A novel tumor-derived exosomal gene signature predicts prognosis in patients with pancreatic cancer
Introduction
Pancreatic cancer is a highly lethal gastrointestinal cancer and is an increasingly common cause of cancer-related deaths. Global Cancer Statistics 2020 revealed that there were 495,773 new cases of pancreatic cancer and 466,003 related deaths worldwide in 2020 (1). It is characterized by an insidious onset and development of early metastasis (2). Owing to difficulties in early detection, patients with pancreatic cancer are often diagnosed at an advanced stage or with distant metastases, and the 5-year survival rate is less than 9% (3). In addition, routine tumor biomarkers, such as carcinoembryonic antigen and carbohydrate antigen 19-9 do not seem to be reliable for the early detection and outcome prediction of pancreatic cancer (4). Therefore, new biomarkers of pancreatic cancer are urgently needed.
Exosomes, a subset of extracellular vehicles with an average diameter of approximately 100 nm (5), exist in various biological fluids, including blood (6). They carry multiple cargo pertaining to the cells of origin, such as mRNAs, nucleic acids, microRNAs, and proteins, and play a pivotal role in intercellular communication, affecting various cellular processes in human cancers, including pancreatic cancer (7-10). The components of exosomes have been recognized as diagnostic or prognostic biomarkers, as well as anti-cancer drug delivery carriers (11). Additionally, the contents of exosomes are related to cell type, and tumor-derived exosomes may provide a unique signature of tumor progression, which is invaluable for the early detection and prognosis of pancreatic cancer (12). However, the potential mechanisms by which tumor-derived exosomes promote pancreatic cancer progression have not yet been fully explored.
In this study, we downloaded the gene expression data of pancreatic cancer solid tumor and blood-derived exosome tissue samples from The Cancer Genome Atlas (TCGA) and exoRBase 2.0 databases, respectively. The patients in TCGA database belonged to Asia, North America, and South America. We analyzed the overlapping differentially expressed genes (DEGs) in the two datasets, which were considered pancreatic cancer-related exosomal DEGs. Functional enrichment, protein-protein interaction (PPI) network, and weighted gene co-expression network analyses (WGCNA) were performed to screen key exosomal DEGs. Moreover, we constructed a tumor-derived exosomal gene signature based on the TCGA dataset, which was validated using the external validation dataset GSE62452. The prognostic value of this gene signature and its relationship with various pathways and immune cell infiltration were analyzed. Our findings provide a novel perspective for the development of promising biomarkers for pancreatic cancer. We present this article in accordance with the TRIPOD reporting checklist (available at https://tcr.amegroups.com/article/view/10.21037/tcr-23-2354/rc).
Methods
Data source and data preprocessing
The study was conducted in accordance with the Declaration of Helsinki (as revised in 2013). The pancreatic cancer transcriptome data in TCGA, normalized with log2 (fpkm+1) transformation, were downloaded from the UCSC Xena database on October 20, 2023. This dataset contained 182 samples, and the detection platform used was the Illumina HiSeq 2000 RNA Sequencing. After corresponding to the clinical information, 181 samples were used for analysis, including 177 solid tumor samples with clinical prognostic information (Table S1) and four normal control samples.
The expression profiling data of blood-derived exosome tissue samples from patients with pancreatic cancer and healthy controls were downloaded from the exoRBase 2.0 database (13). This dataset included 164 tumor-derived exosome tissue samples and 118 normal control samples, and the detection platform was GPL20795HiSeq × Ten. For data preprocessing, expression profiling data were subjected to quantile standardization using the R3.6.1 preprocess Core package (version 1.40.0) (14).
Pancreatic cancer-related gene expression profiling data [accession number: GSE62452 (15)] were downloaded from the Gene Expression Omnibus (GEO) (16) repository, and the platform used was the GPL6244 [HuGene-1_0-st] Affymetrix Human Gene 1.0 ST Array [transcript (gene) version]. There were 130 pancreatic cancer samples in this dataset, of which 65 with clinical prognostic information (Table S2) were retained and included in the analysis.
DEGs screening
The R3.6.1 limma package (version 3.34.7) (17) was used to screen the DEGs between pancreatic cancer and normal control samples in the TCGA and exoRBase 2.0 datasets, respectively. The threshold values for DEG screening were |log2 fold change (FC)| >0.5 and false discovery rate (FDR) <0.05. The overlapping DEGs identified from the two datasets were retained, which were considered pancreatic cancer-related exosomal DEGs and used for subsequent analysis.
Functional enrichment analysis
To elucidate the function of pancreatic cancer-related exosomal DEGs, Gene Ontology (GO)-biological process (BP) terms, and Kyoto Encyclopedia of Genes and Genomes (KEGG) pathway enrichment analyses were performed using DAVID (version 6.8) (18,19). The cutoff value was set at P<0.05.
PPI network
Potential interactions between the proteins coding for pancreatic cancer-related exosomal DEGs were obtained using the STRING (version 11.0) (20) database with a confidence interaction score of 0.4. A PPI network was established using Cytoscape (version 3.9.0) (21). The CentiScaPe (version 2.2) (22) plug-in in Cytoscape was used to analyze the topological properties of the PPI network. The modules of the PPI network were obtained using the Mcode (version 1.4.2) (23) plug-in in Cytoscape, with the following parameters: degree cutoff =2, node score cutoff =0.2, and k-core =2. Functional enrichment analysis of the DEGs in each module was performed using DAVID.
Construction of gene co-expression networks
WGCNA can be used to identify gene modules associated with diseases by constructing gene co-expression networks (24). In this study, based on all the genes that were expressed in the TCGA and exoRBase 2.0, the gene modules that were associated with disease and stable across datasets were identified using R3.6.1 WGCNA (version 1.61) (25). The TCGA dataset was used as the main analysis dataset and the exoRBase 2.0 dataset served as the validation dataset. For the WGCNA, we first calculated the pairwise expression correlation between the two datasets to ensure comparability. We then defined the adjacency function, gene module division, module stability evaluation, and correlation analysis between the stable modules and pancreatic tumors. The co-expression modules were identified using the following parameters: number of genes in each module >200 and cut height =0.995. Module stability across different datasets was evaluated using the preservation parameter Z-score, which indicates the stability of the module. In general, 5< Z <10 indicates that the module is stable, whereas Z >10 indicates that the module is highly stable. The identified pancreatic cancer-related exosomal DEGs were mapped to each WGCNA module, and the significant enrichment parameters, including fold enrichment and enrichment significance of DEGs in the module, were calculated using a hypergeometric algorithm (26). The threshold value was set at P<0.05 and fold enrichment >1.
Construction of the prognostic signature
Based on the clinical survival information of pancreatic cancer samples in TCGA dataset, univariate Cox regression analysis for DEGs in key disease-related modules was conducted using the survival package (version 2.41-1) (27) to initially investigate overall survival-related genes in patients with pancreatic cancer. DEGs with P<0.05 were screened and then used for multivariate Cox regression analysis to identify DEGs with independent prognostic value. The threshold value was a log-rank P value less than 0.05. By further screening with the least absolute shrinkage and selection operator (LASSO) Cox regression analysis using R3.6.1 lars package (version 1.2) (28), the prognostic signature was built by DEGs screened by LASSO regression analysis and its risk score was calculated as follows: risk score = ∑ Coef DEGs × Exp DEGs, where Coef DEGs represents the regression coefficient of DEGs and the Exp DEGs indicates the expression level of DEGs.
Evaluation of the prognostic signature
Using the median risk score as the cutoff value, pancreatic cancer samples in the TCGA dataset were classified into high-risk or low-risk groups. The Kaplan-Meier survival curve was plotted using the R3.6.1 survival package (version 2.41-1) to analyze the difference in OS between the two risk groups. Based on the expression level of target DEGs in the validation dataset GSE62452datase, the risk score of each pancreatic cancer sample was calculated. These samples were also assigned to high- and low-risk groups according to the median score, followed by Kaplan-Meier survival curve analysis to compare differences in prognostic time. The predictive power of this prognostic model for the two datasets was analyzed using the TimeROC package in R.
Gene set enrichment analysis (GSEA)
To explore the potential mechanism associated with the prognostic signature, differentially activated KEGG pathways in the two risk groups of the TCGA dataset were identified using GSEA (29). The enrichment score (ES), normalized enrichment score (NES), and nominal P value of the pathways were calculated. The cutoff value was adjusted to P<0.05.
Evaluation of immune cell infiltration of two risk groups
To explore the potential relationship between the prognostic signature and immune cell infiltration, the proportion of 22 types of immune cells in pancreatic cancer samples in the TCGA and exoRBase 2.0 datasets was evaluated using the CIBERSORT algorithm (30), and the differences between the two risk groups were compared using t-test in R3.6.1. Moreover, the correlation between the expression of DEGs in the prognostic signature and the proportion of differentially expressed immune cells was analyzed in pancreatic cancer tumor samples from TCGA and exoRBase 2.0 datasets, respectively.
Statistical analysis
The data from the public databases were merged and analyzed using the different packages in R3.6.1. Univariate and multivariate Cox regression analyses and LASSO regression analysis were used to screen the optimal prognostic signature DEGs associated with pancreatic cancer. DEGs with P<0.05 in the univariate analysis were included for multivariate analysis, and the DEGs with a log-rank P value <0.05 were used for LASSO regression analysis. The overall survival time of the established prognostic signature was predicted by KM curves. The hazard ratio (HR) of individual factors was estimated along with a 95% confidence interval. Patients were divided into high- and low-risk groups according to the median risk score, and then the predictive power of this prognostic model was assessed through ROC analysis and the AUC values. Welch one-way ANOVA was used to analyze the correlation between the optimal prognostic signature DEGs and neoplasm histologic grade, followed by the Bonferroni correction or t-test. The statistical differences of the immune cells between the high- and low-risk groups were compared using t-test in R 3.6.1.
Results
Identification of DEGs
A flowchart of the bioinformatics analysis used in this study is shown in Figure 1. Based on FDR <0.05, and |log2 FC| >0.5, 538 (Table S3) and 575 DEGs (Table S4) were identified in pancreatic cancer solid tissue samples from the TCGA dataset and pancreatic cancer-related blood-derived exosome tissue samples from the exoRBase 2.0, respectively (Figure 2A). A total of 166 overlapping DEGs (48 downregulated and 118 upregulated) were identified from the two datasets and considered to be pancreatic cancer-related exosomal DEGs (Figure 2B, Table S5).

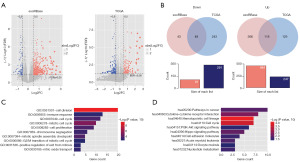
Functional enrichment analysis for pancreatic cancer-related exosomal DEGs
We performed a functional enrichment analysis of pancreatic cancer-related exosomal DEGs to explore their functions. These DEGs were significantly enriched in 52 GO-BP terms and 10 KEGG pathways with P<0.05, and the top ten according to the P value of significance was respectively listed in Figure 2C,2D. Notably, several GO functions and pathways were associated with the cell cycle.
PPI network analysis and hub gene identification
To screen for key genes in pancreatic cancer, a PPI network was built using the proteins encoding pancreatic cancer-related exosomal DEGs, including 121 genes and 626 interactions (Figure 3A). The top 20 nodes are listed in Table 1 according to node degree, from high to low. Cyclin dependent kinase 1 (CDK1) had the highest node degree, followed by cell division cycle 6 (CDC6) and TPX2 microtubule nucleation factor (TPX2). Using the Mcode plug-in in Cytoscape, four PPI network modules were identified (Figure 3B). Moreover, the DEGs in the four modules were remarkably implicated in 14, 8, 6, and 17 GO-BP terms (Figure 3C), as well as 7, 5, 1, and 4 KEGG pathways (Figure 3D).
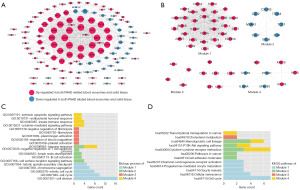
Table 1
Symbol | Average shortest path length | Betweenness centrality | Closeness centrality | Edge count |
---|---|---|---|---|
CDK1 | 1.36111111 | 0.13561851 | 0.73469388 | 34 |
CDC6 | 1.3902439 | 0.04044994 | 0.71929825 | 34 |
TPX2 | 1 | 0.27555277 | 1 | 33 |
BUB1B | 1.40909091 | 0.00504749 | 0.70967742 | 33 |
KIF11 | 1.25 | 0.16099508 | 0.8 | 33 |
UBE2C | 1 | 0.06926346 | 1 | 32 |
CDT1 | 1.38709677 | 0.12683345 | 0.72093023 | 32 |
BIRC5 | 1.41304348 | 0 | 0.70769231 | 32 |
BUB1 | 1.44444444 | 0 | 0.69230769 | 31 |
KIF2C | 1.30769231 | 0.04019763 | 0.76470588 | 31 |
TOP2A | 1.33333333 | 0.00463376 | 0.75 | 31 |
CDKN3 | 1.54285714 | 0.11293346 | 0.64814815 | 31 |
CDCA5 | 1.47368421 | 0.00537849 | 0.67857143 | 31 |
TK1 | 1.25 | 0.28135209 | 0.8 | 30 |
CEP55 | 1.63333333 | 0.02558414 | 0.6122449 | 30 |
ZWINT | 0 | 0 | 0 | 30 |
MCM10 | 1.3 | 0.02721109 | 0.76923077 | 30 |
CDCA2 | 1.48717949 | 0.00422545 | 0.67241379 | 30 |
FOXM1 | 1.58333333 | 0.50591839 | 0.63157895 | 29 |
HJURP | 1.29411765 | 0.02653192 | 0.77272727 | 29 |
The top 20 nodes were ranked based on edge count (node degree). PPI, protein-protein interaction.
Construction of co-expression modules associated with pancreatic cancer by WGCNA
To confirm that the gene expression levels in different datasets were comparable, pairwise expression correlation analyses were conducted for all detected genes between TCGA and exoRBase 2.0. Gene expression of the two datasets was positively correlated (cor =0.54, P<1e−200), as was connectivity (cor =0.16, P=1.7e−11) (Figure 4A), indicating that the data in the two datasets were comparable. Based on the TCGA dataset, the scale-free fit index and mean connectivity of network were calculated and the power of β=9 (scale free R2=0.9) was selected (Figure 4B). Nine distinct modules were identified in the hierarchical cluster tree (Figure 4C). The same module partitioning pattern was performed using another dataset exoRBase 2.0 dataset (Figure 4D). The correlation between the modules and clinical traits (pancreatic cancer patients and normal controls) is shown in Figure 4E. The stability of the nine modules in the three datasets was evaluated using the Z-score. The Z-scores of the two modules (black and green) were higher than five (Table 2), indicating that the two modules were significantly stable across datasets and might be implicated in pancreatic cancer.
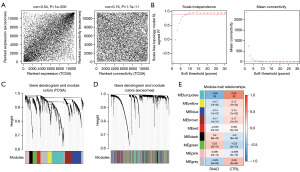
Table 2
ID | Color | Module size | Preservation Z-score | DEGs count | Enrichment information | |
---|---|---|---|---|---|---|
Enrichment fold (95% CI) | Phyper | |||||
Module 1 | Black | 289 | 11.7630993 | 40 | 4.557 (3.069–6.644) | 1.23E−12 |
Module 2 | Blue | 772 | 0.6696825 | 10 | 0.467 (0.199–0.811) | 6.23E−03 |
Module 3 | Brown | 617 | 2.7583373 | 1 | 0.0534 (0.00134–0.303) | 8.33E−07 |
Module 4 | Green | 339 | 5.5771807 | 30 | 2.914 (1.871–4.412) | 2.74E−06 |
Module 5 | Grey | 1,050 | 0.6472349 | 20 | 0.627 (0.371–1.009) | 5.10E−02 |
Module 6 | Pink | 203 | 1.9469039 | 7 | 1.136 (0.443–2.442) | 6.77E−01 |
Module 7 | Red | 309 | 3.1597558 | 5 | 0.533 (0.169–1.285) | 2.20E−01 |
Module 8 | Turquoise | 1,019 | 2.3888051 | 34 | 1.099 (0.729–1.614) | 6.20E−01 |
Module 9 | Yellow | 343 | 4.6472171 | 3 | 0.288 (0.0585–0.865) | 1.78E−02 |
DEGs, differentially expressed genes; CI, confidence interval.
Moreover, the identified pancreatic cancer-related exosomal DEGs were mapped to each WGCNA module, and 150 DEGs overlapped with the module genes. Among the nine modules, the black and green modules were significantly enriched, with 40 and 30 DEGs, respectively. Notably, they were significantly stable between the two datasets. The fold enrichment of the black and green modules was higher than 1, and their P values of enrichment significance were less than 0.05 (Table 2). Therefore, 70 DEGs from the two modules were selected for subsequent analyses.
Construction of the prognostic signature for pancreatic cancer
Based on the clinical survival information of pancreatic cancer samples in the TCGA dataset, univariate Cox regression analysis of the 70 pancreatic cancer-related exosomal DEGs in the black and green modules indicated that the expression levels of 58 DEGs were related to overall survival. Multivariate Cox regression analysis revealed that 11 of the 58 DEGs were independent prognostic factors. Furthermore, LASSO regression analysis demonstrated that six of the 11 pancreatic cancer-related exosomal DEGs were good candidates for establishing the prognostic signature, including Basic Helix-Loop-Helix ARNT like 2 (ARNTL2, also known as BMAL2), Four and A half LIM domains 2 (FHL2), keratin 19 (KRT19), matrix metallopeptidase 1 (MMP1), cell division cycle-associated 5 (CDCA5), and kinesin family member 11 (KIF11) (Figure 5A,5B). The risk score was then calculated as follows: risk score = (0.16730921) × Exp ARNTL2 + (−0.02127664) × Exp FHL2 + (0.01673997) × Exp KRT19 + (0.02643164) × Exp MMP1 + (0.0298823) × Exp CDCA5 + (0.03783781) × Exp KIF11. Moreover, survival analysis confirmed that only high expression of FHL2 was associated with favorable overall survival in patients with pancreatic cancer, whereas high expression of the other five genes was related to poor overall survival (Figure 5C).
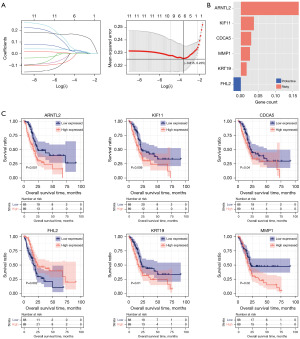
Evaluation of the prognostic signature
After calculating the risk score for each sample, the pancreatic cancer samples in TCGA and GSE62452 datasets were classified into high-risk or low-risk groups. The overall survival of patients with pancreatic cancer with a high-risk score in the two datasets was shorter than that of patients with a low-risk score (Figure 6A). Moreover, patients with pancreatic cancer in the two datasets were ranked according to their risk scores, and patients with a higher risk score tended to have a shorter survival time (Figure 6B). Furthermore, receiver operating characteristic (ROC) analysis demonstrated that the area under the ROC curve (AUC) of the prognostic signature for predicting the overall survival of patients with pancreatic cancer in TCGA and GSE62452 datasets was 0.810 (0.794, 0.787) and 0.754 (0.688, 0.816) (Figure 6C), respectively (Figure 6C), indicating the high predictive power of the prognostic signature. The relationship between each DEG and the histological grade of pancreatic cancer is shown in Figure 7A. The expression levels of ARNTL2, KRT19, MMP1, CDCA5 and KIF11 significantly increased with the increasing grade of pancreatic cancer (P<0.05), which is considered a disease risk factor. However, FHL2 levels decreased with an increase in disease grade, indicating that FHL2 may be a protective factor. These results are consistent with the prognostic correlations of each DEG.

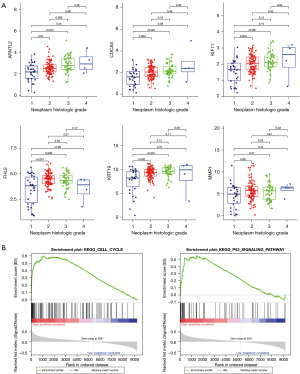
Analysis of key KEGG pathways and immune cell infiltration associated with the prognostic signature
To explore the key mechanisms associated with the prognostic signature, GSEA was performed to explore the differentially activated KEGG pathways between different risk groups based on TCGA dataset. We found that 15 KEGG pathways, such as the cell cycle and P53 signaling pathway, were differentially activated between the high-risk and low-risk groups (Figure 7B).
The prognostic signature was linked with immune cell infiltration
To explore the potential correlation of the prognostic signature with immune cell infiltration, we calculated the proportion of 22 types of immune cells in pancreatic cancer samples in TCGA dataset and compared their differences between different risk groups. We found that regulatory T cells (Tregs), resting myeloid dendritic cells, M0 macrophages, and activated myeloid dendritic cells were positively associated with the risk score, while naïve B cells and CD8+ T cells were negatively associated with the risk score (Figure 8A). Subsequently, the proportion of these six types of immune cells in pancreatic cancer samples in the TCGA and exoRBase 2.0 datasets were extracted, followed by analysis of their correlation with six pancreatic cancer-related exosomal DEGs in the prognostic signature. A significant correlation was observed between the six DEGs and immune cell infiltration in pancreatic cancer samples in TCGA dataset (Figure 8B). For instance, ARNTL2 was negatively correlated with the infiltration level of CD8+ T cells, and KRT19 was positively correlated with the infiltration level of Tregs. In pancreatic cancer samples in the exoRBase 2.0 dataset, CDCA5 was negatively correlated with CD8+ T cell infiltration levels, and MMP1 was positively correlated with macrophage M0 infiltration levels (Figure 8C). These data indicated that the prognostic signature correlated with immune cell infiltration in pancreatic cancer samples.
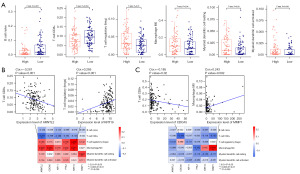
Discussion
Pancreatic cancer is a devastating disease with poor prognosis. One of the current challenges is the inability to diagnose patients in a timely manner, which is a key factor in effectively avoiding distant metastases and improving patient survival. Accumulating evidence has shown that exosomes and their cargo have the potential to mediate the progression of pancreatic cancer and are promising non-invasive biomarkers for the early detection and prognosis of this malignancy (31-33). To reveal the potential effects of tumor-derived exosomal genes in pancreatic cancer, we screened pancreatic cancer-related exosomal DEGs based on TCGA and exoRBase 2.0 data and then built a tumor-derived exosomal gene signature using six DEGs (ARNTL2, FHL2, KRT19, MMP1, CDCA5, and KIF11) that showed high predictive performance for prognosis. In addition, this prognostic signature is associated with the differential activation of several pathways, such as the cell cycle, and the infiltration of some immune cells, such as Tregs and CD8+ T cells. These findings provide important information for future pancreatic cancer research.
Exosomes in bodily fluids, such as blood, provide a rich source of biomarkers for multiple diseases because they reflect the pathological state of cells (34,35). Tumor cells secrete numerous exosomes, and tumor-derived exosomes contain various bioactive molecules that exert various functions in cancer progression (36). Fu et al. revealed that exosomal tripartite motif-containing 3 plays a tumor-suppressive role in gastric cancer (37). Shimizu et al. have reported that exosomal CD47 plays a key role in immune evasion in ovarian cancer (38). Moutinho-Ribeiro et al. demonstrated that the expression of glypican-1 is elevated in the circulating exosomes of patients with pancreatic ductal adenocarcinoma, which might be mined as a reliable biomarker for the diagnosis of pancreatic ductal adenocarcinoma (39). These data indicate that exosomal genes are the main contributors to cancer development and progression. Moreover, a growing number of studies have recently sought to explore prognostic exosomal gene signatures using public databases. For instance, Zhu et al. established a novel exosomal gene signature consisting of two exosomal genes (MYL6B and THOC2) that can predict hepatocellular carcinoma prognosis and may guide individualized treatment of patients (40). Li et al. identified a 19-exosomal gene signature that could predict the outcomes of lung adenocarcinoma (41). However, there have been no studies on prognostic gene signatures constructed using exosomal genes in pancreatic cancer. To fill this gap, we performed a comprehensive bioinformatics analysis of expression and exosome data from public databases and constructed a six-exosome gene signature that was strongly correlated with pancreatic cancer prognosis.
Our exosomal gene signature showed high prognostic prediction value in both the training and independent validation cohorts and was composed of six DEGs, including ARNTL2, FHL2, KRT19, MMP1, CDCA5, and KIF11. In the signature model, only FHL2 was a favorable gene for pancreatic cancer prognosis, whereas the other genes were associated with a poor prognosis. FHL2 is a focal adhesion adapter that plays an essential role in the regulation of multiple cellular functions, such as cell survival, by interacting with other cellular proteins (42). Zienert et al. have demonstrated that FHL2 plays a key role in regulating the survival and radioresistance of pancreatic cancer cells (43). ARNTL2 is a member of the PER-ARNT-SIM (PAS) superfamily and encodes a basic helix–loop–helix transcription factor. Wang et al. revealed that ARNTL2 expression was increased in pancreatic ductal adenocarcinoma and that elevated ARNTL2 expression promoted the development of pancreatic ductal adenocarcinoma and was positively related to poor prognosis (44). KRT19 overexpression is strongly linked to carcinogenesis, metastasis, and unfavorable prognosis in pancreatic cancer (45,46). MMP1, a member of the MMP family, promotes the migration and invasion of various tumor cells, including pancreatic cancer cells (47). CDCA5 is a CDCA protein implicated in the cell cycle. Xing et al. confirmed that CDCA5 expression is associated with poor prognosis in pancreatic cancer (48). Elevated expression of KIF11, one member of a kinesin essential for the configuration of the bipolar spindle, has been linked to a poor prognosis in pancreatic cancer (49,50). Notably, the pancreatic cancer-related exosomal DEGs were significantly enriched in several GO functions and pathways associated with cell cycle progression. In addition, GSEA revealed that key pathways such as the cell cycle were differentially activated between the different risk groups stratified by the exosomal gene signature. Aberrancy in cell cycle progression is a key mechanism controlling tumorigenesis and cell cycle regulators are promising anticancer therapeutic targets (51,52). Taken together, we speculate that these exosomal DEGs may contribute to pancreatic cancer by regulating the cell cycle and believe that our constructed exosomal gene signature has the potential for personalized outcome prediction for pancreatic cancer.
In addition, tumor-derived exosomes can be taken up by various immune cells, thereby changing the infiltration pattern of the tumor microenvironment and affecting the malignant behavior of tumor cells (53,54). Moreover, the immune landscape is closely associated with pancreatic cancer progression, and new immunotherapy approaches may be promising therapeutic options in the near future (55-57). Therefore, the immunological characteristics of the constructed exosomal gene signatures were explored. Antitumor immune cells, such as CD8+ T cells, were negatively related to the risk score of the exosomal gene signature, whereas immunosuppressive immune cells, such as Tregs, were positively linked to the risk score. The spatial distribution of CD8+ T cells affects the survival of pancreatic cancer patients (58). The efficacy of checkpoint blockade immunotherapy largely depends on the number and status of CD8+ T cells (59,60). Tregs exert immunosuppressive effects on the pathogenesis of pancreatic cancer (61,62). Based on our findings, we speculate that these infiltrating immune cells may be responsible for the unfavorable prognosis of high-risk patients.
Conclusions
In conclusion, our study constructed a six-exosome gene signature that can accurately predict pancreatic cancer prognosis. These exosomal genes may contribute to the development and prognosis of pancreatic cancer by affecting the activation of key pathways such as the cell cycle and the infiltration of immune cells such as CD8+ T cells and Tregs. However, further experimental validation studies are required to confirm our findings.
Acknowledgments
Funding: This study was supported by
Footnote
Reporting Checklist: The authors have completed the TRIPOD reporting checklist. Available at https://tcr.amegroups.com/article/view/10.21037/tcr-23-2354/rc
Peer Review File: Available at https://tcr.amegroups.com/article/view/10.21037/tcr-23-2354/prf
Conflicts of Interest: All authors have completed the ICMJE uniform disclosure form (available at https://tcr.amegroups.com/article/view/10.21037/tcr-23-2354/coif). The authors have no conflicts of interest to declare.
Ethical Statement: The authors are accountable for all aspects of the work in ensuring that questions related to the accuracy or integrity of any part of the work are appropriately investigated and resolved. The study was conducted in accordance with the Declaration of Helsinki (as revised in 2013).
Open Access Statement: This is an Open Access article distributed in accordance with the Creative Commons Attribution-NonCommercial-NoDerivs 4.0 International License (CC BY-NC-ND 4.0), which permits the non-commercial replication and distribution of the article with the strict proviso that no changes or edits are made and the original work is properly cited (including links to both the formal publication through the relevant DOI and the license). See: https://creativecommons.org/licenses/by-nc-nd/4.0/.
References
- Sung H, Ferlay J, Siegel RL, et al. Global Cancer Statistics 2020: GLOBOCAN Estimates of Incidence and Mortality Worldwide for 36 Cancers in 185 Countries. CA Cancer J Clin 2021;71:209-49. [Crossref] [PubMed]
- Kolbeinsson HM, Chandana S, Wright GP, et al. Pancreatic Cancer: A Review of Current Treatment and Novel Therapies. J Invest Surg 2023;36:2129884. [Crossref] [PubMed]
- Yousuf S, Qiu M, Voith von Voithenberg L, et al. Spatially Resolved Multi-Omics Single-Cell Analyses Inform Mechanisms of Immune Dysfunction in Pancreatic Cancer. Gastroenterology 2023;165:891-908.e14. [Crossref] [PubMed]
- Tarasiuk A, Mackiewicz T, Małecka-Panas E, et al. Biomarkers for early detection of pancreatic cancer - miRNAs as a potential diagnostic and therapeutic tool? Cancer Biol Ther 2021;22:347-56. [Crossref] [PubMed]
- Tan F, Li X, Wang Z, et al. Clinical applications of stem cell-derived exosomes. Signal Transduct Target Ther 2024;9:17. [Crossref] [PubMed]
- Liu C, Xia C, Xia C. Biology and function of exosomes in tumor immunotherapy. Biomed Pharmacother 2023;169:115853. [Crossref] [PubMed]
- Hui J, Zhou M, An G, et al. Regulatory role of exosomes in colorectal cancer progression and potential as biomarkers. Cancer Biol Med 2023;20:575-98. [Crossref] [PubMed]
- Lakey JRT, Wang Y, Alexander M, et al. Exosomes; a Potential Source of Biomarkers, Therapy, and Cure for Type-1 Diabetes. Int J Mol Sci 2023;24:15713. [Crossref] [PubMed]
- Li T, Jiao J, Ke H, et al. Role of exosomes in the development of the immune microenvironment in hepatocellular carcinoma. Front Immunol 2023;14:1200201. [Crossref] [PubMed]
- Skouras P, Gargalionis AN, Piperi C. Exosomes as Novel Diagnostic Biomarkers and Therapeutic Tools in Gliomas. Int J Mol Sci 2023;24:10162. [Crossref] [PubMed]
- Jo H, Shim K, Jeoung D. Exosomes: Diagnostic and Therapeutic Implications in Cancer. Pharmaceutics 2023;15:1465. [Crossref] [PubMed]
- Fang X, Lan H, Jin K, et al. Pancreatic cancer and exosomes: role in progression, diagnosis, monitoring, and treatment. Front Oncol 2023;13:1149551. [Crossref] [PubMed]
- Lai H, Li Y, Zhang H, et al. exoRBase 2.0: an atlas of mRNA, lncRNA and circRNA in extracellular vesicles from human biofluids. Nucleic Acids Res 2022;50:D118-28. [Crossref] [PubMed]
- Zhu L, Lou Y, Xiao Q, et al. Establishment and Evaluation of Exosomes-Related Gene Risk Model in Hepatocellular Carcinoma. Biochem Genet 2024;62:698-717. [Crossref] [PubMed]
- Chen S, Hu S, Zhou B, et al. Telomere-related prognostic biomarkers for survival assessments in pancreatic cancer. Sci Rep 2023;13:10586. [Crossref] [PubMed]
- Deng B, Liao F, Liu Y, et al. Comprehensive analysis of endoplasmic reticulum stress-associated genes signature of ulcerative colitis. Front Immunol 2023;14:1158648. [Crossref] [PubMed]
- Yu Z, Qiu B, Zhou H, et al. Characterization and application of a lactate and branched chain amino acid metabolism related gene signature in a prognosis risk model for multiple myeloma. Cancer Cell Int 2023;23:169. [Crossref] [PubMed]
- Huang da W. Sherman BT, Lempicki RA. Systematic and integrative analysis of large gene lists using DAVID bioinformatics resources. Nat Protoc 2009;4:44-57. [Crossref] [PubMed]
- Huang da W. Sherman BT, Lempicki RA. Bioinformatics enrichment tools: paths toward the comprehensive functional analysis of large gene lists. Nucleic Acids Res 2009;37:1-13. [Crossref] [PubMed]
- Szklarczyk D, Kirsch R, Koutrouli M, et al. The STRING database in 2023: protein-protein association networks and functional enrichment analyses for any sequenced genome of interest. Nucleic Acids Res 2023;51:D638-46. [Crossref] [PubMed]
- Franz M, Lopes CT, Fong D, et al. Cytoscape.js 2023 update: a graph theory library for visualization and analysis. Bioinformatics 2023;39:btad031. [Crossref] [PubMed]
- Hosseinpour Z, Rezaei Tavirani M, Akbari ME. Stage Analysis of Breast Cancer Metabolomics: A System Biology Approach. Asian Pac J Cancer Prev 2023;24:1571-82. [Crossref] [PubMed]
- Yin X, Wu Q, Hao Z, et al. Identification of novel prognostic targets in glioblastoma using bioinformatics analysis. Biomed Eng Online 2022;21:26. [Crossref] [PubMed]
- Zeng J, Lai C, Luo J, et al. Functional investigation and two-sample Mendelian randomization study of neuropathic pain hub genes obtained by WGCNA analysis. Front Neurosci 2023;17:1134330. [Crossref] [PubMed]
- Langfelder P, Horvath S. WGCNA: an R package for weighted correlation network analysis. BMC Bioinformatics 2008;9:559. [Crossref] [PubMed]
- Wagener M, Förster S. Fast calculation of scattering patterns using hypergeometric function algorithms. Sci Rep 2023;13:780. [Crossref] [PubMed]
- Yang J, Zhou F, Zhou X, et al. Survival and prognosis analysis of systemic lupus erythematosus patients with pulmonary hypertension: A systematic review and meta-analysis. Medicine (Baltimore) 2023;102:e34947. [Crossref] [PubMed]
- Huang J, Zhang J, Zhang F, et al. Identification of a disulfidptosis-related genes signature for prognostic implication in lung adenocarcinoma. Comput Biol Med 2023;165:107402. [Crossref] [PubMed]
- Sun T, Wang M, Liang W, et al. Revealing mechanism of Methazolamide for treatment of ankylosing spondylitis based on network pharmacology and GSEA. Sci Rep 2023;13:15370. [Crossref] [PubMed]
- Wei C, Wei Y, Cheng J, et al. Identification and verification of diagnostic biomarkers in recurrent pregnancy loss via machine learning algorithm and WGCNA. Front Immunol 2023;14:1241816. [Crossref] [PubMed]
- Liu H, Qiao S, Fan X, et al. Role of exosomes in pancreatic cancer. Oncol Lett 2021;21:298. [Crossref] [PubMed]
- Nakamura K, Zhu Z, Roy S, et al. An Exosome-based Transcriptomic Signature for Noninvasive, Early Detection of Patients With Pancreatic Ductal Adenocarcinoma: A Multicenter Cohort Study. Gastroenterology 2022;163:1252-1266.e2. [Crossref] [PubMed]
- Channon LM, Tyma VM, Xu Z, et al. Small extracellular vesicles (exosomes) and their cargo in pancreatic cancer: Key roles in the hallmarks of cancer. Biochim Biophys Acta Rev Cancer 2022;1877:188728. [Crossref] [PubMed]
- Di Donato M, Medici N, Migliaccio A, et al. Exosomes: Emerging Modulators of Pancreatic Cancer Drug Resistance. Cancers (Basel) 2023;15:4714. [Crossref] [PubMed]
- Huang Q, Zhong X, Li J, et al. Exosomal ncRNAs: Multifunctional contributors to the immunosuppressive tumor microenvironment of hepatocellular carcinoma. Biomed Pharmacother 2024;173:116409. [Crossref] [PubMed]
- Castillo-Peña A, Molina-Pinelo S. Landscape of tumor and immune system cells-derived exosomes in lung cancer: mediators of antitumor immunity regulation. Front Immunol 2023;14:1279495. [Crossref] [PubMed]
- Fu H, Yang H, Zhang X, et al. Exosomal TRIM3 is a novel marker and therapy target for gastric cancer. J Exp Clin Cancer Res 2018;37:162. [Crossref] [PubMed]
- Shimizu A, Sawada K, Kobayashi M, et al. Exosomal CD47 Plays an Essential Role in Immune Evasion in Ovarian Cancer. Mol Cancer Res 2021;19:1583-95. [Crossref] [PubMed]
- Moutinho-Ribeiro P, Batista IA, Quintas ST, et al. Exosomal glypican-1 is elevated in pancreatic cancer precursors and can signal genetic predisposition in the absence of endoscopic ultrasound abnormalities. World J Gastroenterol 2022;28:4310-27. [Crossref] [PubMed]
- Zhu J, Tang B, Gao Y, et al. Predictive Models for HCC Prognosis, Recurrence Risk, and Immune Infiltration Based on Two Exosomal Genes: MYL6B and THOC2. J Inflamm Res 2021;14:4089-109. [Crossref] [PubMed]
- Li J, Gao X, Tian S, et al. Exploring exosome data to identify prognostic gene signatures for lung adenocarcinoma. Future Oncol 2021;17:4745-56. [Crossref] [PubMed]
- Pan B, Wan L, Li Y, et al. Comprehensive pan-cancer analysis identifies FHL2 associated with poor prognosis in lung adenocarcinoma. Transl Cancer Res 2023;12:1516-34. [Crossref] [PubMed]
- Zienert E, Eke I, Aust D, et al. LIM-only protein FHL2 critically determines survival and radioresistance of pancreatic cancer cells. Cancer Lett 2015;364:17-24. [Crossref] [PubMed]
- Wang Z, Liu T, Xue W, et al. ARNTL2 promotes pancreatic ductal adenocarcinoma progression through TGF/BETA pathway and is regulated by miR-26a-5p. Cell Death Dis 2020;11:692. [Crossref] [PubMed]
- Li W, Li T, Sun C, et al. Identification and prognostic analysis of biomarkers to predict the progression of pancreatic cancer patients. Mol Med 2022;28:43. [Crossref] [PubMed]
- Sun Z, Zhou R, Dai J, et al. KRT19 is a Promising Prognostic Biomarker and Associates with Immune Infiltrates in Serous Ovarian Cystadenocarcinoma. Int J Gen Med 2023;16:4849-62. [Crossref] [PubMed]
- Qiu J, Feng M, Yang G, et al. PRKRA promotes pancreatic cancer progression by upregulating MMP1 transcription via the NF-κB pathway. Heliyon 2023;9:e17194. [Crossref] [PubMed]
- Xing C, Wang Z, Zhu Y, et al. Integrate analysis of the promote function of Cell division cycle-associated protein family to pancreatic adenocarcinoma. Int J Med Sci 2021;18:672-84. [Crossref] [PubMed]
- Gu X, Zhu Q, Tian G, et al. KIF11 manipulates SREBP2-dependent mevalonate cross talk to promote tumor progression in pancreatic ductal adenocarcinoma. Cancer Med 2022;11:3282-95. [Crossref] [PubMed]
- Shi S, Guo D, Ye L, et al. Knockdown of TACC3 inhibits tumor cell proliferation and increases chemosensitivity in pancreatic cancer. Cell Death Dis 2023;14:778. [Crossref] [PubMed]
- Oropeza E, Seker S, Carrel S, et al. Molecular portraits of cell cycle checkpoint kinases in cancer evolution, progression, and treatment responsiveness. Sci Adv 2023;9:eadf2860. [Crossref] [PubMed]
- Shimada R, Ishiguro KI. Cell cycle regulation for meiosis in mammalian germ cells. J Reprod Dev 2023;69:139-46. [Crossref] [PubMed]
- Głuszko A, Szczepański MJ, Ludwig N, et al. Exosomes in Cancer: Circulating Immune-Related Biomarkers. Biomed Res Int 2019;2019:1628029. [Crossref] [PubMed]
- Kugeratski FG, Kalluri R. Exosomes as mediators of immune regulation and immunotherapy in cancer. FEBS J 2021;288:10-35. [Crossref] [PubMed]
- Dolton G, Rius C, Wall A, et al. Targeting of multiple tumor-associated antigens by individual T cell receptors during successful cancer immunotherapy. Cell 2023;186:3333-3349.e27. [Crossref] [PubMed]
- Goodman RS, Johnson DB, Balko JM. Corticosteroids and Cancer Immunotherapy. Clin Cancer Res 2023;29:2580-7. [Crossref] [PubMed]
- Yi M, Li T, Niu M, et al. Exploiting innate immunity for cancer immunotherapy. Mol Cancer 2023;22:187. [Crossref] [PubMed]
- Picard FSR, Lutz V, Brichkina A, et al. IL-17A-producing CD8(+) T cells promote PDAC via induction of inflammatory cancer-associated fibroblasts. Gut 2023;72:1510-22. [Crossref] [PubMed]
- Liu Z, Zhang Y, Ma N, et al. Progenitor-like exhausted SPRY1(+)CD8(+) T cells potentiate responsiveness to neoadjuvant PD-1 blockade in esophageal squamous cell carcinoma. Cancer Cell 2023;41:1852-1870.e9. [Crossref] [PubMed]
- van der Leun AM, Traets JJH, Vos JL, et al. Dual Immune Checkpoint Blockade Induces Analogous Alterations in the Dysfunctional CD8+ T-cell and Activated Treg Compartment. Cancer Discov 2023;13:2212-27. [Crossref] [PubMed]
- Boelaars K, Goossens-Kruijssen L, Wang D, et al. Unraveling the impact of sialic acids on the immune landscape and immunotherapy efficacy in pancreatic cancer. J Immunother Cancer 2023;11:e007805. [Crossref] [PubMed]
- Tang T, Huang X, Lu M, et al. Transcriptional control of pancreatic cancer immunosuppression by metabolic enzyme CD73 in a tumor-autonomous and -autocrine manner. Nat Commun 2023;14:3364. [Crossref] [PubMed]