Development of an immunogenic cell death-related lncRNAs signature for prognostic risk assessment in gastric cancer
Highlight box
Key findings
• Our research shows that the immunogenic cell death-related long non-coding RNAs (lncRNAs) signature (ICDlncSig), associated with immune cell infiltration and immune therapy response, is closely linked to clinical outcomes in gastric cancer (GC) patients and suggests potential for targeted molecular therapy.
What is known and what is new?
• GC is notably influenced by genetic mutations, epigenetic changes, and signaling pathways; Helicobacter pylori is a primary cause. Treatments include surgery, chemotherapy, and newer immunotherapies like checkpoint inhibitors.
• The role of lncRNAs in GC pathology is emerging as a crucial aspect, with studies highlighting their influence on cell behavior and treatment resistance. The development of an lncRNA-based prognostic model is a novel approach for assessing patient outcomes and guiding treatment choices.
What is the implication, and what should change now?
• Increase the use and development of lncRNA-based prognostic models to refine patient treatment plans and enhance outcomes. Further research into the mechanisms and therapeutic potentials of lncRNAs in GC could provide new avenues for targeted therapies. Integration of immunotherapy and immunogenic cell death-inducing treatments should be explored more deeply in clinical settings to optimize their efficacy and expand their use in standard care protocols for GC.
Introduction
Gastric cancer (GC) is the fifth most common cancer worldwide and the third leading cause of cancer-related death (1,2). Helicobacter pylori is the leading cause of GC (3,4). The growth, spread, and metastasis of GC are influenced by genetic mutations, epigenetic alterations, and abnormal molecular signaling pathways (5). The prognosis for GC remains poor even though significant advances have been made in its treatment, and the 5-year survival rate is less than 30% (6-8). In addition, the onset of GC is relatively insidious, and it is often at an advanced stage when undergoing diagnosis, leading to a high mortality rate (9). Current studies are examining immunotherapeutic approaches, and they are approved as monotherapies as new treatment options.
Immune checkpoint therapy has been shown to be clinically more effective than conventional therapeutic modalities, with the most classical programmed death-ligand 1 (PD-L1) able to bind to programmed immune cells death 1, leading to T-cell suppression and apoptosis (10-12). It is believed that gallectin-9 and its receptor T-cell immunoglobulin and mucin-containing domain 3 (Tim3) act as co-suppressive receptors for immune cells. Tim3 is overexpressed on failing T cells in chronic viral infection or cancer, and in addition (13-15), Tim3 presents on dendritic cells (DCs) can be hyperfunctional (16-18). The two aforementioned receptors play a crucial role in the immune system’s resistance to tumors, including immunogenic cell death (ICD). This endoplasmic reticulum (ER) stress-induced apoptotic approach can activate the corresponding immune system by secreting or stimulating the release of various danger-associated molecular patterns (DAMPs). All cytotoxic therapies can lead to apoptosis, but three anthracyclines and oxaliplatin can induce ICD. The secretion of Association of Tennis Professionals and the release of the cell death-associated protein high mobility group box 1 are also considered to be two additional markers of ICD (19-21). Cytotoxic agents are able to be accurately predicted by the detection of these features to induce ICD in cancer cells. Among them are cytostatic agents, including oxaliplatin and anthracyclines, as well as treatments that cause ER stress, like radiotherapy. When DCs are stimulated, a cellular cascade is generated, at which point the ICD triggers an antigenic immune response against the dead cells. In addition, therapeutically driven ICD has been shown to produce an immune response against cancer. However, only a few ICD induction treatments are currently being utilized, so more in-depth exploration is needed to improve clinical care. It is also unclear how ICD induction can be used in clinical treatment protocols for GC. In two clinical case reports, ICD has been described after radiation therapy, and in one, sandalwood alcohol was described as an ICD-inducing agent (22-24). Additionally, ICD caused by treatment is a rare occurrence.
It has been shown that long non-coding RNAs (lncRNAs), which has a transcriptional length exceeding 200 nucleotides, plays an important role in growth control. The highly expressed PCED1B-AS1 in GC cell lines and tissues upregulates the expression of MAP2K7 by acting as a sponge for miR-3681-3p, thereby accelerating cell proliferation and inhibiting apoptosis, potentially serving as a therapeutic target for GC (25). Additionally, the methylation of PSMA3-AS1 and MIR22HG suppresses apoptosis in GC cells and promotes tumorigenesis through the miR-411-3p or MIR22HG-miR-24-3p-SERTAD1 axis (26). Similarly, upregulated SNHG26 interacts with nucleolin, regulating c-Myc expression, ultimately promoting the development of malignant tumors in GC by promoting energy metabolism through hexokinase 2 (27). Furthermore, research has found that hnRNPA2B1, as a methyladenosine reader, is upregulated in GC cells and tissues, interacting with lncRNA NEAT1 to enhance its stability, ultimately maintaining cell stemness and exacerbating chemoresistance in GC through the Wnt/β-catenin pathway (28). The study of lncRNAs prognosis models has also emerged alongside functional and mechanistic studies. Li et al. constructed a prognostic model based on 11 long noncoding RNAs involved in exosomes, which was found to be significantly associated with certain components of GC’s immune microenvironment and could be used in this context (29). The model the authors developed for prognosis of GC patients is based on four pyroptosis-related lncRNAs, has been validated with various samples, and has proved effective in guiding the immune microenvironment, demonstrating the feasibility of molecularly targeted therapies for prognostic indications (30). According to Luo et al., 12 necrosis-related long noncoding RNAs are used to determine the prognosis of GC cases (31). Although GC-related lncRNAs and their biological function have not yet been well studied, there is a lack of evidence regarding their clinical significance.
According to Figure 1, an lncRNAs signature significantly correlated to the ICD has been developed and validated in this study, and its clinical implications and associations with clinical characteristics of patients with GC have been evaluated. Additionally, we successfully established a nomogram, offering a more accurate prediction of GC patients’ prognoses. In addition, we also investigated the correlation between ICD-related lncRNAs signatures (ICDlncSig) and immune cell infiltration, as well as the response to immunotherapy, and found through in vitro experiments that proliferation, migration, and invasion of GC cells have been associated with AP002954.1 and AP000695.1. It may be possible to enhance prognosis assessment and treatment for GC patients through a better understanding of the pathophysiology of immunogenic death. We present this article in accordance with the TRIPOD reporting checklist (available at https://tcr.amegroups.com/article/view/10.21037/tcr-24-344/rc).
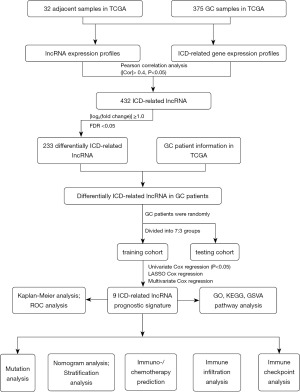
Methods
Data acquisition
On May 1, 2022, the relevant RNA sequencing data, and corresponding clinical characteristics of GC patient samples (including 375 GC tissues and 32 adjacent tissues) were downloaded from The Cancer Genome Atlas (TCGA) (https://portal.gdc.cancer.gov/). By using the “limma” package in R, differentially expressed genes were identified utilizing transcript data from the TCGA_STAD dataset [conditions: false discovery rate (FDR) =0.05 and log2(fold change) =1.0]. The study was conducted in accordance with the Declaration of Helsinki (as revised in 2013).
Recognizing ICD-related lncRNAs in GC
Thirty-two genes associated with immunogenic death were obtained from recent studies and these genes are shown in Table S1 (32). Based on Pearson correlation analysis, 432 lncRNAs associated with ICD were identified from the expression levels of ICD-related genes (based on |Pearson correlation coefficient| >0.4 and P<0.05). Thereafter, 233 differentially expressed lncRNAs associated with ICD were screened according to FDR <0.05 and |log2(fold change)| ≥1.0.
Development of a prognostic signature for GC based on ICD-related lncRNAs
By combining the expression matrix with clinical data, 337 GC patients with complete survival data were obtained, from which the missing clinical data were censored. There were 236 patients in the training cohort, and 101 patients in the testing cohort. Seventy percent of the sample went through training, and thirty percent went through testing. Identifiers of lncRNAs associated with survival were identified using univariate Cox regression analysis (P<0.05). Subsequently, ICDlncSig for GC were constructed by least absolute shrinkage and selection operator (LASSO) regression models and multivariate Cox regression analysis. Below are the risk scores.
Therein, β denotes the coefficient value, and exp the level of lncRNAs expression.
Patients were divided into high-risk and low-risk groups based on the median risk score in the training cohort. Subsequently, using the same approach, patient risk scores for the other cohorts were calculated, and based on the median risk score of the training cohort, two subgroups were formed. Then patients in high- and low-risk cohorts were analyzed using Kaplan-Meier survival analysis. P<0.05 indicates statistical significance.
To finish the evaluation of the specificity and predictive accuracy of risk models based on clinicopathological characteristics, the “timeROC” software package was used to construct time-dependent receiver operating characteristic (ROC) curves.
Gene function enrichment analysis using Gene Ontology (GO) and Kyoto Encyclopedia of Genes and Genomes (KEGG)
Cellular components (CCs), molecular functions (MFs), and biological processes (BPs) are all included in the GO database. Systematic analyses of gene function in biological pathways are conducted using the KEGG database. In order to analyze functional and pathway enrichment clustering of the differential genes within the prognostic model, the clusterProfiler package in R was used. P>0.05 for FDR was considered a significant difference.
Analysis of gene set variation (GSVA)
In certain gene sets, GSVA scores were obtained for each sample of the TCGA dataset using the GSVA package and the single-sample gene set enrichment analysis (ssGSEA) method (http://www.bioconductor.org) (33). A GSVA score indicates the degree to which a genome is enriched in each sample.
Nomogram construction and calibration
Based on the predictive risk model, the Nomogram was drawn using RMS and a total score was calculated based on the risk score and clinical characteristics. The nomogram visualizes GC patients’ estimated survival probabilities at 1-, 3-, and 5-year using the R package “survival”. As a next step, model diagnostics were performed using the package “survminer”, including the Schoenfeld residuals. To evaluate the prediction accuracy and clinical validity of the model, the area under the ROC was calculated, the calibration analysis was conducted, and the decision curve analysis (DCA) was conducted.
Tumor-infiltrating immune cell analysis
We assessed the level of immune infiltration among two ICDlncSig subgroups using several algorithms (including MCPCOUNTER, TIMER, CIBERSORT, QUANTISEQ, EPIC algorithms, CIBERSORT-ABS, and XCELL) (34). Based on ssGSEA (33), a comparison was made between different immune cells and pathways. The ESTIMATE algorithm (which includes EstimateScores, ImmuneScores, and StromalScores) was used to compare tumor cells with normal cells (35). To provide more possibilities for clinical immunotherapy, we analyzed the relationship between immune checkpoint genes and ICDlncSig.
Forecasting immunotherapeutic responses
The utilization of the tumor immune dysfunction and rejection (TIDE) algorithm enables the assessment of the response of patients with GC to immunotherapy, aiding clinicians in determining the optimal treatment approach. This algorithm, through the analysis of immune dysfunction and rejection within tumors, provides crucial support for personalized medicine (36). The pRRophetic software package (37) is used to calculate the half-maximal inhibitory concentration (IC50, µM) for each sample in order to predict drug sensitivity.
Quantitative real-time PCR analysis (qRT-PCR) of total RNA
Total RNA was isolated from GC cells using FastPure® Cell/Tissue Total RNA Isolation Kit V2 (Vazyme Biotech Co., Ltd., China), followed by reverse transcription using a reverse transcription kit (Thermo Fisher Scientific, MA, USA). After reversing transcribed RNA from GC cells into cDNA, the diluted cDNA was diluted 10-fold and used for further experiments. In this procedure, PCR was conducted with Q5 (Thermo Fisher Scientific, MA, USA), with denaturation at 95 ℃ for 10 seconds, annealing at 60 ℃ for 30 seconds, and extension at 72 ℃ for 30 seconds. Internal molecular references were all GAPDH rRNAs. Primer sequences are shown in Table S2.
Cell culture and transfection
Gastric epithelial cells (GES-1) along with GC cell lines (AGS, SGC-7901, HGC-27, MKN-45, and MKN-1) were obtained from the Chinese Academy of Sciences located in Shanghai, China. These cells were maintained in Roswell Park Memorial Institute (RPMI) 1640 medium (acquired from Corning, NY, USA), which was supplemented with 10% fetal bovine serum (FBS, sourced from Gibco, Thermo Fisher Scientific) and 1% antibiotic-antimycotic solution (procured from New Cell & Molecular Biotech Co., Ltd., Suzhou, China). The cultures were incubated in a CO2 incubator that was set to maintain a humid environment with 5% CO2 at a temperature of 37 ℃. For the transfection of plasmids into the GC cells, Lipo 3000 reagent (sourced from Invitrogen, Carlsbad, CA, USA) was utilized following the manufacturer’s protocols.
Vitro functional assays
To evaluating cell proliferation, cells were seeded at densities of either 3×103 or 1×103 evenly across the wells of 96-well or 6-well plates respectively, following a 48-hour period post-transfection. To facilitate the creation of a growth curve, 5 µL of CCK8 solution (provided by Dojindo, Osaka, Japan) was dispensed into each well subsequent to cell adherence. The optical density (OD) was recorded at a wavelength of 450 nm daily over a span of 5 days. After a culture duration of 14 days, cell quantification was carried out utilizing crystal violet to fix and stain the cells situated within 6-well plates.
For the evaluation of cell migration and invasion capabilities, cells were allocated at densities of 5×104 for migration assays and 8×104 for invasion assays, positioning them within the respective chambers of a 24-well plate. The invasion chambers had been pre-coated with Matrigel to facilitate this process. A fixed and stained procedure was employed using crystal violet both 48 hours following cell seeding and again after an additional 48-hour period; subsequent to these steps, randomly chosen fields were captured for photographic documentation.
Statistical analysis
To determine whether the differences were significant, we used log-rank tests based on Kaplan-Meier survival rates. Univariate and multifactor analyses were conducted using the Cox proportional risk model with stepwise regression (LRForward). A nomogram was constructed and validated according to the Iasonos guidelines. As a way of measuring the accuracy of the prognostic model, we used Harrell’s consistency index (c-index) and a time-dependent ROC curve. Statistical significance was determined by P<0.05.
Results
ICDlncSig construction for GC
To identify lncRNAs associated with ICD in GC tissues, Pearson correlation analysis was performed (|Cor| >0.4, P<0.05) on the lncRNA and ICD-related mRNA expression profiles of 375 GC tissues and 32 adjacent tissues in the TCGA database and identified 432 ICD-related lncRNAs, 233 of which were considered to be differentially expressed (Figure 2A,2B). A training cohort (70%) and a testing cohort (30%) were randomly selected from GC samples to determine the clinical significance of ICDlncSig. A univariate Cox regression analysis identified 67 lncRNAs associated with survival (hazard ratio >1, P<0.05) in the training cohort (Table 1). Furthermore, a risk model with nine ICDs was obtained using LASSO Cox regression analysis and based on minimum systolic values. Nine of the lncRNAs were associated with patient survival except for AC116158.1 (Figure S1).
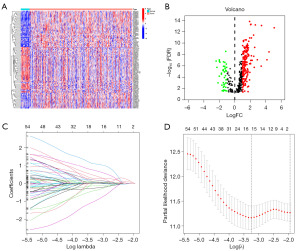
Table 1
LncRNA | HR | 95% CI | P value |
---|---|---|---|
ADAMTS9-AS2 | 2.514 | 1.313–4.813 | 0.005 |
LINC01140 | 2.699 | 1.153–6.321 | 0.02 |
AC090044.1 | 2.185 | 1.108–4.309 | 0.02 |
AC008808.2 | 1.443 | 1.072–1.942 | 0.02 |
AC006033.2 | 2.551 | 1.090–5.974 | 0.03 |
AC074131.1 | 2.265 | 1.097–4.676 | 0.03 |
SETBP1-DT | 1.789 | 1.075–2.977 | 0.03 |
FBXO30-DT | 2.81 | 1.071–7.373 | 0.04 |
FENDRR | 1.256 | 1.022–1.543 | 0.03 |
AC008759.3 | 2.3 | 1.246–4.244 | 0.008 |
RAP2C-AS1 | 2.156 | 1.249–3.723 | 0.006 |
GAS1RR | 5.089 | 2.069–12.520 | <0.001 |
AC093278.2 | 1.552 | 1.031–2.338 | 0.04 |
AC116158.1 | 4.631 | 1.668–12.854 | 0.003 |
AL035461.2 | 0.729 | 0.543–0.978 | 0.04 |
AC110995.1 | 2.541 | 1.284–5.026 | 0.007 |
AL357054.4 | 1.675 | 1.053–2.664 | 0.03 |
ZNF582-AS1 | 2.213 | 1.195–4.101 | 0.01 |
AC010478.1 | 1.738 | 1.146–2.637 | 0.009 |
MAGI2-AS3 | 1.862 | 1.313–2.640 | <0.001 |
SREBF2-AS1 | 0.483 | 0.281–0.830 | 0.008 |
AC092718.3 | 0.645 | 0.492–0.845 | 0.001 |
LINC02185 | 2.733 | 1.112–6.715 | 0.03 |
Z99289.1 | 1.824 | 1.050–3.168 | 0.03 |
SNHG15 | 0.612 | 0.424–0.884 | 0.009 |
AP003071.4 | 1.321 | 1.054–1.656 | 0.02 |
AF001548.1 | 1.22 | 1.013–1.469 | 0.04 |
SNHG14 | 1.873 | 1.170–2.996 | 0.009 |
LINC01094 | 2.015 | 1.238–3.278 | 0.005 |
BNC2-AS1 | 1.932 | 1.200–3.111 | 0.007 |
LINC02106 | 1.881 | 1.159–3.054 | 0.01 |
AL139147.1 | 3.887 | 1.431–10.562 | 0.008 |
AC048382.2 | 2.68 | 1.290–5.567 | 0.008 |
SENCR | 1.808 | 1.012–3.229 | 0.045 |
AC068790.7 | 1.849 | 1.077–3.174 | 0.03 |
AP001189.3 | 1.421 | 1.047–1.927 | 0.02 |
NR2F2-AS1 | 3.162 | 1.449–6.898 | 0.004 |
AC135012.3 | 3.065 | 1.156–8.130 | 0.02 |
MIR100HG | 1.36 | 1.121–1.650 | 0.002 |
LINC01537 | 4.928 | 1.552–15.649 | 0.007 |
LINC00106 | 0.721 | 0.538–0.967 | 0.03 |
LINC02773 | 2.453 | 1.186–5.076 | 0.02 |
LRRK2-DT | 3.752 | 1.061–13.272 | 0.04 |
AC025165.1 | 3.6 | 1.440–9.003 | 0.006 |
AP001528.1 | 1.835 | 1.206–2.791 | 0.005 |
PGM5P4-AS1 | 2.302 | 1.110–4.771 | 0.03 |
THBS4-AS1 | 1.751 | 1.140–2.687 | 0.01 |
CYP1B1-AS1 | 3.572 | 1.148–11.116 | 0.03 |
LINC01614 | 1.318 | 1.076–1.615 | 0.008 |
AP002954.1 | 1.473 | 1.023–2.122 | 0.04 |
CADM3-AS1 | 2.782 | 1.082–7.154 | 0.03 |
AC144548.1 | 0.362 | 0.136–0.965 | 0.04 |
LINC02613 | 2.513 | 1.075–5.874 | 0.03 |
AC037198.1 | 1.514 | 1.116–2.054 | 0.008 |
AC116914.1 | 0.478 | 0.232–0.987 | 0.046 |
RBMS3-AS3 | 2.232 | 1.036–4.807 | 0.04 |
AC012409.3 | 5.172 | 1.550–17.266 | 0.008 |
MIR99AHG | 1.814 | 1.182–2.785 | 0.006 |
LINC02829 | 2.472 | 1.131–5.403 | 0.02 |
AL049838.1 | 2.091 | 1.343–3.257 | 0.001 |
MSC-AS1 | 1.654 | 1.033–2.648 | 0.04 |
AC104825.1 | 1.505 | 1.037–2.184 | 0.03 |
LINC00092 | 1.93 | 1.033–3.605 | 0.04 |
AC006059.1 | 2.135 | 1.090–4.180 | 0.03 |
NR2F1-AS1 | 2.211 | 1.388–3.521 | 0.001 |
AP000695.1 | 1.67 | 1.110–2.512 | 0.01 |
lncRNA, long non-coding RNA; HR, hazard ratio; CI, confidence interval.
Prognostic models were constructed using nine lncRNAs associated with ICD and risk scores were obtained: Risk score =1.3530× expression (exp) of AC116158.1 + 1.1381 × exp of AC048382.2 + 0.9209 × exp of AC068790.7 - 0.5092 × exp of LINC00106 + 0.4768 × exp of AP002954.1 − 1.9875 × exp of AC144548.1 − 0.5983 × exp of AC116914.1 + 0.4029 × exp of AL049838.1 + 0.4345 × exp of AP000695.1. In the above equation, six genes (AC116158.1, AC048382.2, AC068790.7, AP002954.1, AL049838.1, AP000695.1) have positive coefficients and are risk genes; the other three genes (LINC00106, AC144548.1, AC116914.1) have negative coefficients and are protection genes (Figure 2C,2D).
Basic validation of ICDlncSig
High-risk and low-risk subgroups of GC patients were formed based on their median risk scores. Six upregulated ICD-lncRNAs and three downregulated ICD-RNAs were present in the high-risk group of GC patients, and their overall survival time was inversely proportional to the risk score (Figure 3A). In addition, Kaplan-Meier curve analysis revealed that low-risk group patients survived longer than high-risk group patients (Figure 3B). Subsequently, using ROC curves to analyze the area under the curve (AUC) values for the ICDlncSig in training cohorts, the 1-, 3-, and 5-year survival rates were 0.766, 0.732, and 0.813, respectively (Figure 3C). In both testing and entire cohorts, the same risk scoring formula is used, resulting in similar results. Similarly, the AUC values of the ICDlncSig in the testing cohort and the entire cohort were analyzed using ROC curves. The 1-, 3-, and 5-year survival rates in the testing cohort were 0.673, 0.604, and 0.504, respectively, and the 1-, 3-, and 5-year survival rates for the entire cohort were 0.741, 0.701, and 0.741, respectively (Figure 3D-3I). As indicated above, prognoses for GC can be predicted with good sensitivity and specificity using the risk model.
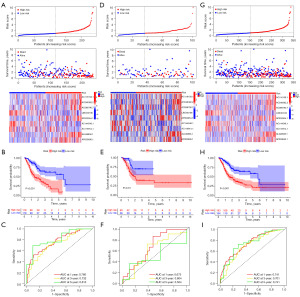
ICDlncSig and clinicopathologic characteristics in GC: correlation analysis
Using the entire cohort, we performed univariate and multivariate Cox regression analyses to determine if ICDlncSig is an independent predictor of GC. Based on univariate Cox regression analysis, the overall survival of GC patients was strongly influenced by the patient’s age, tumor stage, and ICDlncSig (Table 2). Using multivariate Cox regression analysis, ICDlncSig was identified as an independent prognostic indicator for GC patients (Table 2). Afterwards, we analyzed the correlation between ICDlncSig and clinicopathological characteristics, as shown in Figure 4A, with significant differences in age, histological grade, and tumor T-stage between patients in the high- and low-risk groups. In addition, the AUC values of ICDlncSig risk scores were found to be 0.741, 0.701, and 0.741 for 1-, 3-, and 5-year, respectively, as assessed by ROC curves (Figure 4B). Prognostic analysis of the entire cohort by clinical stratification based on different clinical-pathological correlation factors revealed that the prognosis of GC patients with high-risk label was worse in all clinical stratification subgroups except the M1 stage (Figure 4C). Based on the clinical trait composition ratios of each subgroup. Stage M1 patients with high-risk and low-risk labels showed no significant difference in prognosis. The above-mentioned reasons may be due to the low number of patients with stage M1 GC (Figure 4D). These findings indicate that ICDlncSig is independent of other clinicopathological factors in predicting prognosis in patients with GC.
Table 2
Clinicopathological characteristics | Univariable analysis | Multivariable analysis | |||||
---|---|---|---|---|---|---|---|
HR | 95% CI | P value | HR | 95% CI | P value | ||
Age | 1.021 | 1.003–1.039 | 0.02 | 1.033 | 1.014–1.052 | <0.001 | |
Gender | 1.268 | 0.872–1.845 | 0.21 | 1.349 | 0.918–1.982 | 0.13 | |
Grade | 1.336 | 0.947–1.885 | 0.10 | 1.236 | 0.871–1.755 | 0.24 | |
Stage | 1.479 | 1.193–1.833 | <0.001 | 1.559 | 1.240–1.958 | <0.001 | |
Risk score | 1.302 | 1.201–1.412 | <0.001 | 1.344 | 1.230–1.468 | <0.001 |
ICDlncSig, ICD-related lncRNAs signature; GC, gastric cancer; HR, hazard ratio; CI, confidence interval.
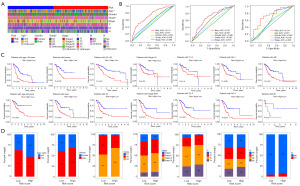
Pathways and functions associated with the ICDlncSig
To further explore the functions performed by ICDlncSig and the pathways involved, enrichment analyses were performed. GO enrichment analysis showed that results indicated that these genes were most significantly enriched in collagen-containing extracellular matrix, contractile fiber (CC processes), muscle system process, muscle contraction (BP processes), and actin binding, glycosaminoglycan binding (MF processes) (Figure 5A and available online: https://cdn.amegroups.cn/static/public/tcr-24-344-1.docx). Additionally, KEGG pathway enrichment demonstrated these genes were significantly enriched in Wnt, cGMP-PKG, and Hedgehog pathways (Figure 5B and Table S3). The above results demonstrated that the screened differential genes might be correlated to the promoting progress of GC. In addition, further GSVA pathway enrichment analysis of nine lncRNAs revealed that 26 pathways, including UV_RESPONSE_DN, TNFA_SIGNALING_VIA_NFK, TGF_BETA_SIGNALING, PANCREAS_BETA_CELLS, were positively correlated with risk score. UNFOLDED_PROTEIN_RESPONSE, SPERMATOGENESIS, OXIDATIVE_PHOSPHORYLATION, MYC_TARGETS_V2, and 10 other pathways were negatively correlated with risk score (Figure 5C). Activation of these markers may be involved in the process of tumor progression. This may lead to poor survival rates for GC patients.
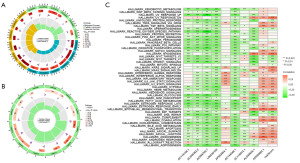
Prognostic nomogram construction and evaluation
This risk model was applied clinically by developing a nomogram that included several clinicopathological factors, including the ICDlncSig risk score, to predict overall survival for all patients with GC over 1, 3, and 5 years (Figure 6A). According to Schoenfeld’s residuals test, our Cox model meets proportional hazards (Figure 6B). In the ROC curve analysis, the model predicted the 1-, 3-, and 5-year survival of GC patients with AUC values of 0.726, 0.754, and 0.727, respectively (Figure 6C), and its c-index =0.7032, indicating that The nomogram has better discrimination ability. Subsequently, we similarly established calibration curves for nomogram and found that the survival predicted by nomogram for GC patients correlated closely with the actual survival outcomes (Figure 6D). Finally, DCA was used to evaluate the clinical effectiveness of the model. The DCA curve of the nomogram is shown in Figure 6E. The nomogram is generally associated with high clinical net benefit rates, according to our data. Based on these studies, the nomogram is more accurate than individual diagnostic features in predicting survival among GC patients.
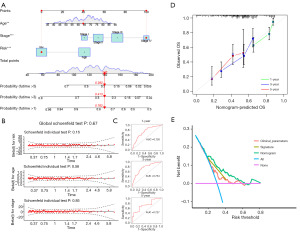
Infiltration of immune cells by ICDlncSig models
Several algorithms were used to compare immune infiltration between high-risk and low-risk groups. Figure 7A shows the bubbles of all apparently different immune response graphs. Comparative analysis of immune cell subsets showed that immune cells [including immature DCs (IDCs), Mast cells, activated DCs (aDCs), neutrophils, B cells, plasmacytoid DCs (pDCs), CD8+ T cells, DCs, macrophages, natural killer (NK) cells, T helper cells, Treg, Tfh, and tumor-infiltrating lymphocyte (TIL)] and immune function [chemokine receptor (CCR), check-point, antigen presenting cell (APC) co-inhibition, T cell co-stimulation, APC co-stimulation, human leukocyte antigen (HLA), parainflammation, inflammation-promoting, type II IFN response, T cell co-inhibition, type I IFN response] had significantly different infiltration levels (Figure 7B,7C). As a result of further analysis, all immune cells with differential immune infiltration and function scored higher in the high-risk subgroup. Both groups were also evaluated for their tumor microenvironment and characteristics such as EstimateScore, ImmuneScore, and StromalScore. Then the results showed patients who were at high risk for GC had higher immune, stromal, and estimate scores (Figure 7D). Based on these results, ICDlncSig may be associated to some extent with tumor-infiltrating immune cells in GC.
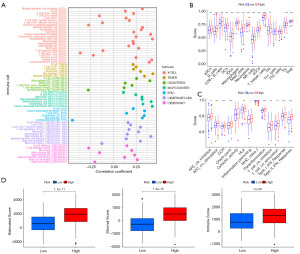
The therapeutic effect of the ICDlncSig model
It was found that immune checkpoints play a crucial role in immunotherapy. Therefore, according to our analysis of expression profiles in both groups, high-risk individuals expressed highly expressed immune checkpoint genes (TNFSF14, CD28, CD27, CD276, IDO2, TNFSF18, CD40LG, BTLA, LAIR1, TNFRSF4, NRP1, CD86, TIGIT, TNFRSF8, TNFSF4, CD200, CD48, PDCD1LG2, CD200R1, HAVCR2), except for TNFRSF25 (Figure 8A). Following that, a relationship between ICDlncSig and immune checkpoint genes was examined. A positive correlation exists between the ICDlncSig risk score and 14 immune checkpoint genes (TNFSF4, TNFSF14, PDCD1LG2, NRP1, LAIR1, HAVCR2, CD86, CD48, CD40LG, CD28, CD27, CD200R1, CD200, BTLA) (P<0.001, Figure 8B). Additionally, the TIDE algorithm was used to evaluate the ability of ICDlncSig to predict the benefit of immunotherapy. TIDE scores differed significantly between patients with different risk labels, indicating that low-risk patients would benefit more from immunotherapy than high-risk patients (Figure 8C). As a result, we assessed the sensitivity of different groups of patients to six commonly used chemotherapy drugs (dasatinib, clobazam, methotrexate, bortezomib, sunitinib, and imatinib), and by comparing the IC50 values of the drugs, we found that patients with high-risk GC had lower IC50 (µM) for dasatinib (P=2×10−9), clobazam (P=1.8×10−4), bortezomib (P=1.8×10−4), sunitinib (P=6.9×10−9) and imatinib (P=7.6×10−6) had lower IC50 values (Figure 8D). There may be a greater sensitivity to these five drugs in high-risk patients. For methotrexate (P=3×10−4), the IC50 value was higher, indicating that patients with high risk were less sensitive to methotrexate drugs (Figure 8D).
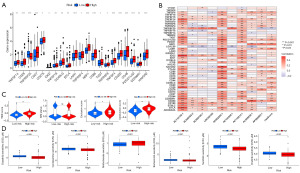
Correlation of ICDlncSig with tumor mutation burden (TMB)
Several studies have found an association between TMB and immunotherapy response (38,39). Thus, we assessed how somatic genomic mutations differed among different groups of ICDlncSig (Figure 9A, Figure S2). The top twenty genes with mutation rates exceeding 10% were identified based on both scoring subgroups. The quantitative TMB analysis revealed significant differences between groups with high and low risks (P=2.1×10−4, Figure 9B). ICDlncSig scores and TMB showed a negative correlation (R=−0.28, P=1.5×10−7, Figure 9C). After determining the optimal cutoff value for TMB (2.394), we divided the patients into groups based on their TMB levels. Compared with patients with high TMB levels, patients with low TMB levels had significantly worse survival prospects (Figure 9D). Prognostic stratification was also evaluated by combining TMB and ICDlncSig subgroups. Subsequently, we stratified the data and performed survival analysis and found that the high or low TMB did not affect the assessment of prognosis based on the ICDlncSig group (Figure 9E). In summary, ICDlncSig can make some predictions of response to immunotherapy, and this ability correlates with TMB.
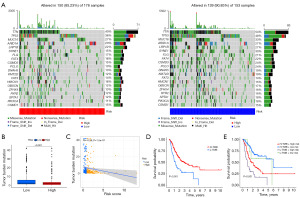
An evaluation of lncRNAs in GC samples
A comparison of the expression levels of nine lncRNAs in GC tissues and cell lines is shown in Figure S3 and Figure 10A. We found that although the nine genes showed different upregulation or downregulation in GC tissues and cells, only AP002954.1 and AP000695.1 exhibited consistent upregulation in both GC tissues and all GC cells. Therefore, we explored the effects of AP002954.1 and AP000695.1 on GC cells. We found that successful knockdown of AP002954.1 and AP000695.1 resulted in varying degrees of decrease in the proliferation rate and quantity of GC cells (Figure 10B-10D). Additionally, transwell experiments also demonstrated a corresponding reduction in the number of migrated and invasive GC cells after knockdown of AP002954.1 and AP000695.1 (Figure 10E,10F). AP002954.1 and AP000695.1, two cancer genes found in the model, are believed to promote GC cell proliferation, migration, and invasion.
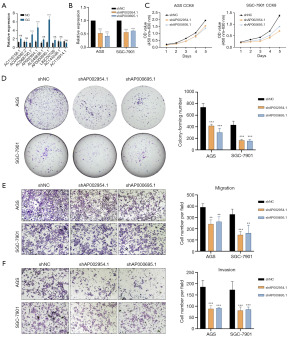
Discussion
In recent research, tumor immunotherapy, which activates the immune system to combat tumor cells, has demonstrated promising capabilities in fostering specific anti-tumor immunity and long-term immune memory (40,41). However, the immunosuppressive nature of the tumor microenvironment renders most patients insensitive to such treatments, thus limiting the effectiveness of immunotherapy. To overcome this obstacle, the induction of ICD has been proposed as an effective strategy. ICD can activate the immune system by releasing DAMPs and tumor-associated antigens, transforming immunosuppressive “cold” tumors into immune-active “hot” tumors. This conversion enhances the immunological microenvironment and promotes adaptive anti-tumor immune responses (42,43).
In therapeutic approaches that achieve ICD, modalities such as chemotherapy, targeted therapy, oncolytic viruses, photodynamic therapy (PDT), photothermal therapy (PTT), and radiation therapy have all been shown to possess the capability to induce ICD (44-46). Notably, when these treatments are integrated with nanotechnology-based drug delivery systems, they can effectively enhance therapeutic efficacy and exhibit remarkable outcomes in tumor treatment (40,47-49). Moreover, lncRNAs play intricate roles in the development and progression of tumors, influencing tumor behavior through the regulation of cellular proliferation, apoptosis, and necroptosis (50,51). Recent studies suggest that certain lncRNAs may play a pivotal role in tumor immune evasion by affecting ICD regulation. Furthermore, prognostic models based on lncRNAs signatures for patients with GC have been reported (52-54). However, due to GC’s high heterogeneity and the variability in analytical strategies employed in these studies, the predictive accuracy of these models requires improvement. Hence, researchers are tasked with further exploring lncRNAs features associated with ICD to enhance the accuracy of prognostic predictions and devise more personalized therapeutic strategies. This effort could not only provide new perspectives for the treatment of GC but could also serve as a reference for the therapy of other tumor types, especially in scenarios involving the combined use of ICD-inducing treatments and immunotherapy. Such integrative research endeavors promise to unveil critical molecular and cellular mechanisms underlying tumor immunology, thereby propelling the innovation of cancer treatment modalities.
Using nine ICD-related lncRNAs, we derived a prognostic signature for GC that could serve as an independent predictive factor. The ICDlncSig can thus be used to predict the survival of GC patients as a prognostic model. In three datasets the ICDlncSig model showed moderate predictive power. Furthermore, the newly developed nomogram can be used to intuitively predict the GC patients’ survival and improve clinical decision-making. Nine lncRNAs were identified as being associated with poor prognosis, including AC116158.1, AC048382.2, AC068790.7, AP0029541, AL049838.1, AP000695.1, LINC00106, AC144548.1 and AC116914.1. These nine lncRNAs have been included in previous studies to predict the prognosis of GC patients. For instance, Wang et al. constructed an lncRNA signature related to autophagy, which includes AC068790.7 and LINC00106, as effective prognostic indicators. This signature effectively guides immunotherapy and precision treatment for patients with GC (55). Zhang et al. constructed an inflammation-related lncRNAs signature, which includes ten lncRNAs such as AP000695.1. With an AUC of 0.788, this signature substantiates its utility in predicting the prognosis of GC (56). Wu et al. constructed a risk score model for lncRNAs that predicted the prognosis of GC patients, which contained LINC00106 in the lncRNA and was more accurate for patients with stage II–IV (57). All these studies suggest that it is more likely that the above molecules have a role in regulating the biological function of GC.
In this study, we investigated the molecular mechanisms by which ICDlncSig participates in GC by GO, KEGG, and GSVA pathway enrichment analysis and showed that TGF_BETA_SIGNALING, PANCREAS_BETA_CELLS and pathways, which was common in cancer, might play a vital role in the poor prognoses of GC patients who were labeled with high risk. Previous study has shown that LINC00106 is lowly expressed in thyroid patients, and it attenuates the migration and invasive ability of TC by inhibiting EMT (58). A comprehensive understanding of the important interactions between ICDlncSig and tumor immunomodulation is therefore crucial for the development of clinical immunotherapy. ImmuneScore, StromalScore, immune cell subpopulations, and immune checkpoints are suitable tools for characterizing the immune microenvironment of GC. As a result of immunosuppression, tumor cells can evade immune surveillance, promoting their survival and progression (59,60). According to the results of our analysis, GC patients with high-risk status had higher levels of immune cell infiltration than those with low-risk status. The findings also suggest that ICDlncSig can be viewed as a novel immune indicator of GC in the high-risk group. The clinical decision-making surrounding cancer treatment has also been improved by immune checkpoint inhibitors (61,62). An extensive list of immune checkpoint genes was upregulated in patients with renal clear cell carcinoma with a high risk, according to Bai et al. (63). Wang et al. also found that high-risk GC patients expressed higher levels of PD-L1, PD-1, and PDCD1LG2 (55). As in previous studies, ICDlncSig scores were positively correlated with the levels of immunoglobulin-like growth factor receptor genes (TNFSF4, TNFSF14, PDCD1LG2, CD28, CD27). The key finding of our study is that high-risk GC patients exhibited increased sensitivity to dasatinib, clobazam, bortezomib, sunitinib, and imatinib, which demonstrated significant anti-tumor activity in our research. This provides crucial insights for further exploration of their potential application in GC treatment. Our ICDlncSig model may assist clinicians in predicting the likelihood of patients responding to immunotherapy, thereby allowing for more personalized treatments.
In spite of the fact that ICDlncSig shows promise, the current study has a number of limitations. As noted, we validated our signature mainly with the TCGA database, and external validation of ICDlncSig needs to be done with a different database and large multicenter cohort. In addition, we need to explore the key roles of these nine ICD-related lncRNAs in GC to further improve the later clinical treatment modalities.
Conclusions
In summary, the ICD-related lncRNAs signature we constructed can effectively predict the prognosis of GC patients and may become a clinical guidance tool in the future. Moreover, the key lncRNAs in the model, AP002954.1 and AP000695.1, can regulate the proliferation, migration, and invasion of GC cells, and may serve as therapeutic targets for GC patients.
Acknowledgments
Funding: This project was supported by
Footnote
Reporting Checklist: The authors have completed the TRIPOD reporting checklist. Available at https://tcr.amegroups.com/article/view/10.21037/tcr-24-344/rc
Data Sharing Statement: Available at https://tcr.amegroups.com/article/view/10.21037/tcr-24-344/dss
Peer Review File: Available at https://tcr.amegroups.com/article/view/10.21037/tcr-24-344/prf
Conflicts of Interest: All authors have completed the ICMJE uniform disclosure form (available at https://tcr.amegroups.com/article/view/10.21037/tcr-24-344/coif). The authors have no conflicts of interest to declare.
Ethical Statement: The authors are accountable for all aspects of the work in ensuring that questions related to the accuracy or integrity of any part of the work are appropriately investigated and resolved. The study was conducted in accordance with the Declaration of Helsinki (as revised in 2013).
Open Access Statement: This is an Open Access article distributed in accordance with the Creative Commons Attribution-NonCommercial-NoDerivs 4.0 International License (CC BY-NC-ND 4.0), which permits the non-commercial replication and distribution of the article with the strict proviso that no changes or edits are made and the original work is properly cited (including links to both the formal publication through the relevant DOI and the license). See: https://creativecommons.org/licenses/by-nc-nd/4.0/.
References
- Smyth EC, Nilsson M, Grabsch HI, et al. Gastric cancer. Lancet 2020;396:635-48. [Crossref] [PubMed]
- Sung H, Ferlay J, Siegel RL, et al. Global Cancer Statistics 2020: GLOBOCAN Estimates of Incidence and Mortality Worldwide for 36 Cancers in 185 Countries. CA Cancer J Clin 2021;71:209-49. [Crossref] [PubMed]
- González CA, Megraud F, Buissonniere A, et al. Helicobacter pylori infection assessed by ELISA and by immunoblot and noncardia gastric cancer risk in a prospective study: the Eurgast-EPIC project. Ann Oncol 2012;23:1320-4. [Crossref] [PubMed]
- Choi IJ, Kim CG, Lee JY, et al. Family history of gastric cancer and helicobacter pylori treatment. N Engl J Med. 2020;382:427-36. [Crossref] [PubMed]
- Zhang LH, Wang Z, Li LH, et al. Vestigial like family member 3 is a novel prognostic biomarker for gastric cancer. World J Clin Cases 2019;7:1954-63. [Crossref] [PubMed]
- Allemani C, Weir HK, Carreira H, et al. Global surveillance of cancer survival 1995-2009: analysis of individual data for 25,676,887 patients from 279 population-based registries in 67 countries (CONCORD-2). Lancet 2015;385:977-1010. [Crossref] [PubMed]
- Abdi E, Latifi-Navid S, Abedi Sarvestani F, et al. Emerging therapeutic targets for gastric cancer from a host-Helicobacter pylori interaction perspective. Expert Opin Ther Targets 2021;25:685-99. [Crossref] [PubMed]
- Zhuang M, Gao W, Xu J, et al. The long non-coding RNA H19-derived miR-675 modulates human gastric cancer cell proliferation by targeting tumor suppressor RUNX1. Biochem Biophys Res Commun 2014;448:315-22. [Crossref] [PubMed]
- Tan Y, Chen Q, Xing Y, et al. High expression of COL5A2, a member of COL5 family, indicates the poor survival and facilitates cell migration in gastric cancer. Biosci Rep 2021;41:BSR20204293. [Crossref] [PubMed]
- Bardhan K, Anagnostou T, Boussiotis VA. The PD1:PD-L1/2 Pathway from Discovery to Clinical Implementation. Front Immunol 2016;7:550. [Crossref] [PubMed]
- Pulko V, Liu X, Krco CJ, et al. TLR3-stimulated dendritic cells up-regulate B7-H1 expression and influence the magnitude of CD8 T cell responses to tumor vaccination. J Immunol 2009;183:3634-41. [Crossref] [PubMed]
- Schneider T, Hoffmann H, Dienemann H, et al. Non-small cell lung cancer induces an immunosuppressive phenotype of dendritic cells in tumor microenvironment by upregulating B7-H3. J Thorac Oncol 2011;6:1162-8. [Crossref] [PubMed]
- Tieu R, Amancha PK, Villinger F, et al. TIM-3, a Possible Target for Immunotherapy in Cancer and Chronic Viral Infections. Austin Virol Retro Virol 2014;1:6. [PubMed]
- Wang F, He W, Zhou H, et al. The Tim-3 ligand galectin-9 negatively regulates CD8+ alloreactive T cell and prolongs survival of skin graft. Cell Immunol 2007;250:68-74. [Crossref] [PubMed]
- Lu X, Yang L, Yao D, et al. Tumor antigen-specific CD8+ T cells are negatively regulated by PD-1 and Tim-3 in human gastric cancer. Cell Immunol 2017;313:43-51. [Crossref] [PubMed]
- de Mingo Pulido Á, Gardner A, Hiebler S, et al. TIM-3 Regulates CD103+ Dendritic Cell Function and Response to Chemotherapy in Breast Cancer. Cancer Cell 2018;33:60-74.e6. [Crossref] [PubMed]
- Fromm PD, Kupresanin F, Brooks AE, et al. A multi-laboratory comparison of blood dendritic cell populations. Clin Transl Immunology 2016;5:e68. [Crossref] [PubMed]
- Patel J, Bozeman EN, Selvaraj P. Taming dendritic cells with TIM-3: another immunosuppressive strategy used by tumors. Immunotherapy 2012;4:1795-8. [Crossref] [PubMed]
- Apetoh L, Obeid M, Tesniere A, et al. Immunogenic chemotherapy: discovery of a critical protein through proteomic analyses of tumor cells. Cancer Genomics Proteomics 2007;4:65-70. [PubMed]
- Chaput N, De Botton S, Obeid M, et al. Molecular determinants of immunogenic cell death: surface exposure of calreticulin makes the difference. J Mol Med (Berl) 2007;85:1069-76. [Crossref] [PubMed]
- Tesniere A, Panaretakis T, Kepp O, et al. Molecular characteristics of immunogenic cancer cell death. Cell Death Differ 2008;15:3-12. [Crossref] [PubMed]
- Sato H, Suzuki Y, Yoshimoto Y, et al. An abscopal effect in a case of concomitant treatment of locally and peritoneally recurrent gastric cancer using adoptive T-cell immunotherapy and radiotherapy. Clin Case Rep 2017;5:380-4. [Crossref] [PubMed]
- Bonilla CE, Esguerra J, Mendoza Díaz S, et al. Abscopal Effect After Palliative Radiotherapy in a Patient with a Gastric Adenocarcinoma Disseminated to Retroperitoneal Space: Case Report from a Latin American Reference Center and Review of the Literature. Cureus 2019;11:e6235. [Crossref] [PubMed]
- Liu SH, Lee WJ, Lai DW, et al. Honokiol confers immunogenicity by dictating calreticulin exposure, activating ER stress and inhibiting epithelial-to-mesenchymal transition. Mol Oncol 2015;9:834-49. [Crossref] [PubMed]
- Cao J, Yang Y, Duan B, et al. LncRNA PCED1B-AS1 mediates miR-3681-3p/MAP2K7 axis to promote metastasis, invasion and EMT in gastric cancer. Biol Direct 2024;19:34. [Crossref] [PubMed]
- Ci Y, Zhang Y, Zhang X. Methylated lncRNAs suppress apoptosis of gastric cancer stem cells via the lncRNA-miRNA/protein axis. Cell Mol Biol Lett 2024;29:51. [Crossref] [PubMed]
- Wu ZH, Wang YX, Song JJ, et al. LncRNA SNHG26 promotes gastric cancer progression and metastasis by inducing c-Myc protein translation and an energy metabolism positive feedback loop. Cell Death Dis 2024;15:236. [Crossref] [PubMed]
- Wang J, Zhang J, Liu H, et al. N6-methyladenosine reader hnRNPA2B1 recognizes and stabilizes NEAT1 to confer chemoresistance in gastric cancer. Cancer Commun (Lond) 2024;44:469-90. [Crossref] [PubMed]
- Li C, Zhang Z, Peng E, et al. Role of an Exosomes-Related lncRNAs Signature in Tumor Immune Microenvironment of Gastric Cancer. Front Cell Dev Biol 2022;10:873319. [Crossref] [PubMed]
- Wang Z, Cao L, Zhou S, et al. Construction and Validation of a Novel Pyroptosis-Related Four-lncRNA Prognostic Signature Related to Gastric Cancer and Immune Infiltration. Front Immunol 2022;13:854785. [Crossref] [PubMed]
- Luo L, Li L, Liu L, et al. A Necroptosis-Related lncRNA-Based Signature to Predict Prognosis and Probe Molecular Characteristics of Stomach Adenocarcinoma. Front Genet 2022;13:833928. [Crossref] [PubMed]
- Xu M, Lu JH, Zhong YZ, et al. Immunogenic Cell Death-Relevant Damage-Associated Molecular Patterns and Sensing Receptors in Triple-Negative Breast Cancer Molecular Subtypes and Implications for Immunotherapy. Front Oncol 2022;12:870914. [Crossref] [PubMed]
- Hänzelmann S, Castelo R, Guinney J. GSVA: gene set variation analysis for microarray and RNA-seq data. BMC Bioinformatics 2013;14:7. [Crossref] [PubMed]
- Xu Q, Xu H, Chen S, et al. Immunological Value of Prognostic Signature Based on Cancer Stem Cell Characteristics in Hepatocellular Carcinoma. Front Cell Dev Biol 2021;9:710207. [Crossref] [PubMed]
- Yoshihara K, Shahmoradgoli M, Martínez E, et al. Inferring tumour purity and stromal and immune cell admixture from expression data. Nat Commun 2013;4:2612. [Crossref] [PubMed]
- Jiang P, Gu S, Pan D, et al. Signatures of T cell dysfunction and exclusion predict cancer immunotherapy response. Nat Med 2018;24:1550-8. [Crossref] [PubMed]
- Geeleher P, Cox N, Huang RS. pRRophetic: an R package for prediction of clinical chemotherapeutic response from tumor gene expression levels. PLoS One 2014;9:e107468. [Crossref] [PubMed]
- Wang F, Wei XL, Wang FH, et al. Safety, efficacy and tumor mutational burden as a biomarker of overall survival benefit in chemo-refractory gastric cancer treated with toripalimab, a PD-1 antibody in phase Ib/II clinical trial NCT02915432. Ann Oncol 2019;30:1479-86. [Crossref] [PubMed]
- Tang R, Liu X, Wang W, et al. Role of tumor mutation burden-related signatures in the prognosis and immune microenvironment of pancreatic ductal adenocarcinoma. Cancer Cell Int 2021;21:196. [Crossref] [PubMed]
- Li Z, Lai X, Fu S, et al. Immunogenic Cell Death Activates the Tumor Immune Microenvironment to Boost the Immunotherapy Efficiency. Adv Sci (Weinh) 2022;9:e2201734. [Crossref] [PubMed]
- Jin MZ, Wang XP. Immunogenic Cell Death-Based Cancer Vaccines. Front Immunol 2021;12:697964. [Crossref] [PubMed]
- Galluzzi L, Vitale I, Warren S, et al. Consensus guidelines for the definition, detection and interpretation of immunogenic cell death. J Immunother Cancer 2020;8:e000337. [Crossref] [PubMed]
- Kroemer G, Galluzzi L, Kepp O, et al. Immunogenic cell death in cancer therapy. Annu Rev Immunol 2013;31:51-72. [Crossref] [PubMed]
- Ahmed A, Tait SWG. Targeting immunogenic cell death in cancer. Mol Oncol 2020;14:2994-3006. [Crossref] [PubMed]
- Alzeibak R, Mishchenko TA, Shilyagina NY, et al. Targeting immunogenic cancer cell death by photodynamic therapy: past, present and future. J Immunother Cancer 2021;9:e001926. [Crossref] [PubMed]
- Li Y, Zhang H, Li Q, et al. CDK12/13 inhibition induces immunogenic cell death and enhances anti-PD-1 anticancer activity in breast cancer. Cancer Lett 2020;495:12-21. [Crossref] [PubMed]
- Lau TS, Chan LKY, Man GCW, et al. Paclitaxel Induces Immunogenic Cell Death in Ovarian Cancer via TLR4/IKK2/SNARE-Dependent Exocytosis. Cancer Immunol Res 2020;8:1099-111. [Crossref] [PubMed]
- Duan X, Chan C, Lin W. Nanoparticle-Mediated Immunogenic Cell Death Enables and Potentiates Cancer Immunotherapy. Angew Chem Int Ed Engl 2019;58:670-80. [Crossref] [PubMed]
- Fu L, Zhou X, He C. Polymeric Nanosystems for Immunogenic Cell Death-Based Cancer Immunotherapy. Macromol Biosci 2021;21:e2100075. [Crossref] [PubMed]
- LncRNA-HOTAIR promotes endothelial cell pyroptosis by regulating the miR-22/NLRP3 axis in hyperuricemia. J Cell Mol Med 2023;27:1918-21. [Crossref] [PubMed]
- Cao C, Li J, Li G, et al. Long Non-coding RNA TMEM220-AS1 Suppressed Hepatocellular Carcinoma by Regulating the miR-484/MAGI1 Axis as a Competing Endogenous RNA. Front Cell Dev Biol 2021;9:681529. [Crossref] [PubMed]
- Xiao S, Liu X, Yuan L, et al. A Ferroptosis-Related lncRNAs Signature Predicts Prognosis and Therapeutic Response of Gastric Cancer. Front Cell Dev Biol 2021;9:736682. [Crossref] [PubMed]
- Zhang J, Piao HY, Wang Y, et al. Development and validation of a three-long noncoding RNA signature for predicting prognosis of patients with gastric cancer. World J Gastroenterol 2020;26:6929-44. [Crossref] [PubMed]
- Tian X, Zhu X, Yan T, et al. Differentially Expressed lncRNAs in Gastric Cancer Patients: A Potential Biomarker for Gastric Cancer Prognosis. J Cancer 2017;8:2575-86. [Crossref] [PubMed]
- Wang W, Pei Q, Wang L, et al. Construction of a Prognostic Signature of 10 Autophagy-Related lncRNAs in Gastric Cancer. Int J Gen Med 2022;15:3699-710. [Crossref] [PubMed]
- Zhang S, Li X, Tang C, et al. Inflammation-Related Long Non-Coding RNA Signature Predicts the Prognosis of Gastric Carcinoma. Front Genet 2021;12:736766. [Crossref] [PubMed]
- Wu Y, Deng J, Lai S, et al. A risk score model with five long non-coding RNAs for predicting prognosis in gastric cancer: an integrated analysis combining TCGA and GEO datasets. PeerJ 2021;9:e10556. [Crossref] [PubMed]
- Wang XJ, Zheng HT, Xu J, et al. LINC00106 prevents against metastasis of thyroid cancer by inhibiting epithelial-mesenchymal transition. Eur Rev Med Pharmacol Sci 2020;24:10015-21. [PubMed]
- Black GE, Sokol KK, Moe DM, et al. Impact of a novel phosphoinositol-3 kinase inhibitor in preventing mitochondrial DNA damage and damage-associated molecular pattern accumulation: Results from the Biochronicity Project. J Trauma Acute Care Surg 2017;83:683-9. [Crossref] [PubMed]
- Kuroda H, Jamiyan T, Yamaguchi R, et al. Tumor microenvironment in triple-negative breast cancer: the correlation of tumor-associated macrophages and tumor-infiltrating lymphocytes. Clin Transl Oncol 2021;23:2513-25. [Crossref] [PubMed]
- Llovet JM, Montal R, Sia D, et al. Molecular therapies and precision medicine for hepatocellular carcinoma. Nat Rev Clin Oncol 2018;15:599-616. [Crossref] [PubMed]
- Pitt JM, Vétizou M, Daillère R, et al. Resistance Mechanisms to Immune-Checkpoint Blockade in Cancer: Tumor-Intrinsic and -Extrinsic Factors. Immunity 2016;44:1255-69. [Crossref] [PubMed]
- Bai Z, Zhao Y, Yang X, et al. A Novel Prognostic Ferroptosis-Related Long Noncoding RNA Signature in Clear Cell Renal Cell Carcinoma. J Oncol 2022;2022:6304824. [Crossref] [PubMed]