Development and validation of a clinical diagnostic model for surgical site infection after surgery in patients with gastric cancer
IntroductionOther Section
Gastric cancer is the most prevalent malignant tumor globally. According to the GLOBOCAN 2020 report, there were over one million new cases of gastric cancer worldwide in 2020, with over 760,000 deaths. The incidence ranks fifth globally, while the mortality rate stands at fourth (1). Surgery is the primary means of treating gastric cancer (2,3). Currently, surgical site infection (SSI) is a significant clinical concern following gastric cancer surgery, with approximately 10% of post-gastric cancer surgery patients globally facing the risk of SSI (4). This risk is closely associated with factors such as patient age, surgery duration, and surgical technique (5-7).
In recent years, there has been a growing emphasis on reducing SSIs following gastric cancer surgeries (8,9). Researchers from both domestic and international backgrounds have dedicated efforts to investigate potential factors contributing to postoperative SSIs in gastric cancer and developing relevant prediction models. Kosuga et al. employed logistic regression to validate that factors such as gender, chronic liver disease, and total gastrectomy were linked to the risk of SSI (10). Conversely, Mao et al. examined 682 postoperative gastric cancer patients and identified myasthenia gravis as a significant factor for abdominal infections, subsequently creating a relevant prediction model (11). Kim et al. demonstrated that the visceral-to-subcutaneous-fat ratio served as an independent predictor of SSIs following gastric resection using logistic regression analysis (12). With the continuous development of artificial intelligence, robotics is expected to replace traditional surgery as the mainstream way to treat gastric cancer (13-15). However, despite the promising future, there are still some challenges and knowledge gaps in comprehensively considering the impact of robotic surgery on SSIs after gastric cancer surgery and in constructing a sound prediction scheme. At the current stage, an exhaustive assessment of existing SSI prediction models, highlighting their predictive accuracy, methodological strengths and weaknesses, limitations, and future research directions, will help to promote the further development of the field.
This study considered comprehensive risk factors for the occurrence of SSI post-gastric cancer surgery and established a nomogram with excellent discriminatory characteristics. Clinicians can utilize this nomogram as a decision-making tool for customizing individualized treatment for gastric cancer patients. It also serves as a valuable reference for the prevention and treatment of SSI after gastric cancer surgery. We present this article in accordance with the TRIPOD reporting checklist (available at https://tcr.amegroups.com/article/view/10.21037/tcr-24-79/rc).
MethodsOther Section
Patients
A retrospective analysis was conducted on patients who underwent radical gastric cancer surgery at Gansu Provincial People’s Hospital from June 2021 to March 2023 (677 cases) and at The First Hospital of Lanzhou University from January 2021 to June 2021 (187 cases). Inclusion criteria comprised: (I) age greater than 18 years; and (II) patients diagnosed with primary gastric adenocarcinoma through histology. Exclusion criteria were as follows: (I) non-primary gastric cancer; (II) history of previous gastric resection; (III) plan to switch from laparoscopic or robot-assisted surgery to open surgery; (IV) T4b or palliative resection; and (V) incomplete clinical or pathological data. Finally, 763 patients were included in the final analysis. Patients from the hospital with a larger sample size (training set) were used to derive the nomogram (601 cases), while patients from the other hospital constituted the validation set (162 cases). Pathological diagnosis and staging were performed according to the eighth edition of the American Joint Committee on Cancer (AJCC) tumor-node-metastasis (TNM) classification system for gastric cancer (16). This study adhered to the Declaration of Helsinki (as revised in 2013) and was approved by the Ethics Committee of Gansu Provincial People’s Hospital (No. 2023-535) and the Ethics Committee of The First Hospital of Lanzhou University (No. LDYYLL2024-305). The study was a retrospective case-control study and was approved by the institutional review boards of the participating institutions; therefore, informed consent was not required.
Definition of SSI
According to the guidelines of the Centers for Disease Control and Prevention (CDC), SSIs are defined as follows: the definition includes organ/space SSI and incisional SSI (17). In essence, superficial incisional SSI is confined to the skin and subcutaneous tissue, while deep incisional SSI refers to infections involving the fascial and muscle layers. Organ/space infection involves any organ or space beyond the abdominal wall incision layer.
Data collection
The following variables were extracted from medical records in two central databases: age, gender, body mass index (BMI), American Society of Anesthesiologists (ASA) grade, chronic diseases (hypertension, diabetes, cardiovascular diseases, pulmonary diseases), abdominal surgery history, hemoglobin concentration, albumin concentration, lymphocyte count, white blood cell count, perioperative blood transfusion (BTF), operation time, intraoperative blood loss, operation method (robotic-assisted surgery, laparoscopic surgery, open surgery), total gastrectomy, reconstruction method, combined resection, lymphadenectomy, tumor location, tumor diameter, tumor stage, duration of prophylactic antibiotic use.
Research design
In this clinical diagnostic prediction study, a dataset of gastric cancer patients obtained from Gansu Provincial People’s Hospital was utilized as the training set, while data from postoperative gastric cancer patients from The First Hospital of Lanzhou University served as the external validation set. The study design encompassed a univariate analysis of all collected variables as independent variables, with the presence or absence of SSI serving as the dependent variable. Subsequently, multiple statistically significant factors were incorporated into a multifactorial logistic regression analysis. The predictive performance of the model were assessed through the area under the receiver operating characteristic (ROC) curve (AUC), calibration curve, and decision curve analysis (DCA).
Statistical analysis
Continuous data were expressed as mean ± standard deviation or median (interquartile range) based on the data distribution. Data comparison was conducted using t-tests or Mann-Whitney U tests. Categorical variables were represented as frequencies (percentage values) and compared using the χ2 test or Fisher’s exact test.
After screening for independent predictors of SSI through univariate analysis, a predictive model for the probability of developing an SSI after gastric cancer surgery was created by incorporating all variables with P values of less than 0.05 in a multivariate logistic regression model. Prediction probabilities and odds ratios (ORs) with a 95% confidence interval (CI) were calculated. The model’s predictive performance was evaluated based on its discrimination and calibration. Discrimination was assessed using the AUC, while calibration was evaluated using calibration curves to reflect the agreement between observed and predicted results (18). DCA was performed to measure the net benefits at corresponding threshold probabilities (19). In two-sided statistical tests, a P value of 0.05 or lower was considered statistically significant. All statistical analyses were performed with R v4.3.0 and SPSS 25.0.
ResultsOther Section
Clinical characteristics of patients
Table 1 presents the clinical and pathological characteristics of patients in the training and validation sets. In the training set, a total of 601 patients meeting the inclusion criteria were included in the study, among whom 64 individuals (10.6%) experienced postoperative SSI. In the validation set, there were 162 patients, with 19 individuals (11.7%) developing postoperative SSI. The majority of patients were male, comprising 453 males (75.4%) in the training set and 125 males (77.2%) in the validation set. Regarding surgical procedures, there were 155 cases (25.8%) and 9 cases (5.6%) of Da Vinci robot-assisted surgery, 275 cases (45.8%) and 86 cases (53.1%) of laparoscopic surgery, and 171 cases (28.5%) and 67 cases (41.4%) of open surgery in the training and validation sets, respectively. Statistically significant differences were observed between the two groups in ASA grade (P=0.047), preoperative albumin (P<0.001), operation method (P<0.001), and reconstruction (P=0.01).
Table 1
Variables | Training cohort (n=601) | Validation cohort (n=162) | P value |
---|---|---|---|
Gender | 0.64 | ||
Male | 453 (75.4) | 125 (77.2) | |
Female | 148 (24.6) | 37 (22.8) | |
Age (years) | 61 [55–68] | 60 [52–67] | 0.08 |
BMI (kg/m2) | 22.1 [19.8–24.5] | 22.1 [20.1–24.6] | 0.76 |
Hypertension | 0.58 | ||
No | 508 (84.5) | 134 (82.7) | |
Yes | 93 (15.5) | 28 (17.3) | |
Diabetes mellitus | 0.39 | ||
No | 560 (93.2) | 154 (95.1) | |
Yes | 41 (6.8) | 8 (4.9) | |
Cardiovascular disease | 0.68 | ||
No | 555 (92.3) | 148 (91.4) | |
Yes | 46 (7.7) | 14 (8.6) | |
Pulmonary disease | 0.83 | ||
No | 561 (93.3) | 152 (93.8) | |
Yes | 40 (6.7) | 10 (6.2) | |
Abdominal surgery history | 0.32 | ||
No | 526 (87.5) | 137 (84.6) | |
Yes | 75 (12.5) | 35 (15.4) | |
ASA | 0.047 | ||
1 | 8 (1.3) | 7 (4.3) | |
2 | 528 (87.9) | 136 (84.0) | |
3 | 65 (10.8) | 19 (11.7) | |
Preoperative laboratory tests | |||
White blood cell count (×109/L) | 5.3 [4.2–6.5] | 5.4 [4.4–6.5] | 0.71 |
Lymphocyte count (×109/L) | 1.3 [1.0–1.7] | 1.3 [1.1–1.6] | 0.65 |
Hemoglobin (g/L) | 129.00 [99.00–147.00] | 132.00 [110.75–146.25] | 0.18 |
Albumin (g/L) | 38.6 [35.5–41.5] | 42.0 [39.5–45.6] | <0.001 |
Operation time (min) | 240 [200–290] | 247 [210–300] | 0.31 |
Blood loss (mL) | 100 [50–200] | 100 [95–200] | 0.09 |
Operation method | <0.001 | ||
Robotic-assisted surgery | 155 (25.8) | 9 (5.6) | |
Laparoscopic surgery | 275 (45.8) | 86 (53.1) | |
Open surgery | 171 (28.5) | 67 (41.4) | |
Total gastrectomy | 0.96 | ||
No | 325 (54.1) | 88 (54.3) | |
Yes | 276 (45.9) | 74 (45.7) | |
Reconstruction | 0.01 | ||
B-I | 76 (12.6) | 7 (4.3) | |
B-II | 227 (37.8) | 77 (47.5) | |
Roux-en-Y | 278 (46.3) | 72 (44.4) | |
Other | 20 (3.3) | 6 (3.7) | |
Combined resection | 0.18 | ||
No | 539 (89.7) | 151 (93.2) | |
Yes | 62 (10.3) | 11 (6.8) | |
Lymphadenectomy | 0.20 | ||
D1 | 38 (6.3) | 6 (3.7) | |
D2 | 563 (93.7) | 156 (96.3) | |
Tumor location | 0.92 | ||
Lower | 306 (50.9) | 85 (52.5) | |
Middle | 184 (30.6) | 47 (29.0) | |
Upper | 111 (18.5) | 30 (18.5) | |
Tumor diameter (cm) | 4.0 [3.0–6.0] | 4.0 [2.5–5.5] | 0.08 |
Tumor stage | 0.31 | ||
1 | 137 (22.8) | 35 (21.6) | |
2 | 143 (23.8) | 48 (29.6) | |
3 | 321 (53.4) | 79 (48.8) | |
Perioperative BTF | 0.07 | ||
No | 457 (76.0) | 134 (82.7) | |
Yes | 144 (24.0) | 28 (17.3) |
Data are presented as n (%) or median [IQR]. SSI, surgical site infection; BMI, body mass index; ASA, American Society of Anesthesiologist; BTF, blood transfusion; IQR, interquartile range.
Risk factor in the training set
Univariate and multivariate analyses were conducted on the training set to further construct the nomogram. Eight variables were found to be closely associated with SSI through univariate analysis. Subsequently, multivariate logistic regression analysis on this basis identified age (≥65 years: OR =2.53, 95% CI: 1.40–4.58, P=0.002), operation time (≥280 minutes: OR =9.12, 95% CI: 4.59–18.12, P<0.001), operation method (laparoscopic surgery: OR =1.71, 95% CI: 0.78–3.73, P=0.18; open surgery: OR =6.07, 95% CI: 2.53–14.55, P<0.001), total gastrectomy (OR =2.18, 95% CI: 1.18–4.03, P=0.01), and tumor diameter (≥5 cm: OR =2.05, 95% CI: 1.14–3.68, P=0.02) as independent risk factors for SSI (Table 2).
Table 2
Variables | Univariate analysis | Multivariate analysis | |||
---|---|---|---|---|---|
OR (95% CI) | P value | OR (95% CI) | P value | ||
Gender | 0.25 | ||||
Male | 1 | ||||
Female | 0.68 (0.35–1.31) | ||||
Age (years) | <0.001 | 0.002 | |||
<65 | 1 | 1 | |||
≥65 | 2.93 (1.70–5.05) | 2.53 (1.40–4.58) | |||
BMI (kg/m2) | 0.53 | ||||
<25 | 1 | ||||
≥25 | 1.22 (0.66–2.25) | ||||
Hypertension | 0.44 | ||||
No | 1 | ||||
Yes | 1.30 (0.66–2.54) | ||||
Diabetes mellitus | 0.11 | ||||
No | 1 | ||||
Yes | 0.20 (0.03–1.46) | ||||
Cardiovascular disease | 0.96 | ||||
No | 1 | ||||
Yes | 1.03 (0.39–2.69) | ||||
Pulmonary disease | 0.12 | ||||
No | 1 | ||||
Yes | 0.20 (0.03–1.50) | ||||
Abdominal surgery history | |||||
No | 1 | ||||
Yes | 0.70 (0.29–1.69) | 0.43 | |||
ASA | 0.59 (0.25–1.40) | 0.23 | |||
1 | |||||
2 | |||||
3 | |||||
White blood cell count (×109/L) | 0.51 | ||||
<4 | 1 | ||||
≥4 | 1.28 (0.61–2.68) | ||||
Lymphocyte count (×109/L) | 0.21 | ||||
<0.8 | 1 | ||||
≥0.8 | 0.59 (0.27–1.33) | ||||
Hemoglobin (g/L) | 0.36 | ||||
<110 | 1 | ||||
≥110 | 0.78 (0.46–1.33) | ||||
Albumin (g/L) | 0.18 | ||||
<30 | 1 | ||||
≥30 | 0.53 (0.21–1.34) | ||||
Operation time (min) | <0.001 | <0.001 | |||
<280 | 1 | 1 | |||
≥280 | 5.70 (3.26–9.94) | 9.12 (4.59–18.12) | |||
Blood loss (mL) | 0.63 | ||||
<100 | 1 | ||||
≥100 | 1.15 (0.65–2.05) | ||||
Operation method | 0.02 | ||||
Robotic-assisted surgery | 1 | 1 | |||
Laparoscopic surgery | 1.19 (0.58–2.45) | 0.63 | 1.71 (0.78–3.73) | 0.18 | |
Open surgery | 2.23 (1.09–4.58) | 0.03 | 6.07 (2.53–14.55) | <0.001 | |
Total gastrectomy | <0.001 | 0.01 | |||
No | 1 | 1 | |||
Yes | 3.41 (1.93–6.03) | 2.18 (1.18–4.03) | |||
Reconstruction | 0.003 | ||||
B-I | 1 | ||||
B-II | 0.59 (0.21–1.67) | 0.32 | |||
Roux-en-Y | 2.25 (0.92–5.50) | 0.08 | |||
Other | 1.30 (0.24–6.97) | 0.76 | |||
Combined resection | 0.02 | ||||
No | 1 | ||||
Yes | 2.25 (1.13–4.49) | ||||
Lymphadenectomy | 0.28 | ||||
D1 | 1 | ||||
D2 | 2.23 (0.52–9.48) | ||||
Tumor location | 0.25 | ||||
Lower | 1 | ||||
Middle | 0.84 (0.45–1.59) | 0.60 | |||
Upper | 1.60 (0.85–3.03) | 0.15 | |||
Tumor diameter (cm) | 0.001 | 0.02 | |||
<5 | 1 | 1 | |||
≥5 | 2.40 (1.41–4.10) | 2.05 (1.14–3.68) | |||
Tumor stage | 1.57 (1.09–2.26) | 0.01 | |||
1 | |||||
2 | |||||
3 | |||||
Perioperative BTF | 0.61 | ||||
No | 1 | ||||
Yes | 1.17 (0.65–2.11) | ||||
Duration of prophylactic antibiotics (days) | 0.77 | ||||
≤2 | 1 | ||||
>2 | 1.09 (0.61–1.96) |
SSI, surgical site infection; OR, odds ratio; CI, confidence interval; BMI, body mass index; ASA, American Society of Anesthesiologists; BTF, blood transfusion.
Development and validation of the nomogram
Based on the independent features identified in the multivariate analysis, a nomogram was created to predict the incidence of SSI in gastric cancer patients. By summing the results of the five predictive factors, the nomogram can calculate the likelihood of SSI. The score of each variable was transformed linearly to a 100‐point scale. The higher the total score, the higher the estimated incidence of SSI (Figure 1).
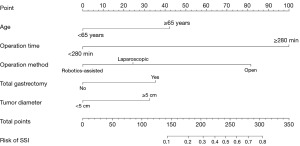
Performances of the nomogram
Following the ROC analysis of the training set, the AUC was determined to be 0.834 (95% CI: 0.790–0.878), exhibiting a specificity of 65.4% and a sensitivity of 89.1% (Figure 2A). Within the validation set, the AUC was calculated as 0.798 (95% CI: 0.699–0.879), demonstrating a specificity of 72% and a sensitivity of 73.7% (Figure 2B). This indicates that the model has good discriminative ability and accuracy. In the calibration plots for the training and validation sets, the model shows good fit and calibration with the ideal curve (Figure 3). The Y-axis of the decision curve represents the net benefit. The predictive model is represented by the red line, the all-treatment strategy is shown as the gray line, and the no-treatment strategy is represented by the black line. In the threshold probability range of the DCA curve, the net benefit for patients is higher than the other two extreme curves. When the patient’s threshold probability exceeds 5%, the treatment plan created by the nomogram model is more valuable than the “all or none” strategy (Figure 4).
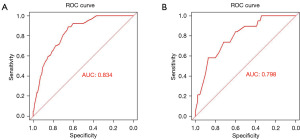
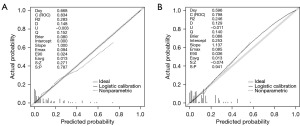
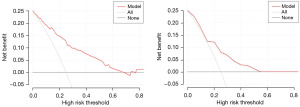
DiscussionOther Section
Current gastric cancer treatment primarily involves surgical resection, but SSI is a common complication and an important clinical outcome indicator for elective surgery (20,21). SSI can lead to prolonged hospital stays, septic shock, multi-organ failure, and even death (22,23). Although studies have been conducted on SSI after gastric cancer surgery, the numerous influencing factors make accurate prediction challenging. In this study, the incidence of SSI after gastric cancer surgery was 10.6%, comparable to a study in Japan (10).
The study analyzed data of 601 patients undergoing gastric cancer resection for 25 potential variables associated with SSI. The following independent risk factors were identified: age (≥65 years), operation time (≥280 minutes), operation method (laparoscopic surgery, open surgery) tumor size (≥5 cm), and total gastrectomy. The nomogram showed good diagnostic performance (AUC =0.834) and was externally validated with data from another hospital. Additionally, according to DCA, the predictive model demonstrated excellent performance in clinical application.
SSI is more common in the elderly, and many studies have investigated related risk factors. In this study, advanced age was also identified as a risk factor in multivariate analysis. This suggests that healthcare professionals should pay more attention to these patients, who are more prone to infections and have longer postoperative recovery times (24-26). Furthermore, longer surgery duration (>280 minutes) was an independent risk factor for SSI, consistent with previous research. Kim et al. reported that the duration of surgery was a risk factor for SSI after gastrectomy (27). This has been demonstrated in patients undergoing gastrectomy. Surgeons need to make efforts to shorten the duration of surgery to prevent SSI Shortening the duration of surgery to prevent SSI infection.
Total gastrectomy and tumor size were identified as independent risk factors for SSI. The impact of these factors on infection is closely related to expanded surgical scope, prolonged surgery duration, and increased intraoperative bleeding (28-30). Moreover, multi-organ resection may be another independent risk factor for infection, as mentioned in previous research results (31).
While risk factors for SSI based on surgical approach have been studied, with the development of various surgical methods such as laparoscopic and robotic gastrectomy, the previous nomogram neglected factors affecting SSI in gastric cancer patients after robotic surgery (32-34). In this study, we considered robotic surgery as a factor, with an SSI incidence of 10.6% (64/601), where the SSI incidence for robotic surgery was 7.7% (12/155), laparoscopic surgery was 9.1% (25/275), and open surgery was 15.8% (27/171). These results indicate that the SSI incidence for minimally invasive surgery (e.g., laparoscopic or robotic) is significantly lower than that for open surgery (P=0.03), highlighting minimally invasive surgery as a dominant factor in SSI after gastric cancer surgery. It should be incorporated into the nomogram, and from a postoperative infection prevention perspective, patients are recommended to undergo such surgeries.
The study has several limitations. Firstly, it adopts a retrospective design, which may introduce selection bias. Secondly, differences in the proportion of inpatients and referral bias may be reasons for the distinct baseline characteristics between the two patient groups. Thirdly, the small sample size may impact the accuracy of the model. Hence, conducting more multicenter randomized controlled trials is warranted to enhance the model’s effectiveness.
ConclusionsOther Section
Herein, we constructed a nomogram model to predict postoperative SSI in gastric cancer using logistic regression analysis. The training group and validation group had AUC of 0.834 and 0.798, respectively, indicating excellent predictive capability. The nomogram model can serve as a reference for the selection of elective surgery and surgical methods, effectively reducing the risk of postoperative SSI in gastric cancer patients.
AcknowledgmentsOther Section
Funding: This work was supported by
FootnoteOther Section
Reporting Checklist: The authors have completed the TRIPOD reporting checklist. Available at https://tcr.amegroups.com/article/view/10.21037/tcr-24-79/rc
Data Sharing Statement: Available at https://tcr.amegroups.com/article/view/10.21037/tcr-24-79/dss
Peer Review File: Available at https://tcr.amegroups.com/article/view/10.21037/tcr-24-79/prf
Conflicts of Interest: All authors have completed the ICMJE uniform disclosure form (available at https://tcr.amegroups.com/article/view/10.21037/tcr-24-79/coif). The authors have no conflicts of interest to declare.
Ethical Statement: The authors are accountable for all aspects of the work in ensuring that questions related to the accuracy or integrity of any part of the work are appropriately investigated and resolved. This study adhered to the Declaration of Helsinki (as revised in 2013) and was approved by the Ethics Committee of Gansu Provincial People’s Hospital (No. 2023-535) and the Ethics Committee of The First Hospital of Lanzhou University (No. LDYYLL2024-305), respectively, and was exempted from the requirement of informed consent because it was a retrospective case-control study and the study was approved by the institutional review boards of each participating site.
Open Access Statement: This is an Open Access article distributed in accordance with the Creative Commons Attribution-NonCommercial-NoDerivs 4.0 International License (CC BY-NC-ND 4.0), which permits the non-commercial replication and distribution of the article with the strict proviso that no changes or edits are made and the original work is properly cited (including links to both the formal publication through the relevant DOI and the license). See: https://creativecommons.org/licenses/by-nc-nd/4.0/.
ReferencesOther Section
- Sung H, Ferlay J, Siegel RL, et al. Global Cancer Statistics 2020: GLOBOCAN Estimates of Incidence and Mortality Worldwide for 36 Cancers in 185 Countries. CA Cancer J Clin 2021;71:209-49. [Crossref] [PubMed]
- Hoshi H. Management of Gastric Adenocarcinoma for General Surgeons. Surg Clin North Am 2020;100:523-34. [Crossref] [PubMed]
- Angelicone I, de Giacomo F, Priore A, et al. Radiotherapy in gastric cancer: does it still play a significant role? Dig Med Res 2023;6:25. [Crossref]
- Ushiku H, Hosoda K, Yamashita K, et al. A Risk Model for Surgical Site Infection in the Gastric Cancer Surgery Using Data of 790 Patients. Dig Surg 2015;32:472-9. [Crossref] [PubMed]
- Tu RH, Huang CM, Lin JX, et al. A scoring system to predict the risk of organ/space surgical site infections after laparoscopic gastrectomy for gastric cancer based on a large-scale retrospective study. Surg Endosc 2016;30:3026-34. [Crossref] [PubMed]
- Han WH, Oh YJ, Eom BW, et al. Prognostic impact of infectious complications after curative gastric cancer surgery. Eur J Surg Oncol 2020;46:1233-8. [Crossref] [PubMed]
- Han X, Kong D, He X, et al. A meta-analysis of the effect of laparoscopic gastric resection on the surgical site wound infection in patients with advanced gastric cancer. Int Wound J 2023;20:4300-7. [Crossref] [PubMed]
- Dong Z, Liu G, Tu L, et al. Establishment of a prediction model of postoperative infection complications in patients with gastric cancer and its impact on prognosis. J Gastrointest Oncol 2023;14:1250-8. [Crossref] [PubMed]
- Sakamoto S, Wada I, Omichi K, et al. Risk factors for remnant gastric cancer after distal gastrectomy for gastric cancer: a retrospective database review. J Gastrointest Oncol 2023;14:2334-45. [Crossref] [PubMed]
- Kosuga T, Ichikawa D, Komatsu S, et al. Clinical and surgical factors associated with organ/space surgical site infection after laparoscopic gastrectomy for gastric cancer. Surg Endosc 2017;31:1667-74. [Crossref] [PubMed]
- Mao CC, Chen XD, Lin J, et al. A Novel Nomogram for Predicting Postsurgical Intra-abdominal Infection in Gastric Cancer Patients: a Prospective Study. J Gastrointest Surg 2018;22:421-9. [Crossref] [PubMed]
- Kim JH, Kim J, Lee WJ, et al. A High Visceral-To-Subcutaneous Fat Ratio is an Independent Predictor of Surgical Site Infection after Gastrectomy. J Clin Med 2019;8:494. [Crossref] [PubMed]
- Isobe T, Murakami N, Minami T, et al. Robotic versus laparoscopic distal gastrectomy in patients with gastric cancer: a propensity score-matched analysis. BMC Surg 2021;21:203. [Crossref] [PubMed]
- Lu J, Zheng CH, Xu BB, et al. Assessment of Robotic Versus Laparoscopic Distal Gastrectomy for Gastric Cancer: A Randomized Controlled Trial. Ann Surg 2021;273:858-67. [Crossref] [PubMed]
- Tian Y, Cao S, Kong Y, et al. Short- and long-term comparison of robotic and laparoscopic gastrectomy for gastric cancer by the same surgical team: a propensity score matching analysis. Surg Endosc 2022;36:185-95. [Crossref] [PubMed]
- Amin MB, Greene FL, Edge SB, et al. The Eighth Edition AJCC Cancer Staging Manual: Continuing to build a bridge from a population-based to a more "personalized" approach to cancer staging. CA Cancer J Clin 2017;67:93-9.
- Mangram AJ, Horan TC, Pearson ML, et al. Guideline for prevention of surgical site infection, 1999. Hospital Infection Control Practices Advisory Committee. Infect Control Hosp Epidemiol 1999;20:250-78; quiz 279-80. [Crossref] [PubMed]
- Kramer AA, Zimmerman JE. Assessing the calibration of mortality benchmarks in critical care: The Hosmer-Lemeshow test revisited. Crit Care Med 2007;35:2052-6. [Crossref] [PubMed]
- Vickers AJ, Elkin EB. Decision curve analysis: a novel method for evaluating prediction models. Med Decis Making 2006;26:565-74. [Crossref] [PubMed]
- Baiocchi GL, Giacopuzzi S, Marrelli D. Cutoff values of major surgical complications rates after gastrectomy. Updates Surg 2018;70:251-5. [Crossref] [PubMed]
- Matsuda A, Yamada T, Ohta R, et al. Surgical Site Infections in Gastroenterological Surgery. J Nippon Med Sch 2023;90:2-10. [Crossref] [PubMed]
- Claudino MM, Lopes JR, Rodrigues VD, et al. Postoperative complication rate and survival of patients with gastric cancer undergoing immunonutrition: A retrospective study. Nutrition 2020;70:110590. [Crossref] [PubMed]
- Nagata T, Adachi Y, Taniguchi A, et al. Prognostic impacts of categorized postoperative complications in surgery for gastric cancer. Asian J Surg 2023;46:451-7. [Crossref] [PubMed]
- Endo S, Tsujinaka T, Fujitani K, et al. Risk factors for superficial incisional surgical site infection after gastrectomy: analysis of patients enrolled in a prospective randomized trial comparing skin closure methods. Gastric Cancer 2016;19:639-44. [Crossref] [PubMed]
- Horasan ES, Dağ A, Ersoz G, et al. Surgical site infections and mortality in elderly patients. Med Mal Infect 2013;43:417-22. [Crossref] [PubMed]
- Qiao YQ, Zheng L, Jia B, et al. Risk factors for surgical-site infections after radical gastrectomy for gastric cancer: a study in China. Chin Med J (Engl) 2020;133:1540-5. [Crossref] [PubMed]
- Kim JH, Kim J, Lee WJ, et al. The incidence and risk factors for surgical site infection in older adults after gastric cancer surgery: A STROBE-compliant retrospective study. Medicine (Baltimore) 2019;98:e16739. [Crossref] [PubMed]
- Mu C, Zhu C, Li T, et al. Risk factors of post-operation surgical site infection for gastric cancer patients underwent total gastrectomy. Asian J Surg 2023; [Crossref]
- Hayashi M, Yoshikawa T, Yura M, et al. Intraoperative blood loss as an independent prognostic factor for curative resection after neoadjuvant chemotherapy for gastric cancer: a single-center retrospective cohort study. Surg Today 2021;51:293-302. [Crossref] [PubMed]
- Zhang Y, Wang Z, Basharat Z, et al. Nomogram of intra-abdominal infection after surgery in patients with gastric cancer: A retrospective study. Front Oncol 2022;12:982807. [Crossref] [PubMed]
- Xiao H, Xiao Y, Quan H, et al. Intra-abdominal infection after radical gastrectomy for gastric cancer: Incidence, pathogens, risk factors and outcomes. Int J Surg 2017;48:195-200. [Crossref] [PubMed]
- Wang Z, Chen J, Wang P, et al. Surgical Site Infection After Gastrointestinal Surgery in China: A Multicenter Prospective Study. J Surg Res 2019;240:206-18. [Crossref] [PubMed]
- Silva AFD, Mendes KDS, Ribeiro VDS, et al. Risk factors for the development of surgical site infection in bariatric surgery: an integrative review of literature. Rev Lat Am Enfermagem 2023;31:e3798. [PubMed]
- Inokuchi M, Sugita H, Otsuki S, et al. Laparoscopic distal gastrectomy reduced surgical site infection as compared with open distal gastrectomy for gastric cancer in a meta-analysis of both randomized controlled and case-controlled studies. Int J Surg 2015;15:61-7. [Crossref] [PubMed]