specific protein tyrosine kinase (LCK) (11). Research has shown that these proteins control the transduction of T cell receptor signaling (11). SH2D4A was shown to inhibit cell proliferation in a human embryonic kidney cell line (12). SH2D4A also plays different roles in different human cancers, and previous studies have shown the effect of SH2D4A as an oncogene. Hepatocellular carcinoma (HCC) usually results in the deletion and downregulation of six gene clusters on chromosome 8p, including SH2D4A, and the low expression of these genes is connected to a worse prognosis for HCC patients (10,13).
Molecular studies have shown that tumor-infiltrating immune cells (TIICs) can promote and regulate tumor progression and growth through interactions among different cell types (14). There has been some advancement in the understanding of immune cell infiltration in central nervous system malignancies. However, little is known about its role in the origin of tumors and patient prognosis (14). CD8+ T cell enrichment is related to glioma hypermutation at diagnosis or recurrence. Notably, following radiation therapy, glioma short-term recurrence has been linked to M2 macrophages (5). Research has shown that glioma-associated macrophages/monocytes promote glioma growth and invasion by acting as tumor-supporting cells that can infiltrate gliomas from the circulation (15).
Few studies have examined SH2D4A in GBM, and there are little data available. We systematically investigated the relationship between SH2D4A and GBM prognosis and validated it using different datasets. GBM patients with high SH2D4A expression have better clinical prognosis than those with low SH2D4A expression. Notably, the knockout of SH2D4A can significantly inhibit the proliferation and migration of U87 cells (16). The unique and complex immune microenvironment of gliomas is an important obstacle to immunotherapy (17). The infiltration of immune cells may be regulated by SH2D4A expression patterns. Therefore, we investigated the potential mechanisms and effects of SH2D4A and the glioma immune microenvironment. Our findings might help to improve the efficacy of immunotherapy. The Cancer Genome Atlas (TCGA) and the Chinese Glioma Genome Atlas (CGGA) were the data sources in this study. Cell Type Identification by Estimating Relative Subsets of RNA Transcripts (CIBERSORT), xCELL, and Tumor Immunity Estimation Resource (TIMER)/TIMER 2.0 were used to measure the density of large TIICs in various TMEs. We present this article in accordance with the TRIPOD reporting checklist (available at https://tcr.amegroups.com/article/view/10.21037/tcr-23-2000/rc).
Methods
Datasets and data collection
This study was conducted in accordance with the Declaration of Helsinki (as revised in 2013). Clinical data and TCGA RNA-sequencing transcriptome data were retrieved from TCGA dataset (18) (http://cancergemome.nih.gov/). The CGGA dataset (19) (http://www.CGGA.org.cn) was used to collect the messenger RNA (mRNA) expression data (mRNAseq 325) and associated clinicopathological characteristics. To formalize the pooled gene numbers into gene symbols for analysis, we used Perl (20) scripts to acquire the Genotype-Tissue Expression (GTEx) data from the University of California, Santa Cruz (UCSC) Xena (http://Xena.UCSC.edu/). R software was used to process the raw data, and a robust multi-array analysis was conducted to normalize and compensate for the backdrop.
Expression analysis by Gene Expression Profile Interaction Analysis (GEPIA)
GEPIA (21) (http://GEPIA.cancer-pku.cn/index.html) was used to confirm the relationship between the clinicopathological data and SH2D4A expression in GBM. To determine the differential expression of SH2D4A, we created box plots using the disease status (tumor or normal) as a variable.
Cell culture
The T98G, U87, and HA1800 cell lines were purchased from the Shanghai Cell Bank, Type Culture Collection Committee, Chinese Academy of Sciences. The cells were cultured using Dulbecco’s Modified Eagle Medium (Wisent, Canada) supplemented with 10% fetal bovine serum (Wisent). All the cell lines were stored in a humidified atmosphere containing 5% carbon dioxide at 37 ℃.
PPI network analysis
GeneMANIA (22) (http://genemania.org/) was used to predict the functionally comparable genes of the hub genes and build protein-protein interaction (PPI) networks once SH2D4A was added to its dataset. Additionally, GeneMANIA was used to make predictions about the relationships, pathways, physiological and biochemical responses, co-expressions, and co-localizations between the functionally related genes and the main gene. To examine the interaction network of the hub gene-encoded proteins, the resulting hub genes were added to the STRING dataset (version 11.5, https://string-db.org/). The necessary minimal interaction score was set to 0.4 (medium confidence level).
LinkedOmics analysis
We used LinkedOmics (http://www.linkedomics.org/) to analyze multivariate histological data for 32 different cancer types (23). The co-expression of the SH2D4A gene was examined using Pearson correlations, and the results were displayed as a volcano map and a heat map.
Independent prognostic role of the risk signature
Univariate and multivariate Cox regression analyses were conducted, the outcomes of the independent predictor analyses were presented as forest plots, and the patients were divided into high- and low-risk groups based on median risk scores to ascertain whether the risk profile associated with high SH2D4A expression was dependent on other clinicopathological factors (e.g., age, gender, and radiotherapy) that predicted patient overall survival (OS). Log-rank tests and a Kaplan-Meier OS analysis were then used. The aforementioned analyses were all completed using the R program. A P value <0.05 indicated a statistically significant difference.
Development and assessment of the nomogram
A nomogram generates custom predictive models that show the likelihood of clinical events using simple graphs of statistical predictive models. In this study, the nomogram was drawn using the R “survival” and “rms” packages, and the nomogram included scores for age, sex, radiation status, and the risk associated with SH2D4A. Calibration curves were used to assess the accuracy of the nomogram in predicting the one-, two-, and three-year survival of patients with glioma. The more closely the calibration curves’ projected and actual curves coincided, the greater their predictive value of the nomogram. An analysis of the receiver operating characteristic curves was conducted to evaluate the accuracy of the prediction model.
Functional enrichment analysis
A Gene Set Enrichment Analysis (GSEA) is a computational technique for evaluating whether there are consistently different biological states between two states, and the statistical significance of a collection of genes selected a priori (24). The Kyoto Encyclopedia of Genes and Genomes (KEGG) pathways and Gene Ontology (GO) biological processes (BPs) related to risk factors were evaluated using the gene set variation analysis (GSVA) package in R. We identified the various BPs that were enriched in the high- and low-risk groups by comparing the differences in the scores across the risk groups after the GSVA software assessed the GO BPs and KEGG pathways in each sample. To find the differentially expressed genes (DEGs) and gene sets in the various groups, the “limma” package in R was used. The “clusterProfiler” package in R was used to conduct the GO and KEGG analysis of the DEGs to further validate the feature-related KEGG pathways and GO processes.
Assessing the number of TIICs using TIMER/TIMER2.0
We used the TIMER/TIMER2.0 (25,26) as a complete resource to investigate the immune infiltration systems of various cancer types (https://cistrome.shinyapps.io/timer/). TIMER2.0 uses a previously described statistical technique known as deconvolution, which estimates the number of TIICs (27). The abundance of TIIC subtypes [B cells, CD4+ T cells, CD8+ T cells, macrophages, neutrophils, and dendritic cells (DCs)] and the expression of SH2D4A were analyzed and visualized.
xCELL analysis and CIBERSORT analysis
An R package called “xCell” was used to calculate the integrated levels of 64 different cell types, including 14 stromal cells. Using CIBERSORT, the abundance scores of each sample for 21 different immune cell types were precisely quantified (T cells gamma delta information was missing in 22 immune cell types in this study). We used xCELL and CIBERSORT to determine the ratio of stromal cell to immune cell abundance in the GBM samples separately.
Real-time quantitative reverse transcription polymerase chain reaction (qRT-PCR)
Using qRT-PCR, the relative mRNA expression of SH2D4A was evaluated. TRIzol (Invitrogen) was used to extract the total RNA from the GBM cell lines. RNA was reverse transcribed into complementary DNA using a reverse transcription kit (Vazyme, China). The target gene was amplified in a final volume of 20 µL with the SYBR qPCR Master Mix (Vazyme). The data were acquired and processed automatically by the ABI 7500 machine (Applied Biosystems, USA). The relative expression of the genes was calculated using the 2−ΔΔCt method. The following primers were used for the experiments: SH2D4A forward:5'-AGAAAGGAAGAGGCGGTGAGAGG-3' and reverse: 5'-ACTGAGGCTTGGGTGGAAGGG-3' with ACTIN as the internal reference; and forward: 5'-CCTGGCACCCAGCACAAT-3' and reverse: 5'GGGCCGGACTCGTCATAC-3'.
Statistical analysis by R-4.2.1
TCGA statistics were combined and run through R 4.2.1. The R package “ggplot2” was used to create a volcano plot of the DEGs. Cox regression was used to examine the correlations between the clinical data and SH2D4A expression. To determine the effects of SH2D4A expression and the other clinicopathological variables (e.g., age, sex, and radiation) on survival, multifactorial and univariate Cox regression analyses were performed. The cut-off value was set at P<0.05.
Results
Relationship between the prognosis of GBM and SH2D4A expression
Based on TCGA dataset, we first analyzed the DEGs of the mRNAs in GBM. The analysis showed that there were 6,765 DEGs, of which 3,413 were upregulated and 3,352 were downregulated, as shown in the generated volcano plot (Figure 1A). Using the GEPIA dataset, we examined the SH2D4A expression pattern in the GBM tissues. We found that the expression level of SH2D4A was significantly higher in the GBM tissues than the normal peritumor tissues (Figure 1B). Interestingly, compared with the normal brain tissues, SH2D4A was observed to be upregulated in highly aggressive GBM tissues but not in low-grade glioma (LGG) tissues (Figure 1B). Thus, the results showed that SH2D4A is significantly upregulated in GBM and has prognostic value for GBM. We verified the differential expression between the GBM cells (U87 and T98G) and normal human astrocyte HA1800 cells by qRT-PCR (Figure 1C). Compared to the HA1800 cells, SH2D4A was upregulated in the GBM cells, especially in the U87 cells. In conclusion, these findings suggest that SH2D4A promotes malignancy in GBM. We examined the connection between SH2D4A expression and pan-cancer in the TIMER2.0 dataset to verify the outcomes of the GEPIA dataset study and further investigate the link between SH2D4A expression and GBM. We confirmed that SH2D4A expression was upregulated in GBM, renal small-pigmented cell carcinoma, cervical adenocarcinoma, cervical squamous cell carcinoma, thyroid cancer, and gastric cancer. Conversely, the SH2D4A expression pattern was decreased in head and neck squamous cell carcinoma, renal clear cell carcinoma, and lung squamous carcinoma (Figure 1D).
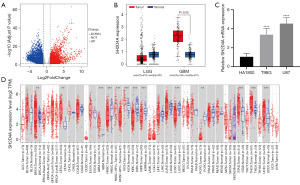
The clinical and prognostic significance of SH2D4A expression according to the CGGA dataset
We conducted an online study of the CGGA dataset comprising mRNA expression data (mRNAseq 325 array) and the accompanying clinicopathological characteristics to further evaluate their predictive value for GBM. We discovered that SH2D4A was considerably upregulated in GBM (Figure 2A-2E). Interestingly, consistent with the GEPIA and TCGA results, GBM patients with high SH2D4A expression had poorer survival outcomes (Figure 3A-3D). Shorter survival times were associated with upregulated SH2D4A expression in patients classified with grades III and IV GBM under the classification system of the World Health Organization (P<0.05). Additionally, there was a substantial correlation between SH2D4A differential expression and age, 1p19q deletion status, and the isocitrate dehydrogenase mutation (Figure 2A-2E). The above results suggest that SH2D4A is differentially expressed in gliomas and may be a potential biomarker for GBM progression.
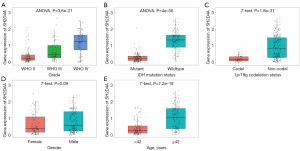
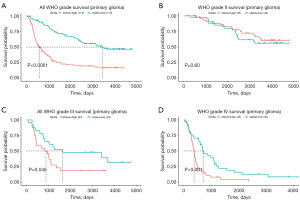
SH2D4A co-expression network
The GeneMANIA website was used to identify functionally similar genes and build a PPI network by entering the specific gene needed; that is, SH2D4A. In the PPI, 19 functionally similar genes were located in the outer loop, and the hub genes were located in the inner loop (Figure 4A). Based on the STRING dataset, the PPI network of these crossover genes was constructed, and the following 10 hub genes were identified: SH2D4A, SH3GL1, LYN, PPP1CB, PPP1R7, PPP1CA, DTD1, GRB2, DBNL, and HCLS1 (Figure 4B). The co-expression pattern of SH2D4A in TCGA cohort was studied using the functional module of LinkedOmics to examine the biological significance of SH2D4A in TCGA. Based on the RNA sequencing, we screened 19,660 genes associated with SH2D4A (and a false discovery rate <0.01) (Figure 4C). Two heatmaps were generated displaying the top 50 significant genes both negatively and positively correlated with SH2D4A (Figure 4D,4E).
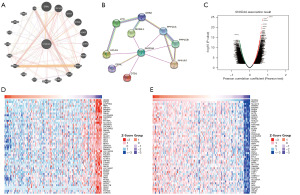
SH2D4A acted as an independent risk factor of a poor prognosis in GBM patients
Using least absolute shrinkage and selection operator (LASSO) regression and the “glmnet” R package, we screened the 107 samples of TCGA mRNA-sequencing data. The change in trajectory for each variable is plotted in Figure 5A, while Figure 5B displays the confidence range for each. The one-way Cox regression analysis identified SH2D4A expression as a risk factor affecting the prognosis of GBM patients (Table 1). Based on the median risk score for each cohort, the samples from TCGA cohort were split into low- and high-risk groups. According to the Kaplan-Meier analysis, patients in the low-risk group had better outcomes than those in the high-risk group (Figure 5C). In a multifactorial Cox analysis, SH2D4A expression and radiation status were identified as independent predictor variables of GBM (Figure 6A and Table 2).
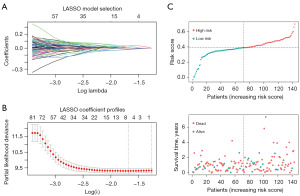
Table 1
Clinical characteristics | HR (95% CI) | P value |
---|---|---|
Risk | 1.59 (1.03, 2.47) | 0.04 |
Gender | 1.06 (0.67, 1.69) | 0.80 |
Radiation therapy | 0.21 (0.11, 0.42) | <0.001 |
Age | 1.02 (1, 1.04) | 0.02 |
SH2D4A | 1.36 (1.1, 1.67) | 0.004 |
TCGA, The Cancer Genome Atlas; HR, hazard ratio; CI, confidence interval.
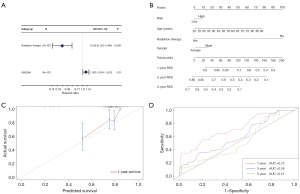
Table 2
Clinical characteristics | HR (95% CI) | P value |
---|---|---|
Radiation therapy | 0.249 (0.126, 0.494) | <0.001 |
SH2D4A | 1.303 (1.054, 1.612) | 0.01 |
HR, hazard ratio; CI, confidence interval.
In TCGA dataset, we developed a nomogram based on age, sex, radiation status, and risk score to predict one, two-, and three-year OS. The score of each factor in the nomogram indicated how much risk it posed in terms of OS (Figure 6B). For the one-year OS rate in TCGA cohort, the calibration curves revealed a significant agreement between the predicted survival time and actual survival time (Figure 6C). An area under the curve of 0.72 was found for the anticipated time-dependent one-year survival nomogram (Figure 6D). However, additional pertinent clinical investigations are required to confirm the validity of this nomogram.
SH2D4A-related signaling pathways in glioblastoma
To further investigate the biological role of SH2D4A in GBM, a GSEA was performed (Figure 7A). The results showed a number of functions were enriched in the highly expressed region of SH2D4A, including ECM organization and cytokine-mediated signaling pathways. The two KEGG items, neuroactive ligand-receptor interactions and protein digestion and uptake, showed significant enrichment differences in the SH2D4A high expression phenotype (Figure 7B). We performed a GO analysis of the BP, molecular function (MF), and cellular component (CC) (Figure 7C). The GO analysis showed that the significantly enriched BP included epidermal growth and ECM tissue, the significantly enriched CC included collagen containing the ECM, and the significantly enriched MF included DNA binding transcription repressor activity and the RNA polymerase II-specific and ECM structural constituent.
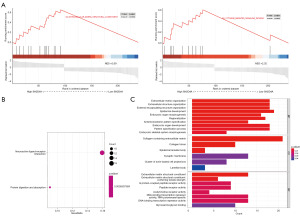
Relationship between immune cells that invade tumors and SH2D4A expression
We also sought to determine if immune infiltration in GBM was correlated with SH2D4A expression. Using the CIBERSORT method, we first examined the immune infiltration of 22 immune cell subpopulations in GBM tissue. The fraction of immune cells in each GBM sample is depicted using various colors in Figure 8A, while the immune cell population is represented by the length of the bars in the bar graph. According to the graphs, the GBM tissues had comparatively large concentrations of M0, M1, and M2 macrophages and monocytes. Next, using the R package, we generated a correlation heat map to identify the correlation between the above eight hub genes and 21 immune cells (Figure 8B). The results revealed a positive correlation between SH2D4A and the expression of T cells with activated CD4 memory cells, and a negative correlation between SH2D4A and activated natural killer cells. To determine the association between SH2D4A and other stromal cells, we calculated the levels of 64 immune cells using the xCELL algorithm (Figure 8C). The findings revealed that SH2D4A expression was correlated with type 1 T helper cells, neurons, plasma cells, megakaryocytes, and eosinophils.
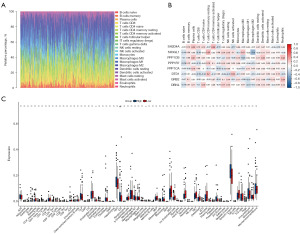
We also examined the relationship between SH2D4A expression and the degree of immunological infiltration using TIMER. Our findings demonstrated that higher levels of SH2D4A expression were linked to a worse prognosis and a compromised immune response in GBM. Additionally, there was a negative correlation between the levels of SH2D4A expression and B cell infiltration (r=−0.094, P=5.50e−02), CD4+ T cells (r=−0.116, P=1.79e−02), CD8+ T cells (r=−0.046, P=3.52e−01), macrophages (r=−0.081, P=1.00e−01), neutrophils (r=−0.192, P=7.87e−05) and DCs (r=0.314, P=5.01e−11) (Figure 9A). Next, a univariate Cox survival analysis was performed using the TIMER data. According to the findings of the univariate analysis, DCs and SH2D4A affected the survival prognosis of GBM patients (Figure 9B). However, the degree of immune infiltration of GBM did not appear to be correlated with variations in the SH2D4A copy number (Figure 9C). The significance of SH2D4A in the immunological infiltration of DCs is clearly supported by our findings.
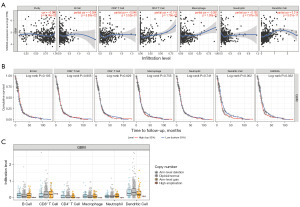
Discussion
Even with the use of the most recent treatments, such as immunotherapy and small molecule-targeted therapy, the median survival time for GBM patients remains about 12–15 months (28). Therefore, to increase the OS of GBM patients, it is crucial that critical chemicals for GBM growth and tumor resistance be identified.
Cell-to-cell interactions in the TME and changes in the expression of particular genes can influence how aggressively GBM grows. A number of ongoing clinical trials are seeking to find novel targets and medications for the treatment of GBM (29). Beta-1,4-galactosyltransferase (B4GALT3) expression is increased in GBM samples, and this high expression predicts poor survival for patients with glioma. B4GALT3 depletion reduces cell viability and the invasion of U251 cells (30). After temozolomide (TMZ) treatment, the small nucleolar RNA host gene 12 (SNHG12) is overexpressed in GBM samples that are resistant to TMZ. It may regulate the proliferation and mortality of TMZ induced cells (31). Researchers obtained the tumor infiltration data of immune cells by analyzing the gene methylation chip, and reported that cells with high infiltration rates in GBM tumor tissue may be potential targets for GBM treatments (32). Studies of SH2D4A in tumor progression are also ongoing. According to a study on the downregulation of SH2D4A in colon cancer, the downregulation of several genes on chromosome 8p, including SH2D4A, promotes cancer development and creates a cold TME with immune dysfunction and poor prognosis (33). Further, SH2D4A has been shown to promote the oncogenic progression of HCT15 and LoVo colorectal cancer cells (34). The role of SH2D4A in gliomas is not yet clear, but a recent study found that SH2D4A can stimulate the migration and proliferation of glioma cells (16).
TIMER was used to explore the association between SH2D4A and immune cell infiltration, and an inverse correlation was found between SH2D4A expression in tumor cells and the infiltration of CD8+ T cells in GBM. Tumor infiltration by CD8+ T cells is one of the key features of effective cancer immunotherapy (35). Therefore, the overexpression of SH2D4A in GBM may limit T cell infiltration, leading to unsatisfactory tumor killing.
According to multifactorial Cox analysis, the expression of SH2D4A in GBM patients can serve as a distinct prognostic factor. As a prospective cancer biomarker, SH2D4A expression patterns were observed to be linked with survival outcomes in the current investigation. We reaffirmed the prognostic significance of SH2D4A in GBM and the correlation between tumor grade and SH2D4A mRNA expression using data from the CGGA dataset. In summary, we analyzed the prognosis of GBM patients based on public datasets and immune infiltration, and found that SH2D4A may be an effective biomarker for predicting the prognosis of GBM patients. However, our analysis had a number of limitations. The bioinformatics analysis was only based on data from public datasets; thus, further in vivo or in vitro experiments need to be conducted to consolidate our research findings.
Conclusions
In addition to being a possible prognostic marker and therapeutic target for GBM, SH2D4A may also accelerate the progression of GBM.
Acknowledgments
Funding: This work was supported by
Footnote
Reporting Checklist: The authors have completed the TRIPOD reporting checklist. Available at https://tcr.amegroups.com/article/view/10.21037/tcr-23-2000/rc
Data Sharing Statement: Available at https://tcr.amegroups.com/article/view/10.21037/tcr-23-2000/dss
Peer Review File: Available at https://tcr.amegroups.com/article/view/10.21037/tcr-23-2000/prf
Conflicts of Interest: All authors have completed the ICMJE uniform disclosure form (available at https://tcr.amegroups.com/article/view/10.21037/tcr-23-2000/coif). The authors have no conflicts of interest to declare.
Ethical Statement: The authors are accountable for all aspects of the work in ensuring that questions related to the accuracy or integrity of any part of the work are appropriately investigated and resolved. The study was conducted in accordance with the Declaration of Helsinki (as revised in 2013).
Open Access Statement: This is an Open Access article distributed in accordance with the Creative Commons Attribution-NonCommercial-NoDerivs 4.0 International License (CC BY-NC-ND 4.0), which permits the non-commercial replication and distribution of the article with the strict proviso that no changes or edits are made and the original work is properly cited (including links to both the formal publication through the relevant DOI and the license). See: https://creativecommons.org/licenses/by-nc-nd/4.0/.
References
- Feng SW, Chang PC, Chen HY, et al. Exploring the Mechanism of Adjuvant Treatment of Glioblastoma Using Temozolomide and Metformin. Int J Mol Sci 2022;23:8171. [Crossref] [PubMed]
- von Rosenstiel C, Wiestler B, Haller B, et al. Correlation of the quantitative level of MGMT promoter methylation and overall survival in primary diagnosed glioblastomas using the quantitative MethyQESD method. J Clin Pathol 2020;73:112-5. [Crossref] [PubMed]
- Perrin SL, Samuel MS, Koszyca B, et al. Glioblastoma heterogeneity and the tumour microenvironment: implications for preclinical research and development of new treatments. Biochem Soc Trans 2019;47:625-38. [Crossref] [PubMed]
- Suvà ML, Rheinbay E, Gillespie SM, et al. Reconstructing and reprogramming the tumor-propagating potential of glioblastoma stem-like cells. Cell 2014;157:580-94. [Crossref] [PubMed]
- Wang Q, Hu B, Hu X, et al. Tumor Evolution of Glioma-Intrinsic Gene Expression Subtypes Associates with Immunological Changes in the Microenvironment. Cancer Cell 2017;32:42-56.e6. [Crossref] [PubMed]
- Hanahan D, Weinberg RA. The hallmarks of cancer. Cell 2000;100:57-70. [Crossref] [PubMed]
- Hanahan D, Coussens LM. Accessories to the crime: functions of cells recruited to the tumor microenvironment. Cancer Cell 2012;21:309-22. [Crossref] [PubMed]
- Jia D, Li S, Li D, et al. Mining TCGA database for genes of prognostic value in glioblastoma microenvironment. Aging (Albany NY) 2018;10:592-605. [Crossref] [PubMed]
- Shergalis A, Bankhead A 3rd, Luesakul U, et al. Current Challenges and Opportunities in Treating Glioblastoma. Pharmacol Rev 2018;70:412-45. [Crossref] [PubMed]
- Quagliata L, Andreozzi M, Kovac M, et al. SH2D4A is frequently downregulated in hepatocellular carcinoma and cirrhotic nodules. Eur J Cancer 2014;50:731-8. [Crossref] [PubMed]
- Lapinski PE, Oliver JA, Bodie JN, et al. The T-cell-specific adapter protein family: TSAd, ALX, and SH2D4A/SH2D4B. Immunol Rev 2009;232:240-54. [Crossref] [PubMed]
- Li T, Li W, Lu J, et al. SH2D4A regulates cell proliferation via the ERalpha/PLC-gamma/PKC pathway. BMB Rep 2009;42:516-22. [Crossref] [PubMed]
- Roessler S, Long EL, Budhu A, et al. Integrative genomic identification of genes on 8p associated with hepatocellular carcinoma progression and patient survival. Gastroenterology 2012;142:957-966.e12. [Crossref] [PubMed]
- Zhong QY, Fan EX, Feng GY, et al. A gene expression-based study on immune cell subtypes and glioma prognosis. BMC Cancer 2019;19:1116. [Crossref] [PubMed]
- Vinnakota K, Hu F, Ku MC, et al. Toll-like receptor 2 mediates microglia/brain macrophage MT1-MMP expression and glioma expansion. Neuro Oncol 2013;15:1457-68. [Crossref] [PubMed]
- Wu Y, Mao M, Wang LJ. Integrated clustering signature of genomic heterogeneity, stemness and tumor microenvironment predicts glioma prognosis and immunotherapy response. Aging (Albany NY) 2023;15:9086-104. [Crossref] [PubMed]
- Jackson CM, Choi J, Lim M. Mechanisms of immunotherapy resistance: lessons from glioblastoma. Nat Immunol 2019;20:1100-9. [Crossref] [PubMed]
- Blum A, Wang P, Zenklusen JC. SnapShot: TCGA-Analyzed Tumors. Cell 2018;173:530. [Crossref] [PubMed]
- Zhao Z, Zhang KN, Wang Q, et al. Chinese Glioma Genome Atlas (CGGA): A Comprehensive Resource with Functional Genomic Data from Chinese Glioma Patients. Genomics Proteomics Bioinformatics 2021;19:1-12. [Crossref] [PubMed]
- Stajich JE, Block D, Boulez K, et al. The Bioperl toolkit: Perl modules for the life sciences. Genome Res 2002;12:1611-8. [Crossref] [PubMed]
- Li C, Tang Z, Zhang W, et al. GEPIA2021: integrating multiple deconvolution-based analysis into GEPIA. Nucleic Acids Res 2021;49:W242-6. [Crossref] [PubMed]
- Franz M, Rodriguez H, Lopes C, et al. GeneMANIA update 2018. Nucleic Acids Res 2018;46:W60-4. [Crossref] [PubMed]
- Vasaikar SV, Straub P, Wang J, et al. LinkedOmics: analyzing multi-omics data within and across 32 cancer types. Nucleic Acids Res 2018;46:D956-63. [Crossref] [PubMed]
- Subramanian A, Kuehn H, Gould J, et al. GSEA-P: a desktop application for Gene Set Enrichment Analysis. Bioinformatics 2007;23:3251-3. [Crossref] [PubMed]
- Li T, Fan J, Wang B, et al. TIMER: A Web Server for Comprehensive Analysis of Tumor-Infiltrating Immune Cells. Cancer Res 2017;77:e108-10. [Crossref] [PubMed]
- Li T, Fu J, Zeng Z, et al. TIMER2.0 for analysis of tumor-infiltrating immune cells. Nucleic Acids Res 2020;48:W509-14. [Crossref] [PubMed]
- Li B, Severson E, Pignon JC, et al. Comprehensive analyses of tumor immunity: implications for cancer immunotherapy. Genome Biol 2016;17:174. [Crossref] [PubMed]
- Zhong J, Wu X, Gao Y, et al. Circular RNA encoded MET variant promotes glioblastoma tumorigenesis. Nat Commun 2023;14:4467. [Crossref] [PubMed]
- Verdugo E, Puerto I, Medina MÁ. An update on the molecular biology of glioblastoma, with clinical implications and progress in its treatment. Cancer Commun (Lond) 2022;42:1083-111. [Crossref] [PubMed]
- Wu T, Li Y, Chen B. B4GALT3 promotes cell proliferation and invasion in glioblastoma. Neurol Res 2020;42:463-70. [Crossref] [PubMed]
- Lu C, Wei Y, Wang X, et al. DNA-methylation-mediated activating of lncRNA SNHG12 promotes temozolomide resistance in glioblastoma. Mol Cancer 2020;19:28. [Crossref] [PubMed]
- Du SZ, Chen C, Qin L, et al. Bioinformatics analysis of immune infiltration in glioblastoma multiforme based on data using a methylation chip in the GEO database. Transl Cancer Res 2021;10:1484-91. [Crossref] [PubMed]
- Matsumoto T, Okayama H, Nakajima S, et al. SH2D4A downregulation due to loss of chromosome 8p is associated with poor prognosis and low T cell infiltration in colorectal cancer. Br J Cancer 2022;126:917-26. [Crossref] [PubMed]
- Xu S, Tang L, Li X, et al. Immunotherapy for glioma: Current management and future application. Cancer Lett 2020;476:1-12. [Crossref] [PubMed]
- Wang N, Zhu L, Wang L, et al. Identification of SHCBP1 as a potential biomarker involving diagnosis, prognosis, and tumor immune microenvironment across multiple cancers. Comput Struct Biotechnol J 2022;20:3106-19. [Crossref] [PubMed]