An ensemble learning model for predicting cancer-specific survival of muscle-invasive bladder cancer patients undergoing bladder preservation therapy
Highlight box
Key findings
• Ensemble learning predicts cancer-specific survival in patients with muscle-invasive bladder cancer undergoing bladder preservation therapy.
What is known and what is new?
• Bladder preservation therapy for muscle-invasive bladder cancer has a high mortality and recurrence rate.
• Ensemble learning has good predictive performance and clinical utility for muscle-invasive bladder cancer treated with bladder preservation therapy.
What is the implication, and what should change now?
• More advanced models are needed to predict patients with different characteristics. In addition, follow-up strategies for muscle-invasive bladder cancer treated with bladder preservation therapy may need to be adjusted.
Introduction
Bladder cancer (BC) is the second most common genitourinary system malignancy after male prostate cancer. Cancer statistics predict that 83,190 estimated new cases of BC will be diagnosed and this will lead to 16,840 deaths in 2024 in the United States. These figures represent 4.2% of all estimated new cancer cases and 2.8% of estimated cancer deaths (1). At the time of initial diagnosis, approximately 25% of BC patients present with muscle-invasive bladder cancer (MIBC), and the prognosis for this condition is significantly worse when compared to those with non-MIBC (NMIBC). The guidelines from both the National Comprehensive Cancer Network and the European Association of Urology recommend radical cystectomy (RC) as the gold-standard treatment for patients with MIBC (2,3). However, the bladder’s functions are complex and difficult to replace. Additionally, RC and urinary diversion are very invasive types of surgery that can lead to many complications for patients and these procedures can seriously impair their quality of life as well as their dignity (4-6). For these reasons, some patients either would not tolerate or are unwilling to undergo RC. Since it is difficult to achieve ideal tumor control with a single treatment regimen, the current bladder-preserving therapy (BPT) is primarily a triple combination of surgery, chemotherapy and radiotherapy (also known as trimodality). The advancement of comprehensive treatment research of BC has led to BPT being available to an increasing number of patients with MIBC (7-10).
MIBC patients receiving BPT have an increased probability of tumor recurrence and metastasis when compared to RC, necessitating closer follow-up and aggressive combination therapy for early detection and control of disease progression (11,12). Currently relevant prediction models suffer from small sample sizes and poor prediction performance, a model that can accurately predict their prognosis is urgently needed, and this would be significant for developing treatment plans and follow-up strategies (13,14).
With these considerations in mind, we downloaded a large dataset of MIBC patients receiving BPT from the Surveillance, Epidemiology and End Results (SEER) database. Then we used ensemble learning algorithms to analyze the impact of a series of factors, including demographic characteristics, the American Joint Committee on Cancer’s (AJCC) Tumor, Node, Metastasis (TNM) stage, tumor size, tumor location, histological grade and treatment methods on patients’ cancer-specific survival (CSS). Finally, we identified an optimal model for accurately predicting CSS, with an emphasis on predicting 3- and 5-year CSS in these patients. This model may assist clinicians in making more informed clinical judgements and developing effective follow-up strategies aiming at improving the prognosis of these patients. We present this article in accordance with the TRIPOD reporting checklist (available at https://tcr.amegroups.com/article/view/10.21037/tcr-24-561/rc).
Methods
Data acquisition and patient selection
SEER is a population-based cancer registry with information from 18 of the 50 states representing all country regions, and it covers around a quarter of all cancer patients in the United States (15,16). We requested access to the SEER database from the National Cancer Institute, and all authors adhered to the SEER database’s guidelines throughout the study. Since the clinical data in this study are anonymous, individual informed consent is not required. The study was conducted in accordance with the Declaration of Helsinki (as revised in 2013).
Case lists were downloaded from the SEER 18 Regs Custom Data (with additional treatment fields) using the SEER*Stat 8.3.9.1 software. Cases meeting the following inclusion criteria were used for this study: (I) diagnosed with BC between 2004 and 2016; (II) BC as the first primary malignancy; (III) no history of other malignancies; (IV) histologically confirmed urothelial carcinoma of the bladder; (V) AJCC T stage limited to T2, T3, and T4; (VI) AJCC TNM staging derived from preoperative examinations or transurethral resection of bladder tumor (TURBT) specimens; (VII) received BPT treatment. In addition, the criteria for exclusion were as follows: unknown information from the AJCC TNM stage, age, race, gender, tumor location, tumor size, differentiation grade, radiotherapy record, chemotherapy record and the number of survival months. We further excluded patients who died from non-BC causes and patients who were lost to follow-up within the model prediction period. The data selection procedure is shown in Figure S1. The cases data for this study can be found in Tables available at https://cdn.amegroups.cn/static/public/tcr-24-561-1.xlsx.
Feature selection and predictive targets
We obtained 11 clinicopathological and demographic characteristics from the SEER database, including age at diagnosis, gender, race, AJCC TNM stage, tumor size, tumor location, histological grade, radiotherapy and chemotherapy records, which were selected as independent variables for analysis. Tumor location included the trigone (including the bladder neck and ureteric orifice) and dome as well as the lateral, anterior and posterior walls of the bladder. Correlation coefficients between features were analyzed using the Spearman correlation test. The predictive targets of our models were the 3- and 5-year CSS.
Statistical analysis
This study’s statistical analyses and model establishment were performed using Python (version 3.9.13, Python Software Foundation). The patients were randomly allocated into a training set and a test set at a ratio of 7:3. Various models were constructed using the training set, and their efficacy was assessed using the test set. Subsequently, to determine whether there were disparities in the distribution of baseline features between the training and test sets, the Mann-Whitney U and Chi-squared (χ2) tests were utilized for analyzing continuous and categorical variables, respectively. For continuous variables, the distribution was presented as median (interquartile range), and for categorical variables, as frequencies (%).
Model construction and performance comparison
We used the training set to establish four models based on Catboost, Random Forest (RF), Stacking (including Support Vector Machine, Decision Tree, and Logistic Regression), and traditional Logistic Regression (LR) to predict the 3- and 5-year CSS of patients. Random grid search was used for fine-tuning the models. Through repeated debugging, the hyperparameters of the models were confirmed, enabling all four algorithms to fully learn and analyze the data from the training set. After successfully constructing the models, their predictive performance was evaluated using the test set.
The following procedures were taken to compare the model performance. Initially, receiver operating characteristic (ROC) curve analysis was used to assess model distinguishability, and prediction accuracy was compared using the area under curves (AUC). Second, the reliability of the models was assessed using calibration plots. Third, net benefits and clinical utility were assessed using decision curve analysis (DCA) and clinical impact curves (CICs).
Interpretation of the model
In order to interpret complex models, we used SHapley Additive exPlanations (SHAP) based on cooperative game theory. The bar charts showed the relative importance of different features; the beeswarm plots showed the contribution of different feature values to the prediction for each case. In this study, the selected model was interpreted using SHAP values, which may make it easier for physicians to comprehend the rationale behind the model predictions.
Results
Baseline characteristics of cohorts
Ultimately, our study included 2,374 and 2,179 patients for analysis in order to establish the 3- and 5-year models, respectively. The median ages of the patients were 74 and 75 years, and the median survival times were 16 and 14 months, respectively. Table 1 presents the results of the other basic characteristics and variance analysis for the overall population and the training and test sets for the 3- and 5-year cohorts. The Mann-Whitney U and Chi-squared (χ2) tests showed no statistical difference between the feature distributions of the training and test sets (3-year CSS: age P=0.98, tumor size P=0.89, survival months P=0.18, race P=0.36, gender P=0.91, tumor location P=0.48, T stage P=0.15, N stage P=0.37, M stage P=0.80, histological grade P=0.39, radiation P=0.29, chemotherapy P=0.60. 5-year CSS: age P=0.13, tumor size P=0.50, survival months P=0.90, race P=0.84, gender P=0.54, tumor location P=0.21, T stage P=0.59, N stage P=0.94, M stage P=0.10, histological grade P=0.054, radiation P=0.33, chemotherapy P=0.07). In addition, the Spearman correlation heatmap showed no moderate or strong correlations between the different characteristics (Figure S2).
Table 1
Variables | 3-year CSS | 5-year CSS | |||||||
---|---|---|---|---|---|---|---|---|---|
Overall | Training | Test | P | Overall | Training | Test | P | ||
Total | 2,374 (100.0) | 1,661 (70.0) | 713 (30.0) | – | 2,179 (100.0) | 1,525 (70.0) | 654 (30.0) | – | |
Age (years) | 74 [19] | 74 [19] | 75 [18] | 0.98 | 75 [19] | 74 [19] | 75 [18] | 0.13 | |
Tumor size (mm) | 40 [23] | 40 [23] | 41 [21] | 0.89 | 42 [25] | 43 [25] | 40 [21] | 0.50 | |
Survival months | 16 [47] | 16 [45] | 17 [48] | 0.18 | 14 [46] | 14 [45] | 13 [48] | 0.90 | |
Race | 0.36 | 0.84 | |||||||
Black | 169 (7.1) | 111 (6.7) | 58 (8.1) | 155 (7.1) | 108 (7.1) | 47 (7.2) | |||
Other | 133 (5.6) | 97 (5.8) | 36 (5.1) | 123 (5.7) | 89 (5.8) | 34 (5.2) | |||
White | 2,072 (87.3) | 1,453 (87.5) | 619 (86.8) | 1,901 (87.2) | 1,328 (87.1) | 573 (87.6) | |||
Gender | 0.91 | 0.54 | |||||||
Female | 682 (28.7) | 476 (28.7) | 206 (28.9) | 633 (29.1) | 437 (28.7) | 196 (30.0) | |||
Male | 1,692 (71.3) | 1,185 (71.3) | 507 (71.1) | 1,546 (70.9) | 1,088 (71.3) | 458 (70.0) | |||
Tumor location | 0.48 | 0.21 | |||||||
Trigone | 538 (22.7) | 371 (22.3) | 167 (23.4) | 490 (22.5) | 349 (22.9) | 141 (21.6) | |||
Dome | 299 (12.6) | 198 (11.9) | 101 (14.2) | 274 (12.6) | 182 (11.9) | 92 (14.1) | |||
Lateral | 949 (40.0) | 677 (40.8) | 272 (38.1) | 880 (40.4) | 630 (41.3) | 250 (38.2) | |||
Anterior | 179 (7.5) | 129 (7.8) | 50 (7.0) | 155 (7.1) | 112 (7.3) | 43 (6.6) | |||
Posterior | 409 (17.2) | 286 (17.2) | 123 (17.3) | 380 (17.4) | 252 (16.5) | 128 (19.6) | |||
T stage | 0.15 | 0.59 | |||||||
T2 | 1,906 (80.3) | 1,350 (81.3) | 556 (78.0) | 1,728 (79.3) | 1,213 (79.6) | 515 (78.7) | |||
T3 | 259 (10.9) | 175 (10.5) | 84 (11.8) | 245 (11.2) | 174 (11.4) | 71 (10.9) | |||
T4 | 209 (8.8) | 136 (8.2) | 73 (10.2) | 206 (9.5) | 138 (9.0) | 68 (10.4) | |||
N stage | 0.37 | 0.94 | |||||||
N0 | 2,124 (89.5) | 1,492 (89.8) | 632 (88.6) | 1,935 (88.8) | 1,354 (88.8) | 581 (88.8) | |||
N1 | 151 (6.3) | 106 (6.4) | 45 (6.3) | 148 (6.8) | 105 (6.9) | 43 (6.6) | |||
N2 | 99 (4.2) | 63 (3.8) | 36 (5.0) | 96 (4.4) | 66 (4.3) | 30 (4.6) | |||
M stage | 0.80 | 0.10 | |||||||
M0 | 2,058 (86.7) | 1,438 (86.6) | 620 (87.0) | 1,864 (85.5) | 1,292 (84.7) | 572 (87.5) | |||
M1 | 316 (13.3) | 223 (13.4) | 93 (13.0) | 315 (14.5) | 233 (15.3) | 82 (12.5) | |||
Histological grade | 0.39 | 0.054 | |||||||
Low grade | 110 (4.6) | 81 (4.9) | 29 (4.1) | 104 (4.8) | 64 (4.2) | 40 (6.1) | |||
High grade | 2,264 (95.4) | 1,580 (95.1) | 684 (95.9) | 2,075 (95.2) | 1,461 (95.8) | 614 (93.9) | |||
Radiation | 0.29 | 0.33 | |||||||
No/unknown | 1,700 (71.6) | 1,200 (72.2) | 500 (70.1) | 1,568 (72.0) | 1,088 (71.3) | 480 (73.4) | |||
Yes | 674 (28.4) | 461 (27.8) | 213 (29.9) | 611 (28.0) | 437 (28.7) | 174 (26.6) | |||
Chemotherapy | 0.60 | 0.07 | |||||||
No/unknown | 1,361 (57.3) | 958 (57.7) | 403 (56.5) | 1,273 (58.4) | 872 (57.2) | 401 (61.3) | |||
Yes | 1,013 (42.7) | 703 (42.3) | 310 (43.5) | 906 (41.6) | 653 (42.8) | 253 (38.7) |
Continuous variables are shown as median [interquartile range] and classification variables are shown as frequency and percentages. MIBC, muscle-invasive bladder cancer; CSS, cancer-specific survival.
Model performance comparison
We used ROC curves to evaluate the discrimination of the four models, with the AUC representing the predictive accuracy of each model. The ROC curve showed that the Catboost and RF models had the highest predictive accuracy in the training sets of both the 3- and 5-year CSS (AUC =0.85 and 0.87, respectively), compared with Stacking (AUC =0.82 and 0.84, respectively) and LR (AUC =0.74 and 0.75, respectively) (Figure 1A,1B). The accuracy of the Catboost and RF models with the test set of the 3- and 5-year CSS was also greater than that of other models (AUC =0.80 and 0.83 for each model, respectively) (Figure 1C,1D).
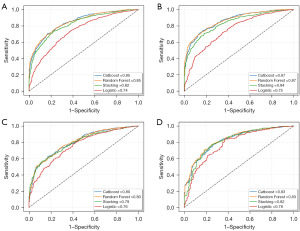
The calibration plots illustrate the alignment between the model predictions and the actual events. The 45-degree straight line on each graph represents the perfect match between the actual events (y-axis) and predicted events (x-axis). The closer the distance between the two curves, the higher the calibration accuracy. The calibration plots for both sets of patients at the 3- and 5-year CSS demonstrated that all models’ calibration plots closely aligned with the ideal 45-degree diagonal. Notably, the Catboost and Stacking models exhibited the highest concordance between predicted probabilities and actual survival rates, followed by the RF and LR models, respectively (Figure 2).
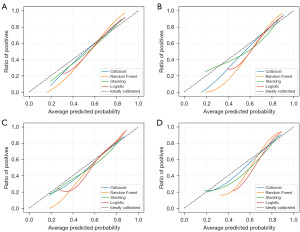
We used DCA to further assess the clinical utility and net benefit of the models. DCA estimates the net benefit by calculating the difference between the true positive rate and the false positive rate, weighted by the odds of the selected risk threshold probability. The DCA graphs are shown in Figure 3. DCA curves showed that each of the models achieved a net clinical benefit. Among them, the three ensemble learning models had higher net benefits, with the Catboost models of the 3- and 5-year CSS achieving the highest net benefit across the whole probability threshold, narrowly ahead of the RF model. In contrast, the traditional LR model had the lowest net benefit across the whole probability threshold.
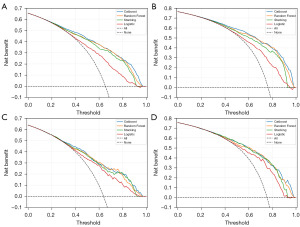
CIC is a novel clinical evaluation method developed by Kerr et al. and is based on DCA (17). Figure 4 shows the CIC of the predictive models in the test set. The four solid curves indicate the number of cases predicted as death by the four different models at each threshold probability. The four dashed curves refer to the actual number of deaths that occurred out of the number of cases predicted as deaths by the model. According to the curves, the number of death cases predicted by the Catboost and Stacking models were closest to the actual number of death cases. As the risk threshold increased, the number of cases predicted by both models quickly approached the actual number that occurred.
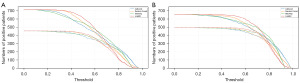
Model interpretation
Through a series of comparisons and evaluations, we selected the Catboost model for its high accuracy, reliability and net benefit as the final model for predicting the 3- and 5-year CSS of MIBC patients receiving BPT. SHAP values were further calculated for the Catboost model to assess the importance of each feature on the predictive outcome. Figure 5 shows the interpretation of SHAP, which indicated that age, M stage, tumor size, chemotherapy, T stage and gender were the most important features in the model for predicting the 3-year CSS. In contrast, M stage, age, tumor size, gender, N stage and T stage were the most important features in the model for predicting the 5-year CSS. Patients who were older, female, at a more advanced stage and not receiving chemotherapy with their tumors located in the trigone had a relatively worse prognosis compared to the other patients.
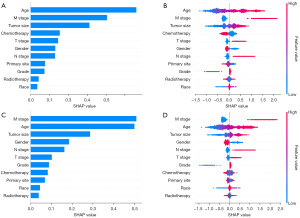
Model deployment
As mentioned above, we selected the relatively best-performing Catboost model and used it to develop an online risk calculator (https://weiliwei666-bladder-streamapp-6cdcfq.streamlit.app/#real-time-prediction) for predicting the 3- and 5-year CSS in MIBC patients receiving BPT.
Discussion
MIBC represents approximately 25% of newly diagnosed BC and it is often a highly aggressive disease with a significantly worse prognosis than NMIBC (18). The current standard therapy for MIBC is neoadjuvant chemotherapy followed by RC, an approach that could lead to severe complications. However, with the development of various lines of research on BPT, some carefully-selected MIBC patients can achieve acceptable tumor control results and a better quality of life (19-22). The American Society of Clinical Oncology, American Urological Association, Society of Urologic Oncology and American Society for Radiation Oncology (ASCO/AUA/SUO/ASRO) joint guidelines state that clinicians should consider BPT in patients with non-metastatic MIBC who wish to preserve their bladder or who have severe comorbidities that preclude radical surgery (23). Since MIBC patients receiving BPT have a higher rate of tumor recurrence, an individualized treatment and follow-up strategy would be advantageous to both the patient and the clinician. With this in mind, a predictive model that can stratify MIBC patients and accurately predict their prognosis would be a valuable tool.
There is a need of more research on the prognosis of MIBC patients receiving BPT. Koga et al. analyzed the 5-year CSS of 170 patients with cT2-4aN0M0 bladder cancer who received initial BPT and presented their associated risk stratification and risk factors (14). However, the study was descriptive, and it included only a small number of cases and it lacked predictive ability. Coen et al. used 325 cases to establish a nomogram to predict the complete response as well as the CSS and disease-free survival in MIBC patients receiving BPT. Nevertheless, the relatively small sample size (325 cases), the small number of included features (6 features) and the poor accuracy of the nomogram (Harrell concordance index =0.61) led to its still somewhat flawed clinical application (13).
In this study, we evaluated the predictive performance and clinical utility of four different models using ROC curves, calibration plots, DCA and CIC, respectively. The ROC curve compared the differentiation of the models, and this suggested that the Catboost and RF models had the highest accuracy for both, the 3- and 5-year models (test set AUC =0.80 and 0.83 for each model, respectively), followed by Stacking (test set AUC =0.79 and 0.82, respectively) and LR (test set AUC =0.76 and 0.78, respectively). These findings indicate that the three models based on ensemble learning have higher accuracy than traditional LR. With advancements in computer hardware and model algorithms, various emerging model algorithms have been widely applied. Ensemble learning models are increasingly used in medical research due to their superior predictive performance, greater flexibility and generalization, and their ability to handle complex nonlinear data. In contrast, LR, which is derived from linear regression, has more stringent data requirements and is better suited for fitting linear data. For the nonlinear heterogeneous data obtained in medical research, ensemble learning models perform better (24).
The calibration plots for the models in the test set show that the curves for the Catboost and Stacking models were closer to the ideal 45° diagonal, indicating that their predictions were closer to reality. On the other hand, RF and LR tended to overestimate the mortality risk across the most predicted probabilities. DCA and CIC are emerging methods for evaluating the net benefit and clinical utility of a model (17,25). The DCA curves suggested that net benefits were obtained for all four models, with Catboost being the model yielding the highest net benefit, narrowly ahead of the RF model. The CIC results illustrated that the predictions of all four models converge towards the actual results as the prediction threshold increases. In contrast, the predictions of the Catboost and Stacking models were closest to the actual data and they converged faster as the threshold increased.
Finally, to explain our model, we used SHAP values to analyze the importance of each feature on the prediction results. The SHAP results indicated slight differences in the importance of the features between the 3- and 5-year models. Age, M stage, tumor size, chemotherapy, T stage and gender were the most important features in the model for predicting the 3-year CSS. The M stage, age, tumor size, gender, N stage and T stage were the most important features in the model for predicting the 5-year CSS.
Age at diagnosis had a crucial role in our models and previous studies have also shown a higher mortality rate in older patients with BC. This may be due to poorer tumor differentiation, more delayed disease diagnosis and comorbidities in the older population (26). In addition, the 3- and 5-year CSS were worse for female and black patients, although the exact mechanism to explain this remains unclear. Various epidemiological investigations have shown that female and black BC patients have more advanced disease and a worse prognosis, which may be due to differences in genetics, anatomy, sex hormones, economic status and environmental factors (27). Another feature of interest was tumor location, with tumors in the trigone appearing to have a worse 3-year CSS. In addition, tumors located in the trigone and bladder neck have been reported to be associated with a more advanced stage of the disease as well as lymph node involvement. Both of these would lead to a poorer prognosis for the patient (28).
Several studies have demonstrated the role of chemotherapy in patients with MIBC (29,30). Notably, in our models, receiving chemotherapy improved the 3-year CSS of patients, while it is debatable whether there was any improvement for the 5-year CSS. On the other hand, radiotherapy contributed less to the predicted results of both the 3- and 5-year models, and its role in this patient population still needs to be further investigated. Notably, our web calculator shows a more positive effect of radiotherapy and chemotherapy in patients with a higher risk of death.
Despite several strengths, our study also has some limitations. Firstly, this study relies on retrospective data, and to bolster the clinical application of this model, it is imperative to incorporate prospective clinical data. Secondly, the unknown status of radiotherapy and chemotherapy for some patients may affect the model’s predictions. Clear clinical data needs to be collected in the future to improve the model’s efficacy. Thirdly, understaging after TURBT is a common clinical issue, which may lead to inaccurate predictions by the model. More accurate staging data needs to be collected in the future for retraining the model. Fourthly, the model’s dataset is exclusively derived from the North American population, which may limit its generalizability. Finally, the developed model needs external validation in different centers, which can increase the robustness of the model.
In summary, we established a model to predict the 3- and 5-year CSS of MIBC patients receiving BPT based on a novel Catboost algorithm. Our model has excellent predictive performance and might assist clinicians in better managing such patients.
Conclusions
The Catboost model demonstrates superior predictive accuracy and clinical utility in predicting CSS among MIBC patients undergoing BPT, potentially aiding clinicians in optimizing personalized treatment decisions for this patient population.
Acknowledgments
The authors extend their appreciation to Dr. Dev Sooranna from Imperial College London for providing English language editing assistance for the manuscript.
Funding: This study was sponsored by
Footnote
Reporting Checklist: The authors have completed the TRIPOD reporting checklist. Available at https://tcr.amegroups.com/article/view/10.21037/tcr-24-561/rc
Peer Review File: Available at https://tcr.amegroups.com/article/view/10.21037/tcr-24-561/prf
Conflicts of Interest: All authors have completed the ICMJE uniform disclosure form (available at https://tcr.amegroups.com/article/view/10.21037/tcr-24-561/coif). The authors have no conflicts of interest to declare.
Ethical Statement: The authors are accountable for all aspects of the work in ensuring that questions related to the accuracy or integrity of any part of the work are appropriately investigated and resolved. This study was conducted in accordance with the Declaration of Helsinki (as revised in 2013).
Open Access Statement: This is an Open Access article distributed in accordance with the Creative Commons Attribution-NonCommercial-NoDerivs 4.0 International License (CC BY-NC-ND 4.0), which permits the non-commercial replication and distribution of the article with the strict proviso that no changes or edits are made and the original work is properly cited (including links to both the formal publication through the relevant DOI and the license). See: https://creativecommons.org/licenses/by-nc-nd/4.0/.
References
- Siegel RL, Giaquinto AN, Jemal A. Cancer statistics, 2024. CA Cancer J Clin 2024;74:12-49. [Crossref] [PubMed]
- Flaig TW, Spiess PE, Agarwal N, et al. Bladder Cancer, Version 3.2020, NCCN Clinical Practice Guidelines in Oncology. J Natl Compr Canc Netw 2020;18:329-54. [Crossref] [PubMed]
- Witjes JA, Bruins HM, Cathomas R, et al. European Association of Urology Guidelines on Muscle-invasive and Metastatic Bladder Cancer: Summary of the 2020 Guidelines. Eur Urol 2021;79:82-104. [Crossref] [PubMed]
- Hampson A, Vincent A, Dasgupta P, et al. Radical cystectomy complications and perioperative mortality. BJU Int 2019;124:3-4. [Crossref] [PubMed]
- Nieuwenhuijzen JA, de Vries RR, Bex A, et al. Urinary diversions after cystectomy: the association of clinical factors, complications and functional results of four different diversions. Eur Urol 2008;53:834-42; discussion 842-4. [Crossref] [PubMed]
- Kretschmer A, Grimm T, Buchner A, et al. Midterm Health-related Quality of Life After Radical Cystectomy: A Propensity Score-matched Analysis. Eur Urol Focus 2020;6:704-10. [Crossref] [PubMed]
- Shi H, Zhang W, Bi X, et al. Neoadjuvant Chemotherapy-Guided Bladder-Sparing Treatment for Muscle-Invasive Bladder Cancer: Results of a Pilot Phase II Study. Cancer Res Treat 2021;53:1156-65. [Crossref] [PubMed]
- Coen JJ, Zhang P, Saylor PJ, et al. Bladder Preservation With Twice-a-Day Radiation Plus Fluorouracil/Cisplatin or Once Daily Radiation Plus Gemcitabine for Muscle-Invasive Bladder Cancer: NRG/RTOG 0712-A Randomized Phase II Trial. J Clin Oncol 2019;37:44-51. [Crossref] [PubMed]
- Balar AV, Kamat AM, Kulkarni GS, et al. Pembrolizumab monotherapy for the treatment of high-risk non-muscle-invasive bladder cancer unresponsive to BCG (KEYNOTE-057): an open-label, single-arm, multicentre, phase 2 study. Lancet Oncol 2021;22:919-30. [Crossref] [PubMed]
- Varughese M, Treece S, Drinkwater KJ. Radiotherapy Management of Muscle Invasive Bladder Cancer: Evaluation of a National Cohort. Clin Oncol (R Coll Radiol) 2019;31:637-45. [Crossref] [PubMed]
- Konieczkowski DJ, Efstathiou JA, Mouw KW. Contemporary and Emerging Approaches to Bladder-Preserving Trimodality Therapy for Muscle-Invasive Bladder Cancer. Hematol Oncol Clin North Am 2021;35:567-84. [Crossref] [PubMed]
- Zhiyu Z, Qi Z, Zhen S, et al. The Effect of Tri-Modality Therapy with Bladder Preservation for Selective Muscle-Invasive Bladder Cancer. Technol Cancer Res Treat 2021;20:15330338211062323. [Crossref] [PubMed]
- Coen JJ, Paly JJ, Niemierko A, et al. Nomograms predicting response to therapy and outcomes after bladder-preserving trimodality therapy for muscle-invasive bladder cancer. Int J Radiat Oncol Biol Phys 2013;86:311-6. [Crossref] [PubMed]
- Koga F, Fujii Y, Masuda H, et al. Pathology-based risk stratification of muscle-invasive bladder cancer patients undergoing cystectomy for persistent disease after induction chemoradiotherapy in bladder-sparing approaches. BJU Int 2012;110:E203-8. [Crossref] [PubMed]
- Doll KM, Rademaker A, Sosa JA. Practical Guide to Surgical Data Sets: Surveillance, Epidemiology, and End Results (SEER) Database. JAMA Surg 2018;153:588-9. [Crossref] [PubMed]
- Wu WT, Li YJ, Feng AZ, et al. Data mining in clinical big data: the frequently used databases, steps, and methodological models. Mil Med Res 2021;8:44. [Crossref] [PubMed]
- Kerr KF, Brown MD, Zhu K, et al. Assessing the Clinical Impact of Risk Prediction Models With Decision Curves: Guidance for Correct Interpretation and Appropriate Use. J Clin Oncol 2016;34:2534-40. [Crossref] [PubMed]
- Cumberbatch MGK, Jubber I, Black PC, et al. Epidemiology of Bladder Cancer: A Systematic Review and Contemporary Update of Risk Factors in 2018. Eur Urol 2018;74:784-95. [Crossref] [PubMed]
- Giacalone NJ, Shipley WU, Clayman RH, et al. Long-term Outcomes After Bladder-preserving Tri-modality Therapy for Patients with Muscle-invasive Bladder Cancer: An Updated Analysis of the Massachusetts General Hospital Experience. Eur Urol 2017;71:952-60. [Crossref] [PubMed]
- Mak RH, Hunt D, Shipley WU, et al. Long-term outcomes in patients with muscle-invasive bladder cancer after selective bladder-preserving combined-modality therapy: a pooled analysis of Radiation Therapy Oncology Group protocols 8802, 8903, 9506, 9706, 9906, and 0233. J Clin Oncol 2014;32:3801-9. [Crossref] [PubMed]
- Johnson SB, Yu JB. Bladder Preserving Trimodality Therapy for Muscle-Invasive Bladder Cancer. Curr Oncol Rep 2018;20:66. [Crossref] [PubMed]
- Fabiano E, Durdux C, Dufour B, et al. Long-term outcomes after bladder-preserving tri-modality therapy for patients with muscle-invasive bladder cancer. Acta Oncol 2021;60:794-802. [Crossref] [PubMed]
- Ghandour R, Singla N, Lotan Y. Treatment Options and Outcomes in Nonmetastatic Muscle Invasive Bladder Cancer. Trends Cancer 2019;5:426-39. [Crossref] [PubMed]
- Schober P, Vetter TR. Logistic Regression in Medical Research. Anesth Analg 2021;132:365-6. [Crossref] [PubMed]
- Vickers AJ, Elkin EB. Decision curve analysis: a novel method for evaluating prediction models. Med Decis Making 2006;26:565-74. [Crossref] [PubMed]
- Madeb R, Messing EM. Gender, racial and age differences in bladder cancer incidence and mortality. Urol Oncol 2004;22:86-92. [Crossref] [PubMed]
- Fajkovic H, Halpern JA, Cha EK, et al. Impact of gender on bladder cancer incidence, staging, and prognosis. World J Urol 2011;29:457-63. [Crossref] [PubMed]
- Weiner AB, Desai AS, Meeks JJ. Tumor Location May Predict Adverse Pathology and Survival Following Definitive Treatment for Bladder Cancer: A National Cohort Study. Eur Urol Oncol 2019;2:304-10. [Crossref] [PubMed]
- Adjuvant Chemotherapy for Muscle-invasive Bladder Cancer. A Systematic Review and Meta-analysis of Individual Participant Data from Randomised Controlled Trials. Eur Urol 2022;81:50-61. [Crossref] [PubMed]
- James ND, Hussain SA, Hall E, et al. Radiotherapy with or without chemotherapy in muscle-invasive bladder cancer. N Engl J Med 2012;366:1477-88. [Crossref] [PubMed]