A novel gene signature based on endoplasmic reticulum stress for predicting prognosis in hepatocellular carcinoma
Highlight box
Key findings
• A novel gene signature based on endoplasmic reticulum stress (ERS) is established and can predict hepatocellular carcinoma (HCC) prognosis.
• TMX1 can promote the malignant phenotype of HCC cells.
• BRSK2 can promote the apoptosis induced by ERS.
What is known and what is new?
• HCC remains one of the most common human cancers, the death cases induced by HCC are increasing these years. It is reported that ERS plays a crucial role in the pathogenesis of HCC. Moreover, studies have shown that gene signature based on ERS can predict the prognosis of HCC.
• We constructed a new ERS-related gene signature to predict the prognosis of HCC, and we explored the biological functions of TMX1 and BRSK2.
What is the implication, and what should change now?
• Our study may provide a new understanding of relationship between ERS and HCC, and may supply a practical tool for clinicians.
• The biological functions of genes other than TMX1 and BRSK2 in our gene signature need to be further explored.
Introduction
Hepatocellular carcinoma (HCC) accounts for 75–85% of all primary liver cancer, which is the third leading reason for malignancy related death cases (1). The heterogeneity of HCC is very high, including three levels: interpatient heterogeneity, intertumoural heterogeneity and intratumoural heterogeneity (2). The prognosis of HCC patients varies significantly around the world (3). The high level of heterogeneity in HCC patients makes prognostic prediction challenging. So, it is necessary to construct effective and precise prognostic models for HCC patients to improve diagnosis and treatment.
Endoplasmic reticulum (ER) plays a very important role in protein synthesis and processing, lipid metabolism and calcium storage (4). A third of polypeptides transported to the ER fail to fold correctly (5), and endoplasmic reticulum stress (ERS) occurs when the misfolded proteins cannot be disposed of properly, which can activate the unfolded protein response (UPR). UPR contains three branches activated by three ER transmembrane proteins: IRE1α, PERK, ATF6 (6). ERS has very important effects on HCC including tumor growth, invasion, metastasis, angiogenesis, and drug resistance (7). Researchers have reported that ERS can activate TRIM25 in HCC, which promotes the growth of cancer cells (8). P4Hb and GRP78 are molecular chaperones included in ERS. Xia et al. found that P4Hb may accelerate the progression of HCC by downregulating GRP78 and upregulating epithelial-mesenchymal transition (EMT) under ERS (9). Liu et al. showed that ERS was activated in HCC cells treated with sorafenib. ERS-induced autophagy can lead to sorafenib resistance in HCC cell (10). Yet, few prognostic models based on ERS have been developed for HCC patients.
We constructed an ERS-related gene signature containing 9 genes. EXTL3 is located at chromosome 8p21.1 and take part in the signal transduction of islet-derived proteins, which play roles in stimulating the growth of islet β-cell, gut immune defenses, tissue regeneration (11). In the tumor microenvironment, CXCL8 is an essential proinflammatory chemokine (12). It was reported that ERS can promote the expression of CXCL8 in breast carcinomas (13). MAGEA3 has prognostic value in a variety of cancers and can affect cell migration, invasion, proliferation and cisplatin-induced apoptosis (14,15). TMX1, which is a transmembrane thiol isomerase, is the first family member shown to negatively regulate platelets (16). TPP1 is a vital component of the telomere-binding protein shelterin complex, protecting chromosome ends from cellular deoxyribonucleic acid (DNA) damage (17). BRSK2 can regulate ERS-nduced apoptosis (18), insulin secretion and β-cell biology (19). CREB3L1 is an ER-resident transmembrane transcription factor. This molecule is cleaved by regulated intramembrane proteolysis, followed by activation as a transcription factor (20). PIK3R1 encodes p85α subunit of PI3K enzymes and PIK3R1 is considered as a tumor-suppressor gene traditionally (21), but another study showed that overexpression of PIK3R1 accelerates HCC progression in cell lines (22). The protein encoded by ATP2A3 gene is Ca2+-ATPase3 from the sarco/endoplasmic reticulum (SERCA3) which plays a very important role in maintaining intracellular Ca2+ homeostasis (23).
In this study, we downloaded the data of ribonucleic acid sequencing (RNA-seq) and clinical information of HCC patients from public databases. The Cox regression analysis and least absolute shrinkage and selection operator (LASSO) regression analysis were performed to construct ERS-related gene signature. The cases were divided into high- and low-risk groups based on the ERS-related gene signature in The Cancer Genome Atlas (TCGA) cohort. Following this, we examined the differences between high- and low-risk groups in terms of messenger ribonucleic acid (mRNA) expression patterns, immune status, tumor mutation burdens (TMB) and copy number variants (CNV). Finally, we established a predictive nomogram according to the ERS-related gene signature and clinicopathological variables. We present this article in accordance with the TRIPOD reporting checklist (available at https://tcr.amegroups.com/article/view/10.21037/tcr-24-191/rc).
Methods
The study was conducted in accordance with the Declaration of Helsinki (as revised in 2013).
The collection and preprocessing of the data
The ribonucleic acid (RNA) sequencing data of liver hepatocellular carcinoma (TCGA-LIHC) were obtained from the UCSC Xena database (http://xena.ucsc.edu/), and the clinical data were obtained from the cBioPortal FOR CANCER GENOMICS database (https://www.cbioportal.org/). The cases with 0 days survival time were deleted, and finally 362 cases were included in subsequent analysis. The validation cohort data of another 232 HCC cases were extracted from the International Cancer Genomics Consortium (ICGC) database (https://dcc.icgc.org/projects/LIRI-JP). The ERS-related gene set (GO BP RESPONSE TO ENDOPLASMIC RETICULUM STRESS) which contains 295 genes was retrieved from Molecular Signature Database (MSigDB, http://www.gsea-msigdb.org/gsea/msigdb/index.jsp). Among them, 291 genes were found in TCGA data sets and are supplied in Table S1.
Establishment of a prognostic ERS-related gene signature
The “survival” R package was applied to identify ERS-related genes in connection with overall survival by Univariate Cox analysis in the TCGA cohort. The genes with a criterion that P<0.05 in Univariate Cox analysis were adopted to perform LASSO regression analysis with the “glmnet” R package. The model parameter λ was identified by tenfold cross-validation with the minimum criteria. Multivariate Cox analysis was used to establish the ERS-related prognostic model by R package “survival”. Subsequently, the RiskScore of each HCC patient was calculated according to the mRNA expression level of involved genes and their coefficients. The formula of RiskScore was established as follows:
The cases of HCC from TCGA and International Cancer Genome Consortium (ICGC) databases were classified into high- and low-risk groups based on the median RiskScore.
Evaluation of the prognostic gene signature
The Kaplan-Meier (K-M) survival curves were performed to compare overall survival (OS) between high- and low-risk groups using the “survival” R package in the TCGA and ICGC cohort. The “timeROC” R package was applied to plot receiver operating characteristic (ROC) curves to assess the accuracy of the gene signature. Boxplots were drawn to explore the relationship between the RiskScore and clinicopathologic features.
The confirmation of differentially expressed genes (DEGs) between high- and low-risk group patients and functional enrichment analysis
The “limma” R package was applied to screen DEGs with the criteria Padj<0.05 and |log2FC| >1. The “clusterProfiler” R package was used to perform Gene Ontology (GO) and Kyoto Encyclopedia of Genes and Genomes (KEGG) pathway analysis with DEGs.
Estimation of immune status
ESTIMATE algorithm was applied to explore the difference in immune microenvironmental and stromal status between high- and low-risk groups with the R package “estimate”. The difference of immune infiltration level between high- and low-risk groups was estimated by the CIBERSORT algorithm and microenvironment cell population (MCP)-counter algorithm. The relationship between the ERS-related RiskScore and each immune cell and 13 immune-related pathways were investigated by single sample gene set enrichment analysis (ssGSEA).
Gene set enrichment analysis (GSEA)
To explore the differences between pathways in the TCGA database between high- and low-risk groups, we performed GSEA analysis using GSEA 4.1.0 software. H.all. V2023.2. Hs. Symbols was selected as reference gene set. A false discovery rate (FDR) <0.25 and P<0.05 was considered significantly enriched.
TMB and CNV analysis
The maf format file of TMB was downloaded by the “maftools” R package. Masked copy number segment of CNV was obtained from TCGA GDC portal (https://portal.gdc.cancer.gov/), and was analyzed by GISTIC2 with default parameters for arm-level and focal CNVs.
Establishment and assessment of the predictive nomogram
The connection between clinicopathological parameters and RiskScore as well as overall survival was estimated by Univariate Cox regression. The R package “survival” was applied to perform Multivariate Cox regression of parameters with P<0.05. The predictive nomogram for overall survival was constructed by independent prognostic parameters (P<0.05) confirmed by multivariate Cox regression. Time-dependent ROC curves and calibration plots were applied to evaluate the precision of the nomogram with the “survivalROC” and “rms” R packages.
Cell culture and reverse transcription-quantitative polymerase chain reaction (RT-qPCR)
Huh7 and HepG2 cells lines were obtained from the Cell Bank of the Chinese Academy of Sciences and were cultured in Dulbecco’s Modified Eagle Medium (DMEM) with 10% fetal bovine serum (FBS). The small interfering RNAs (siRNAs) of TMX1 and BRSK2 were purchased from GenePharma corporation (Shanghai, China). The Lipofectamine RNAiMax was used to transfect the siRNAs into the cells. The siRNAs sequences were showed below: siTMX1-1: 5'-GGACGAGAACUGGAGAGAATT-3' (sense) and 5'-UUCUCUCCAGUUCUCGUCCTT-3' (antisense); siTMX1-2: 5'-GGGAGAAGAUCU UGAGGUUTT-3' (sense) and 5'-AACCUCAAGAUCUUCUCCCTT-3' (antisense). siBRSK2-1: 5'-CAUCCGCAUCGCAGACUUUTT-3' (sense) and 5'-AAAGUCUGCGAUGCGGAUGTT-3' (antisense); siBRSK2-2: 5'-CUUCGACGAUGACAACUUGTT-3' (sense) and 5'-CAAGUUGUCAUCGUCGAAGTT-3' (antisense); the control siRNA: 5'-UUCUCCGAACGUGUCACGUTT30-3' (sense) and 5'-ACGUGACACGUUCGGAGAATT-3' (antisense). In the RT-qPCR assay, 2 µg RNA were used to generate complementary DNA (cDNA). The primer sequences of TMX1 are displayed below: forward primer AGTCCTGGTGCTGTTGCTTT; reverse primer 5'-CTCTCCAGTTCTCGTCCGTG-3'. The primer sequences of glyceraldehyde-3'-phosphate dehydrogenase (GAPDH) are shown below: forward primer 5'-GACAGTCAGCCGCATCTTCT-3'; reverse primer 5'-GCGCCCAATACGACCAAATC-3'. BRSK2: 5'-GCTGGCGAAGAAGTCCTGGT-3' (forward) and 5'-CGTGGCCTTGTACTCGGC-3' (reverse).
Cell counting kit-8 (CCK8) and 5-ethynyl-2'-deoxyuridine (EDU) assays
For CCK8 assay, 2×103 cells were seeded into 96-well plates. CCK-8 was purchased from MedChemExpress corporation (HY-K0301). At the right time, the CCK8 reagent was put into wells according to instructions. After 1 h, the optical density (OD) values were detected at 450 nm. For EDU assay, 3×105 cells were put into per well of 96-well plates. EDU reagent was obtained from RiboBio company (Guangzhou C10310-1). After receiving treatment, the cells were cultured with EDU reagent for 2 h, then the assay was performed according to EDU kit instructions.
Wound healing and transwell assays
Before the assays, Huh7 and HepG2 cells were treated with Mitomycin C to inhibit proliferation. For Wound healing assay, 3×105 cells were put into per well of 6-well plates, then the siRNA of TMX1 were transfected into cells. After 24 h, the cells were scratched, and the images of 0, 12, 24 h were collected. For Transwell assay, 2×105 cells were resuspended with DMEM without FBS and were put into upper chamber. Then the DMEM with 10% FBS was put into lower chamber. After 24 h, the cells were stained with 1% crystal violet solution and collected images. Three replications were performed for each assay.
Terminal deoxynucleotidyl transferase dUTP nick end labeling (TUNEL) assay
Huh7 and HepG2 cells were prepared into round coverslips. The cells were treated with 1 μg/mL tunicamycin which is ERS inducer. Then cells were incubated with TUNEL reagent for 2 hours. After washed with phosphate balanced solution (PBS), the coverslips were exposed to 4,6-diamino-2-phenylindole (DAPI) for 20 min to facilitate nuclear staining. Subsequently, fluorescent microscope was used to take pictures. The TUNEL-positive cells were considered as apoptotic cells, and were quantified by the TUNEL-positive rate (TUNEL-positive cells/DAPI).
Statistical analysis
SPSS software (Version 26), R software (Version 4.1.2) and Xiantaoxueshu (https://www.xiantao.love) were used for all statistical analysis. Student’s t-test or Chi-squared test was applied to compare the differences between different variables, with the criteria of P<0.05.
Results
Identification of ERS-related genes and construction of prognostic ERS-related gene signature
ERS-related gene set (GO BP RESPONSE TO ENDOPLASMIC RETICULUM STRESS) was screened from MSigDB database. Finally, 291 genes (Box S1) were identified in TCGA-LIHC RNA-seq. A prognostic signature was built using these ERS-related genes.
Univariate Cox regression analysis was applied to explore correlations between ERS-related genes and overall survival of 362 HCC patients in the TCGA database. It showed that 67 genes (Table S1) were significantly associated with the overall survival of HCC patients (P<0.05). Subsequently, the ERS-related genes that had a better predictive performance were selected by LASSO regression analysis (Figure 1A,1B). The results showed that 16 genes (Table S1) were better associated with the overall survival of HCC patients (P<0.05). Finally, Multivariate Cox analysis was applied to further narrow down the range of prognostic ERS-related genes and construct the prognostic model. The result of multivariate Cox analysis was shown in Figure 1C. The RiskScore of each HCC patient involved in the study was calculated as follows:
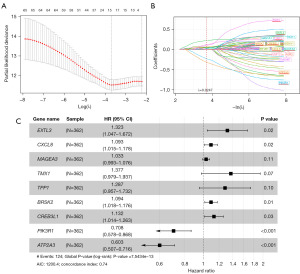
The validation of the 9 genes signature in the train set
Based on the median cut-off value, the HCC patients were divided into high- (n=181) and low-risk groups (n=181) in TCGA cohort (Figure 2A). The mortality of HCC patients increased with the RiskScore increasing (Figure 2B). A comparison of the expression levels of the genes in the prognostic model between the two groups can be seen in Figure 2C. The K-M curves depicted that the cases in high-risk group had obviously reduced overall survival than those in low-risk group (Figure 2D, P<0.001). In order to evaluate the precision of the 9 gene signature, the time-dependent ROC analysis was performed. As shown in Figure 2E, area under the curves (AUCs) were 0.784 at 1 year, 0.780 at 2 years, and 0.793 at 3 years.
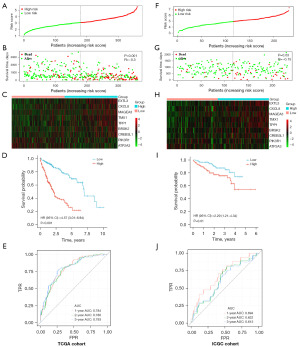
The confirmation of the 9 genes signature in the validation set
Similarly, RiskScore were calculated in the ICGC cohort of HCC patients, and the cases were categorized into high- (n=116) and low-risk (n=116) groups based on the median RiskScore (Figure 2F). Patients in the high-risk group had a higher chance of dying earlier (Figure 2G). The expression differences of 9 genes are shown in Figure 2H. As shown in K-M curves, the cases of the high-risk group had a shorter survival time (Figure 2I). Furthermore, the AUCs of the 9 genes signature was 0.694 at 1 year, 0.622 at 2 years, and 0.613 at 3 years (Figure 2J).
The relationship between RiskScore and clinicopathological factors
In the TCGA cohort, the high RiskScore was significantly related to higher tumor stage (T stage), higher clinical stage, higher degree of vascular invasion and higher neoplasm histologic grade (Figure 3A-3D).
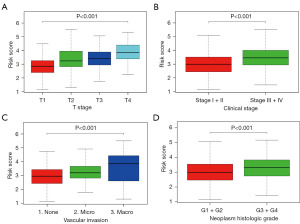
DEGs between different patient groups and their functional enrichment analysis
In the TCGA cohort, 717 genes were confirmed as DEGs between high- and low-risk groups patients with the criteria of Padj<0.05 and |log2FC| >1 (Figure 4A). The functions of those DEGs were explored by GO and KEGG enrichment analysis. These DEGs were mostly enriched in mitotic nuclear division, immunoglobulin complex, and regulation of mitotic sister chromatid segregation among the biological process (BP), cellular component (CC), and molecular function (MF) categories, respectively (Padj<0.05, Figure 4B-4D). The category of metabolism of cytochrome P450 cell cycle was mostly enriched in KEGG analysis (Padj<0.05, Figure 4E). The study have shown that ERS is closely related to the metabolism of various substances (24). This is consistent with KEGG items such as tyrosine metabolism, glycolysis and gluconeogenesis, etc. (Figure 4E). In addition, ER stress has been shown to influence cancer cell proliferation (25). Our KEGG items cell cycle (Figure 4E), BP items mitotic nuclear division (Figure 4B) and most of MF items (Figure 4D) is related to proliferation closely.
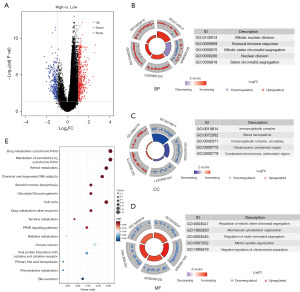
Comparison of immune status between high- and low-risk groups
ESTIMATE algorithm was applied to explore microenvironmental immune and stromal status between different groups in the TCGA cohort. The tumor purity was higher while stromal score, immune score, estimate score were lower in the high-risk group (Padj<0.001, Figure 5A). The infiltration status of immune cell subtypes was estimated by the CIBERSORT algorithm. As shown in violin plot Figure 5B, the high-risk group had obviously higher proportion of T cells follicular helper (P=0.04), T cells regulatory (P=0.001), NK cells activated (P=0.006), macrophages M0 (P<0.001), eosinophils (P=0.04), neutrophils (P=0.002). In contrast, the high-risk group had lower B cells naïve (P=0.001), plasma cells (P=0.049), T cells CD8 (P<0.001), T cells CD4 memory resting (P=0.02), NK cells resting (P=0.01), monocytes (P=0.02). Besides, antigen-presenting cells (APC) co-inhibition (P=0.002), CC chemokine receptor (CCR) (P<0.001), check-point (P=0.001), cytolytic activity (P<0.001), human leukocyte antigen (HLA) (P<0.001), inflammation-promoting (P<0.001), parainflammation (P=0.03), T cell co-inhibition (P<0.001), T cell co-stimulation (P<0.001), type II interferon (IFN) response (P<0.001) were obviously lower in the high-risk group (Figure 5C). In MCP-counter analysis, the high-risk group had a lower proportion of T cells (P<0.001), CD8 T cells (P<0.001), cytotoxic lymphocytes (P<0.001), B lineage (P<0.001), NK cells (P<0.001), myeloid dendritic cells (P=0.049), neutrophils (P=0.002), endothelial cells (P<0.001), fibroblasts (P=0.001) (Figure 5D). As shown in the results of ssGSEA analysis, the RiskScore was associated negatively with effector memory CD8 T cell, natural killer cell, T follicular helper cell, type I T helper cell, gamma delta T cell, immature B cell, activated B cell, macrophage (Figure 5E-5L). According to these results, immune infiltration of anti-tumor cells such as CD8+ cells was reduced in the high-risk group, contributing to a poor survival rate in these patients.
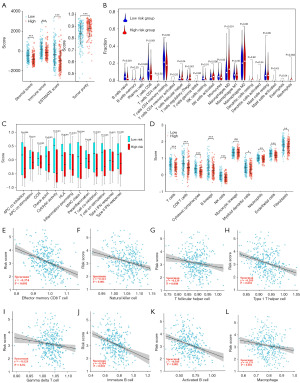
The results of GSEA
Based on the TCGA cohort, we performed GSEA. The results showed that UPR, glycolysis, DNA repair, and protein secretion were enriched in the high-risk group (Figure S1A-S1D), and UPR is closely related to ERS.
The status of mutations and copy number variation between different groups
Based on the TCGA cohort, waterfall plots were used to display the mutation patterns in HCC patients. In the high-risk group, the top ten mutation genes were TP53, TTN, CTNNB1, MUC16, PCLO, OBSCN, ALB, APOB, CSMD3, LRP1B (Figure 6A). While, the top ten mutation genes were TTN, CTNNB1, TP53, MUC16, ALB, PCLO, MUC4, ABCA13, FLG, RYR2 in low-risk group (Figure 6B). According to the results, the mutation rate of TP53 was 38% in the high-risk group, while in the low-risk group, it was 19%. Moreover, mutations of MUC16 were also more prevalent in the high-risk group than in the low-risk group. Additionally, the status of somatic copy number variation in different groups was also examined using GISTIC 2.0. Compared to the low-risk group, some oncogenic driver genes such as MYC (8q24.21), MET (7q31.2), MTDH (8q22.1) and RPS6KB1 (17q23.1) were amplified (Figure 6C), whereas tumor suppressor genes such as WRN (8p21.3), CDKN2A (9p21.3), and BRCA2 (13q13.1) were deleted (Figure 6D) in the high-risk group. In the low-risk group, frequently amplified genomic regions included 1q21.3, 5p15.33, 6p21.1 and 11q13.3, while deleted regions contained 1p36.23, 9p21.3 and 13q13.2 (Figure 6C,6D). Briefly, the frequency of genome mutations was higher in the high-risk group than in the low-risk group, suggesting that mutations may be involved in HCC tumorigenesis.
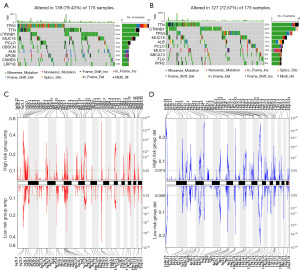
Establishment of a predictive nomogram according to RiskScore
Univariate Cox regression analysis was conducted to examine the relationship between overall survival, RiskScore, and clinicopathological variables in the TCGA cohort (Figure 7A). The hazard ratio of RiskScore was 2.718 [95% confidence interval (CI): 2.173–3.399, P<0.001]. We then performed a multivariate Cox regression analysis on the variables that reached statistical significance (Figure 7B). The hazard ratio of RiskScore was 2.422 (95% CI: 1.805–3.25, P<0.001). The results indicated that RiskScore and Eastern Cooperative Oncology Group (ECOG) performance status were independent prognostic factors. Next, a predictive nomogram according to RiskScore and ECOG performance status was constructed (Figure 7C). As the results illustrated, RiskScore was of great importance in this scoring system. According to the calibration plots, the predictive survival rate and actual survival rate in this nomogram are strongly consistent (Figure 7D-7F). The results of ROC curves showed that the AUCs were 0.851 at 1 year, 0.860 at 2 years, and 0.866 at 3 years (Figure 7G). In conclusion, a novel nomogram based on RiskScore has been developed that can assist clinicians in assessing the prognosis of HCC patients.
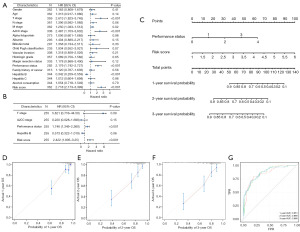
The biological functions of TMX1 and BRSK2 in HCC
In our model, TMX1 had the biggest coefficient suggesting that TMX1 plays an important role in HCC. Then we explored the biological functions of TMX1 in HCC. Our results showed that the expression level of TMX1 were significantly knocked down by siRNAs (Figure 8A). CCK8 and EDU assays showed that the proliferation of Huh7 and HepG2 cells were inhibited after TMX1 knockdown (Figure 8B-8D). Wound healing and transwell assays showed that the migration ability of Huh7 and HepG2 cells were attenuated after TMX1 knockdown (Figure 8E-8G). Our results showed that TMX1 knockdown can inhibit the proliferation and migration of Huh7 and HepG2 cells. On the other hand, we explored the effect of BRSK2 on ERS induced apoptosis. The expression level of BRSK2 were significantly knocked down by siRNAs (Figure S2A,S2B). The results showed that BRSK2 knockdown could promote the apoptosis induced by ERS of Huh7 and HepG2 cells (Figure S3A,S3B).
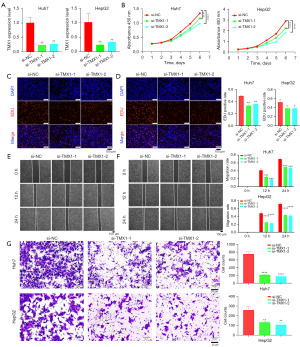
Discussion
Globally, primary liver cancer remains one of the most common types of cancer in humans. In spite of the wide variety of treatment strategies available for primary liver cancer, the patient’s prognosis is dismal, with a 5-year survival rate in America of only 18.1% (26). HCC accounts for the largest proportion of primary liver cancer, of which the overall survival is significantly different around the world. On the other hand, it’s difficult to estimate the prognosis of HCC exactly. As the previous study shown that the ERS has been demonstrated to be a crucial component of the biogenesis and development of HCC (7), but the relationship between ERS and the overall survival of HCC is not clear. Hence it is urgent to explore the relationship between ERS and HCC. Our study aimed to establish a new gene signature according to ERS and to provide assistance for the treatment and diagnosis of HCC. In this study, we established a new 9-genes signature related to ERS. The expression level of EXTL3, CXCL8, MAGEA3, TMX1, TPP1, BRSK2, CREB3L1 were negative while PIK3R1, ATP2A3 were positive with the prognosis of HCC patients. The products of EXTL3 gene are glycosyltransferases which play an important role in the biosynthesis of heparan sulfate (11). A recent study found that PI3K-AKT signaling regulates keratinocyte differentiation via RAG3A-EXTL3 interaction (27). In another study, Zhang et al. found that REG3A/REG3B binding to EXTL3 can promote acinar to ductal metaplasia and stimulate the RAS-RAF-MEK-ERK signaling pathway (28). In the tumor microenvironment, CXCL8 is an essential proinflammatory chemokine (12). It was reported that ERS can promote the expression of CXCL8 in breast carcinomas (13). In other studies, CXCL8 can promote HCC metastasis and progression (29,30) which is consistent with our study. However, the relationship between ERS, CXCL8 and HCC needs to be further explored in the future. MAGEA3 is one of the members of cancer-testis antigen. The aberrant expression of MAGEA3 has been identified in various cancers (31). In a recent study, MAGEA3 has been verified as a driver of tumor progression in HCC (32), and some long non-coding RNAs can influence proliferation, chemoresistance and the progression of HCC via MAGEA3 (33). In the above studies, MAGEA3 can promote cancer progression which is consistent with our study. The product of TMX1 is the disulfide isomerase (PDI) which plays a very important role in ERS (34). Raturi et al. found that TMX1 can regulate the transport of Ca2+ and cancer cell metabolism (35). The transcription product of TPP1 is a lysosomal protease and can cleave substrate N-terminal tripeptides. The high expression level of TPP1 has been found to be associated with a poor prognosis for patients with HCC (36) which is consistent with our study. BRSK2 is a serine/threonine kinase, Wang et al. found that ERS can down-regulate the protein level of BRSK2 and reduce the apoptosis induced by ERS (18). CREB3L1 is a cellular transcription factor in response to ERS (37). Furthermore, CREB3L1 is rarely studied in HCC, but one study found that CREB3L1 is associated with the prognosis of gliomas (38). PIK3R1 encodes p85α subunit of PI3K enzymes and PIK3R1 is considered as a tumor-suppressor gene traditionally (21), but another study showed that overexpression of PIK3R1 accelerates HCC progression in cell lines (22). Furthermore, PIK3R1 can promote the transport of XBP1 isoform 2 to the nuclear, which regulates ER responses and improves liver glucose tolerance (39). In our study, PIK3R1 is a protective factor, which is in conflict with some current studies, and more research is needed in the future to explore the reasons. The protein encoded by ATP2A3 gene is SERCA3 which plays a very important role in maintaining intracellular Ca2+ homeostasis (23). The previous study showed that the overexpression of ATP2A3 can induce apoptosis, suppress cell cycle progression and trigger ERS (40). In HCC cases, the expression of ATP2A3 is downregulated and is related to the poor prognosis of HCC patients (41). In the current study, ATP2A3 was a protective factor, which is consistent with our findings. To conclude, among the 9 genes included in our gene signature, EXTL3, TMX1, BRSK2, and CREB3L1 are related to the overall survival of HCC patients. However, research on them is rare and more effort needs to be taken to investigate the function of those genes in HCC. The roles of residual genes in the process of ERS in HCC need to be identified in the future.
In our study, the RiskScore of each HCC patient was calculated and the immune status was explored in different groups with different methods in the TCGA cohort. The high-risk group had a lower immune infiltration state, and a higher tumor purity. As our results shown, the high-risk group had a higher infiltration of M2 macrophages, regulatory T cells (Tregs) and a lower infiltration of CD8+ T cells. In the results of MCP counter, the infiltration of NK cells was lower in the high-risk group. Besides, most of the immune response are reduced in high-risk group. The major functions of M2 macrophages are anti-inflammatory and immunosuppressive effects (42). It is believed that these cells can suppress the immune system and promote tumor cell proliferation and metastasis (43). Tregs are highly immune suppressive cells. They can suppress effective antitumor immunity, which can contribute to the poor prognosis of various types of human cancers (44). It’s reported that CD8+ T cells can interact with other immune cells, and it is of great importance in the anti-tumor process (45). Clinical studies have illustrated that the high frequency of CD8+ T cells is favorable for cancer-free survival in patients with different cancers (46-48). In humans, NK cells play a very important role in tumor immunosurveillance and reduced NK cells function has been linked with the worse outcomes in cancer patients (49). According to our study, one reason for poor prognosis in the high-risk group is the immunosuppressive microenvironment, these subgroup patients might get more benefit from immunotherapy by targeting CD8+ T cells or NK cells.
Furthermore, we examined the mutation status of the different groups. The results suggested that the mutation rate of TP53, MUC16 was higher in the high-risk group. TP53 gene is a widely studied tumor suppressor gene and mutation of TP53 is common in human malignancies (50). The TP53 protein plays a critical role in the cellular response to different stresses and can maintain genomic integrity (51). MUC16 is also known as CA125, which has been confirmed as a biomarker in ovarian cancer. In addition, it has been demonstrated that it is overexpressed in human cancers. The overexpression of MUC16 can promote disease progression and metastasis in some cancers, thus it is an ideal target for diagnosis and therapy (52). In the analysis of somatic copy number variation, we observed that amplified genomic peaks of oncogenic drivers were discovered in the high-risk group, including MYC, MET, MTDH and RPS6KB1. Besides, some deletion peaks were also observed for tumor suppressor genes such as WRN, CDKN2A, and BRCA2. Those genes are critical for the process of the HCC (53), the amplification of oncogenes and deletion of suppressor genes may lead to poor prognosis in high-risk group. More importantly, the alternations and heterogeneity of genomics have an impact on tumor microenvironment transformation, progression, and treatment resistance (54), thus those mutation can also serve as the therapeutic targets for high-risk group.
In the following analysis, we identified RiskScore and ECOG performance status as independent predictors and constructed a nomogram based on them. ECOG performance status is a widely applied comprehensive scale of symptoms and mobility, which has existed for a long time (55). Compared to other models, our nomogram exhibits robust accuracy and practicality (56,57). Besides, the model can provide a practical tool for clinics to assess the prognosis of HCC patients.
At last, we selected TMX1 which had biggest coefficient to validate its biological functions. The role of TMX1 in HCC is not clear in present study, and our results showed that the ability of proliferation and migration of Huh7 and HepG2 cells were inhibited by TMX1 knockdown. On the other hand, BRSK2 could promote ERS-induced apoptosis of Huh7 and HepG2 cells. Our study showed that TMX1 and BRSK2 act as oncogene in HCC, and the mechanism needs to be explored in further.
There are some shortcomings in this study. First, our study was retrospective and the data were obtained from a public database, a large number of clinical cases are required to confirm the effectiveness of the model. Moreover, the immune states and somatic mutations related to the gene signature need to be explored by basic experiment. Finally, the construction of nomogram was based on the RNA-seq technology, the high cost may limit the clinical application of the model. It is worth noting that our article has been published in advance as a preprint paper (58).
Conclusions
In a word, this study established a 9 genes signature related to ERS, and identified it as an independent prognostic factor for HCC. Furthermore, we constructed a nomogram according to the gene signature and clinical parameters to predict the prognosis of HCC patients. At last, we explored the biological functions of TMX1 and BRSK2 in HCC. Our study may provide a new perspective for HCC.
Acknowledgments
A preprint has previously been published (58). The RNA sequencing data of liver hepatocellular carcinoma (TCGA-LIHC) were obtained from UCSC Xena database (http://xena.ucsc.edu/), and the clinical data were obtained from cBioPortal FOR CANCER GENOMICS database (https://www.cbioportal.org/). The data of the validation cohort were extracted from the International Cancer Genomics Consortium database (https://dcc.icgc.org/projects/LIRI-JP). The ERS-related gene set (GO BP RESPONSE TO ENDOPLASMIC RETICULUM STRESS) which contains 295 genes was retrieved from Molecular Signature Database (MSigDB, http://www.gsea-msigdb.org/gsea/msigdb/index.jsp).
Funding: This work was supported by
Footnote
Reporting Checklist: The authors have completed the TRIPOD reporting checklist. Available at https://tcr.amegroups.com/article/view/10.21037/tcr-24-191/rc
Data Sharing Statement: Available at https://tcr.amegroups.com/article/view/10.21037/tcr-24-191/dss
Peer Review File: Available at https://tcr.amegroups.com/article/view/10.21037/tcr-24-191/prf
Conflicts of Interest: All authors have completed the ICMJE uniform disclosure form (available at https://tcr.amegroups.com/article/view/10.21037/tcr-24-191/coif). The authors have no conflicts of interest to declare.
Ethical Statement: The authors are accountable for all aspects of the work in ensuring that questions related to the accuracy or integrity of any part of the work are appropriately investigated and resolved. The study was conducted in accordance with the Declaration of Helsinki (as revised in 2013).
Open Access Statement: This is an Open Access article distributed in accordance with the Creative Commons Attribution-NonCommercial-NoDerivs 4.0 International License (CC BY-NC-ND 4.0), which permits the non-commercial replication and distribution of the article with the strict proviso that no changes or edits are made and the original work is properly cited (including links to both the formal publication through the relevant DOI and the license). See: https://creativecommons.org/licenses/by-nc-nd/4.0/.
References
- Sung H, Ferlay J, Siegel RL, et al. Global Cancer Statistics 2020: GLOBOCAN Estimates of Incidence and Mortality Worldwide for 36 Cancers in 185 Countries. CA Cancer J Clin 2021;71:209-49. [Crossref] [PubMed]
- Craig AJ, von Felden J, Garcia-Lezana T, et al. Tumour evolution in hepatocellular carcinoma. Nat Rev Gastroenterol Hepatol 2020;17:139-52. [Crossref] [PubMed]
- Yang JD, Hainaut P, Gores GJ, et al. A global view of hepatocellular carcinoma: trends, risk, prevention and management. Nat Rev Gastroenterol Hepatol 2019;16:589-604. [Crossref] [PubMed]
- Schwarz DS, Blower MD. The endoplasmic reticulum: structure, function and response to cellular signaling. Cell Mol Life Sci 2016;73:79-94. [Crossref] [PubMed]
- Schubert U, Antón LC, Gibbs J, et al. Rapid degradation of a large fraction of newly synthesized proteins by proteasomes. Nature 2000;404:770-4. [Crossref] [PubMed]
- Oakes SA. Endoplasmic Reticulum Stress Signaling in Cancer Cells. Am J Pathol 2020;190:934-46. [Crossref] [PubMed]
- Wu J, Qiao S, Xiang Y, et al. Endoplasmic reticulum stress: Multiple regulatory roles in hepatocellular carcinoma. Biomed Pharmacother 2021;142:112005. [Crossref] [PubMed]
- Liu Y, Tao S, Liao L, et al. TRIM25 promotes the cell survival and growth of hepatocellular carcinoma through targeting Keap1-Nrf2 pathway. Nat Commun 2020;11:348. [Crossref] [PubMed]
- Xia W, Zhuang J, Wang G, et al. P4HB promotes HCC tumorigenesis through downregulation of GRP78 and subsequent upregulation of epithelial-to-mesenchymal transition. Oncotarget 2017;8:8512-21. [Crossref] [PubMed]
- Liu D, Fan Y, Li J, et al. Inhibition of cFLIP overcomes acquired resistance to sorafenib via reducing ER stress‑related autophagy in hepatocellular carcinoma. Oncol Rep 2018;40:2206-14. [Crossref] [PubMed]
- Yamada S. Specific functions of Exostosin-like 3 (EXTL3) gene products. Cell Mol Biol Lett 2020;25:39. [Crossref] [PubMed]
- Waugh DJ, Wilson C. The interleukin-8 pathway in cancer. Clin Cancer Res 2008;14:6735-41. [Crossref] [PubMed]
- Marjon PL, Bobrovnikova-Marjon EV, Abcouwer SF. Expression of the pro-angiogenic factors vascular endothelial growth factor and interleukin-8/CXCL8 by human breast carcinomas is responsive to nutrient deprivation and endoplasmic reticulum stress. Mol Cancer 2004;3:4. [Crossref] [PubMed]
- Chen A, Santana AL, Doudican N, et al. MAGE-A3 is a prognostic biomarker for poor clinical outcome in cutaneous squamous cell carcinoma with perineural invasion via modulation of cell proliferation. PLoS One 2020;15:e0241551. [Crossref] [PubMed]
- Khalvandi A, Abolhasani M, Madjd Z, et al. Nuclear overexpression levels of MAGE-A3 predict poor prognosis in patients with prostate cancer. APMIS 2021;129:291-303. [Crossref] [PubMed]
- Gaspar RS, Gibbins JM. Thiol Isomerases Orchestrate Thrombosis and Hemostasis. Antioxid Redox Signal 2021;35:1116-33. [Crossref] [PubMed]
- Wang QL, Gong C, Meng XY, et al. TPP1 is associated with risk of advanced precursors and cervical cancer survival. PLoS One 2024;19:e0298118. [Crossref] [PubMed]
- Wang Y, Wan B, Li D, et al. BRSK2 is regulated by ER stress in protein level and involved in ER stress-induced apoptosis. Biochem Biophys Res Commun 2012;423:813-8. [Crossref] [PubMed]
- Xu R, Wang K, Yao Z, et al. BRSK2 in pancreatic β cells promotes hyperinsulinemia-coupled insulin resistance and its genetic variants are associated with human type 2 diabetes. J Mol Cell Biol 2023;15:mjad033. [Crossref] [PubMed]
- Saito A, Omura I, Imaizumi K. CREB3L1/OASIS: cell cycle regulator and tumor suppressor. FEBS J 2024; Epub ahead of print. [Crossref] [PubMed]
- Vallejo-Díaz J, Chagoyen M, Olazabal-Morán M, et al. The Opposing Roles of PIK3R1/p85α and PIK3R2/p85β in Cancer. Trends Cancer 2019;5:233-44. [Crossref] [PubMed]
- Ai X, Xiang L, Huang Z, et al. Overexpression of PIK3R1 promotes hepatocellular carcinoma progression. Biol Res 2018;51:52. [Crossref] [PubMed]
- Papp B, Launay S, Gélébart P, et al. Endoplasmic Reticulum Calcium Pumps and Tumor Cell Differentiation. Int J Mol Sci 2020;21:3351. [Crossref] [PubMed]
- Sak F, Sengul F, Vatansev H. The Role of Endoplasmic Reticulum Stress in Metabolic Diseases. Metab Syndr Relat Disord 2024; Epub ahead of print. [Crossref] [PubMed]
- Wang H, Mi K. Emerging roles of endoplasmic reticulum stress in the cellular plasticity of cancer cells. Front Oncol 2023;13:1110881. [Crossref] [PubMed]
- Jemal A, Ward EM, Johnson CJ, et al. Annual Report to the Nation on the Status of Cancer, 1975-2014, Featuring Survival. J Natl Cancer Inst 2017;109:djx030. [Crossref] [PubMed]
- Lai Y, Li D, Li C, et al. The antimicrobial protein REG3A regulates keratinocyte proliferation and differentiation after skin injury. Immunity 2012;37:74-84. [Crossref] [PubMed]
- Zhang H, Corredor ALG, Messina-Pacheco J, et al. REG3A/REG3B promotes acinar to ductal metaplasia through binding to EXTL3 and activating the RAS-RAF-MEK-ERK signaling pathway. Commun Biol 2021;4:688. [Crossref] [PubMed]
- Li XP, Yang XY, Biskup E, et al. Co-expression of CXCL8 and HIF-1α is associated with metastasis and poor prognosis in hepatocellular carcinoma. Oncotarget 2015;6:22880-9. [Crossref] [PubMed]
- Sun F, Wang J, Sun Q, et al. Interleukin-8 promotes integrin β3 upregulation and cell invasion through PI3K/Akt pathway in hepatocellular carcinoma. J Exp Clin Cancer Res 2019;38:449. [Crossref] [PubMed]
- Esfandiary A, Ghafouri-Fard S. MAGE-A3: an immunogenic target used in clinical practice. Immunotherapy 2015;7:683-704. [Crossref] [PubMed]
- Craig AJ, Garcia-Lezana T, Ruiz de Galarreta M, et al. Transcriptomic characterization of cancer-testis antigens identifies MAGEA3 as a driver of tumor progression in hepatocellular carcinoma. PLoS Genet 2021;17:e1009589. [Crossref] [PubMed]
- Zhang X, Xu S, Hu C, et al. LncRNA ST8SIA6-AS1 promotes hepatocellular carcinoma progression by regulating MAGEA3 and DCAF4L2 expression. Biochem Biophys Res Commun 2020;533:1039-47. [Crossref] [PubMed]
- Guerra C, Molinari M. Thioredoxin-Related Transmembrane Proteins: TMX1 and Little Brothers TMX2, TMX3, TMX4 and TMX5. Cells 2020;9:2000. [Crossref] [PubMed]
- Raturi A, Gutiérrez T, Ortiz-Sandoval C, et al. TMX1 determines cancer cell metabolism as a thiol-based modulator of ER-mitochondria Ca2+ flux. J Cell Biol 2016;214:433-44. [Crossref] [PubMed]
- Ottermann U, Mravak S, Kremsner PG, et al. Chronic recurrent fever as the sole symptom of Yersinia enterocolitica infection. Dtsch Med Wochenschr 1989;114:335-6. [Crossref] [PubMed]
- Denard B, Seemann J, Chen Q, et al. The membrane-bound transcription factor CREB3L1 is activated in response to virus infection to inhibit proliferation of virus-infected cells. Cell Host Microbe 2011;10:65-74. [Crossref] [PubMed]
- Liu LQ, Feng LF, Nan CR, et al. CREB3L1 and PTN expressions correlate with prognosis of brain glioma patients. Biosci Rep 2018;38:BSR20170100. [Crossref] [PubMed]
- Winnay JN, Boucher J, Mori MA, et al. A regulatory subunit of phosphoinositide 3-kinase increases the nuclear accumulation of X-box-binding protein-1 to modulate the unfolded protein response. Nat Med 2010;16:438-45. [Crossref] [PubMed]
- Zhang Y, Li F, Liu L, et al. Salinomycin triggers endoplasmic reticulum stress through ATP2A3 upregulation in PC-3 cells. BMC Cancer 2019;19:381. [Crossref] [PubMed]
- Hernández-Oliveras A, Izquierdo-Torres E, Meneses-Morales I, et al. Histone deacetylase inhibitors promote ATP2A3 gene expression in hepatocellular carcinoma cells: p300 as a transcriptional regulator. Int J Biochem Cell Biol 2019;113:8-16. [Crossref] [PubMed]
- Allavena P, Sica A, Solinas G, et al. The inflammatory micro-environment in tumor progression: the role of tumor-associated macrophages. Crit Rev Oncol Hematol 2008;66:1-9. [Crossref] [PubMed]
- Boutilier AJ, Elsawa SF. Macrophage Polarization States in the Tumor Microenvironment. Int J Mol Sci 2021;22:6995. [Crossref] [PubMed]
- Takeuchi Y, Nishikawa H. Roles of regulatory T cells in cancer immunity. Int Immunol 2016;28:401-9. [Crossref] [PubMed]
- Reiser J, Banerjee A. Effector, Memory, and Dysfunctional CD8(+) T Cell Fates in the Antitumor Immune Response. J Immunol Res 2016;2016:8941260. [Crossref] [PubMed]
- Mahmoud SM, Paish EC, Powe DG, et al. Tumor-infiltrating CD8+ lymphocytes predict clinical outcome in breast cancer. J Clin Oncol 2011;29:1949-55. [Crossref] [PubMed]
- Djenidi F, Adam J, Goubar A, et al. CD8+CD103+ tumor-infiltrating lymphocytes are tumor-specific tissue-resident memory T cells and a prognostic factor for survival in lung cancer patients. J Immunol 2015;194:3475-86. [Crossref] [PubMed]
- Kmiecik J, Poli A, Brons NH, et al. Elevated CD3+ and CD8+ tumor-infiltrating immune cells correlate with prolonged survival in glioblastoma patients despite integrated immunosuppressive mechanisms in the tumor microenvironment and at the systemic level. J Neuroimmunol 2013;264:71-83. [Crossref] [PubMed]
- Shimasaki N, Jain A, Campana D. NK cells for cancer immunotherapy. Nat Rev Drug Discov 2020;19:200-18. [Crossref] [PubMed]
- Olivier M, Hollstein M, Hainaut P. TP53 mutations in human cancers: origins, consequences, and clinical use. Cold Spring Harb Perspect Biol 2010;2:a001008. [Crossref] [PubMed]
- Aubrey BJ, Strasser A, Kelly GL. Tumor-Suppressor Functions of the TP53 Pathway. Cold Spring Harb Perspect Med 2016;6:a026062. [Crossref] [PubMed]
- Aithal A, Rauth S, Kshirsagar P, et al. MUC16 as a novel target for cancer therapy. Expert Opin Ther Targets 2018;22:675-86. [Crossref] [PubMed]
- Shibata T, Aburatani H. Exploration of liver cancer genomes. Nat Rev Gastroenterol Hepatol 2014;11:340-9. [Crossref] [PubMed]
- Robinson DR, Wu YM, Lonigro RJ, et al. Integrative clinical genomics of metastatic cancer. Nature 2017;548:297-303. [Crossref] [PubMed]
- Simcock R, Wright J. Beyond Performance Status. Clin Oncol (R Coll Radiol) 2020;32:553-61. [Crossref] [PubMed]
- Liu P, Wei J, Mao F, et al. Establishment of a Prognostic Model for Hepatocellular Carcinoma Based on Endoplasmic Reticulum Stress-Related Gene Analysis. Front Oncol 2021;11:641487. [Crossref] [PubMed]
- Liang JY, Wang DS, Lin HC, et al. A Novel Ferroptosis-related Gene Signature for Overall Survival Prediction in Patients with Hepatocellular Carcinoma. Int J Biol Sci 2020;16:2430-41. [Crossref] [PubMed]
- Du X, He Y, Dong P, et al. A novel gene signature based on endoplasmic reticulum stress for overall survival prediction for patients with hepatocellular carcinoma, 13 July 2022, PREPRINT (Version 1). Doi:
10.21203/rs.3.rs-1826210/v1 .10.21203/rs.3.rs-1826210/v1