Construction and validation of senescence risk score signature as a novel biomarker in liver hepatocellular carcinoma: a bioinformatic analysis
Highlight box
Key findings
• In this research, the senescence risk score signature (SRSS) model was constructed and validated as an independent prognostic biomarker for liver hepatocellular carcinoma (LIHC).
What is known and what is new?
• Liver cancer is one of the most frequent fatal malignancies and patients are often diagnosed in advanced stages, contributing to its poor prognosis. Precise biomarkers and more treatment options are desperately needed for liver cancer at an early stage. Moreover, cellular senescence plays a critical role in tumorigenesis when it arises within incipient tumor cells versus stromal cells.
• Comprehensive analyses have selected three senescence-related genes (IGFBP3, SOCS2, and RACGAP1) as the SRSS model which is a novel prognostic biomarker for LIHC.
What is the implication, and what should change now?
• Early diagnosis and prognosis of LIHC could count on the SRSS model by further research.
• New treatments could be developed through the mechanism of cellular senescence in LIHC.
Introduction
Background
As the most frequent fatal malignancy, liver cancer mainly occurs with the risk factor of smoking, cirrhosis, hepatitis B or C virus infection, or nonalcoholic steatohepatitis (1,2). Regrettably, most liver cancers cannot be caught at an early stage, resulting in poor patient outcomes (3). Moreover, the incidence of liver cancer is still rising while the treatments are still limited and not effective enough (3,4). With the booming advancement of the medical realm, numerous treatment options are provided for patients with liver cancer, including surgical resection, local destructive therapies, liver therapy, transplantation, percutaneous ablation, radiation, and transarterial and systemic therapies (5,6). However, the therapeutic effect, survival benefits, and recurrence of liver cancer remain the major problems after those grueling treatments (6,7). In a word, the high mortality rates of liver hepatocellular carcinoma (LIHC) mainly arise from delayed diagnosis, limited accuracy of diagnostic and prognostic biomarkers, and shortage of precise treatment. Therefore, robust biomarkers are desperately needed to accurately diagnose, and forecast patients with LIHC and provide promising therapeutic targets.
While well-known biomarkers like alpha-fetoprotein (AFP), identified 60 years ago, have been used to predict LIHC prognosis and recurrence after liver transplant (8), recent years have witnessed extensive research on treatments such as AFP vaccine and AFP-specific adoptive T-cell transfer for LIHC management (9). However, alternative potential solutions need to be sought with an open-minded approach. Emerging discoveries have highlighted GINS3 (10), and microRNAs signature (11), among other potential biomarkers for LIHC diagnosis and prognosis. Additionally, the considerable role of senescence-related variables in cancers has emerged from recent research (12,13).
Cellular senescence which is not synonymous with aging, is a stress response state in the case of exposure to genotoxic agents, nutrient deprivation, hypoxia, and oncogene activation (14,15). The response could elicit a permanent cell cycle arrest and trigger profound phenotypic changes (16). Notably, senescence is critical in tumorigenesis when it arises within incipient tumor cells versus stromal cells (17). Due to the non-negligibility of senescence, efforts have been made to investigate its innate correlation with cancers (18,19). Moreover, research has concluded that the liver has a unique regenerative capacity in response to a damage event, which is highly associated with senescence (20). The senescence program involves in tumor immune surveillance and the error of this program may facilitate the progression of pre-malignant senescent hepatocytes to LIHC (21,22). Meanwhile, it is unrealistic to directly incorporate senescence-related genes (SRGs) into the LIHC prognostic prediction due to the necessity and complexity of revalidating robust clinical samples. Consequently, given that senescence affects tumor progression and responses to treatment, the senescence risk score signature (SRSS) model could offer enhanced prognostic assessment compared to traditional biomarkers.
Despite the critical role of senescence in LIHC, few studies have unveiled and established a senescence-related model to improve diagnostic and prognostic accuracy. Hence, this study aims to ascertain and construct a novel SRSS model to predict the LIHC prognosis effectively and identify promising therapeutic targets. We present this article in accordance with the TRIPOD reporting checklist (available at https://tcr.amegroups.com/article/view/10.21037/tcr-23-2373/rc).
Methods
Datasets and data preprocessing
The gene expression profiles of GSE22405 (https://cdn.amegroups.cn/static/public/tcr-23-2373-1.xlsx) and GSE57957 (https://cdn.amegroups.cn/static/public/tcr-23-2373-2.xlsx) were collected from the Gene Expression Omnibus (GEO) database (https://www.ncbi.nlm.nih.gov/). The GSE22405 dataset contained 24 non-tumor and 24 LIHC samples. The GSE57957 dataset contained 39 non-tumor and 39 LIHC samples. Moreover, SRGs were directly obtained from the Human Ageing Genomic Resources (HAGR) (https://genomics.senescence.info/cells/) (https://cdn.amegroups.cn/static/public/tcr-23-2373-3.xlsx). The training set was selected after overlapping and differential analysis of the above datasets.
The datasets of LIHC and pancreatic adenocarcinoma (PAAD) from The Cancer Genome Atlas (TCGA) comprising gene expression information, clinical characteristics, and survival materials, were downloaded as the validation sets from Genomic Data Commons (GDC) Data Portal (https://portal.gdc.cancer.gov/). A total of 424 LIHC RNA sequencing (RNAseq) constitute by 377 tumor samples and 50 adjacent-tumor samples. Meanwhile, PAAD samples were obtained with 183 RNAseq from 185 tumor samples and 4 adjacent-tumor samples. Based on the model, the above datasets from TCGA were recalculated and employed as the validation sets.
The study was conducted in accordance with the Declaration of Helsinki (as revised in 2013).
Differential analysis
To ascertain differentially expressed genes (DEGs) in the tumor and non-tumor groups, a differential expression analysis was first conducted based on the expressed genes in LIHC. The DEGs were collected by the “DESeq2” software package in R and log2|fold change (FC)| >1 and the adjusted P value <0.05 was set as the criterion. Therefore, DEGs were selected and visualized in the volcano map by the “ggplot2” package in the R.
Identification of senescence-related DEGs
To discriminate differentially expressed senescence genes (DESGs) in LIHC, DEGs, and SRGs were overlapped and visualized in the Venn diagram by the “ggplot2” package in the R. The “survival” software package in R was used to analyze the prognosis of LIHC. Fourteen DESGs related to the prognosis, which are NUSAP1, CXCL12, IGFBP3, CAP2, TOP2A, CDKN3, ASPM, RND3, PRC1, EGR1, SOCS2, RACGAP1, FST, and H2AFZ, were initially screened for genes mutual to the three datasets.
Enrichment analysis
To determine the underlying functions and pathways of DEGs, the “clusterProfilter” R package was utilized to perform Gene Ontology (GO) and Kyoto Encyclopedia of Genes and Genomes (KEGG) analyses. The GO database is a powerful bioinformatics tool to explore functions of genes in three classifications: biological processes (BPs), cellular components (CCs), and molecular functions (MFs). The KEGG is a comprehensive database that can be roughly divided into three categories: systematic information, genomic information, and chemical information. The adjusted P value <0.05 was considered statistically significant.
Construction of the prognostic model
The prognostic DESGs of LIHC were obtained by Cox regression analysis using “survival” software package in R. Then, three noteworthy prognosis-related senescence genes (IGFBP3, SOCS2, and RACGAP1) were discovered by the least absolute shrinkage and selection operator (LASSO) regression analysis using 10-fold cross-validation to procure independent prognostic genes. Furthermore, regression coefficients of independent prognostic factors were procured by multivariate Cox regression analysis. Eventually, the prognostic model was constructed as senescence risk scoring signature (SRSS) = EXPgene1*β1 + EXPgene2*β2 + EXPgene3*β3 + … + EXPgenen*βn, where EXP represents expression level and β represents the regression coefficient from the multivariate Cox.
Validation of the prognostic value of SRSS
First, the risk score of TCGA-LIHC was assessed via the Kaplan-Meier (KM) plotter and then patients were divided into low- and high-risk groups based on the survival median as the cut-off value. To compare the overall survival (OS) of patients among two groups, after statistical differences were confirmed by Log-rank test and Cox regression, we visualized the KM curves using the “survminer” package in R. And then to validate the prognostic efficiency, time-dependent receiver operating characteristic (ROC) curves were investigated by the “timeROC” package and visualized by the “ggplot2” package in R. The area under the ROC curve (AUC) is a probability value, ranging from 0.5 to −1, which is used to evaluate the accuracy of model prediction. Generally, when the value of AUC is greater than 0.7 and closer to 1, it is considered a good prediction model.
Predictive accuracy and benefit of the nomogram
In addition, the model was visualized as a nomogram for predicting the occurrence of events, and the probability of the individual occurrence time was assessed by the scores of each index in the model of independent individuals with external data, using the “rms” package. Subsequently, the effect of clinical variables on prognosis such as tumor stage, gender, age, and risk score of SRSS, was evaluated by performing univariate Cox regression analyses, and multivariate Cox regression analyses were conducted to ascertain whether a variable is an independent prognostic indicator. The P value <0.05 was considered statistically significant.
The tumor stage was established based on the seventh tumor node metastasis (TNM) classification of the American Joint Committee on Cancer (23).
Meanwhile, to evaluate the function of the established model in clinical benefit, the 1-, 3-, and 5-year decision curve analyses (DCA) were conducted. The x-axis is the threshold probability, and the y-axis is net income. The “survival” and “stdca. R” packages were utilized. Furthermore, the concordance index (C-index) ranging from 0.5 to −1, could be used to evaluate the predictive accuracy of traditional TNM-stage, nomogram, and SRSS. The closer the confidence interval (CI) value is to 1, the better the model is.
Validation of SRSS model in PAAD
Liver cancer and pancreatic cancer were both digestive gland tumors, and metastases are commonly detected in PAAD (24). Considering the histological similarities, degree of malignancy, and intimate connection with the liver, TCGA-PAAD (n=183) was utilized to further substantiate the SRSS model and its general applicability in other tumors. Therefore, the risk scores of patients with PAAD were recalculated using the SRSS formula and then the samples were distributed into low- and high-risk groups based on the survival median as the cut-off value. The survival differences and the accuracy of SRSS in PAAD were evaluated through KM-plotter and ROC curves. Similarly, a nomogram integrating the risk score of SRSS with clinical characteristics such as tumor stage, status, race, and age, was employed to further comprehensively determine the probability of survival in PAAD patients. Furthermore, the DCA diagrams were constructed for 1-, 3-, and 5-year survival to confirm the accuracy and clinical benefit of nomogram in PAAD.
Statistical analysis
Most statistical analyses were performed using R software (version 3.6.3). Excel software was utilized for the simple mathematical analysis and processing. A P value less than 0.05 was considered statistically significant.
Results
Analysis and identification of DEGs
To perform differential expression analysis, GSE22405 and GSE57957 were utilized as the training set. Based on the screening criteria: |log2FC| >1 and P<0.05, a total of 196 and 421 DEGs were identified respectively in LIHC (https://cdn.amegroups.cn/static/public/tcr-23-2373-4.xlsx, https://cdn.amegroups.cn/static/public/tcr-23-2373-5.xlsx) were selected and visualized in the volcano map (Figure 1A,1B). Then DEGs were overlapped with SRGs and then 14 DESGs were procured (Figure 1C, https://cdn.amegroups.cn/static/public/tcr-23-2373-6.xlsx). The results suggested that the DESGs might somehow participate in the progression of LIHC and it was worth further research.

Enrichment analyses
Enrichment analyses were conducted to ascertain the underlying biological function of DEGs in LIHC, including GO and KEGG analyses. As shown in Figure 2A-2C, the noteworthy terms of BP, MF, and CC (2) enrichment analyses were demonstrated. Interestingly, DEGs were enriched in cell proliferation, such as organelle fission, nuclear division, microtubule, tubulin binding, and so on. Moreover, according to the KEGG pathway analysis, DEGs were involved in growth hormone synthesis, secretion, and action pathways in LIHC (Figure 2D). Those biological enrichment analyses showed that DEGs could regulate the process of cell proliferation and are further associated with the development of cancer cells.
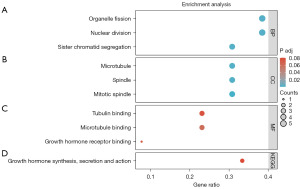
Establishment and prognostic value of SRSS
To discover the underlying senescence genes that could serve as effective biomarkers, univariate and multivariate Cox regression analyses were conducted on the 14 DESGs (Table 1). However, due to insufficient information on H2AFZ, the Cox analyses were undertaken eventually on the other 13 DESGs presented in Table 1. Subsequently, to establish the model of SRSS, 7 DESGs were employed for LASSO regression analysis. As shown in Figure 3A,3B, when the penalty coefficient was 3 the model fit the best, and the equivalent three senescence genes (IGFBP3, SOCS2, and RACGAP1) were adequate for establishing the model (https://cdn.amegroups.cn/static/public/tcr-23-2373-6.xlsx). The heat map, survival status, and risk score of three senescence genes in patients with LIHC also confirmed the prognostic value of SRSS (Figure 3C). Moreover, univariate and multivariate Cox regression analyses were conducted on three senescence genes, and the corresponding regression coefficients were obtained, β1–β3, which were 0.11674, −0.36518, and 0.29059, respectively. Based on the above results, the model was established as follows:
Table 1
Genes | Univariate analysis | Multivariate analysis | |||
---|---|---|---|---|---|
Hazard ratio (95% CI) | P value | Hazard ratio (95% CI) | P value | ||
NUSAP1 | 0.747 (0.221–2.529) | 0.64 | |||
CXCL12 | 0.957 (0.859–1.067) | 0.43 | |||
IGFBP3 | 1.178 (1.048–1.326) | 0.006 | 1.127 (1.002–1.268) | 0.046 | |
CAP2 | 1.053 (0.921–1.205) | 0.45 | |||
TOP2A | 1.229 (1.103–1.368) | <0.001 | 1.036 (0.766–1.400) | 0.82 | |
CDKN3 | 1.253 (1.103–1.423) | <0.001 | 0.893 (0.689–1.158) | 0.39 | |
ASPM | 1.291 (1.123–1.483) | <0.001 | 1.002 (0.722–1.391) | 0.99 | |
RND3 | 1.091 (0.945–1.261) | 0.24 | |||
PRC1 | 4.842 (1.111–21.109) | 0.04 | 0.492 (0.069–3.519) | 0.48 | |
EGR1 | 1.010 (0.908–1.123) | 0.86 | |||
SOCS2 | 0.688 (0.584–0.810) | <0.001 | 0.680 (0.574–0.806) | <0.001 | |
RACGAP1 | 1.403 (1.199–1.641) | <0.001 | 1.485 (1.027–2.146) | 0.04 | |
FST | 1.004 (0.910–1.107) | 0.94 |
DESGs, differentially expressed senescence genes; LIHC, liver hepatocellular carcinoma; CI, confidence interval.
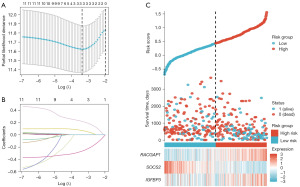
SRSS = EXP IGFBP3*0.11674 + EXP SOCS2*−0.36518 + EXP RACGAP1*0.29059 (EXP: expression level of gene).
Validation of SRSS in LIHC clinical prediction
According to the established formula, the risk score of each patient was calculated directly, and then the high- and low-risk groups were divided based on the median and interquartile range. The KM curve (Figure 4A) showed a worse prognosis [P<0.001, hazard ratio (HR) =2.08, 95% CI: 1.46–2.96] in the high-risk group. Meanwhile, the AUC values of 1-, 3-, and 5-year ROC curves were 0.779, 0.726, and 0.673, respectively (Figure 4B). Moreover, the AUC values of different combinations were also investigated (Table 2). The above results preliminarily indicated SRSS as a novel and promising prognostic biomarker in LIHC.
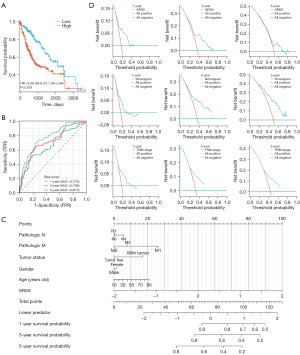
Table 2
Gene combination | AUC value | ||
---|---|---|---|
1 year | 3 years | 5 years | |
IGFBP3 | 0.619 | 0.654 | 0.542 |
SOCS2 | 0.320 | 0.344 | 0.344 |
RACGAP1 | 0.732 | 0.654 | 0.600 |
IGFBP3 + SOCS2 | 0.710 | 0.707 | 0.665 |
IGFBP3 + RACGAP1 | 0.733 | 0.693 | 0.603 |
SOCS2 + RACGAP1 | 0.780 | 0.709 | 0.670 |
IGFBP3 + SOCS2 + RACGAP1 | 0.779 | 0.726 | 0.673 |
AUC, area under the curve.
To determine the prognostic significance of SRSS, further univariate and multivariate Cox regression analyses were conducted for clinicopathological features of LIHC associated with OS (Table 3). Results substantiated that age, tumor status, tumor stage, and risk score were prognostic predictors in TCGA-LIHC, but not gender and race. Significantly, in multivariate Cox regression analysis, the only independent predictor was the risk score. The above results further supported SRSS as a novel and credible biomarker for predicting prognosis in LIHC.
Table 3
Characteristics | Total (N) | Univariate analysis | Multivariate analysis | |||
---|---|---|---|---|---|---|
Hazard ratio (95% CI) | P value | Hazard ratio (95% CI) | P value | |||
Age | 368 | 1.012 (0.998–1.026) | 0.09 | 1.013 (0.998–1.029) | 0.10 | |
Gender | 368 | |||||
Male | 249 | Reference | ||||
Female | 119 | 1.261 (0.885–1.796) | 0.20 | |||
Race | 357 | |||||
White | 183 | Reference | ||||
Black or African-American | 17 | 1.198 (0.520–2.760) | 0.67 | |||
Asian | 157 | 0.756 (0.519–1.101) | 0.14 | |||
Tumor status | 350 | |||||
With tumor | 151 | Reference | Reference | |||
Tumor free | 199 | 0.432 (0.296–0.629) | <0.001 | 0.587 (0.389–0.886) | 0.01 | |
Pathologic stage | 344 | |||||
Stage I | 172 | Reference | Reference | |||
Stage II | 85 | 1.417 (0.868–2.312) | 0.16 | 1.093 (0.645–1.850) | 0.74 | |
Stage III | 83 | 2.734 (1.792–4.172) | <0.001 | 1.831 (1.145–2.927) | 0.01 | |
Stage IV | 4 | 5.597 (1.726–18.148) | 0.004 | 3.316 (0.782–14.066) | 0.10 | |
Pathologic N | 367 | |||||
NX | 113 | Reference | Reference | |||
N0 | 250 | 0.676 (0.467–0.980) | 0.04 | 0.713 (0.451–1.125) | 0.15 | |
N1 | 4 | 1.466 (0.355–6.062) | 0.60 | 0.535 (0.121–2.368) | 0.41 | |
Risk score | 368 | 3.959 (2.642–5.933) | <0.001 | 3.226 (2.044–5.092) | <0.001 |
LIHC, liver hepatocellular carcinoma; OS, overall survival; CI, confidence interval.
Simultaneously, the nomogram was made as a scale to visualize the model for calculating and predicting the prognosis of patients with LIHC (Figure 4C). As shown in Table 4, the C-index of TNM-stage, SRSS, and nomogram were in order of 0.639, 0.696, and 0.686. In conclusion, the predictive ability of SRSS was strongest, followed by the nomogram combined with multiple clinical information and the traditional TNM stage was the weakest. Furthermore, the decision curve analysis (DCA) figures showed that the nomogram integrating multiple clinical information has a better clinical application value, which was also consistent with the above result (Figure 4D). The results supported that the value of clinical application was better with SRSS.
Table 4
Cohorts | Variables | C-index (95% CI) |
---|---|---|
LIHC | TNM-stage | 0.639 (0.607–0.671) |
SRSS | 0.696 (0.672–0.720) | |
Nomogram | 0.686 (0.660–0.712) | |
PAAD | TNM-stage | 0.561 (0.535–0.587) |
SRSS | 0.657 (0.628–0.687) | |
Nomogram | 0.694 (0.662–0.727) |
C-index, concordance index; TNM, tumor node metastasis; SRSS, senescence risk score signature; CI, confidence interval; LIHC, liver hepatocellular carcinoma; PAAD, pancreatic adenocarcinoma.
Validation of SRSS in PAAD
In consideration of the innate connection with LIHC, TCGA-PAAD was utilized as the external validation set, and risk scores for each patient in the PAAD cohort were calculated according to the established SRSS formula. The KM curve showed a worse prognosis in the high-risk group which is consistent with the result of LIHC (Figure 5A). The AUC of the 1-, 3-, and 5-year survival ROC curves (0.697, 0.776, and 0.816 respectively) demonstrated a high level of consistency in predicting OS (Figure 5B). Moreover, the predictive nomogram of PAAD was constructed utilizing clinical features and SRSS (Figure 5C). The C-index values (Table 4) and the DCA figures (Figure 5D) demonstrated that the predictive ability of SRSS was weaker than the comprehensive nomogram in PAAD but stronger than traditional TNM-stage.
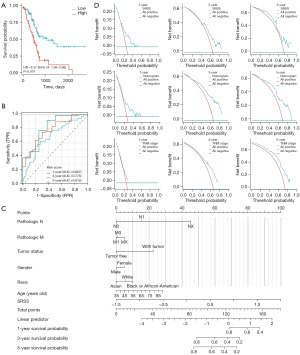
Discussion
LIHC, not only is the most frequent primary liver cancer but also the fourth most common cause of cancer-related death (1,25). Meanwhile, cellular senescence plays a pivotal role in liver homeostasis, disease, and regeneration (26). Currently, there are deficient indexes for early diagnosis, accurate prognosis, and more effective treatments for patients with LIHC (27). Therefore, more and more researchers have made efforts to search underlying biomarkers for precise prognoses and treatments, however, not adequate biomarkers have been found (28-30). As cellular senescence is considered to be a promising strategy for liver diseases including cancer (31,32), hence, we involved ourselves in constructing and validating a SRSS model of LIHC for early diagnosis, prognostic prediction, and providing a promising therapeutic target.
Firstly, to investigate the innate connection between LIHC and the senescence mechanism, GSE22405 and GSE57957 were selected as the training sets. Differential analysis was then conducted to identify 196 and 421 DEGs and 14 DESGs. Following this, DEGs were employed for enrichment analyses. Results showed that those DEGs were significantly enriched in cell proliferation, such as organelle fission, nuclear division, microtubule, tubulin binding, and so on. As exhilarating as the results are, cell proliferation has already been widely known to involve in multiple BPs including tumor development (33,34). Moreover, sustained proliferation is a common property of human cancer (35). The results shed light on the possibility of constructing senescence-related biomarkers in LIHC.
Consequently, among DEGs, three senescence genes, including IGFBP3, SOCS2, and RACGAP1, were identified to be strongly related to prognosis in LIHC based on Cox regression analysis and lasso regression analysis. After preliminary verification of prognostic value in LIHC, the SRSS model was constructed for efficient diagnosis and predicting prognosis. IGFBP3, a kind of protein with pleiotropic ability, could regulate cell proliferation, apoptosis, and differentiation (36,37). Moreover, researchers have found IGFBP3 upregulation in the senescent cells (38). As for SOCS2, it could enhance the efficiency of liver cancer radiotherapy by promoting ferroptosis and improving the prognosis of patients (39). Notably, Yang et al. discovered that overexpressed RACGAP1 promoted the proliferation of LIHC cells by reducing the activation of the Hippo and YAP pathways (40). In conclusion, the function of those three promising biomarkers is consistent with the results of the enrichment analysis, which showed that it could regulate the proliferation of cancer cells in a certain mechanism. Therefore, we hypothesized that the established SRSS model might be a robust biomarker in LIHC and more validation is as follows.
The KM curve showed a worse prognosis in the high-risk group. Meanwhile, the time-dependent ROC curve demonstrated that the prognostic prediction of the SRSS model was in good consistency with the actual results. Furthermore, the risk score of SRSS integrating with clinicopathological features of LIHC was analyzed via univariate and multivariate Cox regression analysis and the result supported the risk score of SRSS as an independent prognostic biomarker in LIHC. For further comprehensive evaluation of the prognosis, the survival probability was scored individually through the nomogram with multiple clinical information and risk scores. Consequently, the C-index and DCA figures thoroughly proved that the predictive ability of SRSS was stronger than the nomogram combined with multiple clinical information and traditional TNM-stage.
As another advanced cancer of the digestive system, PAAD is known for its capability of invasion and metastasis with a high fatality rate (41,42). In consideration of the histological similarities, biological function, pathogenesis, and other intimate connections with the liver (43-45), PAAD was selected as the validation set to further evaluate the SRSS. Based on the data from TCGA-PAAD, the results of the KM curve, ROC curve, nomogram, calibration, and DCA diagram were consistent with the above results.
Conclusions
In this study, three SRGs were screened out after exploring the underlying association with LIHC. The SRSS model based on the three SRGs was constructed and validated to be a novel and promising prognostic model independently for LIHC. Hopefully, groundbreaking treatments will be brought out by targeting this novel biomarker of LIHC.
Acknowledgments
Funding: This study was supported by grants from
Footnote
Reporting Checklist: The authors have completed the TRIPOD reporting checklist. Available at https://tcr.amegroups.com/article/view/10.21037/tcr-23-2373/rc
Peer Review File: Available at https://tcr.amegroups.com/article/view/10.21037/tcr-23-2373/prf
Conflicts of Interest: All authors have completed the ICMJE uniform disclosure form (available at https://tcr.amegroups.com/article/view/10.21037/tcr-23-2373/coif). All authors report that this study was supported by grants from the Medical Scientific Research Foundation of Guangdong Province (No. A2021091 to J.L.), the Fundamental Research Funds for the Central Universities (No. 21622312 to J.L.), the Basic and Applied Basic Research Project of Guangzhou Basic Research Program (No. 2023A04J1917 to J.L.), the Flagship Specialty Construction Project-General Surgery of the First Affiliated Hospital of the Jinan University (No. 711003 to J.L.), the Special Foundation for Scientific Research Development of the Affiliated Shunde Hospital of Jinan University (No. 202101004 to Y.H.), and Guangdong Basic and Applied Research Foundation (No. 2021A1515010259, 2022A1515012581 to J.S.). The authors have no other conflicts of interest to declare.
Ethical Statement: The authors are accountable for all aspects of the work in ensuring that questions related to the accuracy or integrity of any part of the work are appropriately investigated and resolved. The study was performed in accordance with the Declaration of Helsinki (as revised in 2013).
Open Access Statement: This is an Open Access article distributed in accordance with the Creative Commons Attribution-NonCommercial-NoDerivs 4.0 International License (CC BY-NC-ND 4.0), which permits the non-commercial replication and distribution of the article with the strict proviso that no changes or edits are made and the original work is properly cited (including links to both the formal publication through the relevant DOI and the license). See: https://creativecommons.org/licenses/by-nc-nd/4.0/.
References
- Villanueva A. Hepatocellular Carcinoma. N Engl J Med 2019;380:1450-62. [Crossref] [PubMed]
- Sung H, Ferlay J, Siegel RL, et al. Global Cancer Statistics 2020: GLOBOCAN Estimates of Incidence and Mortality Worldwide for 36 Cancers in 185 Countries. CA Cancer J Clin 2021;71:209-49. [Crossref] [PubMed]
- Gravitz L. Liver cancer. Nature 2014;516:S1. [Crossref] [PubMed]
- Anwanwan D, Singh SK, Singh S, et al. Challenges in liver cancer and possible treatment approaches. Biochim Biophys Acta Rev Cancer 2020;1873:188314. [Crossref] [PubMed]
- Vogel A, Meyer T, Sapisochin G, et al. Hepatocellular carcinoma. Lancet 2022;400:1345-62. [Crossref] [PubMed]
- Liu CY, Chen KF, Chen PJ. Treatment of Liver Cancer. Cold Spring Harb Perspect Med 2015;5:a021535. [Crossref] [PubMed]
- Verslype C, Van Cutsem E, Dicato M, et al. The management of hepatocellular carcinoma. Current expert opinion and recommendations derived from the 10th World Congress on Gastrointestinal Cancer, Barcelona, 2008. Ann Oncol 2009;20:vii1-6. [Crossref] [PubMed]
- Norman JS, Li PJ, Kotwani P, et al. AFP-L3 and DCP strongly predict early hepatocellular carcinoma recurrence after liver transplantation. J Hepatol 2023;79:1469-77. [Crossref] [PubMed]
- Hu X, Chen R, Wei Q, et al. The Landscape Of Alpha Fetoprotein In Hepatocellular Carcinoma: Where Are We? Int J Biol Sci 2022;18:536-51. [Crossref] [PubMed]
- Lai T, Peng T, Li J, et al. A novel prognostic biomarker: GINS3 is correlated with methylation and immune escape in liver hepatocellular carcinoma. Transl Cancer Res 2023;12:1145-64. [Crossref] [PubMed]
- Ghafouri-Fard S, Honarmand Tamizkar K, Hussen BM, et al. MicroRNA signature in liver cancer. Pathol Res Pract 2021;219:153369. [Crossref] [PubMed]
- Huang E, Ma T, Zhou J, et al. A novel senescence-associated LncRNA signature predicts the prognosis and tumor microenvironment of patients with colorectal cancer: a bioinformatics analysis. J Gastrointest Oncol 2022;13:1842-63. [Crossref] [PubMed]
- Wang G, Mao Z, Zhou X, et al. Construction and validation of a novel prognostic model using the cellular senescence-associated long non-coding RNA in gastric cancer: a biological analysis. J Gastrointest Oncol 2022;13:1640-55. [Crossref] [PubMed]
- Gorgoulis V, Adams PD, Alimonti A, et al. Cellular Senescence: Defining a Path Forward. Cell 2019;179:813-27. [Crossref] [PubMed]
- Sharpless NE, Sherr CJ. Forging a signature of in vivo senescence. Nat Rev Cancer 2015;15:397-408. [Crossref] [PubMed]
- Birch J, Gil J. Senescence and the SASP: many therapeutic avenues. Genes Dev 2020;34:1565-76. [Crossref] [PubMed]
- Faget DV, Ren Q, Stewart SA. Unmasking senescence: context-dependent effects of SASP in cancer. Nat Rev Cancer 2019;19:439-53. [Crossref] [PubMed]
- Loo TM, Miyata K, Tanaka Y, et al. Cellular senescence and senescence-associated secretory phenotype via the cGAS-STING signaling pathway in cancer. Cancer Sci 2020;111:304-11. [Crossref] [PubMed]
- Rayess H, Wang MB, Srivatsan ES. Cellular senescence and tumor suppressor gene p16. Int J Cancer 2012;130:1715-25. [Crossref] [PubMed]
- Ramboer E, De Craene B, De Kock J, et al. Strategies for immortalization of primary hepatocytes. J Hepatol 2014;61:925-43. [Crossref] [PubMed]
- Eggert T, Wolter K, Ji J, et al. Distinct Functions of Senescence-Associated Immune Responses in Liver Tumor Surveillance and Tumor Progression. Cancer Cell 2016;30:533-47. [Crossref] [PubMed]
- Kang TW, Yevsa T, Woller N, et al. Senescence surveillance of pre-malignant hepatocytes limits liver cancer development. Nature 2011;479:547-51. [Crossref] [PubMed]
- Amin MB, Greene FL, Edge SB, et al. The Eighth Edition AJCC Cancer Staging Manual: Continuing to build a bridge from a population-based to a more "personalized" approach to cancer staging. CA Cancer J Clin 2017;67:93-9.
- Tsilimigras DI, Brodt P, Clavien PA, et al. Liver metastases. Nat Rev Dis Primers 2021;7:27. [Crossref] [PubMed]
- Forner A, Reig M, Bruix J. Hepatocellular carcinoma. Lancet 2018;391:1301-14. [Crossref] [PubMed]
- Ferreira-Gonzalez S, Rodrigo-Torres D, Gadd VL, et al. Cellular Senescence in Liver Disease and Regeneration. Semin Liver Dis 2021;41:50-66. [Crossref] [PubMed]
- Piñero F, Dirchwolf M, Pessôa MG. Biomarkers in Hepatocellular Carcinoma: Diagnosis, Prognosis and Treatment Response Assessment. Cells 2020;9:1370. [Crossref] [PubMed]
- Liu Z, Yang D, Li Y, et al. HN1 as a diagnostic and prognostic biomarker for liver cancer. Biosci Rep 2020;40:BSR20200316. [Crossref] [PubMed]
- Gao YX, Yang TW, Yin JM, et al. Progress and prospects of biomarkers in primary liver cancer Int J Oncol 2020;57:54-66. (Review). [Crossref] [PubMed]
- Gao S, Gang J, Yu M, et al. Computational analysis for identification of early diagnostic biomarkers and prognostic biomarkers of liver cancer based on GEO and TCGA databases and studies on pathways and biological functions affecting the survival time of liver cancer. BMC Cancer 2021;21:791. [Crossref] [PubMed]
- Papatheodoridi AM, Chrysavgis L, Koutsilieris M, et al. The Role of Senescence in the Development of Nonalcoholic Fatty Liver Disease and Progression to Nonalcoholic Steatohepatitis. Hepatology 2020;71:363-74. [Crossref] [PubMed]
- Kim YH, Park TJ. Cellular senescence in cancer. BMB Rep 2019;52:42-6. [Crossref] [PubMed]
- DeBerardinis RJ, Lum JJ, Hatzivassiliou G, et al. The biology of cancer: metabolic reprogramming fuels cell growth and proliferation. Cell Metab 2008;7:11-20. [Crossref] [PubMed]
- Kroemer G, Pouyssegur J. Tumor cell metabolism: cancer's Achilles' heel. Cancer Cell 2008;13:472-82. [Crossref] [PubMed]
- Macheret M, Halazonetis TD. DNA replication stress as a hallmark of cancer. Annu Rev Pathol 2015;10:425-48. [Crossref] [PubMed]
- Ushakov RE, Aksenov ND, Pugovkina NA, et al. Effects of IGFBP3 knockdown on human endometrial mesenchymal stromal cells stress-induced senescence. Biochem Biophys Res Commun 2021;570:143-7. [Crossref] [PubMed]
- Li M, Wu W, Deng S, et al. TRAIP modulates the IGFBP3/AKT pathway to enhance the invasion and proliferation of osteosarcoma by promoting KANK1 degradation. Cell Death Dis 2021;12:767. [Crossref] [PubMed]
- Vassilieva I, Kosheverova V, Vitte M, et al. Paracrine senescence of human endometrial mesenchymal stem cells: a role for the insulin-like growth factor binding protein 3. Aging (Albany NY) 2020;12:1987-2004. [Crossref] [PubMed]
- Chen Q, Zheng W, Guan J, et al. SOCS2-enhanced ubiquitination of SLC7A11 promotes ferroptosis and radiosensitization in hepatocellular carcinoma. Cell Death Differ 2023;30:137-51. [Crossref] [PubMed]
- Yang XM, Cao XY, He P, et al. Overexpression of Rac GTPase Activating Protein 1 Contributes to Proliferation of Cancer Cells by Reducing Hippo Signaling to Promote Cytokinesis. Gastroenterology 2018;155:1233-1249.e22. [Crossref] [PubMed]
- Wang S, Zheng Y, Yang F, et al. The molecular biology of pancreatic adenocarcinoma: translational challenges and clinical perspectives. Signal Transduct Target Ther 2021;6:249. [Crossref] [PubMed]
- Wood LD, Canto MI, Jaffee EM, et al. Pancreatic Cancer: Pathogenesis, Screening, Diagnosis, and Treatment. Gastroenterology 2022;163:386-402.e1. [Crossref] [PubMed]
- Zhang Y, Sun J, Song Y, et al. Roles of fusion genes in digestive system cancers: Dawn for cancer precision therapy. Crit Rev Oncol Hematol 2022;171:103622. [Crossref] [PubMed]
- Nielsen SR, Quaranta V, Linford A, et al. Macrophage-secreted granulin supports pancreatic cancer metastasis by inducing liver fibrosis. Nat Cell Biol 2016;18:549-60. [Crossref] [PubMed]
- Yachida S, Jones S, Bozic I, et al. Distant metastasis occurs late during the genetic evolution of pancreatic cancer. Nature 2010;467:1114-7. [Crossref] [PubMed]