The m5C/m6A/m7G-related non-apoptotic regulatory cell death genes for the prediction of the prognosis and immune infiltration status in hepatocellular carcinoma
Highlight box
Key findings
• We have developed a prognostic model with 5-methylcytosine/N6-methyladenosine/N7-methylguanosine (m5C/m6A/m7G)-related non-apoptotic regulatory cell death (NARCD) genes to predict the prognosis of hepatocellular carcinoma (HCC) patients. This model can offer insights into the effectiveness of immunotherapy and chemotherapy for HCC patients.
What is known and what is new?
• The effects of m5C/m6A/m7G-related genes and NARCD-related genes on cancer progression have been reported, but few studies have examined the effects of m5C/m6A/m7G-related NARCD genes on HCC progression.
• We established the link between m5C/m6A/m7G and NARCD and constructed a prognostic model with m5C/m6A/m7G-related NARCD genes for HCC.
What is the implication, and what should change now?
• This model can predict the prognosis of HCC patients and offer insights into the effectiveness of immunotherapy and chemotherapy for HCC patients.
Introduction
Liver cancer ranks as the sixth most prevalent malignancy worldwide and represents the third leading cause of cancer-related mortality. In 2022 alone, there were approximately 865,000 new cases, resulting in 757,948 fatalities (1). Hepatocellular carcinoma (HCC) accounts for over 90% of all liver cancer cases, making it the primary type of liver cancer. Its principal etiological factors include viral hepatitis, cirrhosis, alcohol consumption, and exposure to aflatoxin-containing foods (2). Despite remarkable advances in surgical techniques, chemotherapy, and nanoparticle technology (3), the prognosis for HCC remains bleak, with a less than 20% 5-year survival rate (4). This underscores the pressing need for a novel prognostic model to facilitate clinical treatment decisions. Regulatory cell death (RCD), often referred to as programmed cell death (PCD), is a precise mechanism for regulating cell demise through well-defined signaling pathways vital for maintaining internal homeostasis and normal tissue growth. RCD can be categorized into apoptotic and non-apoptotic forms. Non-apoptotic RCD (NARCD) encompasses various modalities, including autophagy, ferroptosis, pyroptosis, and necroptosis (5-7). Recent research highlights the considerable influence of NARCD on tumor development. For instance, inhibition of STAT3 triggers ferroptosis, inhibiting gastric cancer progression (8). USP15 enhances autophagy via the TRAF6-BECN1 axis, promoting lung cancer migration and invasion (9). Downregulation of NEK7 induces pyroptosis, inhibiting HCC progression (10). Resibufogenin hinders colorectal cancer growth and metastasis through RIP3-mediated necroptosis (11). Additionally, NARCD is closely associated with antitumor immunity. Autophagy represents an immunogenic cell death (ICD) process that augments the antitumor immune response to chemotherapeutic drugs, reversing chemoresistance in cancers like non-small-cell lung and bladder cancer (5,12). Ferroptosis, pyroptosis, and necroptosis trigger robust antitumor immunity, even in immune checkpoint inhibitor (ICI)-resistant tumors, synergistically enhancing ICI efficacy, holding substantial promise for immunotherapy (13).
Common RNA methylation modifications encompass 5-methylcytosine (m5C), N6-methyladenosine (m6A), and N7-methylguanosine (m7G). Recent studies have revealed that the expression levels of m5C/m6A/m7G-related genes are closely associated with HCC occurrence and progression. Notably, NSUN2-mediated m5C promotes HCC progression by upregulating the expression of the target long non-coding RNA (lncRNA) H19 (14). METTL14-mediated m6A promotes pancreatic cancer growth and metastasis by downregulating PERP expression (15). METTL1, upregulated in HCC, impacts HCC progression via the PTEN/AKT signaling pathway (16). Furthermore, m5C/m6A/m7G-related genes play a pivotal role in regulating NARCD processes (17,18). METTL1 promotes esophageal squamous cell carcinoma through the RPTOR/ULK1/autophagy axis (19). m6A modification enhances ferroptosis by inhibiting SLC7A11 deadenylation in HCC (20). METTL3-mediated m6A promotes necroptosis in colon cancer by reducing TRAF5 expression (21). YTHDF1 enhances autophagy in HCC by promoting the translation of autophagy-related genes, ATG2A and ATG14 (22). Resistance to cell death is a hallmark of cancer cells, and m5C/m6A/m7G-related genes emerge as pivotal players in HCC progression. Thus, studying m5C/m6A/m7G-related NARCD genes offers new avenues for identifying prognostic biomarkers and exploring innovative approaches to treat HCC.
We curated m5C/m6A/m7G-related genes and NARCD-related genes from both literature and public databases and subsequently identified m5C/m6A/m7G-related NARCD genes. Through the utilization of least absolute shrinkage and selection operator (LASSO) regression, we minimized the inclusion of overfitted genes and employed multivariate Cox regression to establish prognostic models. Our model’s accuracy was validated using the International Cancer Genome Consortium (ICGC) database. Furthermore, we delved into the biological functions of the risk genes, immune profiles, and drug responses in both high- and low-risk groups. These findings hold significant promise for the discovery of new biomarkers and the exploration of novel therapeutic strategies for HCC. We present this article in accordance with the TRIPOD reporting checklist (available at https://tcr.amegroups.com/article/view/10.21037/tcr-24-499/rc).
Methods
Data acquisition and preparation
The study was conducted in accordance with the Declaration of Helsinki (as revised in 2013). Transcriptome data from 374 HCC samples were sourced as our training dataset from The Cancer Genome Atlas (TCGA) database (https://www.ncbi.nlm.nih.gov/geo/). For validation, 243 HCC samples were secured from the ICGC database (https://dcc.icgc.org/). Additionally, we obtained m5C/m6A/m7G-related genes from the scientific literature (23-27) and the Molecular Signatures Database (MSigDB; http://software.broadinstitute.org/gsea/index.jsp) (28). NARCD-related genes were gleaned from prior research studies, the Human Autophagy database (http://www.autophagy.lu/index.html), the FerrDb database (http://www.zhounan.org/ferrdb), and MSigDB (29-34).
Cluster analysis
The “limma” package of the R software was used for the identification of differential m5C/m6A/m7G-related genes between normal and tumor HCC groups, employing stringent criteria: |log2fold change (FC)| >1 and P<0.05. Subsequently, we conducted a univariate Cox analysis on these differentially expressed genes. Then, the “ConsensusClusterPlus” package of R software was used for cluster analysis of the HCC sample. The optimal clustering configuration was determined based on the cumulative distribution function (CDF) and the area under the CDF curve. The survival divergence among clusters was visualized via Kaplan-Meier curves.
Uncovering NARCD genes linked to m5C/m6A/m7G
To identify NARCD genes associated with m5C/m6A/m7G, we employed the “pheatmap” package to depict the expression of prognostic genes within distinct groups. Differential gene analysis was performed on groups showcasing high and low expressions using the “limma” package with stringent criteria (|log2FC| >1 and P<0.05). We uncovered intersection genes between differential genes and NARCD genes using a Venn diagram. Co-expression analysis of these intersection genes and m5C/m6A/m7G prognostic genes, driven by Pearson correlation (cor >0.3, P<0.001), unveiled the m5C/m6A/m7G-related NARCD gene.
Construction of a prognostic model
To construct a robust prognostic model, we initiated with univariate Cox analysis of the m5C/m6A/m7G-related NARCD genes. Employing LASSO regression acted as a measure to prevent overfitting. Multifactorial Cox regression was subsequently employed for model development. Risk scores were calculated using the gene expression and the corresponding Cox regression coefficients (coef). Risk score = Σcoef × gene expression. Patients were then classified into high- and low-risk groups based on their median risk scores. Kaplan-Meier curves, crafted using the “survival” and “survminer” packages, highlighted survival disparities. The predictive power of the model was evaluated via 1-/2-/3-year receiver operating characteristic (ROC) curves using the “timeROC” package. External validation was conducted using the ICGC database, and risk scores were computed identically to the training group, with Kaplan-Meier and ROC curves employed for validation.
Nomogram development
For the construction of a prognostic nomogram predicting 1-/2-/3-year survival rates in HCC patients, we harnessed the “rms” package. The accuracy of the nomogram in predicting patient survival was assessed through calibration curves.
Functional enrichment analysis
Differential gene analysis was employed for the high- and low-risk groups using the “limma” package with a stringent threshold of |log2FC| >1 and P<0.05. Gene Ontology (GO) and Kyoto Encyclopedia of Genes and Genomes (KEGG) analyses were conducted to unveil the biological functions associated with these differentially expressed genes.
Analysis of immune cell infiltration
The quantification of immune cell infiltration was conducted with a suite of sophisticated computational tools, namely XCELL, TIMER, QUANTISEQ, MCPCOUNTER, EPIC, CIBERSORT-ABS, and CIBERSORT. Spearman correlation analysis was employed to scrutinize the association between risk scores and immune cell infiltration. Furthermore, to gain insight into the immune landscape, a single sample gene set enrichment analysis (ssGSEA) (35) was executed, revealing the scores pertaining to immune cells and immune function within both high- and low-risk cohorts. The evaluation of Tumor Immune Dysfunction and Exclusion (TIDE) scores, which mirror immunotherapeutic efficacy, was facilitated through the TIDE database (http://tide.dfci.harvard.edu) (36) for the high- and low-risk groups.
Cell communication analysis
For an in-depth examination of cellular communication, single-cell transcriptome data from 12 primary HCC samples and six recurrent HCC samples were obtained from the Chinese National Genebank database (https://db.cngb.org/cnsa/; CNSA: CNP0000650) (37). The transcriptome data underwent normalization via the “Seurat” package’s NormalizeData function, and the top 2,000 variably expressed genes were identified using FindVariableFeatures. Cell types were annotated using data provided by the source (38). Subsequently, the “CellChat” package was leveraged to unravel the intricacies of cell-to-cell communication networks.
Drug sensitivity analysis
In the pursuit of assessing drug sensitivity, the “pRRophetic” package in R software was engaged to determine the half-maximal inhibitory concentration (IC50) for chemotherapy drugs within the high- and low-risk cohorts. An exploration of potential therapeutic compounds was undertaken, utilizing the CMAP database (https://clue.io/) (39). Compound assessments spanned a range from −100 to 100, where a positive score indicated the potential to induce or exacerbate the disease, and a negative score suggested the potential to mitigate or reverse the ailment. Compounds with scores less than −80 were pinpointed as promising candidates for disease treatment.
Quantitative real-time polymerase chain reaction (qRT-PCR)
Human normal hepatocyte cell line (HL7702) and HCC cell lines (Huh7, HepG2, Hep3B) were sourced from Beyotime Biotechnology (Shanghai, China). RNA extraction from these cell lines was conducted using TRIzol reagent (Beyotime Biotechnology). Subsequent complementary DNA (cDNA) synthesis was executed according to the manufacturer’s stipulations, utilizing moloney murine leukemia virus (M-MLV) reverse transcriptase (Beyotime Biotechnology). To ensure data fidelity, the robust reference gene glyceraldehyde-3-phosphate dehydrogenase (GAPDH) was employed as an endogenous control, and each sample was subjected to three replicates. The 2−∆∆CT method was employed for the precise quantification of the relative expression of risk genes.
Statistical analysis
The statistical backbone of this study was R software version 4.1.2. Differences between the two groups were rigorously examined via the Wilcoxon test, while proportions were compared using the Chi-squared test. A threshold of P<0.05 was upheld, signifying statistical significance in the analysis.
Results
Differential expression and prognostic analysis of m5C/m6A/m7G-related genes
We gathered a total of 81 m5C/m6A/m7G-related genes from published sources and MSigDB, revealing differential expression in 70 genes. Upon analysis, we observed 15 differential genes in m5C: 14 were upregulated, and one was downregulated (Figure 1A). For m6A, all 24 differential genes were upregulated (Figure 1B), and for m7G, 31 differential genes were found—29 were upregulated, and two were downregulated (Figure 1C). We also mapped the frequency and locations of copy number mutations in m5C/m6A/m7G-related genes (Figure 1D-1I). Subsequently, we conducted a prognostic analysis, identifying 52 prognostic genes in total: 14 from m5C, 19 from m6A, and 19 from m7G (Figure 2A).
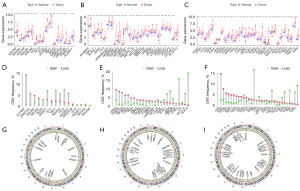
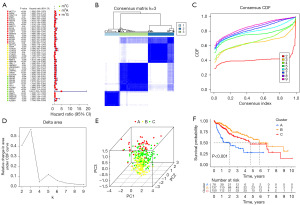
Identification of NARCD genes associated with m5C/m6A/m7G
Utilizing CDF and the area under the CDF curve, we determined that k=3 was the optimal clustering (Figure 2B-2D). Principal Component Analysis (PCA) showcased significant distinctions among the three groups (Figure 2E). Survival analysis demonstrated varied survival rates; cluster B had the higher survival rates compared to clusters A and C (Figure 2F). We visualized the expression of m5C/m6A/m7G-related prognostic genes in the three clusters using a heatmap, revealing cluster A with the highest gene expression and cluster B with the lowest (Figure 3A). By comparing the high-expression cluster (cluster A) and the low-expression cluster (cluster B), we identified 9,949 differential genes and found 175 genes in common between the differential genes and NARCD genes (Figure 3B). We further explored the correlation between these 175 genes and the m5C/m6A/m7G-related prognostic genes (Figure 3C) and ultimately pinpointed 140 m5C/m6A/m7G-related NARCD genes (Figure 4A,4B).
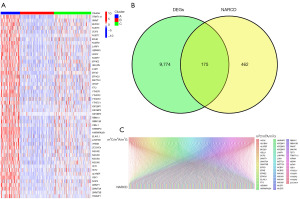
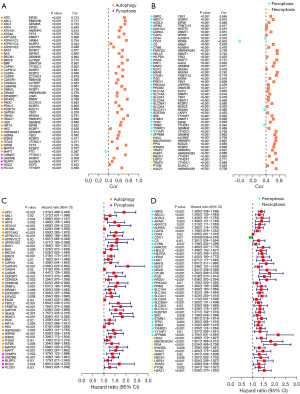
Establishment and validation of the prognostic model
We conducted univariate Cox analysis on 140 NARCD genes related to m5C/m6A/m7G, resulting in the identification of 97 prognosis-associated genes (Figure 4C,4D). Using LASSO regression, we eliminated overfit genes (Figure 5A,5B) and subsequently constructed a multivariate Cox regression model. The risk score was calculated as follows: Risk score = 0.007733 × ATIC + 0.005042 × STMN1 + 0.011566 × HILPDA + 0.003343 × TXNRD1 + 0.076860 × MAPT. Patients were categorized into high- and low-risk groups based on the median risk score. Compared to the high-risk group, the low-risk group exhibited significantly higher overall survival (OS), disease-specific survival (DSS), disease-free interval (DFI), and progression-free interval (PFI) rates (Figure 5C, Figure S1A-S1C). Model performance was assessed using the area under the ROC curve (AUC). The AUC for predicting 1-, 2-, and 3-year OS were 0.784, 0.700, and 0.692, respectively (Figure 5D). The AUC for predicting 1-, 2-, and 3-year DSS were 0.809, 0.733, and 0.730, respectively (Figure S1D). The AUC for predicting 1-, 2-, and 3-year DFI were 0.654, 0.631, and 0.625, respectively (Figure S1E). Finally, the AUC for predicting 1-, 2-, and 3-year PFI were 0.658, 0.650, and 0.636, respectively (Figure S1F). Survival status distribution plots show that as risk scores increase, patients have higher mortality rates and lower survival rates. Heatmap showing 5 risk genes highly expressed in the high-risk group (Figure 5E-5G). To validate our findings, we employed the ICGC dataset for external validation. The risk score was computed using the same formula as the training group. Patients were categorized into high- and low-risk groups based on the median risk score. The results revealed that the high-risk group had significantly lower OS than the low-risk group (Figure 6A). The AUC for predicting 1-, 2-, and 3-year OS were 0.783, 0.785, and 0.803, respectively (Figure 6B). Heatmap of the validation group showed high expression of risk genes in the high-risk group (Figure 6C-6E). In summary, our m5C/m6A/m7G-related NARCD prognostic model demonstrates good accuracy in predicting HCC outcomes.
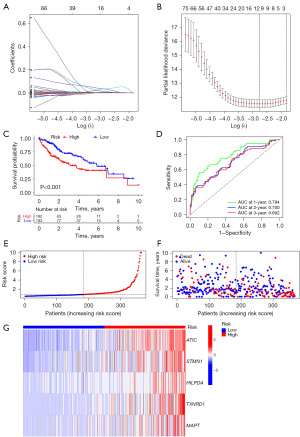
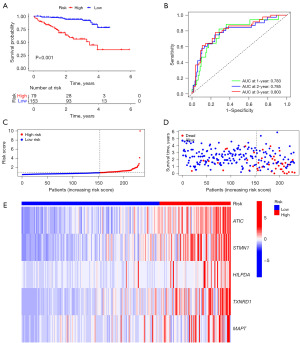
Clinical correlation analysis
Our study aimed to unveil the intricate relationship between risk scores and clinical attributes. In the TCGA cohort, analysis of the correlation between gender and risk scores showed P=0.97 and the analysis of the correlation between gender and risk score showed P=0.40. However, a parallel rise in risk scores with the increasing severity of both tumor grade and clinical stage (Figure S2A-S2D). The same pattern held true within the ICGC cohort, where analysis of the correlation between age and risk scores showed P=0.09 and the analysis of the correlation between gender and risk score showed P=0.78. Furthermore, it was evident that patients in clinical stages III–IV bore higher risk scores compared to their counterparts in clinical stages I–II (Figure S2E-S2G).
Building a nomogram
In our pursuit of enhancing the precision of prognostication for HCC patients, we devised nomograms incorporating age, gender, tumor grade, tumor-node-metastasis (TNM) classification, and risk scores (Figure 7A). These nomograms prophesied the survival rates of HCC patients at 1-, 2-, and 3-year, yielding values of 0.826, 0.725, and 0.679. The outcomes of the calibration curve unequivocally affirmed the nomogram; acumen in prognosticating survival rates for HCC patients (Figure 7B). Furthermore, the ensemble of multiple ROC curves underscored the preeminence of the combined predictive power of clinical factors and risk scores over that of individual factors (Figure 7C-7E).
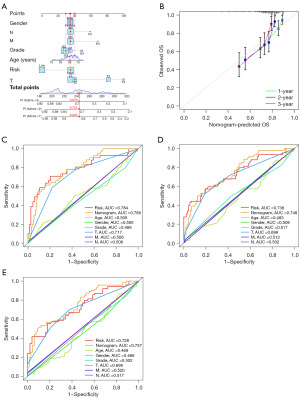
Gene enrichment analysis
To delve into the biological functionalities of risk genes, we meticulously subjected 2,196 differentially expressed genes between the high- and low-risk groups to KEGG and GO analyses. These analyses showed that KEGG pathways exhibited pronounced enrichments in cancer-related domains, for example, bladder cancer, small-cell lung carcinoma, HCC, and the p53 signaling pathway (Figure 8A). The GO analysis encompassed molecular functions (MFs), biological processes (BPs), and cellular components (CCs). Notably, MF enrichments featured functions associated with antigen binding, immunoglobulin receptor binding, and Toll-like receptor binding. The BP category was notably rich in processes including phagocytosis, immunoglobulin-mediated immune responses, and cellular responses to tumor necrosis factors. Furthermore, the CC category enrichments in domains such as immunoglobulin complexes, chromosomal regions, and gene nuclei (Figure 8B). It is worth emphasizing that the GO enrichment results were inherently intertwined with immune-related functions.
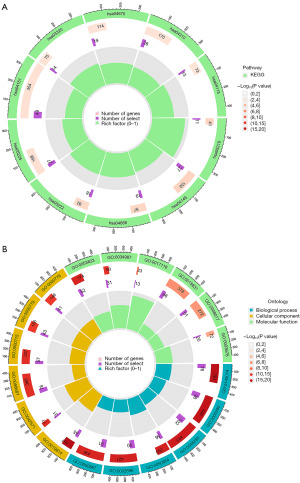
Analysis of the immune cell infiltration
We conducted an analysis to establish the relationship between risk scores and immune cell infiltration. Our findings indicated a positive correlation between risk scores and the majority of immune cell types (Figure 9A). Following this, we delved into a ssGSEA analysis comparing the high- and low-risk groups, focusing on variations in both immune cell abundance and immune-related functions. Within the realm of immune cell populations, several were significantly elevated in the high-risk group, including activated dendritic cells (aDCs), immature dendritic cells (iDCs), macrophages, follicular helper T (Tfh) cells, T helper 1 (Th1) cells, and regulatory T (Treg) cells. On the contrary, mast cells and natural killer (NK) cells displayed a marked reduction in the high-risk group. We also observed noteworthy increases in immune-related functions such as antigen-presenting cells (APCs) co-stimulation, C-C chemokine receptor (CCR), and checkpoint-related activities, alongside elevated expression of human leukocyte antigen (HLA) and major histocompatibility complex I (MHC I) within the high-risk group. Intriguingly, there was a decreased type II interferon (IFN) response in the same high-risk group (Figure 9B). Human tumors are known to exhibit a spectrum of immune subtypes, categorized as C1 (wound healing), C2 (IFN-γ dominant), C3 (inflammation), C4 (lymphocyte depletion), C5 (immunosilence), and C6 [transforming growth factor-β (TGF-β) dominant] (40). Notably, it was evident that both C5 and C6 were absent in the context of HCC. Further exploration of the correlation between each immune subtype and risk scores yielded a significant connection between C1 and high-risk scores, while C3 exhibited a distinct association with low-risk scores (Figure 9C). ICIs hold a pivotal role in the realm of tumor immunotherapy. Our investigation assessed the expression levels of three common immune checkpoints—programmed cell death protein 1 (PD-1), programmed cell death ligand 1 (PD-L1), and cytotoxic T-lymphocyte-associated protein 4 (CTLA4)—in both high- and low-risk groups. Strikingly, our analysis illuminated that all three immune checkpoints were robustly expressed in the high-risk group (Figure 9D-9F). Subsequently, TIDE scores were calculated for the high- and low-risk groups, unveiling a higher TIDE score in the low-risk group (Figure 9G). This intriguing discovery hints at potentially reduced responsiveness to immunotherapy among patients in the low-risk group.
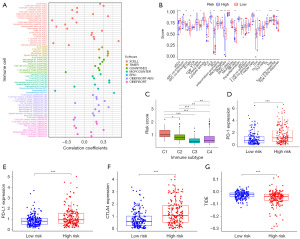
Cell communication analysis
The analysis of immune cell infiltration yielded intriguing results, indicating a heightened level of immune cell infiltration within the high-risk group. This prompted us to delve further into the intricate realm of cellular communication between tumor cells and immune cells in the context of the high-risk group. Our exploration into this cellular communication revealed a network of interactions that sheds light on the signaling mechanisms at play. Specifically, we observed that tumor cells within the high-risk group engaged in active signaling processes through the insulin-like growth factor (IGF) and macrophage migration inhibitory factor (MIF) signaling pathways, reaching out to a spectrum of immune cell types. This communication was directed towards myeloid cells, NK cells, T cells, plasmacytoid dendritic cells (pDCs), and B cells (Figure 10A-10D). Expanding our analysis, we uncovered an intricate interplay where tumor cells in the high-risk group also acted as signal receivers, responding to signals from a variety of immune cells. This dynamic interaction unfolded through the bone morphogenetic protein (BMP) and lymphotoxin-related inducible ligand (LIGHT) signaling pathways, allowing tumor cells to accept signals from myeloid cells, NK cells, T cells, pDCs, and B cells (Figure 10E,10F).
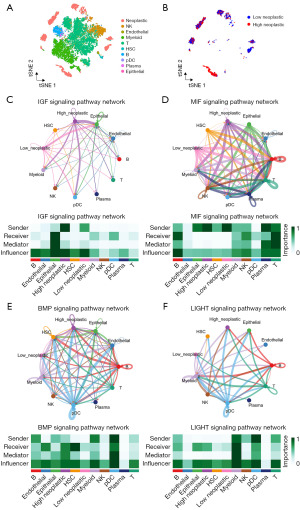
Drug sensitivity analysis
In our investigation, we harnessed the capabilities of the “pRRophetic” package within the R software to delve into the sensitivities of the high- and low-risk groups to a selection of drugs: cisplatin, doxorubicin, erlotinib, and mitomycin C. The results showed that the high-risk group demonstrated heightened sensitivity to cisplatin, doxorubicin, and mitomycin C, while the low-risk group exhibited a greater susceptibility to erlotinib (Figure 11A-11D). Delving deeper into the analysis, we took advantage of the CMAP database to scrutinize the differential genes between these two groups. This inquiry yielded a repertoire of 17 small molecular compounds, each linked to their respective targeted biological pathways (Figure 11E).
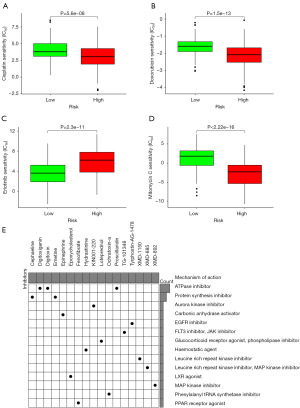
qRT-PCR
qRT-PCR was used to verify the expression of five risk genes in normal tissue and HCC. The results showed that the five risk genes ATIC, HILPDA, MAPT, STMN1, and TXNRD1 (Figure 12A-12E) were more highly expressed in HCC compared to normal tissues.
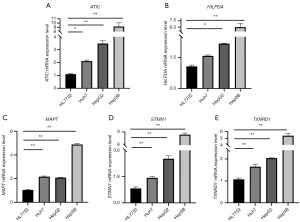
Discussion
HCC represents a foremost contributor to cancer-related mortalities globally, distinguished by its aggressive invasiveness and propensity for metastasis. HCC typically remains asymptomatic in its early stages, affording patients a mere 3-month survival window when detected at advanced stages. This presents a formidable challenge in the realms of diagnosis and treatment, with currently available therapeutic options for HCC remaining notably constrained (41-43). Notably, NARCD plays a pivotal role in the promotion of tumor cell demise and the modulation of tumor immunity (5). Genes associated with m5C/m6A/m7G modifications have been implicated in the initiation and progression of tumors (14-16). Recent investigations have illuminated the role of m5C/m6A/m7G-related genes in influencing tumor progression in HCC through their impact on NARCD (19-22). Nevertheless, the specific implications of m5C/m6A/m7G-related NARCD in HCC prognosis and immune cell infiltration remain unclear. In response, we have developed a prognostic model for m5C/m6A/m7G-related NARCD, providing a novel predictive tool for clinicians in assessing the prognosis of HCC patients.
A prognostic model has been formulated, comprising five genes: ATIC, STMN1, HILPDA, TXNRD1, and MAPT. ATIC, a versatile enzyme, plays a pivotal role in catalyzing the final two steps of de novo purine synthesis in the body. The research underscores a notable upsurge in ATIC expression within HCC, and high ATIC expression correlates with adverse prognostic outcomes. ATIC exerts its influence by orchestrating the AMPK-mTOR-S6K1 signaling cascade, thereby fostering HCC proliferation. Notably, reducing ATIC levels has shown the potential to curb HCC proliferation and migration (44). Additionally, experimental evidence indicates that ATIC can inhibit autophagy in HCC via the AKT/FOXO3 pathway (44). STMN1, characterized as a microtubule-binding protein, possesses the capacity to either promote microtubule depolymerization or suppress microtubule assembly (45). Simultaneously, STMN1 emerges as an oncogenic force, elevated in numerous cancer types and tightly linked to unfavorable cancer prognosis. STMN1 overexpression accelerates HCC cell proliferation, migration, and underpins resistance to sorafenib (46). Investigations suggest STMN1’s ability to activate the HGF/c-MET pathway, thereby stimulating HCC cell growth and invasion (46). FoxM1, by upregulating STMN1 expression, can effectively promote HCC proliferation (45). Furthermore, STMN1 facilitates HCC invasion and metastasis by modulating the epithelial-mesenchymal transition (47). HILPDA, also referred to as HIG2, is a novel lipid droplet protein that can be induced under hypoxic and glucose-deprived conditions, thereby encouraging lipid storage within cells. Research has unveiled a significant upregulation of HIG2 in HCC, correlating with an unfavorable prognosis. Silencing HIG2 has been shown to effectively inhibit the migration and invasion of HCC cells in both in vitro and in vivo settings, while also augmenting the cytotoxicity of NK cells against tumor cells (48). The PVT1/miR-150/HIG2 axis is recognized for its involvement in regulating iron metabolism in HCC (49). TXNRD1, a crucial redox enzyme, plays a pivotal role in maintaining the body’s redox equilibrium. It is noteworthy that TXNRD1 experiences significant upregulation in HCC, which is associated with an adverse prognosis. Reducing TXNRD1 levels can suppress HCC cell proliferation by restraining reactive oxygen species (ROS) levels and enhancing patient sensitivity to sorafenib (50). miR-125b-5p can impede HCC proliferation by inhibiting TXNRD1 expression (51). The inhibition of TXNRD1 through the KEAP1/NRF2 pathway by SLC27A5 effectively curbs HCC proliferation (52). MAPT, classified as a microtubule-associated protein, actively promotes microtubule assembly and preserves microtubule stability (53). Its principal expression occurs within nerve cells and is closely intertwined with neurodegenerative conditions, such as Alzheimer’s disease (54). MAPT’s role in regulating the sensitivity of breast cancer and non-small cell lung cancer to paclitaxel has been well-documented (53,55). Nevertheless, there exists no current literature directly substantiating a connection between MAPT and HCC, necessitating further experimental exploration.
In the pursuit of understanding the biological functionality of risk genes, we embarked on a quest to scrutinize the differential genes that set high- and low-risk groups apart. Employing KEGG and GO analyses, we found risk genes linked to cancer and immunity. What ensued was an exploration of immune cell infiltration within these two groups. A plethora of algorithms, including XCELL, TIMER, QUANTISEQ, MCPCOUNTER, EPIC, CIBERSORT-ABS, and CIBERSORT, were harnessed to calculate the relative proportions of immune cells, all with the aim of dissecting their interplay with risk scores. We found the majority of immune cells displayed a positive correlation with risk scores. ssGSEA painted a picture of differences in immune functionality between the high- and low-risk groups. It was evident that immune players such as aDCs, macrophages, Treg cells, and the MHC class I and II IFN responses, along with the APC co-inhibition, bore significant distinctions between the groups. Dendritic cell (DC) is an APC that activates a tumor-specific cytotoxic immune response to kill tumor cells (56). DCs are APCs that activate tumor-specific cytotoxic immune responses to kill tumor cells (56). Macrophages can be classified into two phenotypes, M1 and M2. Tumor-associated macrophages are predominantly M2 in solid cancers such as HCC and have anti-inflammatory and immunomodulatory effects.M2 macrophages can promote HCC migration by enhancing IL-1β secretion (57). Treg cells can mediate immunosuppression leading to the development of immune tolerance in HCC (58). Type II IFN response can promote cell death in HCC by inducing autophagy through IFN regulatory factor 1 (59). In the quest for deeper understanding, the relationship between immune subtypes and risk scores was probed, ultimately revealing that C1 and C2 subtypes were intimately entwined with high-risk scores, and C3 and C4 subtypes were intimately entwined with low-risk scores. ICIs of PD-1, PD-L1, and CTLA4 are currently approved for the immunotherapy of cancer patients. We delved into the expressions of these key regulators in both the high- and low-risk groups, unveiling higher expression levels within the high-stratum. This intriguing revelation suggests that ICIs might manifest superior therapeutic efficacy among patients in the high-risk category. A pivotal tool in our assessment arsenal was the TIDE score, a metric instrumental in gauging the ability of highly infiltrated cytotoxic T cells to curtail tumor escape (36). Calculating TIDE scores for both the high- and low-risk cohorts we found the low-risk assembly displayed elevated TIDE scores, signifying an augmented probability of immune evasion and subsequently, a less favorable immunotherapeutic response. Beyond the realm of immunotherapy, we embarked on an exploration of platinum, docetaxel, paclitaxel, and erlotinib sensitivities in the high- and low-risk segments. Our observations showed that the high-risk group showcased heightened responsiveness to platinum, docetaxel, and paclitaxel, while the low-risk cohort demonstrated increased sensitivity to erlotinib. We leveraged differential gene expression profiles between the high- and low-risk populations to unearth 17 small molecular compounds from the CMAP database.
Given the higher level of immune cell infiltration in the high-risk group, we delved into the cellular communications between tumor cells and immune cells within this group. The findings unveiled that tumor cells in the high-risk group signal to myeloid cells, NK cells, T cells, pDC cells, and B cells through the IGF and MIF signaling pathways. Simultaneously, they are receptive to signals from myeloid cells, NK cells, T cells, pDC cells, B cells, and plasma cells through the BMP and LIGHT signaling pathways. The IGF signaling pathway, highly conserved and pivotal in regulating growth and development, has been demonstrated to play a crucial role in various cancers, including HCC (60,61). Stromal stem cells recruited to the tumor microenvironment can downregulate IGF signaling pathway transmission, thus inhibiting HCC cell proliferation. Cancer-associated fibroblasts (CAFs) have the capacity to upregulate IGF signaling pathway transmission in non-small cell lung cancer, thereby promoting tumor cell proliferation (62). MIF, an inflammatory factor, can suppress the tumor microenvironment’s immune regulation and, in turn, facilitate tumor progression through the MIF signaling pathway (63). BMP is a member of the TGF-β family. The BMP signaling pathway has the capacity to exert influence on various immune cells, including its impact on the maturation and differentiation of DCs, the polarization of macrophages, activation and homeostasis of T cells, and the differentiation of innate lymphoid cells (ILC) (64). LIGHT belongs to the tumor necrosis factor family. The LIGHT signaling pathway plays a pivotal role in regulating both innate and adaptive immunity. This pathway can regulate immune responses by enhancing T cell proliferation, cytokine secretion, promoting DC maturation, and inducing the infiltration of naïve T lymphocytes, thus augmenting anti-tumor immunity (65,66).
Nonetheless, this study retains certain limitations. The data originated from the TCGA database and were validated by the ICGC database. While the findings offer valuable insights, the sample size remains limited, underscoring the need for additional data collection and validation. Furthermore, the mechanistic underpinnings of the five risk genes in HCC necessitate further experimental exploration.
Conclusions
In summary, we used the TCGA data to construct a prognostic model based on five m5C/m6A/m7G-related NARCD genes and validated using data from the ICGC database. Moreover, prognostic models are shown to be related to immunotherapy and chemotherapeutic drug sensitivity, which may provide a reference for predicting the effects of immunotherapy and chemotherapy.
Acknowledgments
We thank all the people who helped with this study. The datasets used in this study are publicly available from the TCGA (https://www.ncbi.nlm.nih.gov/geo/), ICGC databases (https://dcc.icgc.org/), MSigDB databases (http://software.broadinstitute.org/gsea/index.jsp), Human autophagy database (http://www.autophagy.lu/index.html), FerrDb database (http://www.zhounan.org/ferrdb), Chinese National Genebank database (https://db.cngb.org/cnsa/), and CMAP database (https://clue.io/). We would also like to thank these databases for providing data on patients with hepatocellular carcinoma for this study.
Funding: This research was supported by
Footnote
Reporting Checklist: The authors have completed the TRIPOD reporting checklist. Available at https://tcr.amegroups.com/article/view/10.21037/tcr-24-499/rc
Peer Review File: Available at https://tcr.amegroups.com/article/view/10.21037/tcr-24-499/prf
Conflicts of Interest: All authors have completed the ICMJE uniform disclosure form (available at https://tcr.amegroups.com/article/view/10.21037/tcr-24-499/coif). The authors have no conflicts of interest to declare.
Ethical Statement: The authors are accountable for all aspects of the work in ensuring that questions related to the accuracy or integrity of any part of the work are appropriately investigated and resolved. The study was conducted in accordance with the Declaration of Helsinki (as revised in 2013).
Open Access Statement: This is an Open Access article distributed in accordance with the Creative Commons Attribution-NonCommercial-NoDerivs 4.0 International License (CC BY-NC-ND 4.0), which permits the non-commercial replication and distribution of the article with the strict proviso that no changes or edits are made and the original work is properly cited (including links to both the formal publication through the relevant DOI and the license). See: https://creativecommons.org/licenses/by-nc-nd/4.0/.
References
- Bray F, Laversanne M, Sung H, et al. Global cancer statistics 2022: GLOBOCAN estimates of incidence and mortality worldwide for 36 cancers in 185 countries. CA Cancer J Clin 2024;74:229-63. [Crossref] [PubMed]
- Yang JD, Hainaut P, Gores GJ, et al. A global view of hepatocellular carcinoma: trends, risk, prevention and management. Nat Rev Gastroenterol Hepatol 2019;16:589-604. [Crossref] [PubMed]
- Anwanwan D, Singh SK, Singh S, et al. Challenges in liver cancer and possible treatment approaches. Biochim Biophys Acta Rev Cancer 2020;1873:188314. [Crossref] [PubMed]
- Li X, Li C, Zhang L, et al. The significance of exosomes in the development and treatment of hepatocellular carcinoma. Mol Cancer 2020;19:1. [Crossref] [PubMed]
- Gao W, Wang X, Zhou Y, et al. Autophagy, ferroptosis, pyroptosis, and necroptosis in tumor immunotherapy. Signal Transduct Target Ther 2022;7:196. [Crossref] [PubMed]
- Peng F, Liao M, Qin R, et al. Regulated cell death (RCD) in cancer: key pathways and targeted therapies. Signal Transduct Target Ther 2022;7:286. [Crossref] [PubMed]
- Hartman ML. Non-Apoptotic Cell Death Signaling Pathways in Melanoma. Int J Mol Sci 2020;21:2980. [Crossref] [PubMed]
- Ouyang S, Li H, Lou L, et al. Inhibition of STAT3-ferroptosis negative regulatory axis suppresses tumor growth and alleviates chemoresistance in gastric cancer. Redox Biol 2022;52:102317. [Crossref] [PubMed]
- Kim MJ, Min Y, Jeong SK, et al. USP15 negatively regulates lung cancer progression through the TRAF6-BECN1 signaling axis for autophagy induction. Cell Death Dis 2022;13:348. [Crossref] [PubMed]
- Yan Z, Da Q, Li Z, et al. Inhibition of NEK7 Suppressed Hepatocellular Carcinoma Progression by Mediating Cancer Cell Pyroptosis. Front Oncol 2022;12:812655. [Crossref] [PubMed]
- Han Q, Ma Y, Wang H, et al. Resibufogenin suppresses colorectal cancer growth and metastasis through RIP3-mediated necroptosis. J Transl Med 2018;16:201. [Crossref] [PubMed]
- Xia H, Green DR, Zou W. Autophagy in tumour immunity and therapy. Nat Rev Cancer 2021;21:281-97. [Crossref] [PubMed]
- Tang R, Xu J, Zhang B, et al. Ferroptosis, necroptosis, and pyroptosis in anticancer immunity. J Hematol Oncol 2020;13:110. [Crossref] [PubMed]
- Sun Z, Xue S, Zhang M, et al. Aberrant NSUN2-mediated m(5)C modification of H19 lncRNA is associated with poor differentiation of hepatocellular carcinoma. Oncogene 2020;39:6906-19. [Crossref] [PubMed]
- Wang M, Liu J, Zhao Y, et al. Upregulation of METTL14 mediates the elevation of PERP mRNA N(6) adenosine methylation promoting the growth and metastasis of pancreatic cancer. Mol Cancer 2020;19:130. [Crossref] [PubMed]
- Tian QH, Zhang MF, Zeng JS, et al. METTL1 overexpression is correlated with poor prognosis and promotes hepatocellular carcinoma via PTEN. J Mol Med (Berl) 2019;97:1535-45. [Crossref] [PubMed]
- Wilkinson E, Cui YH, He YY. Roles of RNA Modifications in Diverse Cellular Functions. Front Cell Dev Biol 2022;10:828683. [Crossref] [PubMed]
- Zhi Y, Zhang S, Zi M, et al. Potential applications of N(6) -methyladenosine modification in the prognosis and treatment of cancers via modulating apoptosis, autophagy, and ferroptosis. Wiley Interdiscip Rev RNA 2022;13:e1719. [Crossref] [PubMed]
- Han H, Yang C, Ma J, et al. N(7)-methylguanosine tRNA modification promotes esophageal squamous cell carcinoma tumorigenesis via the RPTOR/ULK1/autophagy axis. Nat Commun 2022;13:1478. [Crossref] [PubMed]
- Liu L, He J, Sun G, et al. The N6-methyladenosine modification enhances ferroptosis resistance through inhibiting SLC7A11 mRNA deadenylation in hepatoblastoma. Clin Transl Med 2022;12:e778. [Crossref] [PubMed]
- Lan H, Liu Y, Liu J, et al. Tumor-Associated Macrophages Promote Oxaliplatin Resistance via METTL3-Mediated m(6)A of TRAF5 and Necroptosis in Colorectal Cancer. Mol Pharm 2021;18:1026-37. [Crossref] [PubMed]
- Li Q, Ni Y, Zhang L, et al. HIF-1α-induced expression of m6A reader YTHDF1 drives hypoxia-induced autophagy and malignancy of hepatocellular carcinoma by promoting ATG2A and ATG14 translation. Signal Transduct Target Ther 2021;6:76. [Crossref] [PubMed]
- Xie S, Chen W, Chen K, et al. Emerging roles of RNA methylation in gastrointestinal cancers. Cancer Cell Int 2020;20:585. [Crossref] [PubMed]
- Ouyang W, Jiang Y, Bu S, et al. A Prognostic Risk Score Based on Hypoxia-, Immunity-, and Epithelialto-Mesenchymal Transition-Related Genes for the Prognosis and Immunotherapy Response of Lung Adenocarcinoma. Front Cell Dev Biol 2022;9:758777. [Crossref] [PubMed]
- Chen Y, Lin H, Miao L, et al. Role of N7-methylguanosine (m(7)G) in cancer. Trends Cell Biol 2022;32:819-24. [Crossref] [PubMed]
- Zhao H, Xu Y, Xie Y, et al. m6A Regulators Is Differently Expressed and Correlated With Immune Response of Esophageal Cancer. Front Cell Dev Biol 2021;9:650023. [Crossref] [PubMed]
- Xu Z, Chen S, Zhang Y, et al. Roles of m5C RNA Modification Patterns in Biochemical Recurrence and Tumor Microenvironment Characterization of Prostate Adenocarcinoma. Front Immunol 2022;13:869759. [Crossref] [PubMed]
- Liberzon A, Subramanian A, Pinchback R, et al. Molecular signatures database (MSigDB) 3.0. Bioinformatics 2011;27:1739-40. [Crossref] [PubMed]
- Fang Q, Chen H. Development of a Novel Autophagy-Related Prognostic Signature and Nomogram for Hepatocellular Carcinoma. Front Oncol 2020;10:591356. [Crossref] [PubMed]
- Xu D, Ji Z, Qiang L. Molecular Characteristics, Clinical Implication, and Cancer Immunity Interactions of Pyroptosis-Related Genes in Breast Cancer. Front Med (Lausanne) 2021;8:702638. [Crossref] [PubMed]
- Zhang X, Yang Q. A Pyroptosis-Related Gene Panel in Prognosis Prediction and Immune Microenvironment of Human Endometrial Cancer. Front Cell Dev Biol 2021;9:705828. [Crossref] [PubMed]
- Xing M, Li J. Diagnostic and prognostic values of pyroptosis-related genes for the hepatocellular carcinoma. BMC Bioinformatics 2022;23:177. [Crossref] [PubMed]
- Zhang Z, Hu X, Qiu D, et al. Development and Validation of a Necroptosis-Related Prognostic Model in Head and Neck Squamous Cell Carcinoma. J Oncol 2022;2022:8402568. [Crossref] [PubMed]
- Zhou N, Bao J. FerrDb: a manually curated resource for regulators and markers of ferroptosis and ferroptosis-disease associations. Database (Oxford) 2020;2020:baaa021. [Crossref] [PubMed]
- Hänzelmann S, Castelo R, Guinney J. GSVA: gene set variation analysis for microarray and RNA-seq data. BMC Bioinformatics 2013;14:7. [Crossref] [PubMed]
- Jiang P, Gu S, Pan D, et al. Signatures of T cell dysfunction and exclusion predict cancer immunotherapy response. Nat Med 2018;24:1550-8. [Crossref] [PubMed]
- Chen FZ, You LJ, Yang F, et al. CNGBdb: China National GeneBank DataBase. Yi Chuan 2020;42:799-809. [PubMed]
- Sun Y, Wu L, Zhong Y, et al. Single-cell landscape of the ecosystem in early-relapse hepatocellular carcinoma. Cell 2021;184:404-421.e16. [Crossref] [PubMed]
- Yang K, Dinasarapu AR, Reis ES, et al. CMAP: Complement Map Database. Bioinformatics 2013;29:1832-3. [Crossref] [PubMed]
- Desgrosellier JS, Cheresh DA. Integrins in cancer: biological implications and therapeutic opportunities. Nat Rev Cancer 2010;10:9-22. [Crossref] [PubMed]
- Chan LK, Tsui YM, Ho DW, et al. Cellular heterogeneity and plasticity in liver cancer. Semin Cancer Biol 2022;82:134-49. [Crossref] [PubMed]
- Keenan BP, Fong L, Kelley RK. Immunotherapy in hepatocellular carcinoma: the complex interface between inflammation, fibrosis, and the immune response. J Immunother Cancer 2019;7:267. [Crossref] [PubMed]
- Kim E, Viatour P. Hepatocellular carcinoma: old friends and new tricks. Exp Mol Med 2020;52:1898-907. [Crossref] [PubMed]
- Li M, Jin C, Xu M, et al. Bifunctional enzyme ATIC promotes propagation of hepatocellular carcinoma by regulating AMPK-mTOR-S6 K1 signaling. Cell Commun Signal 2017;15:52. [Crossref] [PubMed]
- Zhang H, Xia P, Liu J, et al. ATIC inhibits autophagy in hepatocellular cancer through the AKT/FOXO3 pathway and serves as a prognostic signature for modeling patient survival. Int J Biol Sci 2021;17:4442-58. [Crossref] [PubMed]
- Liu J, Li J, Wang K, et al. Aberrantly high activation of a FoxM1-STMN1 axis contributes to progression and tumorigenesis in FoxM1-driven cancers. Signal Transduct Target Ther 2021;6:42. [Crossref] [PubMed]
- Zhang R, Gao X, Zuo J, et al. STMN1 upregulation mediates hepatocellular carcinoma and hepatic stellate cell crosstalk to aggravate cancer by triggering the MET pathway. Cancer Sci 2020;111:406-17. [Crossref] [PubMed]
- Cai Y, Fu Y, Liu C, et al. Stathmin 1 is a biomarker for diagnosis of microvascular invasion to predict prognosis of early hepatocellular carcinoma. Cell Death Dis 2022;13:176. [Crossref] [PubMed]
- Cui C, Fu K, Yang L, et al. Hypoxia-inducible gene 2 promotes the immune escape of hepatocellular carcinoma from nature killer cells through the interleukin-10-STAT3 signaling pathway. J Exp Clin Cancer Res 2019;38:229. [Crossref] [PubMed]
- Xu Y, Luo X, He W, et al. Long Non-Coding RNA PVT1/miR-150/ HIG2 Axis Regulates the Proliferation, Invasion and the Balance of Iron Metabolism of Hepatocellular Carcinoma. Cell Physiol Biochem 2018;49:1403-19. [Crossref] [PubMed]
- Lee D, Xu IM, Chiu DK, et al. Induction of Oxidative Stress Through Inhibition of Thioredoxin Reductase 1 Is an Effective Therapeutic Approach for Hepatocellular Carcinoma. Hepatology 2019;69:1768-86. [Crossref] [PubMed]
- Hua S, Quan Y, Zhan M, et al. miR-125b-5p inhibits cell proliferation, migration, and invasion in hepatocellular carcinoma via targeting TXNRD1. Cancer Cell Int 2019;19:203. [Crossref] [PubMed]
- Gao Q, Zhang G, Zheng Y, et al. SLC27A5 deficiency activates NRF2/TXNRD1 pathway by increased lipid peroxidation in HCC. Cell Death Differ 2020;27:1086-104. [Crossref] [PubMed]
- Ikeda H, Taira N, Hara F, et al. The estrogen receptor influences microtubule-associated protein tau (MAPT) expression and the selective estrogen receptor inhibitor fulvestrant downregulates MAPT and increases the sensitivity to taxane in breast cancer cells. Breast Cancer Res 2010;12:R43. [Crossref] [PubMed]
- Derry PJ, Hegde ML, Jackson GR, et al. Revisiting the intersection of amyloid, pathologically modified tau and iron in Alzheimer's disease from a ferroptosis perspective. Prog Neurobiol 2020;184:101716. [Crossref] [PubMed]
- Cai Y, Jia R, Xiong H, et al. Integrative gene expression profiling reveals that dysregulated triple microRNAs confer paclitaxel resistance in non-small cell lung cancer via co-targeting MAPT. Cancer Manag Res 2019;11:7391-404. [Crossref] [PubMed]
- Cazzetta V, Franzese S, Carenza C, et al. Natural Killer-Dendritic Cell Interactions in Liver Cancer: Implications for Immunotherapy. Cancers (Basel) 2021;13:2184. [Crossref] [PubMed]
- Hu B, Lin JZ, Yang XB, et al. Aberrant lipid metabolism in hepatocellular carcinoma cells as well as immune microenvironment: A review. Cell Prolif 2020;53:e12772. [Crossref] [PubMed]
- Wang Z, He L, Li W, et al. GDF15 induces immunosuppression via CD48 on regulatory T cells in hepatocellular carcinoma. J Immunother Cancer 2021;9:e002787. [Crossref] [PubMed]
- Li P, Du Q, Cao Z, et al. Interferon-γ induces autophagy with growth inhibition and cell death in human hepatocellular carcinoma (HCC) cells through interferon-regulatory factor-1 (IRF-1). Cancer Lett 2012;314:213-22. [Crossref] [PubMed]
- El Tayebi HM, Abdelaziz AI. Epigenetic regulation of insulin-like growth factor axis in hepatocellular carcinoma. World J Gastroenterol 2016;22:2668-77. [Crossref] [PubMed]
- Moeini A, Cornellà H, Villanueva A. Emerging signaling pathways in hepatocellular carcinoma. Liver Cancer 2012;1:83-93. [Crossref] [PubMed]
- Jeong H, Lee SY, Seo H, et al. Recombinant Mycobacterium smegmatis delivering a fusion protein of human macrophage migration inhibitory factor (MIF) and IL-7 exerts an anticancer effect by inducing an immune response against MIF in a tumor-bearing mouse model. J Immunother Cancer 2021;9:e003180. [Crossref] [PubMed]
- Sconocchia T, Sconocchia G. Regulation of the Immune System in Health and Disease by Members of the Bone Morphogenetic Protein Family. Front Immunol 2021;12:802346. [Crossref] [PubMed]
- Lee EH, Kim EM, Ji KY, et al. Axl acts as a tumor suppressor by regulating LIGHT expression in T lymphoma. Oncotarget 2017;8:20645-55. [Crossref] [PubMed]
- Ware CF, Croft M, Neil GA. Realigning the LIGHT signaling network to control dysregulated inflammation. J Exp Med 2022;219:e20220236. [Crossref] [PubMed]