Development and validation of a recurrence risk assessment model for high-grade bladder cancer based on TCGA and GEO
Highlight box
Key findings
• An effective recurrence assessment model was developed and validated for high-grade bladder cancer.
What is known and what is new?
• High-grade bladder cancer has high recurrence rate and a poor prognosis. A robust recurrence prediction model is needed for patient management and clinical therapeutic decision-making. Current recurrence assessment models focus on clinical data or specific functional genes; they are limited in gene types, sample sizes, and efficiency.
• In this study, we developed a new model including various gene types and large screening data which exhibited a high efficiency in recurrence prediction for high-grade bladder cancer. This model has predictive ability for chemotherapy sensitivity, immune response, and targeted efficacy.
What is the implication, and what should change now?
• The recurrence assessment model can divide patient populations and provide a basis for the effectiveness of treatment plans.
Introduction
Bladder cancer is one of the most commonly diagnosed urinary cancers worldwide. It was reported that there were an estimated minimum of 82,290 new cases of and 16,710 deaths from bladder cancer in America in 2023 (1). Muscle-invasive bladder cancer (MIBC) accounts for only 25% of patients with bladder cancer, but it has high rate of invasion and distant metastasis, and results in a poor prognosis, especially among those with high-grade disease (2). The overall incidence of bladder cancer is increasing year by year, which might be associated with the potential influence of tobacco abuse, industrial carcinogens, and population aging (3). However, very few studies have addressed valuable molecular prognostic markers for recurrence in clinical practice; there is an urgent need to discover recurrence assessment markers for high-grade bladder cancer.
Recently, gene microarray and RNA sequencing have been applied to identify novel diagnostic and prognostic signatures for multiple diseases. Schuettfort et al. created a model using a panel of systemic inflammatory response biomarkers to predict tumor-specific survival and recurrence-free survival (RFS) in patients with urothelial carcinoma treated with radical cystectomy, but the effectiveness of selected inflammatory response biomarkers in improving the model’s discrimination ability was limited (4). Another study revealed that extracellular matrix genes could predict survival and recurrence of bladder cancer; the combination of follistatin-like 1 (FSTL1), stage, age, and gender achieved an area under the curve (AUC) value of 0.76 in predicting bladder cancer recurrence (5). Lucas et al. (6) trained a network of digital histopathology slides and clinical data to predict RFS for non-MIBC patients using deep learning. Their results showed that AUC was 0.76 for 5-year recurrence predictions. The abovementioned assessment models focus on clinical data or specific functional genes; they are limited in gene types, sample sizes, and efficiency. A recurrence assessment model with a high prediction efficiency is conducive to early clinical treatment decision-making, and vital for optimizing the prognosis of bladder cancer. Besides, as gene expression detection is convenient and stable, a predictive model constructed by multiple genes is more reliable. Thus, a new model including various gene types and large screening data and presenting a high efficiency in recurrence prediction is required for high-grade bladder cancer. We present this article in accordance with the TRIPOD reporting checklist (available at https://tcr.amegroups.com/article/view/10.21037/tcr-24-256/rc).
Methods
Data download and preprocessing
We downloaded The Cancer Genome Atlas (TCGA) RNA expression profile data of bladder cancer in the form of count value from Xena (https://xena.ucsc.edu/). The corresponding clinical information files including gender, age, tumor differentiation, and tumor stage were also downloaded. Only files containing recurrence-free time and recurrence status were included in further data analysis. The information of 413 bladder cancer patients including 77 recurrences was obtained from TCGA. The selected TCGA data were standardized in the form of transcripts per million (TPM), then, according to the distance between different samples in a cluster, Pearson correlation matrix was used to evaluate the microarray quality. We also downloaded the raw gene microarray expression profiles in the form of original files and clinical information from the Gene Expression Omnibus (GEO) database (http://www.ncbi.nlm.nih.gov/geo/). We set the criteria that sample sizes were larger than 80 and clinical information was in the same form as TCGA data. Finally, GSE5479, GSE57933, and GSE120736 were excluded, and only GSE31684 was suitable for analysis. GSE31684 included 39 recurrences out of 94 bladder cancer patients. All the data were updated as of December 2023. The study was conducted in accordance with the Declaration of Helsinki (as revised in 2013).
Screening differentially expressed RNAs and preparation of single factor risk regression input RNAs
Patients included in this study were divided into two groups according to tumor status. RNA count value in the different groups were extracted with clinical data, and Edge R (version 3.3.0; https://www.r-project.org/) was used to analyze the difference between the two groups; P<0.05 and |log2(fold change)| >1 were selected as the thresholds. Gene Ontology (GO) (http://www.geneontology.org) and Kyoto Encyclopedia of Genes and Genomes (KEGG) pathway analyses were performed to predict the function and interactions among differentially expressed RNAs. For GSE31684 data, Affy package was used to merge the matrix, robust multiarray average (RMA) method was used to standardize the data, and the probe names were transformed to gene names (7).
Evaluation of the model and risk analysis of recurrence
The expression matrix of differently expressed RNAs and the clinical recurrence status were used for single factor Cox analysis. R survival package was used for Cox analysis. RNAs related to bladder cancer recurrence with P<0.05 were obtained for further analysis. The glmnet and survival packages in R were used for least absolute shrinkage and selection operator (LASSO) analysis (8). After removing redundant RNAs, the remaining RNAs were used for multi-factor risk analysis. Finally, the recurrence risk-associated RNAs were used to construct a disease-free or recurrence evaluation model. A risk score was calculated, and according to the maximum Youden value, patients with high-grade bladder cancer in TCGA were divided into high- and low-risk groups. Then, the difference between the two groups in disease-free recurrence and survival was calculated to evaluate the effect of the model. The survival receiver operating characteristic (ROC) package of R was used to evaluate the prediction ability of the model based on the expression of screened RNAs. Finally, the model was verified in GSE31684. The tumor-node-metastasis (TNM) stages of the patients were horizontally compared with those of the model to evaluate the prediction ability in recurrence of bladder cancer. An AUC larger than 0.7 was identified as having a good prediction.
Immune assessment and drug sensitivity prediction
KEGG pathway analysis of selected genes was performed with R package. The enrichment factor was the value ratio between genes and all annotated genes enriched in the pathway. Oncopredict package of R was used to predict sensitivity to chemotherapy drugs (9). ImmuneCellAI (https://guolab.wchscu.cn/ImmuCellAI/#!/) was used to estimate the proportion of 18 types of T cells and 6 other types of immune cells [B cells, natural killer (NK) cells, monocytes, macrophages, neutrophils, and dendritic cells (DCs)], and predict the patient’s response to immune checkpoint inhibitor therapy.
Statistical analysis
Statistical analysis of different RNAs was performed with the analysis of variance (ANOVA) and P<0.05 was considered statistically significant. In comparison, P<0.05 between the two sample groups signaled the existence of differences.
Results
Characteristics of selected data
As shown in Table 1, 217 cases of clinical information of high-grade bladder cancer were selected in TCGA expression spectrum, which included 79 cases of recurrence or progression and 138 cases of complete remission (CR). In Table 2, 87 samples including 38 cases of bladder cancer recurrence and 49 cases of CR were screened from GSE31684 profiles.
Table 1
Parameters | Patients, n (%) |
---|---|
Recurrence | |
Recurrent/progressive | 79 (36.41) |
Disease-free | 138 (63.59) |
Age (years) | |
>67 | 112 (51.61) |
≤67 | 105 (48.39) |
Gender | |
Male | 159 (73.27) |
Female | 58 (26.73) |
Pathologic stage | |
Stage I | 1 (0.46) |
Stage II | 68 (31.34) |
Stage III | 73 (33.64) |
Stage IV | 75 (34.56) |
Pathologic M | |
M0 | 95 (43.78) |
M1 | 8 (3.69) |
Mx | 113 (52.07) |
Pathologic N | |
N0 | 120 (55.30) |
N1 | 24 (11.06) |
N2 | 42 (19.35) |
N3 | 4 (1.84) |
Nx | 27 (12.44) |
Pathologic T | |
T1 | 7 (3.23) |
T2 | 105 (48.39) |
T3 | 76 (35.02) |
T4 | 24 (11.06) |
Tx | 5 (2.30) |
Mx, Nx, Tx: data that had not been thoroughly evaluated. TCGA, The Cancer Genome Atlas; M, metastasis; N, node; T, tumor.
Table 2
Parameters | Patients, n (%) |
---|---|
Recurrence | |
Recurrent/progressive | 57 (65.52) |
Disease-free | 30 (34.48) |
Age (years) | |
≥67 | 63 (72.41) |
<67 | 24 (27.59) |
Gender | |
Male | 5 (5.75) |
Female | 17 (19.54) |
Pathologic T | |
Ta | 54 (62.07) |
T1 | 10 (11.49) |
T2 | 1 (1.15) |
T3 | 38 (43.68) |
T4 | 49 (56.32) |
Metastasis | |
M1 | 51 (58.62) |
M0 | 36 (41.38) |
T, tumor; M, metastasis.
Characteristic and function prediction of included differently expressed RNAs
The screening process and results are presented in Figure 1. Clustering analysis showed the characteristics of the selectively included data of TCGA. A total of 2,876 differentially expressed RNAs including 905 up-regulated RNAs and 1,971 down-regulated RNAs were obtained between tumor and normal tissues (table available at https://cdn.amegroups.cn/static/public/tcr-24-256-1.xlsx). The Volcano map well distinguished tumor and normal tissues (Figure 2A). The heatmap analysis demonstrated no significant differences between patients who had achieved CR and those with disease recurrence (Figure 2B). KEGG pathway analysis showed that the dysregulated RNAs mainly enriched in focal adhesion, calcium signaling pathway, and cell cycle pathway (Figure 2C). GO analysis revealed that regulation of mitotic cell cycle, extracellular matrix organization, and cell-substrate adhesion were mainly enriched in biological process; actin binding, microtubule binding and cell-cell adhesion mediator activity were mainly enriched molecular function; cell-cell junction, endoplasmic reticulum lumen, and actin cytoskeleton were main enriched cellular component (Figure 2D-2F).
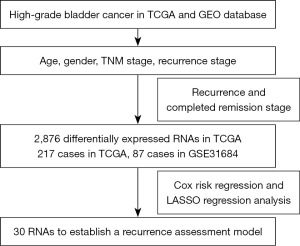
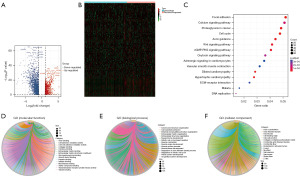
Cox risk regression and LASSO regression analysis
At last, 284 RNAs related to bladder cancer recurrence were obtained (P<0.05) in Cox risk regression (table available at https://cdn.amegroups.cn/static/public/tcr-24-256-2.xlsx). Some 49 from 284 redundant RNAs were obtained in LASSO regression analysis with minimum λ of 0.061. We performed multifactor risk analysis and obtained 30 recurrence-associated RNAs (Figure 3A). We used these 30 RNAs to establish a RFS model for bladder cancer. The risk score in the model for each patient was calculated based on expression levels of these RNAs and weighted by their regression coefficients (Table S1). This risk model presents a higher ability than gender, stage, and age in predicting recurrence of high-grade bladder cancer (Figure 3B).
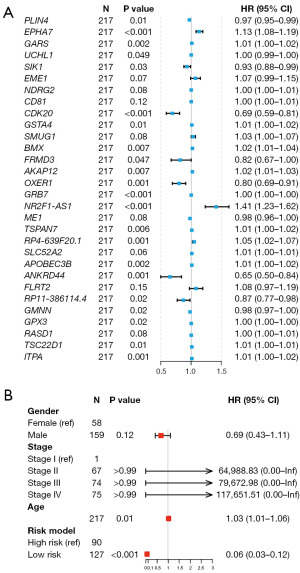
Prognostic analysis of the recurrence risk model
A total of 30 RNAs were used to construct an assessment model for recurrence prediction of high-grade bladder cancer. The results showed that the prediction model obvious distinguish disease-free or recurrence status in TCGA, with an AUC of 0.911, sensitivity of 0.81 and specificity of 0.89, compared to stage with an AUC of 0.641 (Figure 4A). The prediction ability to differentiate disease-free or recurrence status in GSE31684 was low, with an AUC of only 0.533. However, these selected RNAs can construct an adjusted prediction model with an AUC of 0.837 (Figure 4B), whereas tumor stage in prediction of recurrence can reach an AUC of 0.694 in TCGA and 0.617 in GSE31684.
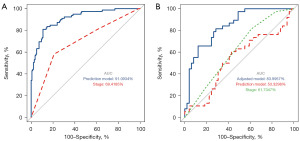
Evaluation and validation for recurrence risk model
The maximum Youden as a cutoff point was used to divide the included patients into high- and low-risk groups for the training set and the verification set. As shown in Figure 5A, the AUC of the prediction model for 3-year recurrence of bladder cancer in TCGA was 0.95. To further evaluate the predictive power of the model risk score, we confirmed its ability in GSE31684 with an AUC of 0.63 for 3-year recurrence (Figure 5B). The prognosis model predicted obvious lower disease-free recurrence time in the high-risk group in the training set (Figure 5C) and the validation set (Figure 5D).
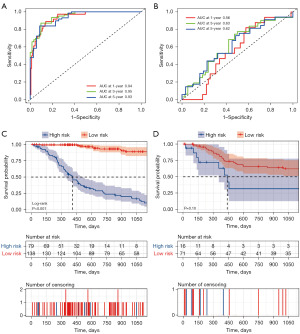
Functional prediction of model genes and efficacy evaluation of adjuvant therapy
We conducted pathway prediction on the included genes of the risk model, and the results showed that these genes were mainly enriched in the glutathione metabolism and peroxisome proliferators-activated receptor (PPAR) signaling pathway (Figure 6A). We used the oncoPredict R package to predict drug sensitivity in the two groups of the risk model, and found that the high-risk group had no difference in sensitivity to traditional chemotherapy drugs such as gemcitabine, paclitaxel, and carboplatin compared to the low-risk group. However, the high-risk group may be more sensitive to chemotherapy with ifosfamide (Figure 6B). At the same time, we analyzed the immune responses of the two groups and found no difference in the expression of immune genes programmed cell death protein ligand 1 (PD-L1) and cytotoxic T-lymphocyte-associated protein 4 (CTLA-4), and there was no difference in immune scores, but the high-risk group had a higher immune response rate (Figure 6C). In addition, we found that the target genes of the PPAR signaling pathway were dysregulated. Poly ADP-ribose polymerase 2 (PARP2) was highly expressed in the high-risk group, whereas the PARP3 gene was lowly expressed in the high-risk group, and PARP1 gene was of no significant difference between these two groups, indicating that the high-risk group might benefit from PARP inhibitors. Besides, antibody-drug conjugate (ADC) of human epidermal growth factor receptor 2 (HER2) revealed no significant differences between the two groups, but the overall expression level of HER2 was higher in the high-risk group (Figure 6D), suggesting that the high-risk group may benefit from HER2 ADC drugs.
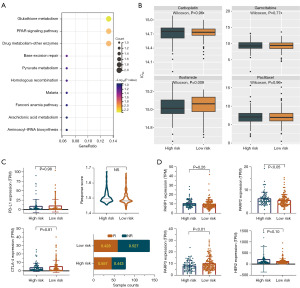
Discussion
In our study, we analyzed the significantly dysregulated RNAs in the TCGA database, and used LASSO Cox regression to construct a risk assessment model to predict the recurrence for bladder cancer. Through the training set in the TCGA database and validation sets in the GEO database, we found that the risk model was accurate in recurrence prediction, and it was more accurate than the gender, age, and stage model. From function prediction of genes in the risk model, we found that the high-risk group of the risk model may benefit from chemotherapy of ifosfamide, immunotherapy, target drug of PARP inhibitor, and HER2-ADC drugs.
Although a statistically significant difference is vital to obtain more reliable results, a high level of criteria limits the study sample size (10). Thus, we set a moderate level of criteria with P<0.05 and |log2(fold change)| >1 for difference selection. Differentially expressed genes present significant function in disease progression (11), so we chose differentially expressed RNAs from tumor tissues and normal tissues rather than directly comparing samples from recurrence and disease-free patients. Our study firstly analyzed differently expressed RNAs in the TCGA data rather than GEO data or other previous studies to construct a risk assessment model to predict tumor recurrence; because TCGA included a sufficient number of patients with bladder cancer, we also selected GSE31684 with 93 patients to construct a model with high reliability. We obtained the risk assessment model with 30 RNAs through Cox and LASSO regression, which presented an AUC of 0.911 in TCGA and 0.533 in GSE31684. However, with the same RNAs, the adjusted risk model reached an AUC of 0.839. We speculated that the differences in diagnostic efficacy between databases were mainly related to the differences in data results caused by detection methods. Even though the original AUC of the validation set GSE31684 was low, the diagnostic model with adjusted coefficients still had high diagnostic effectiveness. This indicated that the screening results had a good ability to distinguish disease status.
Tumor prognosis is often related to stage (12,13). Bladder cancer with later stage is more likely to recur, but the prognosis prediction accuracy of stage has been limited (5). Besides stage, other clinical information as well as parts of RNA detection have been reported as effective tools for predicting recurrence of bladder cancer (14-16). However, these assessment tools or models have also had their limitations in sample sizes or efficiency. Our research based on TCGA and GEO databases showed that the AUC of stage prediction for high-risk bladder cancer was only 0.69. In contrast, the AUC of tumor model prediction of 3-year no-recurrence could reach 0.95, and the RFS rate of low-risk group was significantly lower than that of high-risk group. We also found that the median RFS time of the high-risk group was less than 1.5 years, so accurate grouping and early intervention are extremely important for prognosis.
The first-line treatment for advanced bladder cancer is chemotherapy (17), the main drugs of which are platinum combined with gemcitabine or paclitaxel (18). However, through chemotherapy sensitivity prediction, we found there was no difference in the chemosensitivity of the high- and low-risk groups to platinum, paclitaxel, and gemcitabine, whereas the high-risk group was more sensitive to ifosfamide, a second-line chemotherapy drug for advanced bladder cancer (19), suggesting that ifosfamide may benefit high-risk group. According to the pathway analysis of the included risk-related genes, we found that the PARP pathway was the main enrichment pathway, and PARP inhibitors were also targeted drugs for bladder cancer. Research has shown that combination treatment with PARP inhibitors to MIBC reached 50% of pathological CR rate (20). We found that PARP2, a main target of PARP, was significantly overexpressed in the high-risk group, indicating that the high-risk group may be more limited in the targeted therapeutic effect of PARP inhibitors. Immunotherapy has also represented a main treatment for advanced bladder cancer (21). We analyzed the immune score, immune response, and the expression of commonly used immunosuppressive targets in different groups. The results showed that although there was no difference in immune scores between the two groups, and there was no difference in the expression levels of PD-L1 and CTLA-4, but the high-risk group had a higher proportion of immune responses, which also suggested that the high-risk group would benefit more from immunotherapy. A previous study showed that ADC drugs were an important means of treatment for advanced bladder cancer. The objective response rate of an ADC drug of HER2 for advanced bladder cancer was 51.2%, the disease control rate was 90.7%, and the adverse reactions were mild (22). We found that the expression level of HER2 was higher in the high-risk group, although there was no statistically significant difference between the two groups, which suggested that the high-risk group might benefit more from HER2-ADC drugs.
There were some limitations in our studies. Although datasets GSE5479, GSE57933, and GSE120736 were also associated with recurrence of bladder cancer, they had no clinical information about recurrence or had small sample sizes, thus they were excluded for validation. The included GSE31684 dataset contained incompletely inconsistent clinical information with TCGA, lymph metastasis or distal metastasis were not detailed in this dataset, thus more detailed analysis was unable to proceed. Unlike an accuracy serum prediction model, our model, constructed from solid tumor data, was obviously high in the TCGA database but slightly less effective in the GEO database, but with promotion and popularization of accurate genetic sequencing, the recurrence risk assessment model would present a significant value in clinical practice.
Conclusions
The recurrence risk assessment model is accurate in predicting recurrence of high-grade bladder cancer and can provide guidance for treatment of bladder cancer.
Acknowledgments
Funding: None.
Footnote
Reporting Checklist: The authors have completed the TRIPOD reporting checklist. Available at https://tcr.amegroups.com/article/view/10.21037/tcr-24-256/rc
Peer Review File: Available at https://tcr.amegroups.com/article/view/10.21037/tcr-24-256/prf
Conflicts of Interest: All authors have completed the ICMJE uniform disclosure form (available at https://tcr.amegroups.com/article/view/10.21037/tcr-24-256/coif). The authors have no conflicts of interest to declare.
Ethical Statement: The authors are accountable for all aspects of the work in ensuring that questions related to the accuracy or integrity of any part of the work are appropriately investigated and resolved. The study was conducted in accordance with the Declaration of Helsinki (as revised in 2013).
Open Access Statement: This is an Open Access article distributed in accordance with the Creative Commons Attribution-NonCommercial-NoDerivs 4.0 International License (CC BY-NC-ND 4.0), which permits the non-commercial replication and distribution of the article with the strict proviso that no changes or edits are made and the original work is properly cited (including links to both the formal publication through the relevant DOI and the license). See: https://creativecommons.org/licenses/by-nc-nd/4.0/.
References
- Siegel RL, Miller KD, Wagle NS, et al. Cancer statistics, 2023. CA Cancer J Clin 2023;73:17-48. [Crossref] [PubMed]
- Kim KH, Lee HW, Ha HK, et al. Perioperative systemic therapy in muscle invasive bladder cancer: Current standard method, biomarkers and emerging strategies. Investig Clin Urol 2023;64:202-18. [Crossref] [PubMed]
- Tang Z, Can L, Xuan S, et al. Unveiling the Etiology of Urological Tumors: A Systematic Review of Mendelian Randomization Applications in Renal Cell Carcinoma, Bladder Cancer, and Prostate Cancer. Urol J 2024; Epub ahead of print. [Crossref] [PubMed]
- Schuettfort VM, D'Andrea D, Quhal F, et al. A panel of systemic inflammatory response biomarkers for outcome prediction in patients treated with radical cystectomy for urothelial carcinoma. BJU Int 2022;129:182-93. [Crossref] [PubMed]
- Zhao H, Chen Z, Fang Y, et al. Prediction of Prognosis and Recurrence of Bladder Cancer by ECM-Related Genes. J Immunol Res 2022;2022:1793005. [Crossref] [PubMed]
- Lucas M, Jansen I, van Leeuwen TG, et al. Deep Learning-based Recurrence Prediction in Patients with Non-muscle-invasive Bladder Cancer. Eur Urol Focus 2022;8:165-72. [Crossref] [PubMed]
- Bian Z, Chen J, Liu C, et al. Landscape of the intratumroal microenvironment in bladder cancer: Implications for prognosis and immunotherapy. Comput Struct Biotechnol J 2022;21:74-85. [Crossref] [PubMed]
- Shi H, Yuan X, Liu G, et al. Identifying and Validating GSTM5 as an Immunogenic Gene in Diabetic Foot Ulcer Using Bioinformatics and Machine Learning. J Inflamm Res 2023;16:6241-56. [Crossref] [PubMed]
- Maeser D, Gruener RF, Huang RS. oncoPredict: an R package for predicting in vivo or cancer patient drug response and biomarkers from cell line screening data. Brief Bioinform 2021;22:bbab260. [Crossref] [PubMed]
- Zong L, Zhu Y, Jiang Y, et al. A comprehensive assessment of exome capture methods for RNA sequencing of formalin-fixed and paraffin-embedded samples. BMC Genomics 2023;24:777. [Crossref] [PubMed]
- Goel A, Ward DG, Noyvert B, et al. Combined exome and transcriptome sequencing of non-muscle-invasive bladder cancer: associations between genomic changes, expression subtypes, and clinical outcomes. Genome Med 2022;14:59. [Crossref] [PubMed]
- Secher MS, Hyldgaard J, Jensen JB. The association between gender, stage and prognosis in bladder cancer patients undergoing radical cystectomy. Scand J Urol 2023;57:10-4. [Crossref] [PubMed]
- Zeng H, Wang Q, Xiang Y, et al. PLK3 is linked with higher tumor stage and unfavorable prognosis in patients with colorectal cancer. Biomark Med 2024;18:221-30. [Crossref] [PubMed]
- Varchulova Novakova Z, Harsanyi S, Bevizova K, et al. Expression of BCL2, TP53, FOXA1, and GATA3 in pTa bladder cancer recurrence. Bratisl Lek Listy 2024;125:311-7. [Crossref] [PubMed]
- Lv H, Zhou X, Liu Y, et al. Feasibility analysis of arterial CT radiomics model to predict the risk of local and metastatic recurrence after radical cystectomy for bladder cancer. Discov Oncol 2024;15:40. [Crossref] [PubMed]
- Wu Z, Wang D, Zhang Y, et al. SPP1 mRNA determination based on molecular beacon for the recurrence prognosis of bladder cancer. Transl Androl Urol 2023;12:1834-44. [Crossref] [PubMed]
- Park I, Lee JL. Systemic treatment for advanced urothelial cancer: an update on recent clinical trials and current treatment options. Korean J Intern Med 2020;35:834-53. [Crossref] [PubMed]
- Harada M, Tomisaki I, Minato A, et al. Combination therapy with paclitaxel and gemcitabine after platinum-based chemotherapy in patients with advanced urothelial cancer. Int J Urol 2021;28:970-4. [Crossref] [PubMed]
- Hosomi T, Shibasaki N, Otsuka H, et al. Advanced Bladder Cancer with Multiple Pulmonary Metastases Treated with Paclitaxel/Ifosfamide/Nedaplatin Therapy: Two Case Reports. Hinyokika Kiyo 2023;69:183-8. [PubMed]
- Bhattacharjee S, Sullivan MJ, Wynn RR, et al. PARP inhibitors chemopotentiate and synergize with cisplatin to inhibit bladder cancer cell survival and tumor growth. BMC Cancer 2022;22:312. [Crossref] [PubMed]
- Ren X, Tian Y, Wang Z, et al. Tislelizumab in combination with gemcitabine plus cisplatin chemotherapy as first-line adjuvant treatment for locally advanced or metastatic bladder cancer: a retrospective study. BMC Urol 2022;22:128. [Crossref] [PubMed]
- Wen F, Lin T, Zhang P, et al. RC48-ADC combined with tislelizumab as neoadjuvant treatment in patients with HER2-positive locally advanced muscle-invasive urothelial bladder cancer: a multi-center phase Ib/II study (HOPE-03). Front Oncol 2024;13:1233196. [Crossref] [PubMed]