The role of IQCB1 in liver cancer: a bioinformatics analysis
Highlight box
Key findings
• We explored the role of IQCB1 in liver cancer for the first time.
What is known and what is new?
• IQCB1, also known as NPHP5 or PIQ, is a gene located on the human chromosome 3q21.3 region. This gene encodes a protein known as IQ calmodulin-binding motif-containing protein 1. The protein encoded by IQCB1 plays an important role in the structure and function of primary cilia, a vital cellular component present in almost all cells of the body.
• The role of IQCB1 in liver hepatocellular carcinoma (LIHC) was previously unknown. This study identified a relationship between IQCB1 and the prognosis, immune infiltration, and clinical characteristics of LIHC.
What is the implication, and what should change now?
• IQCB1 has the potential to function as a diagnostic and prognostic molecular marker. More studies are needed to verify its roles in liver cancer.
Introduction
Liver hepatocellular carcinoma (LIHC) is a common type of liver cancer with high morbidity and mortality rates worldwide (1,2). The current treatment options for LIHC include surgical resection, transplantation, local ablation, targeted therapy, and systemic therapy. Surgical resection is considered the most effective treatment for early-stage LIHC, with a 5-year survival rate of up to 50% (3). Liver transplantation is another treatment option for patients with early-stage LIHC (4,5), but it is limited by the availability of donor organs. Local ablation techniques, such as radiofrequency ablation and cryoablation, can be used to treat tumors that are not suitable for surgical resection. Despite the advancements in LIHC treatment, the overall survival (OS) rate remains low due to the late diagnosis of LIHC, high rate of tumor recurrence and metastasis, and poor efficacy of current treatments. Therefore, there is an urgent need to develop new and more effective therapies for LIHC. Current research focuses on identifying novel therapeutic targets, developing personalized treatment strategies, and exploring immunotherapy and gene therapy as potential new treatment options.
IQCB1, also known as nephrocystin-5 (NPHP5), is associated with a ciliopathy called Senior-Løken syndrome (6,7). Ciliopathies are a group of disorders that result from defects in the structure or function of cellular cilia (8,9). Cilia are hair-like structures protruding from the surface of many cell types and are involved in various cellular processes. The relationship between IQCB1 and tumors is currently unclear. However, some studies have shown that the IQCB1 gene may be involved in regulating cell growth and differentiation, which could affect the occurrence and development of tumors (10-12); these findings still need further research and confirmation. In this study, we utilized bioinformatics data to conduct an analysis of the diagnostic, prognostic, and immunological significance of IQCB1 in liver cancer for the first time. The findings serve as a foundational framework for further investigation of IQCB1 in liver cancer and offer novel insights for future research in this field. We present this article in accordance with the TRIPOD reporting checklist (available at https://tcr.amegroups.com/article/view/10.21037/tcr-24-110/rc).
Methods
Data collection and expression analysis
This study was conducted in accordance with the Declaration of Helsinki (as revised in 2013). The RNA-sequencing data were obtained from The Cancer Genome Atlas (TCGA) database (https://portal.gdc.cancer.gov) for the TCGA-LIHC project, transcripts per million (TPM) formatted data was extracted, and data processing was performed by log2(value+1). We collected the GSE25097 dataset from the Gene Expression Omnibus (GEO) database (http://ww.ncbinlm.nih.gov/geo/), including 268 hepatocellular carcinoma (HCC) tumors, 243 adjacent non-tumors, and 40 cirrhotic and 6 healthy liver samples.
Prognostic analysis of IQCB1 gene
The proportional hazards assumption was tested, and survival regression was fitted using the survival package. The results were visualized with the survminer and ggplot2 packages. Prognostic data were obtained from Liu et al.’s study (13).
Immune infiltration analysis
Based on the single sample gene set enrichment analysis (ssGSEA) algorithm provided by R package gene set variation analysis (GSVA) (14,15), 24 types of immune cell markers were utilized to calculate the immunological infiltration of corresponding cloud data (16). Spearman correlation analysis was performed between IQCB1 and immune infiltration matrix data, and the results were visualized using the ggplot2 package.
Drug sensitivity analysis
The chemotherapeutic response for each sample was predicted based on the largest publicly available pharmacogenomics database [Genomics of Drug Sensitivity in Cancer (GDSC), https://www.cancerrxgene.org/]. The prediction process was implemented by R package “pRRophetic” (https://cran.r-project.org/). The samples’ half-maximal inhibitory concentration (IC50) was estimated by ridge regression. All parameters were set as the default values. Using the batch effect of combat and tissue type of all tissues, and the duplicate gene expression was summarized as a mean value.
Statistical analysis
For comparisons between two groups, the Wilcoxon rank sum test was used. For comparisons among three or more groups, the Kruskal-Wallis test was applied. Pairwise analysis involved conducting paired sample t-tests for analysis.
Results
IQCB1 gene expression between normal and tumor tissues
The expression data of IQCB1 messenger RNA (mRNA) in various cancers was obtained from TCGA, including adrenocortical carcinoma (ACC), bladder urothelial carcinoma (BLCA), breast invasive carcinoma (BRCA), cervical squamous cell carcinoma and endocervical adenocarcinoma (CESC), cholangiocarcinoma (CHOL), colon adenocarcinoma (COAD), lymphoid neoplasm diffuse large B-cell lymphoma (DLBC), esophageal carcinoma (ESCA), glioblastoma multiforme (GBM), head and neck squamous cell carcinoma (HNSC), kidney chromophobe (KICH), kidney renal clear cell carcinoma (KIRC), kidney renal papillary cell carcinoma (KIRP), acute myeloid leukemia (LAML), brain lower grade glioma (LGG), LIHC, lung squamous cell carcinoma (LUSC), mesothelioma (MESO), ovarian serous cystadenocarcinoma (OV), pancreatic adenocarcinoma (PAAD), paraganglioma (PCPG), prostate adenocarcinoma (PRAD), rectum adenocarcinoma (READ), sarcoma (SARC), skin cutaneous melanoma (SKCM), stomach adenocarcinoma (STAD), testicular germ cell tumors (TGCT), thyroid carcinoma (THCA), thymoma (THYM), uterine corpus endometrial carcinoma (UCEC), uterine carcinosarcoma (UCS), and uveal melanoma (UVM). Compared to normal tissues, IQCB1 mRNA was upregulated in CHOL, COAD, ESCA, GBM, HNSC, KIRC, KIRP, LIHC, LUAD, LUSC, READ, and STAD and downregulated in KICH and UCEC (Figure 1A). In LIHC samples, IQCB1 was significantly overexpressed compared to in normal tissues (Figure 1B). In the paired analysis of LIHC, IQCB1 also presented with high expression in tumor samples (Figure 1C). The area under the curve (AUC) was 0.885 [95% confidence interval (CI): 0.848–0.922] in the receiver operating characteristic (ROC) curve analysis for diagnostic purposes, indicating that IQCB1 had a better diagnostic value (Figure 1D). The protein expression of IQCB1 for liver cancer was analyzed from The University of ALabama at Birmingham CANcer data analysis Portal (UALCAN) database. IQCB1 had a significant difference in protein expression level in liver cancer and displayed upregulation compared with normal tissues (Figure 1E). Based on the analysis of the GSE25097 dataset, it was found that IQCB1 exhibited significant upregulation in both cirrhotic and tumor tissues. Moreover, a high expression of IQCB1 was also observed between tumor and adjacent non-tumor tissues in the paired analysis (Figure 1F,1G).
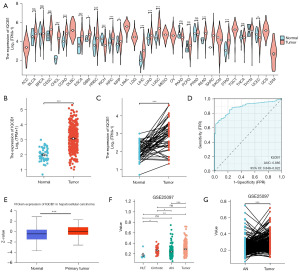
The relationship between IQCB1 gene and clinical pathology and prognosis
Based on the median expression of IQCB1, LIHC patients were divided into high and low groups. In pathological stage, higher expression of IQCB1 was found in stage III and IV than in stage I and II (Figure 2A). In pathological tumor (T) stage, higher expression of IQCB1 was found in T3 and T4 than in T1 and T2 (Figure 2B). IQCB1 expression was correlated with hepatitis B virus (HBV), hepatitis C virus (HCV), and alpha-fetoprotein (AFP). In the group of AFP ≥400 ng/mL, HBV+, and HCV+, IQCB1 was significantly overexpressed (Figure 2C-2E). The expression of IQCB1 was not correlated with node (N) stage, metastasis (M) stage, gender, age, or body mass index (BMI) of patients with LIHC (Figure 2F-2J). Ki-67 is a nuclear antigen of cell proliferation, representing the proliferation status of cells, which can be used to confirm benign tumors or malignant tumors, and is a commonly used detection index in the clinical setting. As shown in Figure 2K, IQCB1 was significantly positively correlated with MKI67 (R=0.650, P<0.001). Compared to the low expression group, the high expression group showed poorer prognosis in terms of OS, progression-free interval (PFI), and disease-specific survival (DSS) (Figure 2L-2N). In stage I, stage III, and T1, the high expression group had worse OS (Figure 2O-2Q). These results showed that IQCB1 was related to the occurrence, development, and prognosis of LIHC.
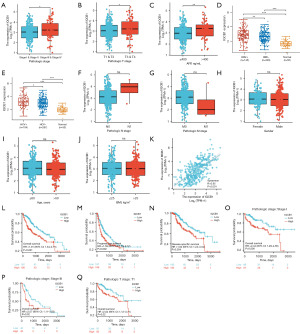
The prognostic value of IQCB1 gene
The time-dependent ROC curve results indicated that the prognostic efficiency of 1-year (AUC =0.700) was better than that of 3-year (AUC =0.620) and 5-year (AUC =0.610) (Figure 3A). A comprehensive analysis utilizing univariate and multivariate Cox regression models was conducted, focusing on the impact of pathology TNM, pathology stage, age, and IQCB1 on OS. Notably, IQCB1 emerged as a significant independent predictor of OS, with a P value of 0.001, as presented in Table 1. Subsequently, we plotted a prognostic nomogram of 1-year, 3-year, and 5-year using these indicators (Figure 3B). Prognostic calibration depicted the difference between the predicted probabilities and the actual probabilities corresponding to different time points for the model. The model fitted well with the diagonal line, indicating that the prognostic function of this model was good (Figure 3C). These results indicated that IQCB1 also had certain prognostic value in LIHC.
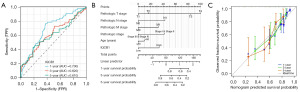
Table 1
Characteristics | Total (N) | Univariate analysis | Multivariate analysis | |||
---|---|---|---|---|---|---|
Hazard ratio (95% CI) | P value | Hazard ratio (95% CI) | P value | |||
Pathologic T stage | 370 | |||||
T1 | 183 | Reference | Reference | |||
T2 | 94 | 1.431 (0.902–2.268) | 0.12 | 1.540 (0.854–2.777) | 0.15 | |
T3 | 80 | 2.674 (1.761–4.060) | <0.001 | 2.505 (0.328–19.114) | 0.37 | |
T4 | 13 | 5.386 (2.690–10.784) | <0.001 | 3.973 (0.438–36.068) | 0.22 | |
Pathologic N stage | 258 | |||||
N0 | 254 | Reference | – | – | ||
N1 | 4 | 2.029 (0.497–8.281) | 0.32 | – | – | |
Pathologic M stage | 272 | |||||
M0 | 268 | Reference | Reference | |||
M1 | 4 | 4.077 (1.281–12.973) | 0.01 | 2.260 (0.525–9.734) | 0.27 | |
Pathologic stage | 349 | |||||
I & II | 259 | Reference | Reference | |||
III & IV | 90 | 2.504 (1.727–3.631) | <0.001 | 1.172 (0.159–8.640) | 0.87 | |
Age (years) | 373 | |||||
≤60 | 177 | Reference | – | – | ||
>60 | 196 | 1.205 (0.850–1.708) | 0.29 | – | – | |
IQCB1 | 373 | |||||
Low | 187 | Reference | Reference | |||
High | 186 | 1.908 (1.342–2.711) | <0.001 | 2.097 (1.330–3.305) | 0.001 |
LIHC, liver hepatocellular carcinoma; CI, confidence interval.
The protein-protein interaction (PPI) network of IQCB1
The 20 proteins related to IQCB1 from the Search Tool for the Retrieval of Interacting Genes/Proteins (STRING) database (https://string-db.org/) were downloaded with an interaction threshold of 0.4 and visualized using Cytoscape software (https://cytoscape.org/) (Figure 4A). The genes encoding these 20 proteins subjected to Gene Ontology (GO) and Kyoto Encyclopedia of Genes and Genomes (KEGG) enrichment analyses. GO analysis included biological process (BP), cellular composition (CC), and molecular function (MF). The Wnt signaling pathway and liver development were enriched in BP. In the KEGG analysis, multiple signal pathways were enriched, including the cyclic adenosine monophosphate (cAMP), Ras, Rap1, cyclic guanosine monophosphate (cGMP)-PKG, and gonadotropin-releasing hormone (GnRH) signaling pathways (Figure 4B). The top 5 hub genes ranked by maximal clique centrality with cytoHubba were IQCB1, RPGR, CEP290, NPHP4, and RPGRIP1L (Figure 4C). The pathways activity of hub genes are illustrated in the Figure 4D. IQCB1 was found to activate pathways including apoptosis, cell cycle, DNA damage response, hormone androgen receptor (AR), hormone estrogen receptor (ER), and PI3K/AKT and to inhibit apoptosis, cell cycle, epithelial-mesenchymal transition, hormone AR, hormone ER, RAS/MAPK, RTK, and TSC/mTOR.
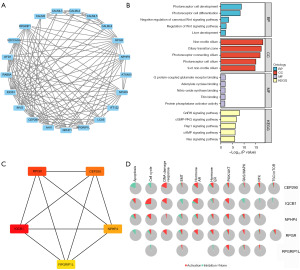
Hub gene construction of a prognosis model
A prognostic model was developed based on a set of 20 genes. Through the application of least absolute shrinkage and selection operator (LASSO) regression analysis, ten genes associated with IQCB1 were assessed and incorporated into the predictive model. This included three genes deemed protective (CALML4, CALML6, RAB8A) and seven genes considered detrimental (RPGRIP1L, RPGRIP1, NPHP3, IQCB1, CALML5, CALML3, ATXN10) as illustrated in Figure 5A,5B. Then, based on this prognostic model, the risk scores of each patient with LIHC in TCGA were calculated: risk score = 0.1438*ATXN10 + 0.4467*CALML3 + (−0.0771)*CALML4 + 0.2152*CALML5 + (−0.0271)*CALML6 + 0.2255*IQCB1 + 0.0365*NPHP3 + (−0.2092)*RAB8A + 0.0047*RPGRIP1 + 0.4076*RPGRIP1L.
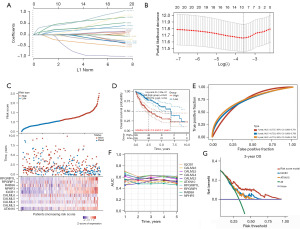
The patients were classified into a high-risk group and a low-risk group based on the median risk score. Figure 5C displays the relationship between risk score and survival time, survival status. In the Kaplan-Meier (KM) survival curve, the model showed a significant difference between the high-risk group [hazard ratio (HR) =2.643] and the low-risk group. The high-risk group had a shorter OS compared to the low-risk group. The median survival time for the high-risk group was 2.5 years, whereas the median survival time for the low-risk group was 6.7 years (Figure 5D). The prognostic AUC values at 1-year, 3-year, and 5-year for this model were 0.737, 0.723, and 0.719, respectively (Figure 5E). We also analyzed the AUC values of these ten genes for the TCGA-LIHC cohort in 5 years. The AUC value of IQCB1 was the largest among these genes, indicating that IQCB1 had the best prognostic ability for OS in patients with LIHC (Figure 5F). We used decision curve analysis R package-ggDCA to construct three models. Figure 5G shows that the risk model outperformed the IQCB1 and ATXN10 genes in predicting OS prognosis over a 3-year period, with IQCB1 being superior to ATXN10.
The correlation between IQCB1 gene and immune infiltration
To investigate the intricate relationship between IQCB1 and the diverse immune cell subsets present within the TME of HCC, a comprehensive assessment of 24 distinct immune cell types was conducted (Figure 6A), including activated dendritic cells (aDC), B cells, CD8 T cells, cytotoxic cells, DC, eosinophils, immature DC (iDC), macrophages, mast cells, neutrophils, natural killer (NK) CD56bright cells, NK CD56dim cells, NK cells, plasmacytoid DC (pDC), T cells, T helper cells (Th), T central memory (Tcm), T effector memory (Tem), T follicular helper (TFH), T gamma delta (Tgd), Th1 cells, Th17 cells, Th2 cells, and regulatory T cells (Treg). IQCB1 was negatively correlated with Th17 cells (R=−0.375) and DC (R=−0.321), whereas it was positively correlated with Th2 cells (R=0.426) (Figure 6B-6D). Th17 cells and DC were significantly enriched in the low IQCB1 expression group (≤ median), whereas Th2 cells were enriched high-expression group (Figure 6E-6G). In addition, we analyzed the relationship between IQCB1 and immunological checkpoints. IQCB1 was found to be positively correlated with PDCD1, CD274, CTLA4, LAG3, TIGIT, IDO1, CD80, CD86, LAIR1, PVR, CD200R1, CD276, HAVCR2, VTCN1, BTLA, and KIR3DL1 (Figure 6H). The association between IQCB1 and CD276 exhibited the highest degree of correlation among the immunological checkpoints examined (R=0.536) (Figure 6I). As shown in Figure 6J,6K, the correlation coefficients between IQCB1 and PDCD1 and CTLA4 were 0.309 and 0.331, respectively. In the high expression of IQCB1 group, there was a significant increase in the expression levels of all three immune checkpoints, namely CD276, PDCD1, and CTLA4 (Figure 6L-6N). These results indicated that IQCB1 may be involved in immune responses, and achieve immune evasion through immune checkpoints, thereby promoting the occurrence and development of liver cancer.
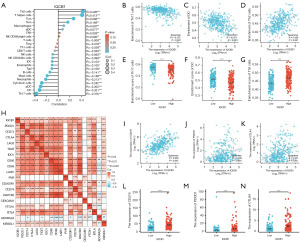
Drug sensitivity analysis of IQCB1 gene in LIHC
We predicted the drug treatment responses for each sample from the TCGA-LIHC according to the GDSC database (Figure 7). The results showed that the IC50 value of several drugs were negatively correlated with the expression level of IQCB1, including BI-2536, NPK76-II-72-1, PIK-93, 5-fluorouracil, methotrexate, AT-7519, PHA-793887, YK4-279, THZ-2-102-1, and FTI-277. Trametinib and erlotinib were positively correlated with the expression of IQCB1. Next, the drug with the highest degree of correlation was selected for further analysis. These findings indicate that patients with high IQCB1 expression may not respond favorably to trametinib and erlotinib, whereas BI-2536 and NPK76-II-72-1 could potentially offer superior therapeutic outcomes. This drug screening approach offers novel insights and strategies for clinical treatment planning.
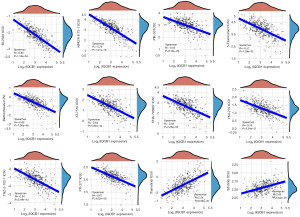
The expression of IQCB1 gene in the TME in single-cell datasets
We used single-cell datasets from the TISCH database, including three datasets, LIHC_GSE140228_10X, LIHC_GSE140228_Smartseq2, and LIHC_GSE98638 (17,18), to analyze the expression of IQCB1 in TME. These three datasets included 12, 10, and 6 distinct cell types, respectively (Figure 8A-8C). As depicted in Figure 8D-8G, heatmaps and violin plots illustrating IQCB1 expression in various cell types across the three datasets were also provided. The analysis of gene expression data from the GSE140228_10X dataset revealed that IQCB1 exhibited high expression levels in Treg, proliferating T cells (Tprolif), and exhausted CD8 T cells (CD8Tex) cell types. Similarly, in the GSE140228_Smartseq2 dataset, IQCB1 was found to be more highly expressed in B cells and CD8Tex, whereas in the GSE98638 dataset, elevated expression of IQCB1 was observed in Tprolif and CD8Tex cell types. IQCB1 enriched in various immune cells, indicating that IQCB1 was related to the TME in liver cancer.
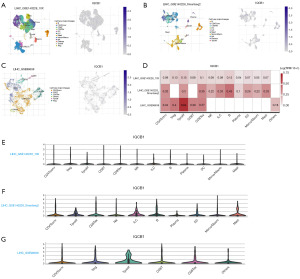
Discussion
The early diagnosis of LIHC is crucial for the treatment and prognosis. The mechanism and function of IQCB1 in liver cancer is completely unclear. In this study, we first used the TCGA database to show that IQCB1 was highly expressed in HCC, and the AUC value of the ROC curve analysis was 0.885. Through prognostic analysis, it was shown that IQCB1 affected the prognosis of patients with liver cancer, and the higher the expression of IQCB1, the worse the prognosis. In time-dependent ROC analysis, the AUC value of IQCB1 in 5-year OS was above 0.6, and multivariate Cox analysis showed that IQCB1 was an independent prognostic factor. Based on the analysis of pathological stage, age, and IQCB1 expression in patients with liver cancer by T, N, and M, a nomogram was developed to offer an alternative approach for predicting the survival outcomes of patients with liver cancer in a clinical setting. The survival time of patients with liver cancer is affected by many factors, and the prognostic value of IQCB1 remains to be further explored. IQCB1 is expected to be a potential biomarker for determining the prognosis, suggesting that patients with LIHC may benefit from using IQCB1 for diagnosis and prognosis.
The 20 proteins related to IQCB1 were downloaded from the STRING database and constructed a PPI network diagram. Through the GO and KEGG pathway enrichment analyses, it was found that these genes were related to the development of the liver and negative regulation of the canonical Wnt signaling pathway and are enriched in multiple signal pathways in the KEGG signaling pathway. Through the GDSC database, the relationship between IQCB1 and the activity of ten tumor-related pathways were analyzed. These signaling pathways were also found to be well enriched, which further increased the reliability of our research. Through LASSO Cox regression, we established a risk model for OS and analyzed TCGA-LIHC patients, with an AUC value of 0.7 or above for 5 years. IQCB1, as a standalone prognostic indicator, had an AUC value of 0.7 within 1 year, and IQCB1 was the best prognostic indicator out of the 9 molecules, indicating that IQCB1 has good prognostic performance.
In the immune analysis, IQCB1 was positively correlated with the infiltration of Th2 cells and negatively correlated with Th17 and DC cells. Th2 cells are a type of helper T cell that play a crucial role in the immune system. They are characterized by producing the cytokines interleukin-4 (IL-4) and interleukin-10 (IL-10), which promote the development of immune responses against parasites and helminths. In the context of tumors, Th2 cells have been associated with the development of certain types of cancer (19,20), in which they contribute to immune evasion and tumor growth. This is due to the pro-tumorigenic effects of Th2 cytokines, such as IL-4 and IL-10, which can inhibit anti-tumor immune responses. The relationship between Th2 cells and tumors is complex and context-dependent. Further research is needed to fully understand the mechanisms underlying Th2 cell involvement in cancer, especially in the development and progression of liver cancer. Immunological checkpoints are expressed on immune cells, which inhibit the function of immune cells and prevent the body from generating effective anti-tumor immune responses, allowing tumors to form an immune escape (21). B7-H3 (B7 homolog 3 protein), also known as CD276, is an type I transmembrane glycoprotein composed of 316 amino acids. It has a similar structure to B7-H1 (PD-L1) and is an important immune checkpoint molecule in the B7-CD28 family. CD276 is an immune checkpoint that is enriched in tumor stem cells. In recent years, immunotherapy targeting CD276 has become a research hotspot in the field of oncology (22-24). IQCB1 is positively correlated with immunological checkpoint molecules CD276, CD274 (PD-L1), and CTLA4. High expression of PD-L1 and CD276 are significantly associated with high grade in HCC patients (25). In colon cancer, the PD1/CTLA4 immune checkpoint upregulates IQCB1 and promotes the infiltration of Th17 immune cells, which affects the occurrence and development of colon cancer (26). However, in our research, there was a negative correlation between IQCB1 and the immune infiltration of Th17 cells, indicating that the role of Th17 cells may vary depending on the type of cancer. Th17 cells, as inflammatory cells, are influenced by the TME, which represents shared molecular, cytokines, and signaling pathways with inflammation. Moreover, due to their lack of specificity in identification, Th17 cell function is largely dependent on the influence of the TME (27-29). In pancreatic cancer, a Th17 cell phenotype has been found to exist. RIP1-mediated macrophage reprogramming leads to a remodeling of the CD4+ T cell phenotype, resulting in high coexpression of IFN-γ, IL-17, T-bet, and RORgt. These cells lose their pro-tumorigenic ability associated with IL-17+ Th17 cells and acquire anti-tumor capability through producing IFN-γ and acquiring a memory phenotype similar to that of Th1 cells (29). CD8Tex have a close relationship with liver cancer. In cases of chronic infections and cancers such as liver cancer, continuous antigenic stimulation can lead to the exhaustion of T cells, resulting in the loss of their original effector functions (30). This exhausted state limits the immune response of T cells against tumors, potentially promoting the progression or deterioration of liver cancer (31). Single-cell datasets showed that IQCB1 was related to the CD8Tex. IQCB1 may play a role in the pathogenesis of liver cancer through its involvement in the depletion of CD8T cells.
These results suggested that IQCB1 may be involved in the immune response, regulating immunological checkpoints to achieve immune evasion and promoting the occurrence and development of HCC. The relationship between IQCB1 and immune checkpoints is a complex and yet to be further studied field. Immune checkpoints are a series of regulatory mechanisms within the immune system that aim to balance immune responses and prevent excessive attacks on self-tissue. These checkpoints typically involve a range of protein interactions, thereby regulating the activation and function of immune cells. Given the paucity of extant research on IQCB1 in the context of liver cancer, the present study undertook an analysis of the potential relationship between IQCB1 and immune cells as well as immune checkpoints. This investigation suggests that such a correlation may facilitate immune evasion, thereby contributing to the pathogenesis and progression of liver cancer.
Finally, we conducted drug sensitivity analysis. BI-2536 is a novel, potent, and highly selective inhibitor of PLK1, which also inhibits PLK2 and PLK3 (32). BI-2536 induces cell mitotic arrest and leads to cancer cell apoptosis (33). In this study, a significant positive correlation was found between IQCB1 and BI-2536. Utilizing drug sensitivity data from the GDSC database, we hypothesized a potential correlation between IQCB1 and various drugs, suggesting the necessity for further investigation into the specific efficacy and mechanisms of action. This provided a new treatment plan for the clinical management of patients with LIHC.
In summary, our study demonstrated the diagnostic, prognostic, and immune value of IQCB1 in HCC, although there were still some deficiencies. These analyses were based on the TCGA database and lack experimental validation. First of all, additional clinical data is required to confirm the diagnostic and prognostic effectiveness of IQCB1 in liver cancer. Furthermore, further investigation into the regulatory mechanisms of IQCB1 is necessary to understand its impact on liver cancer progression and immune evasion. For instance, the relationship between IQCB1 and CD276 warrants exploration to elucidate how IQCB1 influences immune evasion. The potential correlation between CD276 inhibitors and IQCB1 in terms of drug sensitivity remains uncertain, and the potential benefits of CD276 inhibitors for patients expressing IQCB1 require verification.
Conclusions
This study has shown the diagnostic, prognostic, and immune significance of IQCB1 in HCC. IQCB1 serves as a potential molecular marker in liver cancer, suggesting its potential as a promising therapeutic target.
Acknowledgments
Funding: This project has been supported by
Footnote
Reporting Checklist: The authors have completed the TRIPOD reporting checklist. Available at https://tcr.amegroups.com/article/view/10.21037/tcr-24-110/rc
Peer Review File: Available at https://tcr.amegroups.com/article/view/10.21037/tcr-24-110/prf
Conflicts of Interest: All authors have completed the ICMJE uniform disclosure form (available at https://tcr.amegroups.com/article/view/10.21037/tcr-24-110/coif). All authors report funding from National Natural Science Foundation of China (grant No. 81602020) and Tianjin Medical University Cancer Institute & Hospital Research Project (grant No. 1805). The authors have no other conflicts of interest to declare.
Ethical Statement: The authors are accountable for all aspects of the work in ensuring that questions related to the accuracy or integrity of any part of the work are appropriately investigated and resolved. The study was conducted in accordance with the Declaration of Helsinki (as revised in 2013).
Open Access Statement: This is an Open Access article distributed in accordance with the Creative Commons Attribution-NonCommercial-NoDerivs 4.0 International License (CC BY-NC-ND 4.0), which permits the non-commercial replication and distribution of the article with the strict proviso that no changes or edits are made and the original work is properly cited (including links to both the formal publication through the relevant DOI and the license). See: https://creativecommons.org/licenses/by-nc-nd/4.0/.
References
- Zou R, Hao Y, Wang Y, et al. A multicenter retrospective analysis: Factors influencing hepatic adverse events induced by immunotherapy in advanced liver cancer. Cancer Rep (Hoboken) 2024;7:e1918. [Crossref] [PubMed]
- Cai Q, Wu W, Li R, et al. Clinical characteristics and outcomes of patients with primary liver cancer and immune checkpoint inhibitor-associated adrenal insufficiency: A retrospective cohort study. Int Immunopharmacol 2024;127:111337. [Crossref] [PubMed]
- Wang L, Wu Y, Yang N, et al. Self-assembly of maltose-albumin nanoparticles for efficient targeting delivery and therapy in liver cancer. Int J Biol Macromol 2024;258:128691. [Crossref] [PubMed]
- Huang A, Guo DZ, Zhang X, et al. Serial circulating tumor DNA profiling predicts tumor recurrence after liver transplantation for liver cancer. Hepatol Int 2024;18:254-64. [Crossref] [PubMed]
- Lerut J, Julliard O, Ciccarelli O, et al. Hepatocellular cancer and liver transplantation: a Western experience. Recent Results Cancer Res 2013;190:127-44. [Crossref] [PubMed]
- Rishi E, Goel S, Rishi P. Senior-Loken syndrome secondary to IQCB1 mutation in association with retinitis pigmentosa. Can J Ophthalmol 2021;56:e112-4. [Crossref] [PubMed]
- Kruczek K, Qu Z, Welby E, et al. In vitro modeling and rescue of ciliopathy associated with IQCB1/NPHP5 mutations using patient-derived cells. Stem Cell Reports 2022;17:2172-86. [Crossref] [PubMed]
- Horani A, Ferkol TW. Understanding Primary Ciliary Dyskinesia and Other Ciliopathies. J Pediatr 2021;230:15-22.e1. [Crossref] [PubMed]
- Chen X, Shi Z, Yang F, et al. Deciphering cilia and ciliopathies using proteomic approaches. FEBS J 2023;290:2590-603. [Crossref] [PubMed]
- Wang S, Li X, Liu C, et al. Single-cell transcriptomic analysis of the role of HPV16-positive macrophages in cervical cancer prognosis. J Med Virol 2023;95:e28410. [Crossref] [PubMed]
- Chang Z, Miao X, Zhao W. Identification of Prognostic Dosage-Sensitive Genes in Colorectal Cancer Based on Multi-Omics. Front Genet 2020;10:1310. [Crossref] [PubMed]
- de Miguel FJ, Sharma RD, Pajares MJ, et al. Identification of alternative splicing events regulated by the oncogenic factor SRSF1 in lung cancer. Cancer Res 2014;74:1105-15. [Crossref] [PubMed]
- Liu J, Lichtenberg T, Hoadley KA, et al. An Integrated TCGA Pan-Cancer Clinical Data Resource to Drive High-Quality Survival Outcome Analytics. Cell 2018;173:400-416.e11. [Crossref] [PubMed]
- He J, Chen Z, Xue Q, et al. Identification of molecular subtypes and a novel prognostic model of diffuse large B-cell lymphoma based on a metabolism-associated gene signature. J Transl Med 2022;20:186. [Crossref] [PubMed]
- Hänzelmann S, Castelo R, Guinney J. GSVA: gene set variation analysis for microarray and RNA-seq data. BMC Bioinformatics 2013;14:7. [Crossref] [PubMed]
- Yang K, Ma Y, Chen W, et al. CCDC58 is a potential biomarker for diagnosis, prognosis, immunity, and genomic heterogeneity in pan-cancer. Sci Rep 2024;14:8575. [Crossref] [PubMed]
- Zheng C, Zheng L, Yoo JK, et al. Landscape of Infiltrating T Cells in Liver Cancer Revealed by Single-Cell Sequencing. Cell 2017;169:1342-1356.e16. [Crossref] [PubMed]
- Zhang Q, He Y, Luo N, et al. Landscape and Dynamics of Single Immune Cells in Hepatocellular Carcinoma. Cell 2019;179:829-845.e20. [Crossref] [PubMed]
- Chen Y, Sun J, Luo Y, et al. Pharmaceutical targeting Th2-mediated immunity enhances immunotherapy response in breast cancer. J Transl Med 2022;20:615. [Crossref] [PubMed]
- Zhang Q, Qin J, Zhong L, et al. CCL5-Mediated Th2 Immune Polarization Promotes Metastasis in Luminal Breast Cancer. Cancer Res 2015;75:4312-21. [Crossref] [PubMed]
- Dincer HA, Horzum U, Kursunel MA, et al. Liver resection modulates hepatic chemokine levels in breast cancer. Surgery 2023;174:277-82. [Crossref] [PubMed]
- Feng Y, Lee J, Yang L, et al. Engineering CD276/B7-H3-targeted antibody-drug conjugates with enhanced cancer-eradicating capability. Cell Rep 2023;42:113503. [Crossref] [PubMed]
- Wang C, Li Y, Jia L, et al. CD276 expression enables squamous cell carcinoma stem cells to evade immune surveillance. Cell Stem Cell 2021;28:1597-1613.e7. [Crossref] [PubMed]
- Hińcza-Nowak K, Kowalik A, Walczyk A, et al. CD276 as a Candidate Target for Immunotherapy in Medullary Thyroid Cancer. Int J Mol Sci 2023;24:10019. [Crossref] [PubMed]
- Chen L, Huang X, Zhang W, et al. Correlation of PD-L1 and SOCS3 Co-expression with the Prognosis of Hepatocellular Carcinoma Patients. J Cancer 2020;11:5440-8. [Crossref] [PubMed]
- Ben S, Zhu Q, Chen S, et al. Genetic variations in the CTLA-4 immune checkpoint pathway are associated with colon cancer risk, prognosis, and immune infiltration via regulation of IQCB1 expression. Arch Toxicol 2021;95:2053-63. [Crossref] [PubMed]
- Xing C, Wang M, Ajibade AA, et al. Microbiota regulate innate immune signaling and protective immunity against cancer. Cell Host Microbe 2021;29:959-974.e7. [Crossref] [PubMed]
- Xing J, Man C, Liu Y, et al. Factors impacting the benefits and pathogenicity of Th17 cells in the tumor microenvironment. Front Immunol 2023;14:1224269. [Crossref] [PubMed]
- Cerboni S, Gehrmann U, Preite S, et al. Cytokine-regulated Th17 plasticity in human health and diseases. Immunology 2021;163:3-18. [Crossref] [PubMed]
- Liu K, Liu J, Zhang X, et al. Identification of a Novel CD8(+) T cell exhaustion-related gene signature for predicting survival in hepatocellular carcinoma. BMC Cancer 2023;23:1185. [Crossref] [PubMed]
- Li S, Li K, Wang K, et al. Low-dose radiotherapy combined with dual PD-L1 and VEGFA blockade elicits antitumor response in hepatocellular carcinoma mediated by activated intratumoral CD8(+) exhausted-like T cells. Nat Commun 2023;14:7709. [Crossref] [PubMed]
- Jemaà M, Mokdad Gargouri R, Lang F. Polo-like kinase inhibitor BI2536 induces eryptosis. Wien Med Wochenschr 2023;173:152-7. [Crossref] [PubMed]
- Lin RC, Chao YY, Lien WC, et al. Polo‑like kinase 1 selective inhibitor BI2536 (dihydropteridinone) disrupts centrosome homeostasis via ATM‑ERK cascade in adrenocortical carcinoma. Oncol Rep 2023;50:167. [Crossref] [PubMed]