Prognostic value and anti-tumor immunity role of TMED9 in pan-cancer: a bioinformatics study
Highlight box
Key findings
• Transmembrane p24 trafficking protein 9 (TMED9) is over-expressed in the majority of cancers.
• TMED9 demonstrates promising clinical prognostic value and holds potential as a diagnostic marker for cancer.
• TMED9 participates in numerous biological pathways implicated in cancer initiation, development, proliferation, and invasion.
• The expression of TMED9 correlates with the infiltration levels of cancer-associated fibroblasts and immune cells, suggesting its potential involvement in shaping the tumor microenvironment.
What is known and what is new?
• Studies have demonstrated the association between the overexpression of TMED9 and cancer development and proliferative migration in cancers such as ovarian cancer, hepatocellular carcinoma, and breast cancer.
• This study comprehensively and meticulously investigates the expression, biological function, mechanisms of cancer involvement, and immune regulation of TMED9 in various tumors, examining them from multiple perspectives and aspects.
What is the implication, and what should change now?
• TMED9 emerges as a potential diagnostic and prognostic marker for various tumors, offering a crucial theoretical foundation for personalized cancer treatment and the advancement of novel targeted drugs and immunotherapy targets.
Introduction
Transmembrane p24 trafficking protein (TMED) family comprises type I single-channel transmembrane proteins found in all eukaryotic organisms. Members of this family serve as crucial regulators of protein translocation, influencing the composition, structure, and function of various organelles, particularly the endoplasmic reticulum and Golgi apparatus (1). As a member of the TMED family, TMED9 is involved in transporting, modifying, and packaging proteins or lipids into vesicles, delivering them to specific locations (2).
TMED9 was found to be implicated in the development of various cancers. TMED9 expression is upregulated in epithelial ovarian cancer compared to normal ovarian epithelium, showing independent prognostic significance for both disease-free survival (DFS) and overall survival (OS) (3). Over-expression of TMED9 promotes breast cancer cell proliferation and migration, serving as a potential prognostic biomarker and drug target (4). TMED9 has also been considered a poor prognostic and prognostic predictive biomarker for hepatocellular carcinoma patients (5). Over-expression of TMED9 promotes colon cancer metastasis by activating the CNIH4/TGFα/GLI signaling pathway (6).
However, no study has comprehensively and meticulously explored the clinical value, biological function, and its role in anti-tumor immunity of TMED9 in patients with different tumors from a pan-cancer perspective. In this study, we conducted a multidimensional analysis of TMED9 expression and its potential clinical value in pan-cancer using a bioinformatics approach with R language and various databases, including Tumor Immune Estimation Resource 2.0 (TIMER2.0; http://timer.cistrome.org/), The Cancer Genome Atlas (TCGA; https://portal.gdc.cancer.gov/), Genotype-Tissue Expression (GTEx; https://xenabrowser.net/), The University of Alabama at Birmingham CANcer data analysis portal (UALCAN; https://ualcan.path.uab.edu/), Human Protein Atlas (HPA; https://proteinatlas.org), and Gene Expression Profiling Interactive Analysis 2 (GEPIA2; http://gepia2.cancer-pku.cn/#index). Additionally, we investigated the relationship between TMED9 expression and anti-tumor immunity in patients with different cancers. Through a series of correlation and expression analyses, we identified the top 100 differentially co-expressed genes with the strongest correlation with TMED9 expression in 33 different cancers. We further explored the specific biological pathways and signaling pathways associated with cancer development of those genes. We propose that TMED9 is a novel “cancer indicator” and “clinical prognostic diagnostic marker” capable of remodeling the tumor microenvironment (TME), influencing the immunotherapeutic response, and guiding the precision treatment of various cancers. We present this article in accordance with the TRIPOD reporting checklist (available at https://tcr.amegroups.com/article/view/10.21037/tcr-24-258/rc).
Methods
Data collection and preprocessing
We obtained the differential expression of the TMED9 gene between tumor tissues and adjacent normal tissues in all TCGA tumor types using TIMER2.0 (7). Utilizing pan-cancer data from the TCGA database (8), we obtained RNA-sequencing data and processed them uniformly by the Toil process (9). The gene expression levels were shown using a log2(TPM +1) scale, where TPM stands for transcripts per million. The dataset included raw data of TMED9 (ENSG00000184840.11) expression in 727 paracancerous normal tissue samples and 9,807 tumor tissue samples. The data underwent log2(TPM +1) normalization and harmonization based on pan-cancer information from the GTEx dataset, incorporating raw TMED9 expression data from 7,568 paracancerous normal tissue samples. Employing the HPA database (10), we compiled and acquired TMED9 messenger RNA (mRNA) expression data from various normal tissues. This study was conducted in accordance with the Declaration of Helsinki (as revised in 2013).
Expression level of TMED9 in pan-cancer patients
We utilized the “Gene DE” module in TIMER2.0 to acquire the differential expression of the TMED9 gene in all TCGA tumors. We utilized the “limma” R package to obtain the expression data of TMED9 in both TCGA and GTEx. We employed the “ggplot2” package to visualize the data and create box plots for a visual comparison of the differences in TMED9 expression between the 33 tumor tissues and normal tissues.
We retrieved immunohistochemical images depicting the differential expression of TMED9 in tumor tissues and normal tissues across nine cancers: breast invasive carcinoma (BRCA), lung adenocarcinoma (LUAD), lung squamous cell carcinoma (LUSC), stomach adenocarcinoma (STAD), colon adenocarcinoma (COAD), head and neck squamous cell carcinoma (HNSC), kidney renal clear cell carcinoma (KIRC), prostate adenocarcinoma (PRAD), and uterine corpus endometrial carcinoma (UCEC), using the HPA database. Simultaneously, we presented box plots depicting TMED9 protein expression in these tumor tissues and normal tissues to enhance result validation.
Clinical prognostic value of TMED9 in pan-cancer
The impact of TMED9 expression on the prognostic value of patients with 33 tumors in TCGA, encompassing OS and DFS, was assessed using GEPIA2 (11). Subsequently, we classified these tumor patients into TMED9 high-expression (top 50%) and low-expression (bottom 50%) groups based on the median value of TMED9 expression. We compared the progression-free interval (PFI) between TMED9 high- and low-expression groups using Kaplan-Meier curves (http://kmplot.com/analysis/) (12) and multifactorial Cox regression. Proportional risk hypothesis testing and fitted survival regressions were conducted using the “survival” package in R. The survival curves were plotted using the “survminer” package. Receiver operating characteristic (ROC) analysis was executed employing the “pROC” package.
We categorized BRCA patients based on race and age, HNSC patients based on N stage, histologic grade, and disease-specific survival (DSS) event, liver hepatocellular carcinoma (LIHC) patients based on race, histologic grade, weight, height, and alpha-fetoprotein concentration, KIRC patients based on histologic grade, DSS event, pathologic stage, and PFI event, LUAD patients based on N stage, smoker, and OS event, and thyroid carcinoma (THCA) patients based on N stage, T stage, and extra-thyroidal invasion. The “stats” and “car” packages in R software were employed for statistical analysis. Visualization was done using the “ggplot2” package. To assess the prognostic potential of TMED9 in BRCA, HNSC, LIHC, KIRC, LUAD, and THCA, following the methodology outlined by Zhang et al. (13), we conducted both univariate and multivariate Cox regression analyses for OS. It is important to note that samples were included in the multivariate Cox model only if they met the set P value threshold (P<0.1) in the univariate Cox analysis. Proportional risk hypothesis testing and Cox regression analysis were conducted using the “survival” and “rms” packages in R software.
Role of TMED9 expression in pan-cancer in regulating immune infiltrating cells
Utilizing TIMER2.0, we examined the correlation between TMED9 expression in various TCGA tumors and diverse immune cells, such as B cells, macrophages, CD8+ T cells, regulatory T cells, cancer-associated fibroblasts (CAFs), CD4+ T cells, myeloid dendritic cells, mast cells, natural killer (NK) cells, neutrophils, and monocytes. We employed multiple algorithms, including TIMER, Estimating the Proportion of Immune and Cancer cells (EPIC), Quantification of Tumor Infiltrating Immune Cells (QUANTISEQ), Xenobiotic Chemical-Responsive Expression Loci (XCELL), Microenvironment Cell Populations-counter (MCPCOUNTER), Cell-type Identification By Estimating Relative Subsets Of RNA Transcripts (CIBERSORT), CIBERSORT Absolute mode (CIBERSORT-abs), and Tumor Immune Dysfunction and Exclusion (TIDE).
Functional enrichment analysis of TMED9 and its differential co-expressed genes in pan-cancer
We obtained the top 100 differential expression genes (DEGs) most similar to TMED9 from all TCGA tumors and normal tissues using GEPIA2. Subsequently, we conducted paired gene-gene Pearson correlation analyses between TMED9 and its DEGs. We employed the “org.Hs.eg.db” package in R V4.2.1 for the ID conversion of DEGs. Additionally, the “clusterProfiler” and “enrichplot” packages were used for Gene Ontology (GO) and Kyoto Encyclopedia of Genes and Genomes (KEGG) enrichment analysis (14). Furthermore, we acquired gene set enrichment analysis (GSEA) gene set [Hallmarks]h.all.v2022.1.Hs.symbols.gmt from the Molecular Signatures Database (MSigDB; https://www.gsea-msigdb.org/gsea/msigdb/collections.jsp) (15) as a reference gene set. We performed GSEA gene enrichment analysis of TMED9 and its DEGs in six cancers, including BRCA, HNSC, LIHC, KIRC, LUAD, and THCA, using the “ClusterProfiler” and “stringi” R packages. Statistical significance was defined as an adjusted P value <0.05 and false discovery rate (FDR) <0.25, indicating significantly enriched gene sets.
Statistical analysis
All statistical analyses were done using R software (version 4.2.1). We assessed differences in TMED9 expression between pan-cancerous and normal tissues using the Wilcoxon rank sum test. We evaluated survival differences between TMED9 high and low expression groups using the Kaplan-Meier method and the log-rank test. We used independent t-tests to assess data significance for comparisons between two groups. For comparisons involving more than two groups, we employed the Kruskal-Wallis test. We employed univariate and multivariate Cox analyses to identify potential prognostic factors. We considered statistical significance at P<0.05. Asterisks (*, **, and ***) represent P<0.05, P<0.01, and P<0.001, respectively.
Results
Differential expression analysis of TMED9 in pan-cancer patients
We assessed the expression level of TMED9 in pan-cancer using TIMER2.0 (Figure 1A). TMED9 expression was significantly upregulated (P<0.001) in various cancers, including bladder urothelial carcinoma (BLCA), BRCA, cholangiocarcinoma (CHOL), COAD, esophageal carcinoma (ESCA), glioblastoma multiforme (GBM), HNSC, KIRC, kidney renal papillary cell carcinoma (KIRP), LIHC, LUAD, LUSC, PRAD, STAD, and UCEC, compared to normal tissues. TMED9 exhibited high expression in cervical squamous cell carcinoma and endocervical adenocarcinoma (CESC), pancreatic adenocarcinoma (PAAD), pheochromocytoma and paraganglioma (PCPG), and rectum adenocarcinoma (READ); however, the results of the analyses were not discriminatory. In contrast, TMED9 expression was significantly lower (P<0.001) in THCA tissues compared to normal tissues. Tumor and normal groups are represented by red and blue boxes, respectively.
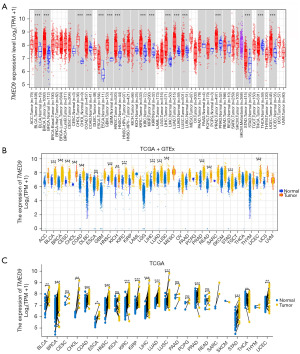
To address the absence of TCGA normal samples, we gathered uniform TCGA + GTEx data from the UCSC Xena (University of California, Santa Cruz Xena Browser) database. Figure 1B illustrates the differential expression of TMED9 in various tumors, including BLCA, BRCA, CESC, CHOL, COAD, HNSC, KICH, KIRC, LIHC, LUAD, LUSC, PRAD, READ, STAD, and UCEC. These findings align broadly with the results obtained from TIMER2.0. Tumor and normal groups are represented by yellow and blue boxes, respectively. Additionally, we analyzed differences in TMED9 expression between different tumors and their paired paracancerous normal tissue samples (Figure 1C). The results revealed elevated TMED9 expression in most tumors compared to paired paracancerous normal tissues, and the identified tumor types broadly aligned with the aforementioned analysis. These results suggest that TMED9 is overexpressed in the majority of cancer types. In addition, using the HPA database we observed that TMED9 was enriched in the choroid plexus and salivary glands (Figure S1). Various colors denote different organs.
Immunohistochemical analysis of TMED9 in different tumor tissues and normal tissues
To validate the expression of TMED9 in pan-cancer, differences in TMED9 mRNA expression and immunohistochemical images in various tumor tissues and normal tissues were verified at the molecular and protein levels, respectively, using UALCAN and HPA databases. Results indicated significant overexpression of TMED9 in nine cancers, namely BRCA, LUAD, LUSC, STAD, COAD, HNSC, KIRC, and PRAD (P<0.001) (Figure 2).
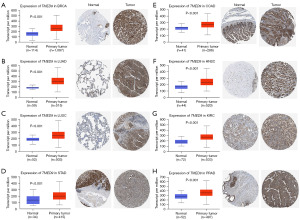
Association of TMED9 with survival in pan-cancer patients
Analyzing the impact of TMED9 expression on the prognostic OS in 33 different cancers, we generated a heat map. The results revealed a higher correlation between TMED9 expression and OS in 5 tumors, including BRCA, HNSC, lower-grade glioma (LGG), LIHC, and uveal melanoma (UVM) (Figure 3A). Results indicated that high expression of TMED9 in BRCA (P=0.002), HNSC (P=0.01), LGG (P<0.001), LIHC (P<0.001), and UVM (P=0.006) was associated with poorer OS of patients (Figure 3B-3F). Using a similar approach as described above, we investigated the DFS of patients based on TMED9 expression in cancers. Illustrated in Figure S2A-S2D, high expression of TMED9 in KIRC (P=0.03), LGG (P<0.001), and mesothelioma (MESO) (P=0.048) was associated with poorer DFS of patients (Figure S2E). Conversely, the low TMED9 expression group in THCA (P=0.03) exhibited worse DFS. Figure S2F-S2I illustrates TMED9 expression and DFS in patients with CHOL (P=0.10), lymphoid neoplasm diffuse large B-cell lymphoma (DLBC) (P=0.24), KICH (P=0.15), and UVM (P=0.28).
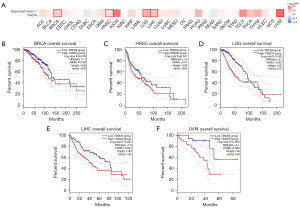
We compared the effects of high and low TMED9 expression on PFI in cancer patients using Kaplan-Meier curves and multifactorial Cox regression. As illustrated in Figure S3, high expression of TMED9 in CESC (P=0.048), HNSC (P=0.001), LGG (P<0.001), COAD (P=0.01), KIRC (P=0.04), and UVM (P=0.04) was associated with poorer PFI in the patients. Conversely, the low TMED9 expression group in THCA (P=0.007) exhibited worse PFI.
Clinical predictive value of TMED9 in different cancers
We evaluated the clinical diagnostic value of TMED9 in various cancers by plotting diagnostic ROC curves for TMED9 expression in patients with BRCA, HNSC, LIHC, KIRC, LUAD, and THCA. The area under the ROC curve values were as follows: 0.880 [95% confidence interval (CI): 0.854–0.906] in BRCA, 0.829 (95% CI: 0.774–0.883) in HNSC, 0.954 (95% CI: 0.930–0.978) in LIHC, 0.841 (95% CI: 0.799–0.882) in KIRC, 0.867 (95% CI: 0.836–0.898) in LUAD, and 0.702 (95% CI: 0.666–0.738) in THCA. These results suggest that TMED9 expression can distinguish between cancerous and normal tissues in these cancers, indicating clinical diagnostic value (Figure 4A-4F).
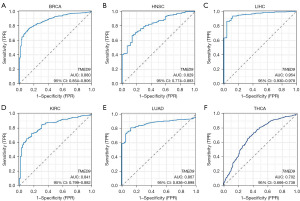
We investigated the correlation between TMED9 and the clinical characteristics of various cancers. Among BRCA patients, TMED9 expression was more pronounced in Asians, Blacks, and African Americans compared to Whites (Figure 5A). Similarly, among LIHC patients, TMED9 expression was more pronounced in Asians than in Whites (Figure 5B). TMED9 expression was upregulated with worse tumor N stage in HNSC and LUAD patients (Figure 5C,5D). Interestingly, high expression of TMED9 in THCA was associated with better N0 staging (Figure 5E), consistent with the above speculation that TMED9 may function as a tumor suppressor oncogene in THCA. High expression of TMED9 in HNSC, LIHC, and KIRC was associated with worse tumor histological grade (Figure 5F-5H). High expression of TMED9 in HNSC and KIRC patients is often associated with death (in DSS event, “yes” indicates that the patient is dead and “no” indicates that the patient is alive) (Figure 5I,5J). Additionally, we observed associations between TMED9 expression and age in BRCA, height, weight, and AFP concentration in LIHC, pathologic stage and PFI event in KIRC, smoker and OS event in LUAD, as well as T-stage and extrathyroidal invasion in THCA (Figure S4). Notably, high expression of TMED9 in THCA was associated with non-invasive staged tumors (T1 and T2 stages), further confirming our hypothesis that TMED9 in THCA may act as a suppressor gene.
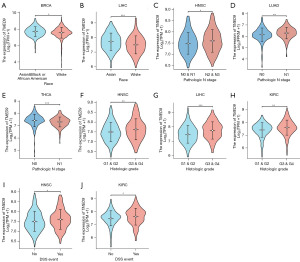
We conducted univariate and multivariate Cox regression analyses of OS for patients with BRCA, HNSC, LIHC, KIRC, LUAD, and THCA to assess the prognostic utility of TMED9. Results showed that high expression of TMED9 was associated with poorer OS in patients with BRCA, HNSC, LIHC, and LUAD. However, there was no statistically significant association between high TMED9 expression and poorer OS in KIRC and THCA patients (Tables S1-S6).
Expression of TMED9 in pan-cancer correlates with immune infiltration
We assessed the correlation between TMED9 expression and the level of immune infiltration in various cancers. Results indicated a positive correlation between TMED9 expression and B cell infiltration in LIHC (Figure 6A) and macrophage infiltration in sarcoma (SARC) (Figure 6B). CD8+ T cell infiltration exhibited a negative correlation with TMED9 expression in PAAD and a positive correlation in UVM (Figure 6C). Regulatory T cell exhibited a positive correlation with TMED9 expression in STAD (Figure 6D). However, there was no significant correlation found between TMED9 expression and infiltration of CD4+ T cell, myeloid dendritic cell, mast cell, NK cell, neutrophil, and monocyte in the majority of cancers (Figure S5).
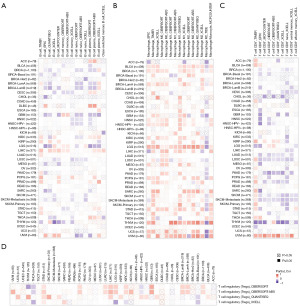
Utilizing EPIC, MCPCOUNTER, XCELL, and TIDE algorithms, we investigated the correlation between CAF infiltration and TMED9 expression in various malignancies. As depicted in Figure 7, TMED9 expression exhibited a positive correlation with CAF infiltration in patients with BLCA, CESC, HNSC, LGG, and thymoma (THYM), and a negative correlation in patients with PRAD.
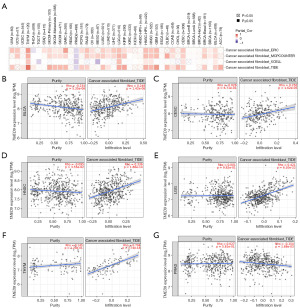
Differential gene and its functional enrichment analysis of TMED9
The top 100 DEGs most associated with TMED9 expression were extracted from all tumor types in the TCGA dataset using GEPIA2; details can be found in Table S7. Figure 8A-8F shows that laminin subunit beta-2 (LAMN2), solute carrier family 39 member 7 (SLC39A7), protein transport protein sec61 subunit alpha isoform 1 (SEC61A1), beta-subunit of prolyl 4-hydroxylase (P4HB), beta-1, 4-galactosyltransferase 7 (B4GALT7), and stromal cell-derived factor 4 (SDF4) are highly correlated with TMED9 expression in most cancer types. Results obtained from using the TIMER2.0 database to verify TMED9 DEGs were consistent with the above findings (Figure 8G and Table S8).
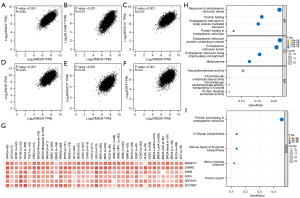
Subsequently, we conducted GO and KEGG analyses on these TMED9 DEGs. The GO analysis indicated the involvement of these DEGs in biological processes, including mainly response to endoplasmic reticulum stress, protein folding, and endoplasmic reticulum to Golgi vesicle-mediated transport (Figure 8H). Figure 8I illustrates the results of KEGG pathway analysis, suggesting the involvement of TMED9 DEGs in signaling pathways, mainly including protein processing in the endoplasmic reticulum and various types of N-glycan biosynthesis.
We conducted GSEA analysis to explore the biological functions associated with TMED9 expression in six tumors related to prognosis as illustrated in Figure 9A, TMED9 expression in BRCA was primarily associated with the Myc targets V2 pathway, reactive oxygen species pathway, and pancreas β cells. Within HNSC, TMED9 was predominantly associated with epithelial-mesenchymal transition (EMT), E2F targeting pathway, and hypoxia (Figure 9B). In LIHC, TMED9 exhibited a primary association with the E2F targeting pathway, G2M checkpoint, and Myc targets V1 pathway (Figure 9C). In KIRC, TMED9 was primarily associated with hypoxia, downregulation of cell cycle targets of TP53 and TP73, and upregulation of the IL-6 signaling pathway (Figure 9D). In LUAD, TMED9 was predominantly associated with DNA strand elongation, hypoxia, and Aerobic Glycolysis (Figure 9E). In THCA, TMED9 was primarily associated with interferon-gamma (IFN-γ) response, inflammatory response, and the IL-6-JAK-STAT3 signaling pathway (Figure 9F).
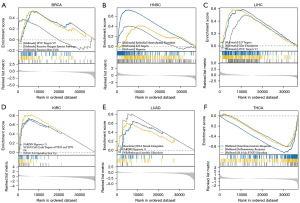
Discussion
In this study, we performed a comprehensive pan-cancer analysis to investigate the role of TMED9 in various cancer types. We observed significant overexpression of TMED9 in most of the 33 TCGA cancer types, particularly in BRCA, LUAD, LUSC, STAD, COAD, HNSC, KIRC, and PRAD, compared to normal tissues. Conversely, TMED9 expression was significantly lower in THCA. Notably, TMED9 enrichment in the choroid plexus, a crucial hub for immune cell (such as CCR6+ and Th17 cells) entry into brain tissue from the periphery (16), suggests a potential link between TMED9 expression and the immune system in the context of cancer biology.
Our analysis revealed that high TMED9 expression was associated with poor OS in BRCA, HNSC, LGG, LIHC, and UVM, poor DFS in KIRC, LGG, and MESO, and poor PFI in CESC, HNSC, LGG, COAD, KIRC, and UVM. Interestingly, low TMED9 expression in THCA was associated with worse DFS and PFI, suggesting a potential tumor-suppressive role for TMED9 in this cancer type. ROC curve analysis demonstrated the diagnostic value of TMED9 expression in distinguishing cancerous tissues from normal tissues in BRCA, HNSC, LIHC, KIRC, LUAD, and THCA. Furthermore, patients with high TMED9 expression were often associated with poorer clinical parameters, including tumor-node-metastasis (TNM) stage, pathologic stage, histologic grade, R-stage, and advanced malignant phenotypes. These findings strongly indicate that TMED9 has an oncogenic role in various cancers and is a particularly valuable in predicting prognosis in BRCA, LIHC, HNSC, KIRC, and LUAD.
The TME plays a crucial role in regulating the immune response to tumors, with significant implications for cancer initiation, progression, metabolism, metastasis, and response to therapy (17). Tumor-infiltrating immune cells are crucial components of the TME (18). We observed that highly expressed TMED9 in many cancers tends to correlate positively with the infiltration degree of immune cells, including B cells, macrophages, CD8+ T cells, and regulatory T cells. Additionally, we found that TMED9 expression in various malignant tumors correlated with the extent of CAFs infiltration. CAFs are known to regulate various tumor-infiltrating immune cells (19), promote tumor immune evasion (20), metastatic invasion of breast cancers (21), and mediate the proliferation and metastasis of ovarian cancer cells through transactivation of LINC00092, a long non-coding RNA involved in increasing glycolysis levels during ovarian cancer metastasis (22). These findings suggest that TMED9 may influence cancer onset, progression, metastasis, and invasion by modulating the infiltration of various immune cells and CAFs in the TME, highlighting its potential as a key player in cancer immunity and tumor progression.
To further elucidate the functional role of TMED9 in cancer, we extracted the top 100 DEGs most correlated with TMED9 expression, and subsequently performed GO and KEGG enrichment analysis. Our results revealed a high correlation between TMED9 expression and genes such as LAMN2, SLC39A7, SEC61A1, P4HB, B4GALT7, and SDF4. GO analysis suggested that these DEGs may participate in various biological processes and pathways closely linked to tumor development, such as response to endoplasmic reticulum stress, protein folding, endoplasmic reticulum to Golgi vesicle-mediated transport, and protein folding in the endoplasmic reticulum. Endoplasmic reticulum stress plays a crucial role in cancer development and is associated with tumor growth, angiogenesis, metastasis, and chemoresistance (23,24). Aberrant Golgi dynamics can alter the TME and immune landscape, enhancing the invasive and metastatic potential of cancer cells (25,26). KEGG pathway analysis also indicated that TMED9-associated DEGs in N-glycan biosynthesis with abnormal N-glycosylation being linked to various aspects of cancer biology, including cell proliferation, migration, and inflammation. Taken together, these findings suggest that the functional expression network of TMED9 plays a crucial role in tumor proliferation and development.
GSEA analysis revealed that TMED9 was predominantly involved in various pathways, including Myc targets V1 and V2 pathways, reactive oxygen species pathway, EMT, G2M checkpoint, E2F targets, hypoxia, DNA strand elongation, IL-6 signaling scar up, IL-6-JAK-STAT3 signaling pathway, aerobic glycolysis, IFN-γ response, and inflammatory response. The Myc targets VI and V2 pathways are involved in the regulation of cell proliferation and apoptosis, with dysregulation of this pathway leading to carcinogenesis (27). EMT can enhance cancer cell invasion and metastasis by boosting cell motility, extracellular matrix degradation, and immune escape (28,29). The G2M checkpoint pathway is linked to pancreatic cancer cell proliferation and drug response (30). The E2F pathway controls cell proliferation in an oncogenic environment compared to normal cells (31). Reactive oxygen species and hypoxia-induced oxidative stress can activate oncogenic signaling pathways, promote DNA damage, and contribute to tumor development (32). IL-6 signaling pathway can contribute to tumorigenesis and progression by activating both the JAK2 and STAT3 signaling pathways. Aerobic glycolysis promotes cancer cell proliferation, and metastasis by activating oncogenic signaling pathways (33,34). IFN-γ signaling enhances programmed death-ligand 1 (PD-L1) expression, leading to immunosuppression (35). Inflammatory cells and the cytokines they produce are likewise associated with tumor progression. Taken together, we suggest that TMED9 may influence cancer development by participating in multiple cancer-related pathways.
This study provides strong evidence that TMED9 is linked to the development and progression of various cancers. However, a limitation of our work is the lack of direct experimental evidence demonstrating how TMED9 influences cancer-related pathways. In addition, it has been observed that TMED9 overexpression promotes autophagy, while TMED9 knockdown diminishes autophagic activity (36,37). Moving forward, we aim to confirm the association of TMED9 with autophagy in pan-cancer.
Conclusions
In this study, we conclude that TMED9 serves as a mediator of multiple tumor-invasive phenotypes and is involved in various biological pathways associated with cancer development. TMED9 may serve as a valid clinical diagnostic marker and an independent prognostic parameter for various cancer types. Additionally, TMED9 can modulate anti-tumor immune responses in pan-cancer patients by influencing the extent of infiltration of immune cells and CAFs in the TME, along with the expression of various immune checkpoint genes. In conclusion, the present study suggests that TMED9 is a novel “cancer indicator” and “clinical prognostic marker” that can remodel the TME, influence the immunotherapeutic response, and guide the precision treatment of various cancers to a certain extent.
Acknowledgments
Funding: This work was supported by
Footnote
Reporting Checklist: The authors have completed the TRIPOD reporting checklist. Available at https://tcr.amegroups.com/article/view/10.21037/tcr-24-258/rc
Data Sharing Statement: Available at https://tcr.amegroups.com/article/view/10.21037/tcr-24-258/dss
Peer Review File: Available at https://tcr.amegroups.com/article/view/10.21037/tcr-24-258/prf
Conflicts of Interest: All authors have completed the ICMJE uniform disclosure form (available at https://tcr.amegroups.com/article/view/10.21037/tcr-24-258/coif). The authors have no conflicts of interest to declare.
Ethical Statement: The authors are accountable for all aspects of the work in ensuring that questions related to the accuracy or integrity of any part of the work are appropriately investigated and resolved. This study was conducted in accordance with the Declaration of Helsinki (as revised in 2013).
Open Access Statement: This is an Open Access article distributed in accordance with the Creative Commons Attribution-NonCommercial-NoDerivs 4.0 International License (CC BY-NC-ND 4.0), which permits the non-commercial replication and distribution of the article with the strict proviso that no changes or edits are made and the original work is properly cited (including links to both the formal publication through the relevant DOI and the license). See: https://creativecommons.org/licenses/by-nc-nd/4.0/.
References
- Zhou L, Li H, Yao H, et al. TMED family genes and their roles in human diseases. Int J Med Sci 2023;20:1732-43. [Crossref] [PubMed]
- Roberts BS, Satpute-Krishnan P. The many hats of transmembrane emp24 domain protein TMED9 in secretory pathway homeostasis. Front Cell Dev Biol 2023;10:1096899. [Crossref] [PubMed]
- Han GH, Yun H, Chung JY, et al. TMED9 Expression Level as a Biomarker of Epithelial Ovarian Cancer Progression and Prognosis. Cancer Genomics Proteomics 2022;19:692-702. [Crossref] [PubMed]
- Ju G, Xu C, Zeng K, et al. High expression of transmembrane P24 trafficking protein 9 predicts poor prognosis in breast carcinoma. Bioengineered 2021;12:8965-79. [Crossref] [PubMed]
- Yang YC, Chien MH, Lai TC, et al. Proteomics-based identification of TMED9 is linked to vascular invasion and poor prognoses in patients with hepatocellular carcinoma. J Biomed Sci 2021;28:29. [Crossref] [PubMed]
- Mishra S, Bernal C, Silvano M, et al. The protein secretion modulator TMED9 drives CNIH4/TGFα/GLI signaling opposing TMED3-WNT-TCF to promote colon cancer metastases. Oncogene 2019;38:5817-37. [Crossref] [PubMed]
- Li T, Fu J, Zeng Z, et al. TIMER2.0 for analysis of tumor-infiltrating immune cells. Nucleic Acids Res 2020;48:W509-14. [Crossref] [PubMed]
- Tomczak K, Czerwińska P, Wiznerowicz M. The Cancer Genome Atlas (TCGA): an immeasurable source of knowledge. Contemp Oncol (Pozn) 2015;19:A68-77. [Crossref] [PubMed]
- Vivian J, Rao AA, Nothaft FA, et al. Toil enables reproducible, open source, big biomedical data analyses. Nat Biotechnol 2017;35:314-6. [Crossref] [PubMed]
- Navani S. Manual evaluation of tissue microarrays in a high-throughput research project: The contribution of Indian surgical pathology to the Human Protein Atlas (HPA) project. Proteomics 2016;16:1266-70. [Crossref] [PubMed]
- Tang Z, Kang B, Li C, et al. GEPIA2: an enhanced web server for large-scale expression profiling and interactive analysis. Nucleic Acids Res 2019;47:W556-60. [Crossref] [PubMed]
- Lánczky A, Győrffy B. Web-Based Survival Analysis Tool Tailored for Medical Research (KMplot): Development and Implementation. J Med Internet Res 2021;23:e27633. [Crossref] [PubMed]
- Zhang ZF. A comprehensive prognostic and immune infiltration analysis of EXOC3L1 in pan-cancer. Front Genet 2022;13:1044100. [Crossref] [PubMed]
- Wu T, Hu E, Xu S, et al. clusterProfiler 4.0: A universal enrichment tool for interpreting omics data. Innovation (Camb) 2021;2:100141. [Crossref] [PubMed]
- Liberzon A, Birger C, Thorvaldsdóttir H, et al. The Molecular Signatures Database (MSigDB) hallmark gene set collection. Cell Syst 2015;1:417-25. [Crossref] [PubMed]
- Lazarevic I, Soldati S, Mapunda JA, et al. The choroid plexus acts as an immune cell reservoir and brain entry site in experimental autoimmune encephalomyelitis. Fluids Barriers CNS 2023;20:39. [Crossref] [PubMed]
- Ten A, Kumeiko V, Farniev V, et al. Tumor Microenvironment Modulation by Cancer-Derived Extracellular Vesicles. Cells 2024;13:682. [Crossref] [PubMed]
- de Visser KE, Joyce JA. The evolving tumor microenvironment: From cancer initiation to metastatic outgrowth. Cancer Cell 2023;41:374-403. [Crossref] [PubMed]
- Desbois M, Wang Y. Cancer-associated fibroblasts: Key players in shaping the tumor immune microenvironment. Immunol Rev 2021;302:241-58. [Crossref] [PubMed]
- Gou Z, Li J, Liu J, et al. The hidden messengers: cancer associated fibroblasts-derived exosomal miRNAs as key regulators of cancer malignancy. Front Cell Dev Biol 2024;12:1378302. [Crossref] [PubMed]
- Ershaid N, Sharon Y, Doron H, et al. NLRP3 inflammasome in fibroblasts links tissue damage with inflammation in breast cancer progression and metastasis. Nat Commun 2019;10:4375. [Crossref] [PubMed]
- Zhao L, Ji G, Le X, et al. Long Noncoding RNA LINC00092 Acts in Cancer-Associated Fibroblasts to Drive Glycolysis and Progression of Ovarian Cancer. Cancer Res 2017;77:1369-82. [Crossref] [PubMed]
- Wang R, Huang Y, He J, et al. The endoplasmic reticulum stress-related genes and molecular typing predicts prognosis and reveals characterization of tumor immune microenvironment in lung squamous cell carcinoma. Discov Oncol 2024;15:37. [Crossref] [PubMed]
- Huang R, Li G, Wang K, et al. Comprehensive Analysis of the Clinical and Biological Significances of Endoplasmic Reticulum Stress in Diffuse Gliomas. Front Cell Dev Biol 2021;9:619396. [Crossref] [PubMed]
- Bui S, Mejia I, Díaz B, et al. Adaptation of the Golgi Apparatus in Cancer Cell Invasion and Metastasis. Front Cell Dev Biol 2021;9:806482. [Crossref] [PubMed]
- Bajaj R, Warner AN, Fradette JF, et al. Dance of The Golgi: Understanding Golgi Dynamics in Cancer Metastasis. Cells 2022;11:1484. [Crossref] [PubMed]
- Ahmadi SE, Rahimi S, Zarandi B, et al. MYC: a multipurpose oncogene with prognostic and therapeutic implications in blood malignancies. J Hematol Oncol 2021;14:121. [Crossref] [PubMed]
- Datta A, Deng S, Gopal V, et al. Cytoskeletal Dynamics in Epithelial-Mesenchymal Transition: Insights into Therapeutic Targets for Cancer Metastasis. Cancers (Basel) 2021;13:1882. [Crossref] [PubMed]
- Lai X, Li Q, Wu F, et al. Epithelial-Mesenchymal Transition and Metabolic Switching in Cancer: Lessons From Somatic Cell Reprogramming. Front Cell Dev Biol 2020;8:760. [Crossref] [PubMed]
- Oshi M, Patel A, Le L, et al. G2M checkpoint pathway alone is associated with drug response and survival among cell proliferation-related pathways in pancreatic cancer. Am J Cancer Res 2021;11:3070-84. [PubMed]
- Xie D, Pei Q, Li J, et al. Emerging Role of E2F Family in Cancer Stem Cells. Front Oncol 2021;11:723137. [Crossref] [PubMed]
- Chen PS, Chiu WT, Hsu PL, et al. Pathophysiological implications of hypoxia in human diseases. J Biomed Sci 2020;27:63. [Crossref] [PubMed]
- Chelakkot C, Chelakkot VS, Shin Y, et al. Modulating Glycolysis to Improve Cancer Therapy. Int J Mol Sci 2023;24:2606. [Crossref] [PubMed]
- Hosios AM, Manning BD. Cancer Signaling Drives Cancer Metabolism: AKT and the Warburg Effect. Cancer Res 2021;81:4896-8. [Crossref] [PubMed]
- Singh S, Kumar S, Srivastava RK, et al. Loss of ELF5-FBXW7 stabilizes IFNGR1 to promote the growth and metastasis of triple-negative breast cancer through interferon-γ signalling. Nat Cell Biol. 2020;22:591-602. Erratum in: Nat Cell Biol 2021;23:1048. [Crossref] [PubMed]
- Kakuta S, Yamaguchi J, Suzuki C, et al. Small GTPase Rab1B is associated with ATG9A vesicles and regulates autophagosome formation. FASEB J 2017;31:3757-73. [Crossref] [PubMed]
- Evans AS, Lennemann NJ, Coyne CB. BPIFB3 interacts with ARFGAP1 and TMED9 to regulate non-canonical autophagy and RNA virus infection. J Cell Sci 2021;134:jcs251835. [PubMed]