TGF-β signaling-related signature for predicting prognosis and therapeutic response in lower-grade glioma
Highlight box
Key findings
• Low-grade glioma (LGG) was divided into three subtypes by the analysis of transforming growth factor-beta (TGF-β) signal-related genes, revealing the molecular subtype diversity of LGG.
• Studies have found associations between different TGF-β subtypes and clinical features, tumor microenvironment (TME), metabolic reprogramming, somatic mutations, and drug sensitivity, providing new ideas for individualized treatment of LGG.
What is known and what is new?
• Through the analysis of the relationship between TGF-β subtypes and immune cell infiltration, metabolic environment, and clinical prognosis, the important role of TGF-β signaling in tumor immune resistance and metabolic reprogramming was revealed.
• A risk model was constructed based on related genes of TGF-β subtypes, which was able to predict the prognosis of LGG patients and provides a reference for clinical decision making.
What is the implication, and what should change now?
• This study provides a basis for further investigation of the relationship of TGF-β subtypes with immune infiltration and metabolic mechanisms, and contributes to a deeper understanding of the pathogenesis of LGG and the development of individualized therapeutic strategies.
Introduction
Low-grade gliomas (LGGs), encompassing World Health Organization (WHO) grade II and III gliomas such as anaplastic oligodendrogliomas, anaplastic astrocytomas, and anaplastic oligoastrocytomas, derive from precursor and glial cells (1). LGG is further classified into the following subtypes based on the isocitrate dehydrogenase (IDH) mutation and 1p19q status (2,3). The major treatment strategies include surgical resection, chemotherapy, and radiotherapy according to pathological characteristics. However, tumor invasiveness and drug resistance may lead to tumor progression (4,5). Therefore, there is an urgent need for new therapeutic approaches. In recent years, immunotherapeutic strategies have achieved significant success across various types of cancer. However, the efficacy of immunotherapy including anti-programmed cell death ligand 1 (PD-L1) immune checkpoint blockade (ICB) and vaccine immunotherapy against glioma-associated antigens in treating gliomas has been constrained by several challenges, including tumor heterogeneity and the immunologically ‘cold’ tumor microenvironment (TME) (6). Therefore, regulating the LGG TME to enhance its sensitivity to immunotherapy is a critical issue that urgently needs to be addressed.
Transforming growth factor-beta (TGF-β) is a multifunctional cytokine that regulates various cellular processes such as proliferation, differentiation, angiogenesis, immune evasion, and epithelial-mesenchymal transition (EMT). It is secreted by different types of cells, including immune cells, tumor cells, and stromal cells (7). Earlier research has shown that cancers exhibit dysregulation in the TGF-β signature. As a result, the initiation and progress of glioma are facilitated by this abnormal pathway through its impact on the maintenance of the stemness of glioma stem cells (GSCs) and their cell proliferation, angiogenesis, tumor invasion, and immunosuppression (8-11). A glioma tissue-based study on humans indicated that TGF-β plays a role in glioma development since it is upregulated in tumors but not in normal brain tissues. Furthermore, elevated TGF-β/Smad signaling triggers the generation of platelet-derived growth factor-B (PDGF-B), thus promoting tumor cell proliferation (12). The promotion of matrix metalloproteinase (MMP) expression and the inhibition of tissue inhibitors of metalloproteinase (TIMP) are also induced by the TGF-β pathway. This ultimately leads to the invasion and migration of glioma cells (13). Furthermore, the TGF-β pathway may inhibit the expression of human leukocyte antigen-DR isotype (HLA-DR) antigen, Fas ligand, interferon (IFN)-γ, granzyme A, and perforin, rendering glioma an immunosuppressive TME (14). Currently, due to the development of gene detection technology and statistical algorithms, an increasing number of gene signals are being calculated to predict the clinical prognosis and TME characteristics of various tumors. However, few studies have systematically and comprehensively explored the clinical significance of TGF-β-related genes in gliomas. Furthermore, several studies have demonstrated lackluster responses to TGF-β-based therapies in various cancers, including gliomas, underscoring a critical issue that demands attention (15-17). The current in-silico analysis might offer a foundation for improving our understanding of LGG subtypes and developing more focused therapeutic strategies.
In this study, we explored the classification of the expression of TGF-β-related genes in LGG. We found that LGG can be categorized into three different types of tumors based on genes related to TGF-β. TGF-β subtypes were analyzed for differences in TME, clinical relevance, drug sensitivity, and somatic mutation. An improved performance in prognostic prediction was achieved through the development of a risk model based on the three subtypes of LGG. These findings may provide information for accurate prognostic assessment as well as stratified and individualized treatment of patients with LGG. We present this article in accordance with the TRIPOD reporting checklist (available at https://tcr.amegroups.com/article/view/10.21037/tcr-24-144/rc).
Methods
Data preparation
The Cancer Genome Atlas (TCGA; http://cancergenome.nih.gov/) and Chinese Glioma Genome Atlas (CGGA; http://www.cgga.org.cn, mRNAseq_693, and mRNAseq_325) datasets were used to download gene expression profile and corresponding clinical information for LGG (18,19). The CGGA RNA sequencing (RNA-seq) datasets were merged and given the name CGGA. The University of California, Santa Cruz (UCSC) website was used to extract gene expression data of normal brain tissues from the Genotype-Tissue Expression (GTEx) dataset (http://xena.ucsc.edu/) (20). Moreover, the Gene Set Enrichment Analysis (GSEA) database (http://www.gsea-msigdb.org/gsea/index.jsp) provided identification of 223 genes associated with TGF-β. RNA-seq is a powerful method for analyzing transcriptomes quantitatively. Here are the key aspects to consider when quantifying RNA-seq data and the common methods of normalization. Library preparation: RNA samples are converted into a library of complementary DNA (cDNA) fragments with adapters attached to each end. The choice of library preparation method (e.g., poly-A selection, ribosomal RNA depletion) can affect which RNA species are sequenced. Sequencing: this step involves sequencing the cDNA fragments to generate raw sequence reads. Sequencing depth (i.e., the number of reads) is crucial for quantification, as it impacts the ability to detect and accurately quantify low-abundance transcripts. Mapping reads: the raw reads are aligned to a reference genome or transcriptome. The efficiency of this step depends on the quality of the reads and the reference sequence used. Counting: once reads are mapped, counts are generated for each gene or transcript, representing the number of reads or fragments aligned. This study was conducted in accordance with the Declaration of Helsinki (as revised in 2013).
There were several methods of normalization as follows. Transcripts per million (TPM): normalizes for total library size and gene length, making it useful for comparing transcript levels within samples (21). Reads/fragments per kilobase of transcript per million mapped reads (RPKM/FPKM): similar to TPM but less suitable for comparing across samples because it does not account for differences in sequencing depth and library composition between samples. Trimmed mean of M-values (TMM): used in edgeR, it normalizes based on the assumption that most genes are not differentially expressed. It adjusts for differences in library composition. Quantile normalization: This method assumes the same distribution of gene expression across samples and adjusts the data accordingly. DESeq’s size factor normalization: used in DESeq, this method estimates size factors based on the median ratio of gene counts relative to a geometric mean per gene (thus stabilizing variance across samples). The data used in this article were extracted from public databases, so quantification of RNA seq is not involved. This study used packages such as DESeq2 and Limma to normalize expression levels between different samples, eliminating the impact of experimental conditions and instrument differences on the data, and correcting systematic errors between different experimental batches.
Identification of genes related to TGF-β that are differential and prognostic
The “Bioconductor Limma” R package was utilized to evaluate the expression of TGF-β genes in normal and glioma tissues, resulting in the identification of differentially expressed genes (DEGs). Genes were considered differentially expressed if they met the criteria of having a false discovery rate (FDR) <0.05 and a fold change >1 (22). Next, the association between the differential genes and the overall survival of patients with LGG was assessed using the “survival” R package. A gene that had a P value less than 0.05 was identified as being a prognostic gene.
Construction and validation of TGF-β subtypes
Consensus clustering was performed using the R package “consensusClusterplus” with the differentially expressed and prognostic TGF-β-related genes (23). ConsensusClusterPlus processes a numerical data matrix, organizing items in columns and features in rows. This function selectively subsamples the matrix based on pItem, pFeature, weightsItem, and weightsFeature, and partitions the data into clusters ranging from 2 to maxK using the specified clustering algorithm in clusterArg. It supports both agglomerative hierarchical clustering (hclust) and k-means clustering, with additional configurations available as described above (24). The consensus heatmap and cumulative distribution function were used to identify the optimal k. The TCGA database served as the training cohort, whereas the CGGA was designated as the validation cohort. One-sided analysis of variance (ANOVA) was used to evaluate the association between TGF-β subtypes and clinical characteristics. In the presented manuscript, we assessed the association between TGF-β and the TCGA subtype. TCGA Sub1 was defined as IDH1 mutation and 1p19q codeletion, Sub2 was defined as IDH1 mutation and 1p19q no codeletion, whereas Sub3 was defined as IDH1 wild type, no matter the status of 1p19q.
Estimation of immune cell infiltration
The R package “GSVA” was utilized to execute single-sample GSEA (ssGSEA) for assessing the infiltration level of 23 immune cells (25). Gene set variation analysis (GSVA) is a non-parametric, unsupervised method used for estimating variations in gene set activity across samples in genomic datasets. When calculating metrics with GSVA, a collection of gene sets is first defined. GSVA then assesses the variation in expression levels of these gene sets within individual samples to calculate an enrichment score for each gene set. This approach is applicable to RNA-seq and microarray data, enabling researchers to globally understand changes in biological processes or signaling pathways under various conditions. The outcomes of GSVA can be utilized for further differential expression analysis or as candidates for biomarkers. The Estimation of STromal and Immune cells in MAlignant Tumor tissues using Expression data (ESTIMATE) algorithm was utilized to estimate the tumor purity, stromal score, and immune score (26). Furthermore, we employed the R package “MCPcounter” to determine the absolute abundance of eight immune cells and two stromal cells (27). It is worth noting that transcriptome data must be normalized. TIMER2.0 (http://cistrome.org/) is an online tool to calculate tumor immune cell infiltration, and we used it to assess the immune cell infiltration of sample in TCGA and CGGA cohorts, respectively (28).
Analysis of T-cell dysfunction and exclusion
The Tumor Immune Dysfunction and Exclusion (TIDE) online algorithm (http://tide.dfci.harvard.edu/) was utilized to predict the response of ICB therapy by assessment of T-cell dysfunction, exclusion, and TIDE. The response of each TGF-β subtype to ICB therapy was evaluated.
Calculation of gene signatures associated with metabolism
From previous studies, a set of 115 signatures related to metabolism were detected (29). The R package “GSVA” was used to calculate the scores for the 115 metabolism-associated signatures in each sample (25).
Construction and validation of the risk model
First, we applied the R packages “samr” and “Veen” to identify DEGs among TGF-β subtypes. Second, the R package “glmnet” was employed to construct a Cox proportional hazards model based on the intersection of DEGs and prognostic genes. The risk score of each sample was estimated using the regression coefficients (30,31). The risk score is equal to the SHD × (−0.24) + AC062021.1 × (−0.21) + SNCG × (−0.13). The training cohort was the TCGA cohort and the validation cohort was the CGGA cohort.
Potential compound prediction
The R package “pRRophetic” was used to evaluate the drug sensitivity of every TGF-β subtype. Data on drug sensitivity were downloaded from the Profiling Relative Inhibition Simultaneously in Mixtures (PRISM) platform. By utilizing the Genomics of Drug Sensitivity in Cancer (GDSC) database, the half-maximal inhibitory concentration (IC50) and prediction accuracy were achieved through ridge regression and 10-fold cross-validation (32,33).
Single-cell RNA analysis
We downloaded two single-cell datasets including GSE70630 and GSE89567 from the Gene Expression Omnibus (GEO) database (34,35). The former contains 4,347 single cells from six LGG samples, whereas the latter contains a total of 6,341 single cells from eight LGG and two GBM samples. Single-cell data analysis was performed using the Seurat R package. Initially, 2,000 highly DEGs were identified through the “findvariablefeatures” function. Following normalization of the genes, a principal component analysis (PCA) dimensionality reduction was performed, and the cells were clustered using the first 20 dimensions through the “findneighbors” and “findclusters” functions. The resolution parameter was set to 0.65, and map visualization was then performed. The classic marker genes collected from the literature were used to define the cell subpopulations (36,37).
Somatic mutation and copy number alteration (CNA) analysis
Mutation data and copy number variation (CNV) data were obtained from cBioPortal (https://www.cbioportal.org/). The Genomic Identification of Significant Targets in Cancer (GISTIC) algorithm was used to assess the CNV. We defined −1 and −2 as copy number loss, 1 and 2 were defined as copy number amplifications, and 0 was used to define the absence of a CNV event. The information from the 1p19q codeletion was extracted directly from the clinical information provided by the cBioPortal website The onco-print tool of the “complexheatmap package” was employed to visualize the somatic mutation and CNA among TGF clusters. The calculation method for tumor mutation burden (TMB) was based on the number of non-silent mutations (26,38-40).
Statistical analysis
R software (version 4.1.0; R Foundation for Statistical Computing, Vienna, Austria) was used for all analyses in this study. The evaluation of the survival difference between the TGF cluster and risk score was conducted through a log-rank test, using Kaplan-Meier analysis. The chi-square test was used to investigate the distinctions in molecular and clinical aspects among the subtypes or risk groups. The three groups were compared using one-way ANOVA. Univariate and multivariate Cox regression analyses were utilized to assess whether the TGF cluster and risk score were predictive factors.
Results
Screening of differential and prognostic TGF-β-related genes
TCGA and GTEx datasets were used to identify differentially expressed TGF-β-related genes. A total of 20 differentially expressed TGF-β-related genes (BMP2, CCNK, CDKN2B, COL3A1, INHBB, LEFTY1, MSTN, MYC, NOG, NRROS, PRKCZ, RBL1, SERPINE1, SMAD9, TGFBR3L, TGIF2, THBS2, TNF, TP53, and USP9Y) were identified between 531 LGGs and 1,151 normal brain tissue samples (Figure 1A,1B). Among the 223 TGF-β-related genes, 131 were associated with LGG prognosis, whereas 12 (BMP2, COL3A1, INHBB, MYC, NOG, PRKCZ, SERPINE1, SMAD9, TGIF2, THBS2, TNF, and TP53) were differentially expressed and prognostic TGF-β-related genes (Figure 1C and Figure S1). DNA mutations in these 12 genes occurred in approximately 46.05% of patients with LGG (Figure 1D-1G). Consensus clusters were performed using these 12 genes. We then assessed the correlation of these genes with immune checkpoints (ICPs) including programmed cell death protein 1 (PD-1), PD-L1, and TIGIT. Our results revealed that SERPINE1 is positively correlated with the expression of PD-L1 and PD-1, whereas NOG and BMP2 are negatively correlated with PD-L1. The remaining correlations were not significant (Figure S2).
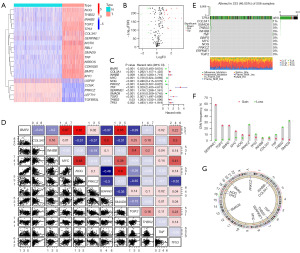
Consensus cluster was performed to obtain three TGF-β subtypes
An unsupervised cluster analysis was performed, which obtained three TGF-β subtypes (A, B, and C) based on the 12 DEGs and prognostic TGF-β-related genes located from the TCGA dataset (Figure S3A-S3L). PCA was employed to assess the resilient disparity in expression properties among the three TGF-β subtypes. In the training cohort, subtype B was linked with the least favorable prognosis, whereas subtype A was linked with the most favorable prognosis (Figure 2A-2C). These results were similarly obtained from the CGGA database (Figure 2D-2F).
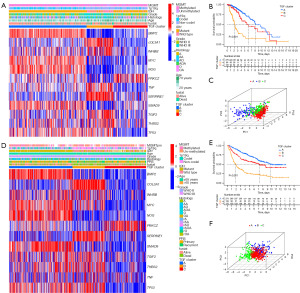
Clinical features of the TGF-β subtypes
The determination of the relationship between molecular and pathological features was necessary to assess the clinical relevance of the identified TGF-β subtypes. Subtype B was found to be enriched with anaplastic astrocytoma, IDH wild type, 1p19q no codeletion, MGMTp unmethylation, and WHO grade III. Meanwhile, subtype A was associated with WHO grade II, IDH mutation, and 1p19q codeletion (Figure 2A-2C). Similar clinical correlations, indicating that different TGF-β subtypes have different malignant clinical characteristics, were also obtained from the CGGA database (Figure 2D-2F).
Immune landscape and potential therapeutic value of TGF-β subtypes
TGF-β signaling influences immunity and is related to the immunosuppressive microenvironment. Therefore, we evaluated the immune infiltration of each TGF-β subtype through ssGSEA, MCPcounter, and TIMER, and identified that subtype B was highly enriched with most immune cells (Figure 3A). The immune, stromal, and ESTIMATE scores demonstrated a higher level for subtype B in comparison to subtypes A and C. Additionally, there was an increase in the immune and stromal scores for subtype B. However, the tumor purity was lower in subtype B than in both subtypes A and C (Figure 3B-3E). In comparison to subtype C, subtype A exhibited elevated immune, stomal, and ESTIMATE scores, but had lower tumor purity. These data were further verified using the CGGA database, which also showed similar results (Figure S4). Furthermore, GSVA indicated that primary immunodeficiency, the intestinal immune network for LGG production, and leukocyte transendothelial migration were enriched in subtype B when compared with subtypes A or C (Figure S5). In the present study, the TIDE online algorithm was employed to predict the response of ICP therapy of LGG patients of each TGF-β subtype. We observed that subtype B may benefit from immunotherapy (Figure 4).
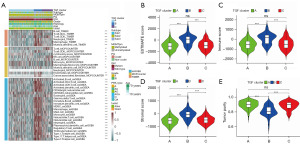
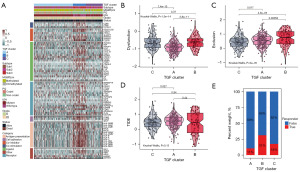
We investigated the correlation between drug sensitivity and the TGF-β subtype in order to determine its potential as a therapeutic option. We observed that AZD6244, bexarotene, bryostatin 1, and rapamycin had a high sensitivity for subtype A. However, the highest drug sensitivity of BIRB.0796, BMS.708163, BMS.754807, and vorinostat was identified in subtype B. Finally, cisplatin, bleomycin, BAY.61.3606, and AZ628 showed the highest sensitivity in subtype C (Figure S6).
Metabolic characteristics of TGF-β subtypes
Metabolic alterations are linked to the TGF-β pathway and the progression of tumors. A calculation of the 115 metabolic characteristics for each sample was conducive to exploring each TGF-β subtype-related metabolic characteristic. The results of differential analyses showed that subtype B was linked with a higher number of metabolic processes, particularly related to carbohydrate, vitamin, and nitrogen metabolism as compared to subtypes A and C. However, subtype C was associated with more metabolic processes related to lipid and amino acid metabolism but was less associated with nucleotide metabolism, whereas subtype A was associated with relatively low tumor metabolism (Figure S7). Similar results were obtained after calculating the metabolic scores in the CGGA cohort.
TGF-β subtypes are associated with diverse somatic variations
The potential somatic variations in TGF-β subtypes were examined, and the highest gene frequency of mutation or in critical pathways and CNAs were determined. Subtype A was characterized by a high frequency of 1p19q codeletion and IDH1, CIC, ATRX, TP53, and FUBP1 mutations. Conversely, subtype B was enriched in CIC, MET, and EGFR amplifications, CDKN2A, CDKN2B, RB1, and PTEN codeletion, and PTEN mutations. Finally, subtype C was enriched in IDH1, ATRX, and TP53 mutations (Figure 5A). Moreover, subtype B showed a high number of segments, high aneuploidy score, and high copy number burden and an increased TMB (Figure 5B-5F).
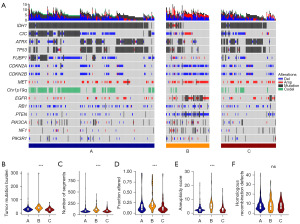
Identification of the DEGs and prognostic genes among TGF-β subtypes
Analysis was performed on the DEGs in the TGF-β subtypes (A vs. B, A vs. C, and B vs. C) present in both TCGA and CGGA datasets. In the TCGA dataset, differential expression analysis revealed 995, 890, and 954 distinct genes in the A vs. B, A vs. C, and B vs. C comparisons, respectively. In the CGGA dataset, the numbers of DEGs were 164, 1,705, and 820 in the A vs. B, A vs. C, and B vs. C comparisons, respectively. Following that, the TCGA and CGGA cohorts led to the identification of three genes that intersected: SHA, AC062021.1, and SNCG (Figure S8A). Both SHA and AC062021.1 were upregulated in LGG, whereas SNCG was downregulated (Figure S8B). Each gene exhibited differential expression among the TGF-β subtypes as well as among the IDH1 and 1p19q status subtypes (Figure S8C,S8D). The survival analysis indicated that all three intersecting genes were associated with prognosis in patients with LGG (Figure S8E-S8J).
Single-cell RNA analysis of DEGs and prognostic genes in tumor or stromal cells
Using human gliomas tissue single-cell datasets, we observed the expression of the abovementioned genes among different cell types. Tumor cells showed high expression levels of SHD, BMP2, and TP53, whereas those of SMAD9 and TNF were enriched in astrocytes and macrophages, respectively. Moreover, PRKCZ and TGIF2 were highly expressed in oligodendrocytes. These results suggest that the abovementioned genes are not population-specific but are derived from the whole tumor and stromal cells (Figure S9).
Calculation of a risk score based on SHD, SNCG, and AC062021.1
A three-gene signature was calculated using regression coefficients (Figure S10). Patients with a high-risk score had shorter overall survival, as indicated by survival analysis (Figure S10). The developed signature was an independent prognostic factor for patients with LGG, as determined by multivariate Cox regression analysis (Figure S11). Upon further investigation, it was found that a high-risk score was more common in anaplastic gliomas, WHO III grade, 1p19q no codeletion, IDH wild type, subtype B TGF-β, Sub2, and Sub3. Moreover, the patients with Sub3, subtype B TGF-β, and WHO grade III had high-risk scores (Figure 6, Figure S12, Tables S1,S2). A nomogram, which is a clinically relevant quantitative method that enables clinicians to predict mortality, was developed for patients with LGG. The nomogram assigns a total score to each patient by adding the scores of each prognostic parameter. The developed signature had a higher area under the curve (AUC) than the factors of age, IDH mutation status, 1p19q codeletion status, and tumor grade. Further validation of this developed signature in the CGGA dataset correlated with a greater consistency of results (Figures 7,8). These data suggest that a risk score based on SHD, SNCG, and AC062021.1 had superior performance in prognosis prediction (Figure 7 and Figure S13).
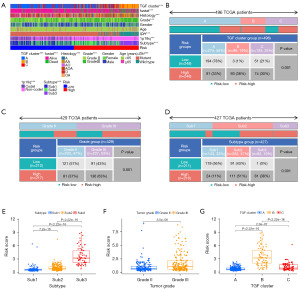
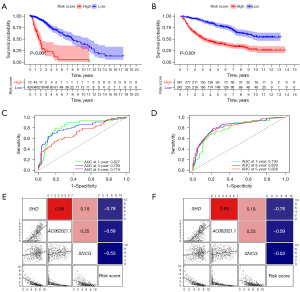
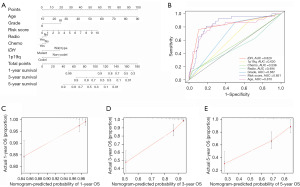
Discussion
The TGF-β signaling pathway has a dual effect on cancer progression, initially impeding tumor growth, but later facilitating it. TGF-β signaling is accountable for regulating the immunosuppressive TME once cancer cells are produced. The presence of inflammatory cells, cytokines, and signal pathways in a microenvironment of inflammation could potentially facilitate the progression of tumors, ultimately resulting in a worse prognosis (19-21). Therefore, exploration of the heterogeneity of TGF-β-related genes in the LGG microenvironment provides important clinical significance, which may yield clues for potential molecular targeting research. In this study, three TGF-β subtypes of LGGs were identified through unsupervised clustering based on TGF-β-related gene expression profiles. Each TGF-β subtype had varying clinical characteristics, immune infiltration, somatic variation, and drug responses. Our research deepens the understanding of TGF-β and its heterogeneity and extends knowledge of the molecular subtypes of LGG.
We systematically examined the differential expression and prognostic roles of TGF-β signaling-related genes in LGG. Most TGF-β-related genes were found to play a crucial role in the advancement of LGG and 131 genes were linked to the prognosis of patients with LGG and 20 genes demonstrated differential expression. There were 12 genes related to TGF-β that presented differential expression and had prognostic significance (BMP2, COL3A1, INHBB, MYC, NOG, PRKCZ, SERPINE1, SMAD9, TGIF2, THBS2, TNF, and TP53). A total of five of the 12 genes were positive factors associated with prognosis (BMP2, MYC, NOG, PRKCZ, and SMAD9), whereas the remaining seven genes were risk factors (COL3A1, INHBB, SERPINE1, TGIF2, THBS2, TNF, and TP53). Indeed, the majority are known to be associated with cancer progression. A previous study has identified BMP2 as an oncogene; however, many conflicting results have been reported depending on the type of cancer, such as lung, breast, and prostate cancers (41). However, few studies have explored the role of BMP2 in glioma; therefore, further experiments should be performed in this area. Moreover, the fibrillar collagen, COL3A1, exists in extensile connective tissues and has previously been reported to possess a malignant phenotype in esophageal squamous cell carcinoma via regulating activation of the NF-κB signaling pathway (42). Indeed, COL3A1 has also been reported to be regulated by GATA-binding protein 6, which promotes propanoate metabolism and a type II IFN response in the recurrence of LGG (43). Furthermore, Myc, a bHLHZip transcription factor, has been implicated in regulating various cancer cellular processes, such as programmed cell death, growth, proliferation, and cell differentiation (44). In addition, the protein-coding gene, INHBB, participates in the synthesis of TGF-β family members and is considered a novel oncogene in various cancer types (45). PRKCZ is a non-typical variant of the serine-threonine protein kinase C, which has a function in controlling cell motility in metastatic clones of pancreatic cancer (46). SERPINE1, an inhibitor of fibrinolysis, plays a role in various malignancies in humans and is part of the signaling cascade involving circular RNA circZNF652/miR-486-5p/SERPINE1 that regulates the aggressiveness of gliomas (47). TGIF2 has been shown to be overexpressed in ovarian cancer and is involved in various other malignancies. In addition, the signal axis composed of TGIF2 and miR-129-5p can regulate the malignant progression of glioma (48). THBS2 represents a disulfide-linked homotrimeric glycoprotein, which mediates the interaction between cells and the cell matrix. Previous reports have indicated that THBS2 is a prognostic biomarker for a variety of human cancers and acts as both a protective and risk factor (49). Finally, p53, which is a primarily studied tumor suppressor gene, has been shown to regulate a series of cell events through transcriptional regulation of a wide range of genes and pathways that form the basis of development and cancer, including DNA damage repair, cell cycle arrest, aging, apoptosis, iron death, autophagy, and metabolic remodeling (50). In this study, LGG was categorized into three separate subtypes utilizing 12 genes that are expressed differentially and have prognostic significance. These genes are BMP2, COL3A1, INHBB, MYC, NOG, PRKCZ, SERPINE1, SMAD9, TGIF2, THBS2, TNF, and TP53. Our study noted that subtype B was linked with shorter overall survival compared to subtypes A or C. Additionally, there were variations in clinical characteristics, TME, metabolism, somatic mutations, and drug sensitivity among each subtype. The discovery expanded the molecular subtyping of diffuse gliomas and enhanced our knowledge of the diversity of TGF-β signaling in this type of tumor.
In the context of tumor immune resistance, local immunosuppressive cytokines are a crucial element, with TGF-β having been identified as an example of an immunosuppressive factor within the TME (51). High infiltration of immune cells was identified as a characteristic of subtype B TGF-β in our study. The infiltration of immune cells was comparably greater in subtype C as compared to subtype A. Subtype C demonstrated elevated immune, stromal, and ESTIMATE scores, whereas subtype A displayed lower scores, accompanied by reduced tumor purity. Although there was an increase in antitumor immune cells including natural killer cells, B cells, and CD8+ T cells in subtype C, these cells were highly susceptible to proto-oncoinflammation. The aforementioned outcome arises due to the expression of different immune mediators and regulators, as well as the diverse activation status of cell types present in the TME (52,53). Indeed, a study on the mechanisms involved in the reversal of this balance through the regulation of TME transformation is important. Such research will allow us to analyze methods to improve the effect of immunotherapy. Furthermore, TGF-β genes exert their effects through M1 to M2 macrophage polarization. Based on our analysis of cellular communication, we found that TGF-related genes primarily regulate the TME, including M1 and M2 macrophages, through the JAM, APP, GRN, PSAP, and MIF signaling pathways. However, the specific mechanisms and the particular genes’ actions on these pathways require further validation through cellular experiments. This may provide evidence for modulating the immune microenvironment in LGG. In the present study, we observed that SERPINE1, a TGF-β gene, is positively correlated with the expression of PD-L1 and PD-1, which suggested that SERPINE1 may be a potential target for enhancing immunotherapy. Future research should further evaluate how SERPINE1 regulates immunotherapy in gliomas.
Certainly, the heterogeneity of the TME in LGGs presents a challenge to current immunotherapy strategies (6). This variability may account for the wide range of treatment responses among patients and could help explain why the results of clinical trials have not met expectations. Current immunotherapies, such as inhibitors of the PD-1/PD-L1 and CTLA-4 pathways, tumor vaccines, immunomodulators, and cell therapies, have shown success in certain types of cancers, but their efficacy in LGGs has been limited (6). This may be partly due to the unique immunosuppressive microenvironment characteristic of LGGs. This environment is typically characterized by a low infiltration of immune cells, which may also exhibit an immunosuppressive phenotype. To enhance therapeutic outcomes, a future research direction may involve a detailed characterization of the cellular components within the LGG TME, including immunosuppressive cells such as regulatory T cells (Tregs), tumor-associated macrophages (TAMs), and myeloid-derived suppressor cells (MDSCs) (54). Another research avenue is to define the molecular pathways within the TME, such as cytokines and chemokines that may regulate immune cell infiltration and function (55). With a better understanding of these cellular and molecular changes, we may identify new therapeutic targets that could be key in modulating the TME and improving response rates in LGG patients. For example, SERPINE1, as a regulator of immune responses and extracellular matrix remodeling, could be a potential target. By targeting SERPINE1 or its related pathways, we might be able to modulate the TME and enhance the efficacy of immunotherapies. More broadly speaking, efforts to improve treatment strategies for LGG will require the use of high-throughput tissue analysis techniques, integrating data from immuno-histochemistry, genomics, transcriptomics, proteomics, and single-cell analyses to build a more comprehensive model of the disease. Through such multidimensional analysis, we can gain a deeper understanding of the biological characteristics of LGG and the potential mechanisms underlying treatment failure, offering more personalized and effective treatment options for patients.
Metabolic reprogramming is a hallmark of cancer (56). Recently, an increasing number of studies have begun to research the role of TGF-β signaling in tumor metabolism, with data indicating that TGF-β acts as a host and cancer metabolic reprogramming cytokine (57,58). This study identified that each TGF-β subtype possesses a different metabolic microenvironment; for example, carbohydrates (particularly glucose), vitamins, nucleotides, and metabolites were enriched in subtype B. However, subtype C displayed a low level of nucleotide metabolism but a high level of metabolic processes associated with lipid and amino acid metabolism. In contrast, subtype A demonstrated relatively low metabolism of the abovementioned metabolic scores. Previous research has indicated that the increase of glycogen synthesis and uncontrolled glycogenolysis are factors in glycogen buildup in cancer cells. This function helps to protect cancer cells from death in low oxygen situations and provides energy in situations where nutrients are limited. This further explains why subtype B TGF-β B produced the worst prognosis. During cancer progression, lipid metabolism reprogramming plays a crucial role in supplying energy, as well as providing biomolecules for membrane synthesis and lipid signals (58,59). TGF-β signaling is mainly connected with cholesterol metabolism, FASN-mediated fatty acid synthesis, and lipid droplets in cancer cells (60,61). Lipid metabolism was enriched in subtype C, which could explain the poor clinical outcome of patients with LGG. Consequently, therapeutic strategies targeting tumor metabolism based on the TGF-β subtype should be the primary focus of future research.
Following identification of an association between the TGF-β subtype with clinical characteristics in the TCGA cohort, we demonstrated that malignant features such as IDH wild type (87.5%) and high-grade glioma (61.5%) were enriched in subtype B. Moreover, we further evaluated the association between the TGF-β subtypes and TCGA subtypes based on IDH mutation status and 1p19q codeletion. A total of 116 (27.0%), 6 (1.4%), and 21 (4.9%) patients classified as Sub1 were assigned to subtypes A, B, and C, respectively. Likewise, 119 (27.7%), 22 (5.1%), and 81 (18.9%) patients classified as Sub2 were assigned to subtypes A, B, and C, respectively. No patients classified as Sub3 were assigned to the A subtype, whereas 56 (13.1%) and 8 (1.9%) were classified into subtypes B and C, respectively. A risk score was constructed from the intersection genes between TGF-β subtypes, and it was found that a high score was significantly linked with malignant characteristics and unfavorable clinical outcomes. Our study has the potential to broaden the understanding of molecular subtyping in LGG.
Conclusions
We divided LGG into three subtypes characterized by varied immune infiltration, metabolism, somatic variations, and prognosis, based on TGF-β signaling-related genes. Further research is required to investigate the immune infiltration and metabolism mechanism related to these TGF-β subtypes.
Acknowledgments
Funding: This study was funded by
Footnote
Reporting Checklist: The authors have completed the TRIPOD reporting checklist. Available at https://tcr.amegroups.com/article/view/10.21037/tcr-24-144/rc
Peer Review File: Available at https://tcr.amegroups.com/article/view/10.21037/tcr-24-144/prf
Conflicts of Interest: All authors have completed the ICMJE uniform disclosure form (available at https://tcr.amegroups.com/article/view/10.21037/tcr-24-144/coif). The authors have no conflicts of interest to declare.
Ethical Statement: The authors are accountable for all aspects of the work in ensuring that questions related to the accuracy or integrity of any part of the work are appropriately investigated and resolved. This study was conducted in accordance with the Declaration of Helsinki (as revised in 2013).
Open Access Statement: This is an Open Access article distributed in accordance with the Creative Commons Attribution-NonCommercial-NoDerivs 4.0 International License (CC BY-NC-ND 4.0), which permits the non-commercial replication and distribution of the article with the strict proviso that no changes or edits are made and the original work is properly cited (including links to both the formal publication through the relevant DOI and the license). See: https://creativecommons.org/licenses/by-nc-nd/4.0/.
References
- Youssef G, Miller JJ. Lower Grade Gliomas. Curr Neurol Neurosci Rep 2020;20:21. [Crossref] [PubMed]
- Louis DN, Perry A, Reifenberger G, et al. The 2016 World Health Organization Classification of Tumors of the Central Nervous System: a summary. Acta Neuropathol 2016;131:803-20. [Crossref] [PubMed]
- Rathore S, Mohan S, Bakas S, et al. Multi-institutional noninvasive in vivo characterization of IDH, 1p/19q, and EGFRvIII in glioma using neuro-Cancer Imaging Phenomics Toolkit (neuro-CaPTk). Neurooncol Adv 2021;2:iv22-34. [Crossref] [PubMed]
- Park JH, Kim HJ, Kim CW, et al. Tumor hypoxia represses γδ T cell-mediated antitumor immunity against brain tumors. Nat Immunol 2021;22:336-46. [Crossref] [PubMed]
- Appolloni I, Alessandrini F, Ceresa D, et al. Progression from low- to high-grade in a glioblastoma model reveals the pivotal role of immunoediting. Cancer Lett 2019;442:213-21. [Crossref] [PubMed]
- Gallus M, Kwok D, Lakshmanachetty S, et al. Immunotherapy Approaches in Isocitrate-Dehydrogenase-Mutant Low-Grade Glioma. Cancers (Basel) 2023;15:3726. [Crossref] [PubMed]
- Danielpour D. Advances and Challenges in Targeting TGF-β Isoforms for Therapeutic Intervention of Cancer: A Mechanism-Based Perspective. Pharmaceuticals (Basel) 2024;17:533. [Crossref] [PubMed]
- Richardson L, Wilcockson SG, Guglielmi L, et al. Context-dependent TGFβ family signalling in cell fate regulation. Nat Rev Mol Cell Biol 2023;24:876-94. [Crossref] [PubMed]
- Wang Q, Xiong F, Wu G, et al. SMAD Proteins in TGF-β Signalling Pathway in Cancer: Regulatory Mechanisms and Clinical Applications. Diagnostics (Basel) 2023;13:2769. [Crossref] [PubMed]
- Ahuja S, Zaheer S. Multifaceted TGF-β signaling, a master regulator: From bench-to-bedside, intricacies, and complexities. Cell Biol Int 2024;48:87-127. [Crossref] [PubMed]
- Wang MY, Liu WJ, Wu LY, et al. The Research Progress in Transforming Growth Factor-β2. Cells 2023;12:2739. [Crossref] [PubMed]
- Bruna A, Darken RS, Rojo F, et al. High TGFbeta-Smad activity confers poor prognosis in glioma patients and promotes cell proliferation depending on the methylation of the PDGF-B gene. Cancer Cell 2007;11:147-60. [Crossref] [PubMed]
- Ye XZ, Xu SL, Xin YH, et al. Tumor-associated microglia/macrophages enhance the invasion of glioma stem-like cells via TGF-β1 signaling pathway. J Immunol 2012;189:444-53. [Crossref] [PubMed]
- Kuburich NA, Sabapathy T, Demestichas BR, et al. Proactive and reactive roles of TGF-β in cancer. Semin Cancer Biol 2023;95:120-39. [Crossref] [PubMed]
- Huang CY, Chung CL, Hu TH, et al. Recent progress in TGF-β inhibitors for cancer therapy. Biomed Pharmacother 2021;134:111046. [Crossref] [PubMed]
- Metropulos AE, Munshi HG, Principe DR. The difficulty in translating the preclinical success of combined TGFβ and immune checkpoint inhibition to clinical trial. EBioMedicine 2022;86:104380. [Crossref] [PubMed]
- Teixeira AF, Ten Dijke P, Zhu HJ. On-Target Anti-TGF-β Therapies Are Not Succeeding in Clinical Cancer Treatments: What Are Remaining Challenges? Front Cell Dev Biol 2020;8:605. [Crossref] [PubMed]
- Hu H, Mu Q, Bao Z, et al. Mutational Landscape of Secondary Glioblastoma Guides MET-Targeted Trial in Brain Tumor. Cell 2018;175:1665-1678.e18. [Crossref] [PubMed]
- Wu F, Wang ZL, Wang KY, et al. Classification of diffuse lower-grade glioma based on immunological profiling. Mol Oncol 2020;14:2081-95. [Crossref] [PubMed]
- The Genotype-Tissue Expression (GTEx) project. Nat Genet 2013;45:580-5. [Crossref] [PubMed]
- Zhao S, Ye Z, Stanton R. Misuse of RPKM or TPM normalization when comparing across samples and sequencing protocols. RNA 2020;26:903-9. [Crossref] [PubMed]
- Ritchie ME, Phipson B, Wu D, et al. limma powers differential expression analyses for RNA-sequencing and microarray studies. Nucleic Acids Res 2015;43:e47. [Crossref] [PubMed]
- Yu G, Wang LG, Han Y, et al. clusterProfiler: an R package for comparing biological themes among gene clusters. OMICS 2012;16:284-7. [Crossref] [PubMed]
- Wilkerson MD, Hayes DN. ConsensusClusterPlus: a class discovery tool with confidence assessments and item tracking. Bioinformatics 2010;26:1572-3. [Crossref] [PubMed]
- Hänzelmann S, Castelo R, Guinney J. GSVA: gene set variation analysis for microarray and RNA-seq data. BMC Bioinformatics 2013;14:7. [Crossref] [PubMed]
- Yoshihara K, Shahmoradgoli M, Martínez E, et al. Inferring tumour purity and stromal and immune cell admixture from expression data. Nat Commun 2013;4:2612. [Crossref] [PubMed]
- Becht E, Giraldo NA, Lacroix L, et al. Estimating the population abundance of tissue-infiltrating immune and stromal cell populations using gene expression. Genome Biol 2016;17:218. [Crossref] [PubMed]
- Li T, Fu J, Zeng Z, et al. TIMER2.0 for analysis of tumor-infiltrating immune cells. Nucleic Acids Res 2020;48:W509-14. [Crossref] [PubMed]
- Yang C, Huang X, Liu Z, et al. Metabolism-associated molecular classification of hepatocellular carcinoma. Mol Oncol 2020;14:896-913. [Crossref] [PubMed]
- van Dijk PC, Jager KJ, Zwinderman AH, et al. The analysis of survival data in nephrology: basic concepts and methods of Cox regression. Kidney Int 2008;74:705-9. [Crossref] [PubMed]
- Tibshirani R. The lasso method for variable selection in the Cox model. Stat Med 1997;16:385-95. [Crossref] [PubMed]
- Yang W, Soares J, Greninger P, et al. Genomics of Drug Sensitivity in Cancer (GDSC): a resource for therapeutic biomarker discovery in cancer cells. Nucleic Acids Res 2013;41:D955-61. [Crossref] [PubMed]
- Geeleher P, Cox N, Huang RS. pRRophetic: an R package for prediction of clinical chemotherapeutic response from tumor gene expression levels. PLoS One 2014;9:e107468. [Crossref] [PubMed]
- Venteicher AS, Tirosh I, Hebert C, et al. Decoupling genetics, lineages, and microenvironment in IDH-mutant gliomas by single-cell RNA-seq. Science 2017;355:eaai8478. [Crossref] [PubMed]
- Tirosh I, Venteicher AS, Hebert C, et al. Single-cell RNA-seq supports a developmental hierarchy in human oligodendroglioma. Nature 2016;539:309-13. [Crossref] [PubMed]
- Zhang X, Lan Y, Xu J, et al. CellMarker: a manually curated resource of cell markers in human and mouse. Nucleic Acids Res 2019;47:D721-8. [Crossref] [PubMed]
- Hao Y, Hao S, Andersen-Nissen E, et al. Integrated analysis of multimodal single-cell data. Cell 2021;184:3573-3587.e29. [Crossref] [PubMed]
- Thorsson V, Gibbs DL, Brown SD, et al. The Immune Landscape of Cancer. Immunity 2019;51:411-2. [Crossref] [PubMed]
- Mermel CH, Schumacher SE, Hill B, et al. GISTIC2.0 facilitates sensitive and confident localization of the targets of focal somatic copy-number alteration in human cancers. Genome Biol 2011;12:R41. [Crossref] [PubMed]
- Wang Z, Jensen MA, Zenklusen JC. A Practical Guide to The Cancer Genome Atlas (TCGA). Methods Mol Biol 2016;1418:111-41. [Crossref] [PubMed]
- Akiyama T, Raftery LA, Wharton KA. Bone morphogenetic protein signaling: the pathway and its regulation. Genetics 2024;226:iyad200. [Crossref] [PubMed]
- Zhou J, Yang Y, Zhang H, et al. Overexpressed COL3A1 has prognostic value in human esophageal squamous cell carcinoma and promotes the aggressiveness of esophageal squamous cell carcinoma by activating the NF-κB pathway. Biochem Biophys Res Commun 2022;613:193-200. [Crossref] [PubMed]
- Huang R, Li Z, Zhu X, et al. Collagen Type III Alpha 1 chain regulated by GATA-Binding Protein 6 affects Type II IFN response and propanoate metabolism in the recurrence of lower grade glioma. J Cell Mol Med 2020;24:10803-15. [Crossref] [PubMed]
- Richart L, Carrillo-de Santa Pau E, Río-Machín A, et al. BPTF is required for c-MYC transcriptional activity and in vivo tumorigenesis. Nat Commun 2016;7:10153. [Crossref] [PubMed]
- Yuan J, Xie A, Cao Q, et al. INHBB Is a Novel Prognostic Biomarker Associated with Cancer-Promoting Pathways in Colorectal Cancer. Biomed Res Int 2020;2020:6909672. [Crossref] [PubMed]
- Della Peruta M, Giagulli C, Laudanna C, et al. RHOA and PRKCZ control different aspects of cell motility in pancreatic cancer metastatic clones. Mol Cancer 2010;9:61. [Crossref] [PubMed]
- Liu L, Xiao S, Wang Y, et al. Identification of a novel circular RNA circZNF652/miR-486-5p/SERPINE1 signaling cascade that regulates cancer aggressiveness in glioblastoma (GBM). Bioengineered 2022;13:1411-23. [Crossref] [PubMed]
- Diao Y, Jin B, Huang L, et al. MiR-129-5p inhibits glioma cell progression in vitro and in vivo by targeting TGIF2. J Cell Mol Med 2018;22:2357-67. [Crossref] [PubMed]
- Yang H, Sun B, Fan L, et al. Multi-scale integrative analyses identify THBS2(+) cancer-associated fibroblasts as a key orchestrator promoting aggressiveness in early-stage lung adenocarcinoma. Theranostics 2022;12:3104-30. [Crossref] [PubMed]
- Xiong Y, Zhang Y, Xiong S, et al. A Glance of p53 Functions in Brain Development, Neural Stem Cells, and Brain Cancer. Biology (Basel) 2020;9:285. [Crossref] [PubMed]
- Tschernia NP, Gulley JL. Tumor in the Crossfire: Inhibiting TGF-β to Enhance Cancer Immunotherapy. BioDrugs 2022;36:153-80. [Crossref] [PubMed]
- Singh R, Mishra MK, Aggarwal H. Inflammation, Immunity, and Cancer. Mediators Inflamm 2017;2017:6027305. [Crossref] [PubMed]
- Zingoni A, Antonangeli F, Sozzani S, et al. The senescence journey in cancer immunoediting. Mol Cancer 2024;23:68. [Crossref] [PubMed]
- Andersen BM, Reardon DA. Immunotherapy approaches for adult glioma: knowledge gained from recent clinical trials. Curr Opin Neurol 2022;35:803-13. [Crossref] [PubMed]
- Lv Q, Zhang Z, Fu H, et al. Predictive Panel for Immunotherapy in Low-Grade Glioma. World Neurosurg 2024;183:e825-37. [Crossref] [PubMed]
- Shi X, Yang J, Deng S, et al. TGF-β signaling in the tumor metabolic microenvironment and targeted therapies. J Hematol Oncol 2022;15:135. [Crossref] [PubMed]
- Guido C, Whitaker-Menezes D, Capparelli C, et al. Metabolic reprogramming of cancer-associated fibroblasts by TGF-β drives tumor growth: connecting TGF-β signaling with "Warburg-like" cancer metabolism and L-lactate production. Cell Cycle 2012;11:3019-35. [Crossref] [PubMed]
- Fosslien E. Cancer morphogenesis: role of mitochondrial failure. Ann Clin Lab Sci 2008;38:307-29. [PubMed]
- Koundouros N, Poulogiannis G. Reprogramming of fatty acid metabolism in cancer. Br J Cancer 2020;122:4-22. [Crossref] [PubMed]
- Damaghi M, Gillies R. Phenotypic changes of acid-adapted cancer cells push them toward aggressiveness in their evolution in the tumor microenvironment. Cell Cycle 2017;16:1739-43. [Crossref] [PubMed]
- Corbet C, Feron O. Tumour acidosis: from the passenger to the driver's seat. Nat Rev Cancer 2017;17:577-93. [Crossref] [PubMed]