Prognostic value of PLEKHA4 and its correlation with tumor-infiltrating immune cells in breast cancer: a comprehensive study based on bioinformatics and clinical analysis validation
Highlight box
Key findings
• A potential prognostic marker for breast cancer was identified.
What is known and what is new?
• It has been previously reported that pleckstrin homology containing family A, number 4 (PLEKHA4) is regulated in glioma and is associated with poor prognosis in glioma patients
• In this study, we found that PLEKHA4 expression was down-regulated or absent in breast cancer and was strongly associated with poor patient prognosis.
• Our findings indicate that PLEKHA4 is an independent prognostic biomarker associated with key signaling pathways and immune infiltration in breast cancer.
What is the implication, and what should change now?
• PLEKHA4 has been linked to the prognosis of breast cancer patients and may exert an antitumor effect in breast cancer. However, further experimental studies are required to confirm the underlying biological regulatory mechanisms.
Introduction
Breast cancer (BC) is a prevalent malignant tumor worldwide, characterized by a significant incidence and fatality rate (1). Globally, around 2,570,000 people received a BC diagnosis in 2020, and the reported number of deaths was approximately 626,000 (2). Most current research classifies BC into four molecular subtypes: luminal A, luminal B, human epidermal growth factor receptor 2 (HER2)-positive, and triple-negative BC (TNBC) (3,4). This classification is based on the assessment of biological markers such as estrogen receptor (ER), progesterone receptor (PR), proliferation index Ki-67, and HER2 (5). Typically, BC expressing hormone receptors have the best prognosis, showing the highest response to endocrine therapy. However, resistance to endocrine therapy also poses a significant challenge. HER2-positive BCs have a moderate prognosis and can be targeted with corresponding drugs. Conversely, patients with TNBC generally face a more unfavorable prognosis as these are limited treatment avenues available and a higher likelihood of immune evasion (6). Studies have shown that TNBC patients with p53 positivity or high Ki-67 index have a poorer prognosis. In addition, it is crucial to take into account of other predictive factors, including age, tumor grade, vascular invasion, lymph node metastasis, tumor-node-metastasis (TNM) stage, and tumor-infiltrating immune cells (7-9). Due to the heterogeneity and complexity of tumors, existing biomarkers have limited effectiveness in predicting BC prognosis (10). Therefore, there is an urgent need for the discovery of novel molecular biomarkers as prognostic indicators to improve patient prognosis and guide personalized treatment strategies in clinical practice.
The use of bioinformatics methods and online analysis tools to identify key target genes associated with tumor initiation and progression has become a trend in cancer research due to the emergence of high-throughput and next-generation sequencing (NGS) technologies. The aim is to elucidate the mechanisms of tumor development and provide more accurate treatment strategies to improve patient prognosis. For example, Zheng et al. identified the HOX antisense intergenic RNA (HOTAIR)-miR-130a-3p-high mobility group-box 3 (HMGB3) axis as a potential prognostic marker in BC through bioinformatics analysis (11). Zhong et al. revealed that conventional dendritic cell (cDC) scores in the tumor microenvironment (TME) are a key predictor of BC prognosis and that cDCs may play an anti-tumor role by up-regulating IL-2 based on TCGA database (12). During our previous investigation, we employed bioinformatics techniques to uncover the pleckstrin homology containing family A, number 4 (PLEKHA4) gene. The outcomes of our research reveal a notable increase in PLEKHA4 expression in glioma and its strong association with the TME. Moreover, we observed that the upregulation of PLEKHA4 expression in glioma patients is linked to an unfavorable prognosis (13). PLEKHA4 is crucially involved in regulating the organization and functioning of cells, including activities like cell movement and cell attachment (14). Moreover, it has been found to be associated with the onset and progression of various types of tumors, including glioma and melanoma (15,16). These findings suggest that PLEKHA4 may be an important immune-related prognostic marker in tumors, but its prognostic value in BC has not been elucidated.
In this study, in order to investigate the expression and associated functions of PLEKHA4 in BC, we conducted immunohistochemistry (IHC) staining to confirm the decreased expression of PLEKHA4 in invasive BC tissues compared to corresponding normal tissues. To further explore the correlation between PLEKHA4 and clinicopathologic features in BC patients, we analyzed tissue microarray (TMA) data. Additionally, we utilized gene set enrichment analysis (GSEA) to identify gene sets enriched in the low and high expression groups of PLEKHA4, as well as the biological processes related to PLEKHA4. Furthermore, we employed the R software to examine the potential relationship between PLEKHA4 and the infiltration of immune cells. This study provides evidence suggesting that PLEKHA4 may be a promising prognostic biomarker of clinical value. We present this article in accordance with the REMARK reporting checklist (available at https://tcr.amegroups.com/article/view/10.21037/tcr-24-67/rc).
Methods
Data collection
We obtained a total of 106 cases diagnosed with infiltrating ductal BC (not otherwise specified), along with 106 non-tumor tissues, from female patients who had undergone initial surgical treatment at The Third Affiliated Hospital of Guangzhou Medical University between January 2013 and May 2022. Cases with indistinct or poorly fixed infiltrative lesions, poorly stained tissue, and incomplete clinical information were excluded. The patients had a median age of 57 years, ranging from 18 to 86 years. None of the 106 patients had previously received chemotherapy, radiotherapy, or other surgeries. High-density TMAs were prepared using well-fixed, paraffin-embedded BC tissues stored at room temperature. The microarrays contained 106 1.0-mm cores. Follow-up data were available for 80 cases, with a follow-up period ranging from 12 to 96 months (median, 54 months). The histopathology and clinical stage of the BC were defined according to the 8th edition of the TNM staging system of the American Joint Committee on Cancer (AJCC). In this study, 59 cases were in stage I + II, and 47 cases were in stage III + IV. Detailed clinicopathological data were obtained from medical records. Table S1 summarizes the detailed clinical and pathological features of the study population.
The study was conducted in accordance with the Declaration of Helsinki (as revised in 2013). The study was approved by the Ethics Review Committee of The Third Affiliated Hospital of Guangzhou Medical University (No. 2024207) and individual consent for this retrospective analysis was waived.
Bioinformatics analysis
To assess the messenger RNA (mRNA) level of PLEKHA4 in various cancer types, we utilized the Tumor Immune Estimation Resource (TIMER)2.0 web server (http://timer.cistrome.org/) to analyze data from The Cancer Genome Atlas (TCGA) and compared them to corresponding adjacent normal tissues. Additionally, we employed the “expression correlation” test from the “TCGA analysis module” in UALCAN (http://ualcan.path.uab.edu/analysis-prot.html) network resources to identify the major subtypes and TP53 mutation status of BC based on sample types. In addition, we gathered PLEKHA4 data from the publicly available TCGA database. By using the Toil process in UCSC XENA (https://xenabrowser.net/datapages/) (17), we standardized the TCGA Genotype-Tissue Expression (GTEx) and transcripts per million (TPM) RNA sequencing (RNAseq) data formats. Subsequently, we extracted BC data from TCGA along with corresponding GTEx normal tissues. We obtained PLEKHA4 expression profiles from RNAseq data of 1,226 BC patients in the TCGA database (https://portal.gdc.cancer.gov). The dataset comprised a total of 1,226 RNAseq samples and 113 paracancer samples. Among these, 1,098 samples had associated clinical information, including instances where clinical data was available without corresponding RNAseq data. Conversely, 1,198 RNAseq samples had accompanying clinical information, including cases where RNAseq data was available without corresponding clinical information. Notably, there were 18 RNAseq samples derived from the same patient. A comprehensive summary of the clinical and pathological characteristics of the study population is provided in Table S2. To gather clinical prognostic information such as overall survival (OS), disease-specific survival (DSS), and progression-free interval (PFI), we excluded controls/normals (since not all projects had controls/normals) and retained clinical data from the datasets mentioned above. The RNAseq data was then transformed to a format similar to microarray-derived data. Specifically, the PLEKHA4-related data was converted from TPM high-throughput sequencing format to fragments per kilobase million (FPKM) format and preserved. We extracted clinical data and prognostic information including OS, DSS, and PFI from all available samples in this study. The R software (version 4.2.1) was utilized to perform proportional hazard hypothesis testing, employing the survival package to fit survival regressions. The results were subsequently visualized using the survminer and ggplot2 packages.
Analysis of PLEKHA4 gene mutation in BC through cBioPortal
Using the cBioPortal online tool (http://www.cbioportal.org), we investigated the mutation status of the PLEKHA4 gene in 1,098 patients with BC. Using cBioPortal (TCGA provisional), we created an OncoPrint diagram that illustrates the different alterations in the PLEKHA4 gene observed in BC. These alterations include mRNA upregulation, mRNA downregulation, gene amplification, and deep deletion. We also plotted a distribution histogram to show the frequency of PLEKHA4 gene mutations in BC tissues from various sources. Furthermore, in cBioPortal, we generated Kaplan-Meier survival curves to assess the impact of PLEKHA4 gene mutations on disease-free survival and OS in a group of 1,098 BC patients.
Immune cell infiltration analysis
Gene set variation analysis (GSVA) is a computational technique that utilizes gene expression data and incorporates the immune infiltration algorithm known as single sample GSEA (ssGSEA). This algorithm allows for the assessment of the relative abundance of immune cells infiltrating tumors in specimens (18). We obtained 24 immune cell markers and their related data from previously published studies and ran ssGSEA in R software to analyze gene expression data from BC specimens to detect the abundance ratio of the 24 immune cells (19,20). Finally, we selected the data with statistical differences (P<0.05) from 1,226 samples, which were divided into two groups on the basis of median expression of PLEKHA4.
Analysis of gene set enrichment
GSEA allows for the analysis of whole-genome expression profile microarray data by comparing genes to predefined gene sets. Through GSEA, it is possible to examine the expression patterns of genes within specific functional gene sets (21). To investigate the biological pathways connected to the progression of BC, we obtained 1,226 GSEA samples from the TCGA database. These samples were classified into two groups based on the expression levels of PLEKHA4: the high expression group (n=613) and the low expression group (n=613) using the median expression as the threshold. We then evaluated the enrichment of pathways for each phenotype by ranking them according to the normalized enrichment score (NES) and the nominal P value, using the expression profiles of PLEKHA4 as the basis.
TMAs block construction
Tissue cores from donor paraffin-embedded tissue blocks were obtained by drilling using a micro drill bit that had a stainless-steel capillary attached to it. The capillary, along with the tissue core, was removed from the micro drill bit using a stainless-steel wire and then transferred into the holes of the recipient block. This procedure was repeated to introduce the desired tissue cores into the prepared holes of the recipient block, ultimately creating a TMA block. The specific preparation method for TMA was conducted as previously described (22).
Hematoxylin and eosin (H&E) and IHC
Standard procedures were conducted to stain H&E on BC paraffin slides. We used a two-step method to perform IHC staining on paraffin-embedded tissue sections. PLEKHA4 protein was detected using a rabbit polyclonal antibody against PLEKHA4 (clone number: nbp1-47331; Novus, Littleton, CO, USA). Briefly, tissue sections were subjected to 10 minutes of pH 9.0 ethylenediaminetetraacetic acid (EDTA) buffer antigen retrieval using a high-pressure boiler. Next, the sections underwent incubation with a diluted 1:150 rabbit anti-PLEKHA4 antibody at a temperature of 37 ℃ for a duration of 2 hours. Following this, the sections were washed and subsequently incubated with a secondary antibody for a period of 30 minutes at room temperature. Finally, 3,3'-diaminobenzidine (DAB) staining reagent was used for visualization. According to the staining intensity and staining ratio of cancer cells, PLEKHA4 immunostaining was interpreted. The intensity of cancer cell staining was scored as follows: 1, weak staining; 2, moderate staining; 3, strong staining. Percentage scoring was based on the proportion of stained cancer cells: 0, negative or <10%; 1, 10–30%; 2, >30–50%; 3, >50%. The final PLEKHA4 IHC score was calculated by multiplying the tumor cell staining intensity score by its percentage score. Cancer cell staining intensity and proportion scores were assessed independently by two experienced pathologists who blinded to patients’ information. In the present study, PLEKHA4 was classified as low [0–4] or high [5–9] expression.
Statistical analysis
For statistical analysis, we utilized SPSS 26.0 software. Specifically, we employed the Pearson’s chi-squared test (χ2 test) to examine the correlation between PLEKHA4 expression and clinicopathological parameters. R software was utilized to organize and conduct the TCGA statistics. Logistic regression was employed to analyze the relationship between PLEKHA4 expression and clinical information. Survival curves were constructed using the Kaplan-Meier method, and the log-rank test was used to assess differences between the survival curves. Univariate and multivariate Cox regression analyses were conducted on TCGA RNAseq data to evaluate whether PLEKHA4 expression serves as an independent prognostic factor. By adjusting for variables such as patient age, cancer stage, ER status, PR status, HER2 status, and radiotherapy, PLEKHA4 was assessed as an independent prognostic factor for OS in BC patients. Statistical significance was determined with a threshold of P<0.05. Univariate Cox proportional hazards estimates were used to determine individual hazard ratios (HRs) for OS. Statistically significant results from the univariate analyses were included in the multivariate analyses. The risk associated with individual factors was measured using 95% confidence intervals (CIs). P values <0.05 were considered significant and a two-tailed approach was used when reporting P values.
Results
Research process
The overall workflow diagram for this study was depicted in Figure 1. The training cohort consisted of 1,226 samples from TCGA, whereas the clinical analysis validation sample was derived from 106 patients. The demographic and clinicopathologic characteristics of these patients were presented in Tables S1,S2, respectively.
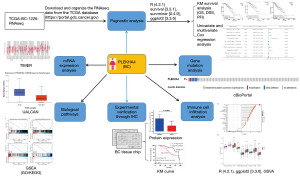
PLEKHA4 is abnormally downregulated in BC
We utilized the TIMER2.0 online analysis website to examine the levels of mRNA expression of PLEKHA4 across various types of cancer, using data from the TCGA database. The findings indicated a significant decrease in PLEKHA4 expression in eight cancer types, including BC, compared with the normal control group (P<0.001). Conversely, PLEKHA4 expression was higher in the remaining eight cancer types (Figure 2A). Moreover, the UALCAN web tool validated a substantial downregulation of PLEKHA4 expression in BC compared with normal samples (P<0.001) (Figure 2B). Additionally, significant variations in PLEKHA4 expression were observed in association with TP53 mutation status, BC subtype, and MYC amplification status (Figure 2C-2E). In order to further investigate the significance of PLEKHA4 expression, we conducted IHC staining on a total of 106 samples, comprising both BC and non-tumor tissues. Consistent with our previous findings, the IHC staining revealed a marked decrease in PLEKHA4 protein expression in BC tissues compared with adjacent normal tissues (Figure 3A,3B). Subsequently, the IHC staining of TMA sections demonstrated differential PLEKHA4 expression in BC. The high magnification images clearly depicted two distinct tissue spots on the right (Figure 3C,3D). Furthermore, the protein levels of PLEKHA4 were significantly correlated with age (P=0.01), molecular subtype (P=0.001), lymph node metastasis (P=0.004), and tumor TNM stage (P<0.001), as shown in Table 1.
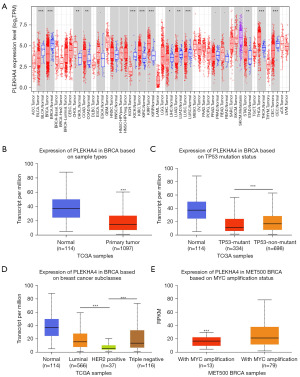
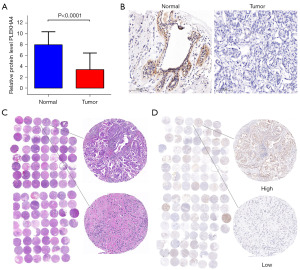
Table 1
Clinicopathological variables | Total, n | PLEKHA4 expression, n | c2 | P | |
---|---|---|---|---|---|
Low expression | High expression | ||||
Age (years) | 6.394 | 0.01 | |||
≤50 | 37 | 7 | 30 | ||
>50 | 69 | 30 | 39 | ||
Tumor size (cm) | 2.899 | 0.90 | |||
<5 | 89 | 28 | 61 | ||
≥5 | 17 | 9 | 8 | ||
Grade | 0.709 | 0.70 | |||
I | 14 | 4 | 10 | ||
II | 35 | 14 | 21 | ||
III | 57 | 19 | 38 | ||
Molecular subtype | 15.606 | 0.001 | |||
Luminal A | 32 | 20 | 12 | ||
Luminal B | 55 | 12 | 43 | ||
HER2 positive | 5 | 1 | 4 | ||
Basl-like | 14 | 4 | 10 | ||
Lymph metastasis | 13.753 | 0.004 | |||
Yes | 25 | 1 | 24 | ||
No | 81 | 36 | 45 | ||
TNM classification | 22.616 | <0.001 | |||
I–II | 59 | 9 | 50 | ||
III–IV | 47 | 28 | 19 |
PLEKHA4, pleckstrin homology containing family A, number 4; HER2, human epidermal growth factor receptor 2; TNM, tumor-node-metastasis.
Relationship between PLEKHA4 expression and clinicopathologic characteristics of BC patients
We obtained important clinical data of BC patients from the TCGA database and then proceeded to examine the connection between PLEKHA4 expression levels and clinicopathological variables using the Wilcoxon rank sum test. Figure 4 demonstrates that there was a noteworthy correlation between PLEKHA4 expression and PR status (P<0.001), HER2 status (P<0.001), and molecular subtype (P<0.001) in BC patients. However, PLEKHA4 expression had a negligible relationship with ER status.
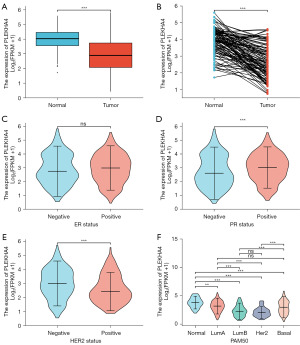
PLEKHA4 predicts poor prognosis in BC patients
A total of 1,226 BC samples were downloaded by us, with the exclusion of control/normal samples (not all programs had control/normal samples). We then retained the clinical information of the patients. The analysis of the data revealed that patients with low expression of PLEKHA4 had significantly shorter OS, DSS, and progression-free survival (PFI) compare with patients with high expression (all log-rank P<0.001, as shown in Figure 5A-5C. The Kaplan-Meyer survival analysis conducted in our cohort demonstrated that patients with lower PLEKHA4 had a shorter OS, which was consistent with the findings obtained from public databases (P=0.01, HR =0.46; 95% CI: 0.20–0.90; as illustrated in Figure 5D). Furthermore, in univariate analysis, age (HR =2.020; 95% CI: 1.465–2.784; P<0.001), T stage (HR =1.608; 95% CI: 1.110–2.329; P=0.01), N stage (HR =2.239, 95% CI 1.567–3.199; P<0.001), M stage (HR =4.254; 95% CI: 2.468–7.334; P<0.001), stage (HR =2.391; 95% CI: 1.703–3.355; P<0.001), radiation therapy (HR =0.576; 95% CI: 0.394–0.841; P=0.004), and PLEKHA4 (HR =0.610; 95% CI: 0.441–0.845; P=0.003) were associated with OS. Moreover, in multivariate analysis, age (HR =3.750; 95% CI: 1.979–7.109; P<0.001), stage (OS: HR =3.759; 95% CI: 1.360–10.388; P=0.01), radiation therapy (OS: HR =0.486; 95% CI: 0.257–0.919; P=0.03), and PLEKHA4 (HR =0.911; 95% CI: 0.479–1.731; P=0.006) were associated with OS (Figure 6).
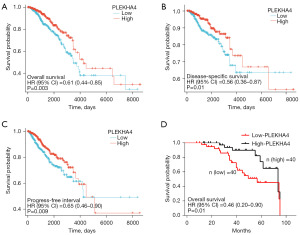
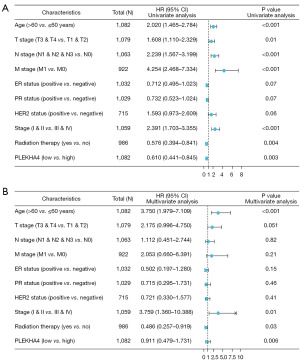
Gene variation of PLEKHA4 in BC patients (cBioPortal)
By analyzing the OncoPrint figure, we found that about 6% (66 out of 1,098) of the sequenced samples had gene variations in PLEKHA4, which included nine amplifications, 56 deep deletions and one missense mutation (Figure 7A). Further evaluation was conducted to examine the association between PLEKHA4 gene variations and the survival of BC patients. We further evaluated the relationship between PLEKHA4 gene variants and survival in BC patients. Kaplan-Meier survival curves showed no significant correlation between PLEKHA4 gene variants and OS in BC patients, but there was a statistically significant correlation between the gene variants and disease-free survival (Figure 7B,7C).
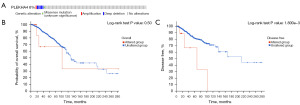
GSEA analysis of PLEKHA4-related signaling pathways
In order to identify the signaling pathways that are influenced by PLEKHA4 in BC, we conducted a differential analysis comparing low and high expression of PLEKHA4 using GSEA. The expression levels of genes in BC, samples were divided into two groups, high and low, based on the median as a threshold. GSEA analysis revealed significant disparities in the enrichment of the Molecular Signature Database (MSigDB) collection (c2.cp.reactome/biocarta/kegg.v6.2.symbols.gmt). We selected the most significant signaling pathways that were enriched based on the standard NES. The results showed differences in enrichment for the cell cycle checkpoint, Notch signaling pathway, nuclear factor erythroid 2-related factor 2 (NRF2) pathway, and Rho GTPases activating protein kinases N (PKNs) classification in cases with low expression of PLEKHA4 (Figure 8).
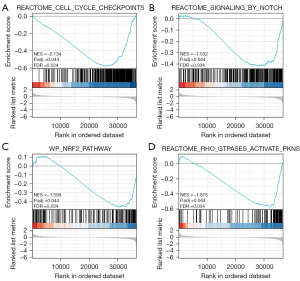
PLEKHA4 positively correlates with tumor-infiltrating immune cells
Afterwards, we conducted ssGSEA enrichment analysis using R software to investigate the association between PLEKHA4 and immune cells in the microenvironment of BC. We examined the expression data of genes from BC patients and determined the relative abundance ratios of 24 different types of immune cells. Subsequently, we divided the data from 1,226 samples into two groups based on the median expression level of PLEKHA4, including only those with a P value of less than 0.05. Spearman correlation analysis was employed to establish the correlation between high or low expression of PLEKHA4 and various concentrations of immune cells. As depicted in Figure 9A, PLEKHA4 showed the strongest positive correlation with natural killer (NK) cells (P<0.001) and conversely, the strongest negative correlation with helper T (Th) cells (P<0.001). Our findings revealed that PLEKHA4 expression significantly impacted NK cells, CD8 T cells, plasmacytoid dendritic cells (pDCs), interdigitating dendritic cells (iDCs), NK CD56dim cells, neutrophils, T cells, and B cells, as illustrated in Figure 9B.
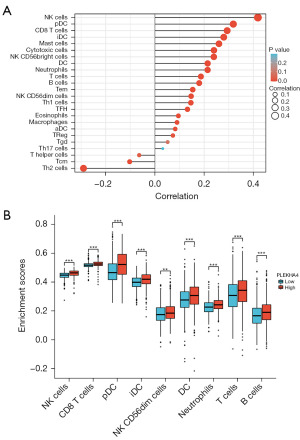
Discussion
PLEKHA4, also recognized as PEPP1, is a phosphate domain-containing protein, the function of which remains largely uncharacterized. Recently, Professor Shami discovered that PLEKHA4 hinders disheveled ubiquitination, which consequently regulates Wnt and planar cell polarity signaling in typical mammalian cells (14). Studies have documented the high expression of PLEKHA4 in melanoma and glioma, along with its involvement in tumor development (15,23). However, PLEKHA4 expression and its role in BC have not yet been reported. In this investigation, we aim to primarily elucidate the distinct functions of PLEKHA4 in BC.
This study explored the clinical and pathological relevance of PLEKHA4 in BC by utilizing prepared TMAs and analyzing gene expression data from publicly available databases. Interestingly, we discovered that PLEKHA4 was abnormally under-expressed or absent in BC, which contradicts previous studies (13,15,23). We speculated that PLEKHA4 may play a tumor suppressive role in BC. To validate this hypothesis, we first examined expression of PLEKHA4 in BC samples and their corresponding normal tissues. The results of the IHC analysis indicated that PLEKHA4 expression was significantly lower in BC tissues compared to adjacent tissues. Furthermore, the expression of PLEKHA4 was found to be negatively correlated with age, clinical stage, and lymph node metastasis of BC patients. Additionally, the expression of PLEKHA4 varied among different molecular subtypes of BC, with the highest rate of negative expression observed in HER2-overexpressing BC and the lowest rate in luminal A BC. Patients with high PR expression may benefit from endocrine therapy, resulting in a better prognosis (24). However, patients with high expression of p53, Ki-67, and amplification of HER2 have been found to be associated with a poor prognosis (25-27). Upon analyzing the relationship between PLEKHA4 expression and these common prognostic biomarkers in BC, it was discovered that the expression of PLEKHA4 had a negative correlation with expression of p53, HER2, and Ki-67, and a positive correlation with the expression of PR. Based on the presented evidence, PLEKHA4 had the potential to act as a protective prognostic factor for individuals with BC. Conversely, a decrease or absence of PLEKHA4 expression suggested a less favorable prognosis. Our suspicions were further supported by the analysis of Kaplan-Meier survival curves. Patients with low levels of PLEKHA4 mRNA experienced worse OS, DSS, and PFI. Furthermore, in the TCGA dataset, cox multivariate regression analysis demonstrated that PLEKHA4 mRNA expression independently predicts prognosis in BC patients. These results align with the findings of our cohort study, which evaluated survival in BC patients with varying PLEKHA4 protein levels. Taken together, these findings suggested that PLEKHA4 has the potential to serve as a novel prognostic marker for individuals with BC.
It is hypothesized that the downregulation of PLEKHA4 expression may predict malignant progression in patients with BC. In order to fully understand the mechanism of PLEKHA4 expression abnormality in BC, we explored the genetic changes of PLEKHA4 in BC using data from cBioPortal. In PLEKHA4 gene-altered BC cases, deep deletion was the main type of genetic change, which was possibly caused by abnormal expression of upstream molecules that regulate target mRNA expression, a typical molecular mechanism of downstream mRNA expression (28). Alterations in the PLEKHA4 gene may be explained by aberrant activity of related genes that could cooperate with PLEKHA4 to promote carcinogenesis. Additional research is required to validate the involvement of PLEKHA4-interacting genes in the development of BC. Furthermore, GSEA analysis was conducted using TCGA data. The analysis revealed enrichment of various pathways in the low expression phenotype of PLEKHA4, including the cell cycle checkpoint, Notch signaling pathway, NRF2 pathway, and Rho GTPase activated by PKNs. It is well known that dysregulation of cell cycle control is a hallmark of cancer development. Cyclin D, CDK4, CDK6, and retinoblastoma proteins are crucial in regulating cell proliferation in BC. They also interact with ERs and the HER2 signaling pathway (29). The Rho GTPase, a member of the Ras GTPase superfamily, is particularly important in BC as it significantly impacts its development, metastasis, and response to treatment (30). These clues imply that PLEKHA4 is involved in cell cycle regulation and activation of GTPases. Currently, ongoing studies are investigating the role of the Notch signaling pathway in tumor immune surveillance. Recent literature has shown that changes in Notch gene expression in different T cell subsets, such as CD4+ and CD8+, help regulate the anti-tumor immune response (31,32). BC is significantly influenced by NRF2. Tumors with elevated NRF2 levels present a strong presence of immune cells, like CD8+ and CD4+ T cells, dendritic cells (DCs), and connective tissue cells, including adipocytes, fibroblasts, and keratinocytes. Additionally, these tumors exhibit low ratios of Th1 cells (33). Tumor-infiltrating lymphocytes (TILs) interact with various cell types to either promote or regulate tumor progression and growth (34). There is a hypothesis that PLEKHA4 may be linked to the infiltration of immune cells in BC. To explore this, we used R software to conduct ssGSEA analysis. The findings revealed a notable positive relationship between PLEKHA4 expression and the levels of NK cells, CD8+ T cells, DCs, neutrophils, and B cells infiltrating in BC. Our findings indicated that the expression of PLEKHA4 in BC was correlated with various immune cells and their markers, implying its potential role in regulating the tumor immune microenvironment.
Several limitations need to be taken into account when evaluating this study. To begin with, it should be noted that this study is retrospective in nature and only includes data from our unit. In order to validate our findings, it is necessary to conduct prospective studies and gather multicenter data. Additionally, this study solely relies on bioinformatics methods to predict the biological function of PLEKHA4 in BC. Further research is needed to validate its function in BC at the cellular and animal levels. Moreover, while our study suggests that the expression level of PLEKHA4 impacts immune cell types in the TME and can serve as a prognostic marker for BC, the specific mechanisms by which PLEKHA4 affects immune cell infiltration in the TME and influences tumor progression require further validation through additional clinical and basic experiments.
Conclusions
To summarize, the clinicopathologic significance of PLEKHA4 in BC patients indicates that it could serve as a novel and valuable prognostic biomarker in medical settings. The decreased expression of PLEKHA4 may play a crucial role in the development of BC. It is strongly advised to conduct further research on the molecular and immunological functions of PLEKHA4 in BC.
Acknowledgments
We would like to thank all tutors and friends for their support.
Funding: This work was supported by
Footnote
Reporting Checklist: The authors have completed the REMARK reporting checklist. Available at https://tcr.amegroups.com/article/view/10.21037/tcr-24-67/rc
Data Sharing Statement: Available at https://tcr.amegroups.com/article/view/10.21037/tcr-24-67/dss
Peer Review File: Available at https://tcr.amegroups.com/article/view/10.21037/tcr-24-67/prf
Conflicts of Interest: All authors have completed the ICMJE uniform disclosure form (available at https://tcr.amegroups.com/article/view/10.21037/tcr-24-67/coif). The authors have no conflicts of interest to declare.
Ethical Statement: The authors are accountable for all aspects of the work in ensuring that questions related to the accuracy or integrity of any part of the work are appropriately investigated and resolved. The study was conducted in accordance with the Declaration of Helsinki (as revised in 2013). The study was approved by the Ethics Review Committee of The Third Affiliated Hospital of Guangzhou Medical University (No. 2024207) and individual consent for this retrospective analysis was waived.
Open Access Statement: This is an Open Access article distributed in accordance with the Creative Commons Attribution-NonCommercial-NoDerivs 4.0 International License (CC BY-NC-ND 4.0), which permits the non-commercial replication and distribution of the article with the strict proviso that no changes or edits are made and the original work is properly cited (including links to both the formal publication through the relevant DOI and the license). See: https://creativecommons.org/licenses/by-nc-nd/4.0/.
References
- Narayan AK, Lee CI, Lehman CD. Screening for Breast Cancer. Med Clin North Am 2020;104:1007-21. [Crossref] [PubMed]
- Xia C, Dong X, Li H, et al. Cancer statistics in China and United States, 2022: profiles, trends, and determinants. Chin Med J (Engl) 2022;135:584-90. [Crossref] [PubMed]
- Tsang JYS, Tse GM. Molecular Classification of Breast Cancer. Adv Anat Pathol 2020;27:27-35. [Crossref] [PubMed]
- Roy M, Fowler AM, Ulaner GA, et al. Molecular Classification of Breast Cancer. PET Clin 2023;18:441-58. [Crossref] [PubMed]
- Chen L, Chen Y, Xie Z, et al. Comparison of immunohistochemistry and RT-qPCR for assessing ER, PR, HER2, and Ki67 and evaluating subtypes in patients with breast cancer. Breast Cancer Res Treat 2022;194:517-29. [Crossref] [PubMed]
- Waks AG, Winer EP. Breast Cancer Treatment: A Review. JAMA 2019;321:288-300. [Crossref] [PubMed]
- Harbeck N, Penault-Llorca F, Cortes J, et al. Breast cancer. Nat Rev Dis Primers 2019;5:66. [Crossref] [PubMed]
- Xiang Y, Zhang N, Lei H, et al. Neutrophil-to-lymphocyte ratio is a negative prognostic biomarker for luminal A breast cancer. Gland Surg 2023;12:415-25. [Crossref] [PubMed]
- Song XQ, Shao ZM. Identification of immune-related prognostic biomarkers in triple-negative breast cancer. Transl Cancer Res 2024;13:1707-20. [Crossref] [PubMed]
- Guo L, Kong D, Liu J, et al. Breast cancer heterogeneity and its implication in personalized precision therapy. Exp Hematol Oncol 2023;12:3. [Crossref] [PubMed]
- Zheng M, Wu L, Xiao R, et al. Integrated analysis of coexpression and a tumor-specific ceRNA network revealed a potential prognostic biomarker in breast cancer. Transl Cancer Res 2023;12:949-64. [Crossref] [PubMed]
- Zhong S, Jia Z, Zhang H, et al. Identification and validation of tumor microenvironment-related prognostic biomarkers in breast cancer. Transl Cancer Res 2021;10:4355-64. [Crossref] [PubMed]
- Zhang W, Li L, Bian PP, et al. PLEKHA4 Is a Prognostic Biomarker and Correlated with Immune Infiltrates in Glioma. Biomed Res Int 2023;2023:4504474. [Crossref] [PubMed]
- Shami Shah A, Batrouni AG, Kim D, et al. PLEKHA4/kramer Attenuates Dishevelled Ubiquitination to Modulate Wnt and Planar Cell Polarity Signaling. Cell Rep 2019;27:2157-2170.e8. [Crossref] [PubMed]
- Shami Shah A, Cao X, White AC, et al. PLEKHA4 Promotes Wnt/β-Catenin Signaling-Mediated G1-S Transition and Proliferation in Melanoma. Cancer Res 2021;81:2029-43. [Crossref] [PubMed]
- He Y, Zheng W, Huo Y, et al. PLEKHA4 promotes glioblastoma progression through apoptosis inhibition, tumor cell migration, and macrophage infiltration. Immunobiology 2023;228:152746. [Crossref] [PubMed]
- Vivian J, Rao AA, Nothaft FA, et al. Toil enables reproducible, open source, big biomedical data analyses. Nat Biotechnol 2017;35:314-6. [Crossref] [PubMed]
- Liang J, Wang X, Yang J, et al. Identification of disulfidptosis-related subtypes, characterization of tumor microenvironment infiltration, and development of a prognosis model in breast cancer. Front Immunol 2023;14:1198826. [Crossref] [PubMed]
- Bindea G, Mlecnik B, Tosolini M, et al. Spatiotemporal dynamics of intratumoral immune cells reveal the immune landscape in human cancer. Immunity 2013;39:782-95. [Crossref] [PubMed]
- Hänzelmann S, Castelo R, Guinney J. GSVA: gene set variation analysis for microarray and RNA-seq data. BMC Bioinformatics 2013;14:7. [Crossref] [PubMed]
.Deng X Thompson JA An R package for Survival-based Gene Set Enrichment Analysis. Res Sq 2023 . [Preprint]. doi: .10.21203/rs.3.rs-3367968/v1 - Zhang W, Qiu F, Jiang Q, et al. Simple method for constructing and repairing tissue microarrays using simple equipment. J Int Med Res 2021;49:3000605211000156. [Crossref] [PubMed]
- Huang B, Pan W, Wang W, et al. Overexpression of Pleckstrin Homology Domain-Containing Family A Member 4 Is Correlated with Poor Prognostic Outcomes and Immune Infiltration in Lower-Grade Glioma. Dis Markers 2022;2022:1292648. [Crossref] [PubMed]
- Zhang M, Yan M, Lv H, et al. Clinical study of first-line endocrine therapy for type ER+/PR+ and ER+/PR- advanced breast cancer. Ann Palliat Med 2021;10:238-43. [Crossref] [PubMed]
- Masci G, Agostinetto E, Giordano L, et al. Prognostic factors and outcome of HER2+ breast cancer with CNS metastases. Future Oncol 2020;16:269-79. [Crossref] [PubMed]
- Marvalim C, Datta A, Lee SC. Role of p53 in breast cancer progression: An insight into p53 targeted therapy. Theranostics 2023;13:1421-42. [Crossref] [PubMed]
- Probert J, Dodwell D, Broggio J, et al. Ki67 and breast cancer mortality in women with invasive breast cancer. JNCI Cancer Spectr 2023;7:pkad054. [Crossref] [PubMed]
- Kim HJ. Cell Fate Control by Translation: mRNA Translation Initiation as a Therapeutic Target for Cancer Development and Stem Cell Fate Control. Biomolecules 2019;9:665. [Crossref] [PubMed]
- Fedele P, Sanna V, Fancellu A, et al. A clinical evaluation of treatments that target cell cycle machinery in breast cancer. Expert Opin Pharmacother 2019;20:2305-15. [Crossref] [PubMed]
- Humphries B, Wang Z, Yang C. Rho GTPases: Big Players in Breast Cancer Initiation, Metastasis and Therapeutic Responses. Cells 2020;9:2167. [Crossref] [PubMed]
- Khosla R, Vyas AK, Trehanpati N. Dichotomy of Notch signalling in regulating tumour immune surveillance. Scand J Immunol 2019;89:e12744. [Crossref] [PubMed]
- Goruganthu MUL, Shanker A, Dikov MM, et al. Specific Targeting of Notch Ligand-Receptor Interactions to Modulate Immune Responses: A Review of Clinical and Preclinical Findings. Front Immunol 2020;11:1958. [Crossref] [PubMed]
- Oshi M, Angarita FA, Tokumaru Y, et al. High Expression of NRF2 Is Associated with Increased Tumor-Infiltrating Lymphocytes and Cancer Immunity in ER-Positive/HER2-Negative Breast Cancer. Cancers (Basel) 2020;12:3856. [Crossref] [PubMed]
- Paijens ST, Vledder A, de Bruyn M, et al. Tumor-infiltrating lymphocytes in the immunotherapy era. Cell Mol Immunol 2021;18:842-59. [Crossref] [PubMed]