RNA sequencing reveals transcriptomic changes in PC-12 cells following plasminogen activator, tissue type overexpression
Highlight box
Key findings
• This study conducted transcriptome sequencing analysis on PC-12 cells overexpressed with plasminogen activator, tissue type (PLAT) and the control group. Differentially expressed genes were identified and found to be significantly enriched in functional pathways related to mitochondrial respiration chain and energy metabolism.
What is known and what is new?
• PLAT is a protein-coding gene that encodes tissue-type plasminogen activator, which converts plasminogen to plasmin. The expression level of the PLAT protein is significantly elevated in pheochromocytoma and paraganglioma with low blood supply.
• The RNA sequencing of PC-12 cells in the PLAT overexpression group and the control group revealed a total of 2,231 differentially expressed genes. Differentially expressed genes were significantly enriched in pathways related to mitochondrial respiratory chain and energy metabolism.
What is the implication, and what should change now?
• This work provides some insights into the role of PLAT in pheochromocytoma and suggests directions for further research on its involvement in tumor development and angiogenesis.
Introduction
Pheochromocytoma is a tumor originating from the chromaffin tissue of the adrenal gland. It is considered a neuroendocrine tumor with malignant potential, along with paraganglioma from the extra-adrenal paraganglia, collectively known as pheochromocytoma and paraganglioma (PPGL) (1-3). Its incidence is not high, with approximately eight cases per million people (1). PPGL can present with a variety of clinical manifestations, and some patients may be asymptomatic. It can also cause symptoms such as secondary hypertension, palpitations, excessive sweating, and headaches by secreting large amounts of catecholamines into the blood, leading to complications in vital organs such as the heart, brain, and kidneys (1,2). In severe cases, it can even be life-threatening. The reported prevalence of PPGL among patients in hypertension clinics is 0.2–0.6% (4).
Plasminogen activator, tissue type (PLAT), also known as tissue plasminogen activator (TPA), is a protein-coding gene that encodes tissue-type plasminogen activator, which converts plasminogen into plasmin (5-7). It plays an important role in tissue remodeling and degradation, cell migration, and many other physiological and pathological events. There is limited research on the association between PLAT and PPGL. Previous studies have only found that the expression level of PLAT protein is significantly increased in PPGL with deficient blood supply (8), and its role in the occurrence and progression of PPGL remains unclear and needs further clarification.
PC-12 cells, first isolated by Greene and Tischler in 1976, are a clonal cell line derived from rat pheochromocytoma of the adrenal medulla (9). They possess high stability and uniformity, can be indefinitely passaged, and are commonly used as an in vitro model for pheochromocytoma and neurologically-related diseases (10). In order to comprehensively investigate the molecular mechanisms of PLAT regulation in pheochromocytoma, this study selected the PC-12 cell line and constructed control and PLAT overexpression cell models using lentiviral transfection techniques. Transcriptomic information of PLAT regulation in PC-12 cells was obtained through RNA sequencing, and differentially expressed genes (DEGs) were screened using bioinformatics methods. The physiological functions and related signaling pathways of these genes were analyzed, aiming to provide new clues for elucidating the role of PLAT in pheochromocytoma. We present this article in accordance with the MDAR reporting checklist (available at https://tcr.amegroups.com/article/view/10.21037/tcr-24-326/rc).
Methods
Cell culture
The PC-12 cells (RRID:CVCL_0481) of rat pheochromocytoma were obtained from the Shanghai Institute of Endocrine and Metabolic Diseases. They were cultured in RPMI 1640 medium containing 10% fetal bovine serum and 1% penicillin-streptomycin under the conditions of 37 ℃ and 5% CO2 in a humidified incubator.
Lentiviral transfection
The construction and packaging of lentiviral vectors were completed by Shanghai Genechem Co., Ltd. (Shanghai, China). For experimental purposes, the negative control (NC) group and overexpression (PLAT-OE) group were established to construct empty vector (virus ID, LVCON335; virus titer: 2.5×108 TU/mL; virus infection volume: 32 µL) and PLAT overexpression lentiviral vectors (virus ID, LVKL70595-1; virus titer: 1.0×109 TU/mL; virus infection volume: 8 µL), respectively. PC-12 cells in the logarithmic growth phase were infected with the viral solution to generate a cell suspension of 3×104 cells/mL, which was then seeded into culture plates for infection at a multiplicity of infection (MOI) of 40. According to the user manual, the complete culture medium was replaced after 12 hours, and the cells were further cultured for 72 hours before they could be used for subsequent experiments.
RNA extraction and quantitative real-time polymerase chain reaction (qPCR)
Total RNA was extracted from PC-12 cells using TRIzol reagent (Invitrogen, Carlsbad, USA). The concentration and purity of the extracted RNA samples were measured using a Nanodrop 2000C spectrophotometer (Thermo Scientific, Waltham, USA). Then, the total RNA was reverse transcribed into cDNA using a reverse transcription kit (Promega, Beijing, China) according to the manufacturer’s instructions. qPCR reactions were performed using SYBR® Green Premix Pro Taq HS kit (Accurate Biology, Changsha, China). The reaction conditions were as follows: pre-denaturation at 95 ℃ for 30 s, polymerase chain reaction (PCR) amplification at 95 ℃ for 5 s and 60 ℃ for 30 s, for a total of 40 cycles. The primers used were as follows: ACTB forward (5'-CGTTGACATCCGTAAAGAC-3'), ACTB reverse (5'-CTGGAAGGTGGACAGTGAG-3'); PLAT forward (5'-GCCCTGACGGATTTGTTG-3'), PLAT reverse (5'-CTCGGTCTGGGTTTCTGC-3'). The experiment was conducted with three replicates.
Western blot
Cells were collected and lysed with cell lysis buffer to extract total cellular proteins. The protein concentration was determined using the bicinchoninic acid (BCA) assay. After sodium dodecyl sulfate-polyacrylamide gel electrophoresis (SDS-PAGE), the proteins were transferred to a polyvinylidene fluoride (PVDF) membrane. The membrane was then blocked with 5% skim milk at room temperature for 2 hours. It was subsequently incubated overnight at 4 ℃ with primary antibodies, including PLAT antibody (Cat. No. ab157469, Abcam, Cambridge, UK) and β-actin antibody (Cat. No. TDY051, TDY Biotech, Beijing, China). After washing with phosphate-buffered saline with tween (PBST), the membrane was incubated with horseradish peroxidase (HRP)-conjugated secondary antibody (Cat. No. AS1107, Aspen Biotech, Wuhan, China) at room temperature for 1 hour. After another round of PBST washing, chemiluminescent substrate (Cat. No. AS1059, Aspen Biotech) was added, and the membrane was exposed and developed in a darkroom.
RNA sequencing
Figure 1A illustrates the flowchart for RNA sequencing, including library construction and sequencing experiments. The integrity, concentration, and purity of the RNA were examined. After passing quality control, the mRNA with poly A tail was enriched using Oligo (dT) magnetic beads, and the enriched mRNA was randomly interrupted using New England Biolabs (NEB, Ipswich, USA) fragmentation buffer. The first strand of cDNA was synthesized using fragmented mRNA as a template and random oligonucleotides as primers. Subsequently, RNase H, dNTPs, DNA polymerase I, and buffer were added to synthesize the second strand of cDNA. The purified double-stranded cDNA was subjected to end repair, A-tailed addition, and ligation of sequencing adapters. cDNA fragments of approximately 250–300 bp were selected, amplified by PCR, and then purified again to obtain the final library. After quality testing of the constructed library, sequencing was performed on the Illumina high-throughput sequencing platform NovaSeq 6000 (San Diego, USA).
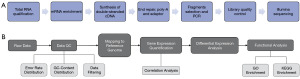
Sequencing data processing
The workflow in Figure 1B describes the data processing process. The image data obtained from high-throughput sequencers were converted into sequence data (reads) using CASAVA base recognition. The raw data underwent several filtering steps, including the removal of adapter sequences, elimination of reads containing N (undetermined bases), and discarding low-quality reads. This yielded clean reads for subsequent analysis. The clean reads were then aligned to the reference genome sequence using HISAT2. Based on the positional information of genes on the reference genome, the number of reads covering each gene within the start-to-stop range was calculated. FeatureCounts was used to compute the read counts mapped to each gene. Subsequently, the fragments per kilobase million (FPKM) value for each gene was calculated based on its length, allowing for the determination of gene expression levels. Correlation coefficients between samples within and between groups were calculated using the FPKM values of all genes for each sample. These coefficients were visualized as heatmaps, providing an intuitive display of inter-group differences and intra-group sample replicates.
Differential gene analysis
After gene expression quantification, the raw read counts were first normalized. Statistical models were then used to calculate the probability of hypothesis testing. Finally, multiple hypothesis testing corrections were performed to obtain the false discovery rate (FDR) values. DESeq2 is utilized for differential expression analysis between two comparison groups. The obtained P values were adjusted using the Benjamini and Hochberg method to control the false discovery rate. The adjusted P values, along with the |log2foldchange (FC)|, serve as thresholds for significant differential expression. Based on the differential analysis results, a volcano plot is generated to visualize the distribution of DEGs. Calculate the correlation coefficients of intra group and inter group samples based on the FPKM values of all genes in each sample, and draw a heat map to visually display the differences and duplication of intra group samples. Hierarchical clustering is performed on the FPKM values of genes, and row normalization is applied using Z-score. A sample distance heatmap is generated to visualize the results.
Enrichment analysis
Gene Ontology (GO) is a comprehensive database that describes gene functions. It primarily categorizes genes based on their inherent functions, providing constraints and descriptions of gene and protein functionality. Kyoto Encyclopedia of Genes and Genomes (KEGG) is a database that integrates genomic, chemical, and systemic functional information. It aims to provide a molecular-level understanding of advanced biological system functions and utilities. The differential gene set was subjected to GO functional enrichment analysis and KEGG pathway enrichment analysis using ClusterProfiler. For gene functional enrichment analysis, gene sets were first constructed by classifying genome annotations, and then the differential gene set was mapped to the background gene set. Both GO functional enrichment and KEGG pathway enrichment used a Padj cutoff of less than 0.05 to determine significant enrichment.
Cell Counting Kit-8 (CCK-8) assay
The NC and PLAT-OE cells in the logarithmic growth phase were digested with 0.25% trypsin and resuspended in complete culture medium. Cell counting was performed using a cell counter, and 100 µL with 2,000 cells per well were seeded into a 96-well plate. The cell density of each experimental group was observed under a microscope to ensure uniformity, and adjustments were made accordingly based on the observation results. Subsequently, the cells were placed in a 37 ℃, 5% CO2 incubator. A total of five sets of 96-well plates were prepared, with three replicates for each sample. On days 1, 2, 3, 4, and 5 of cultivation, the plates were taken out and 10 µL of CCK-8 reagent (DOJINDO, Kumamoto, Japan) was added, followed by further incubation for 2 hours. After thorough shaking, the optical density (OD) value was read at a wavelength of 450 nm using a microplate reader (Tecan Infinite, Männedorf, Switzerland). A cell growth curve was plotted.
Statistical analysis
qPCR utilized the 2−△△Ct relative quantification method to calculate the expression of PLAT mRNA relative to actin beta (ACTB) mRNA. A t-test was employed to analyze and compare the differences between two groups. In cell proliferation assays, the OD values obtained from the spectrophotometer were converted. OD450 (fold) represented the fold change in OD450 values relative to day 1 for each experimental group, indicating the proliferation rate for each day. A t-test was used to compare the differences between the two groups. Prior to comparison, a test for homogeneity of variances (F-test) was conducted on the data of each group. If the F-test value is greater than 0.05, the P value is obtained by the two sample test with equal variance. If the F-test value is less than 0.05, the P value is obtained by the two sample test with heteroscedasticity. The statistical analysis process involved in RNA sequencing has been mentioned earlier. All experiments were conducted with three biological replicates each, followed by analysis. Microsoft Excel 2019, R-4.0.4, and GraphPad Prism 8 software were used for data analysis and graphing. P<0.05 indicated a statistically significant difference between the two groups (*), while P<0.01 denoted a highly significant difference (**). P>0.05 indicated no statistically significant difference between the two groups.
Results
Cell model construction
The mRNA and protein expression levels of PLAT in PC-12 cells were examined using qPCR and Western blot. The results showed a significant increase in both mRNA level (Figure 2A) and protein level (Figure 2B) in PLAT-OE group compared to NC group. The transfection efficiency was confirmed to be as expected, indicating successful cell model construction. The cell proliferation assay results (Figure 2C) demonstrated that on the 5th day of culture, the number of viable cells in the PLAT-OE group was significantly higher than that in the NC group (P<0.01).
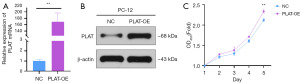
Sequencing data quality control
Filtering, sequencing error rate check, and guanine and cytidine (GC) content distribution check were performed on the raw data to obtain clean reads for subsequent analysis. The summarized data are presented in Table 1. The range of clean reads for each group was between 5.84–6.80 G. Analysis of base quality and composition revealed a GC content range of 47.34–50.17%. The Q20 value for all samples was not less than 96.74%, and the Q30 value was not less than 91.70%. This indicates that the sequencing data obtained has high quality and can be used for subsequent bioinformatics analysis.
Table 1
Sample | Raw reads | Raw bases (G bp) | Clean reads | Clean bases (G bp) | Error rate (%) | Q20 (%) | Q30 (%) | GC PCT (%) |
---|---|---|---|---|---|---|---|---|
NC 1 | 46039226 | 6.91 | 45155442 | 6.77 | 0.03 | 97.38 | 92.97 | 48.25 |
NC 2 | 42675070 | 6.40 | 42168466 | 6.33 | 0.03 | 97.17 | 92.48 | 47.34 |
NC 3 | 48023926 | 7.20 | 45356692 | 6.80 | 0.03 | 97.28 | 92.77 | 50.17 |
OE 1 | 45323052 | 6.80 | 43214324 | 6.48 | 0.03 | 97.74 | 93.75 | 49.82 |
OE 2 | 40550758 | 6.08 | 39150552 | 5.87 | 0.03 | 97.03 | 92.29 | 49.47 |
OE 3 | 40242748 | 6.04 | 38917662 | 5.84 | 0.03 | 96.74 | 91.70 | 49.62 |
Clean reads: the number of reads filtered by raw reads; clean bases (clean reads ×150 bp): the number of bases filtered by raw bases (raw reads ×150 bp); error rate: the overall error rate of sequencing data; Q20 and Q30: the possibility of one error per 100 or 1,000 bp, respectively, with corresponding accuracy of 99% and 99.9%. A higher score means a lower probability of error; GC PCT: the percentage of G and C in the four bases in clean reads. RNA, ribonucleic acid; NC, negative control; OE, overexpression.
Differential gene analysis
We drew a sample correlation heat map (Figure 3A). The gene expression profiles of two groups were presented using a Venn diagram (Figure 3B), with 13,284 genes expressed in the NC group and 13,112 genes expressed in the PLAT-OE group. A total of 12,783 genes were expressed in both groups. The DEGs were clustered and presented as a heatmap (Figure 3C). Using the criteria |log2FC| >0 and P value <0.05, a total of 2,231 DEGs were identified. Among them, compared to the NC group, the PLAT-OE group had 1,022 upregulated genes and 1,209 downregulated genes. A volcano plot was generated to visualize the significant DEGs (Figure 3D). Table 2 provides a list of selected DEGs in the PLAT-OE group, including upregulated and downregulated genes, distinguished by the positive and negative values of log2FC.
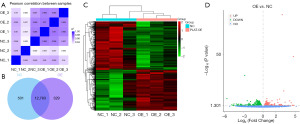
Table 2
Gene ID | Gene name | Gene description | Log2FC | P value | Padj |
---|---|---|---|---|---|
ENSRNOG00000021201 | Txnip | Thioredoxin interacting protein | 0.5880 | 9.72E−09 | 2.91E−05 |
ENSRNOG00000027191 | Birc6 | Baculoviral IAP repeat-containing 6 | −0.8687 | 5.71E−07 | 8.55E−04 |
ENSRNOG00000003515 | Ephx1 | Epoxide hydrolase 1 | 0.6061 | 8.13E−07 | 1.04E−03 |
ENSRNOG00000005505 | Wbp11l1 | WW domain-binding protein 11-like 1 | 1.3901 | 1.00E−06 | 1.13E−03 |
ENSRNOG00000058866 | Myo5a | Myosin VA | −0.7631 | 1.32E−06 | 1.31E−03 |
ENSRNOG00000011066 | March6 | Membrane associated ring-CH-type finger 6 | −0.5627 | 1.49E−06 | 1.34E−03 |
ENSRNOG00000006789 | Ddit3 | DNA-damage inducible transcript 3 | 0.4854 | 2.78E−06 | 2.27E−03 |
ENSRNOG00000000593 | Rev3l | REV3 like, DNA directed polymerase zeta catalytic subunit | −0.6535 | 3.86E−06 | 2.47E−03 |
ENSRNOG00000020451 | Cd81 | Cd81 molecule | 0.4432 | 4.12E−06 | 2.47E−03 |
ENSRNOG00000015488 | Tead1 | TEA domain transcription factor 1 | −0.7206 | 5.54E−06 | 2.82E−03 |
DEG, differentially expressed gene; PLAT, plasminogen activator, tissue type; OE, overexpression; ID, identity document; FC, fold change; Padj, adjusted P values.
GO functional enrichment analysis
GO functional enrichment analysis of DEGs revealed 46 significantly enriched GO functions (Padj<0.05, the same below), of which 23 were enriched in biological processes (BPs) and 23 were enriched in cell components (CCs). We have listed the five most significant GO items separately. In the category of BPs, DEGs are mainly enriched in mitochondrial electron transport, nicotinamide adenine dinucleotide (NADH) to ubiquinone; positive regulation of cellular component biogenesis; adenosine triphosphate (ATP) synthesis coupled electric transport; histone modification and peptidyl-lysine modification. In the category of CCs, DEGs are mainly enriched in the mitochondrial protein complex; inner mitochondrial membrane protein complex; mitochondrial memory part; mitochondrial respiratory chain and respiratory chain complex. Table 3 shows more detailed information on these GO entries. Appendix 1 lists the specific genes enriched by these GO entries.
Table 3
Category | GO ID | Description | Gene ratio | Padj |
---|---|---|---|---|
BP | GO:0006120 | Mitochondrial electron transport, NADH to ubiquinone | 12/1,782 | 2.81E−02 |
BP | GO:0044089 | Positive regulation of cellular component biogenesis | 82/1,782 | 2.81E−02 |
BP | GO:0042773 | ATP synthesis coupled electron transport | 22/1,782 | 2.81E−02 |
BP | GO:0016570 | Histone modification | 83/1,782 | 2.81E−02 |
BP | GO:0018205 | Peptidyl-lysine modification | 69/1,782 | 2.81E−02 |
CC | GO:0098798 | Mitochondrial protein complex | 68/1,807 | 1.58E−05 |
CC | GO:0098800 | Inner mitochondrial membrane protein complex | 42/1,807 | 2.08E−05 |
CC | GO:0044455 | Mitochondrial membrane part | 55/1,807 | 4.05E−04 |
CC | GO:0005746 | Mitochondrial respiratory chain | 28/1,807 | 7.37E−04 |
CC | GO:0098803 | Respiratory chain complex | 27/1,807 | 1.07E−03 |
GO, Gene Ontology; ID, identity document; Padj, adjusted P values; BP, biological process; CC, cellular component; NADH, nicotinamide adenine dinucleotide; ATP, adenosine triphosphate.
KEGG pathway enrichment analysis
All DEGs were enriched into seven significantly enriched KEGG signaling pathways, as shown in Figure 4, which were mainly related to thermogenesis; oxidative phonology; Parkinson’s disease; Huntington disease; retrograde endocannabinoid signaling; lysine degradation and non-alcoholic fatty liver disease (NAFLD). Appendix 2 lists the specific genes enriched by these KEGG pathways.
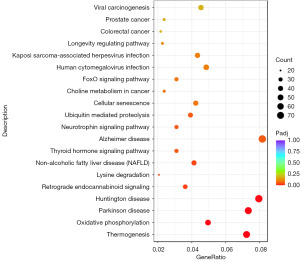
Discussion
GO functional enrichment analysis mainly focuses on three aspects. Firstly, in terms of mitochondrial electron transport and ATP synthesis. In the category of biological processes, DEGs significantly enrich in “mitochondrial electron transport, NADH to ubiquinone” and “ATP synthesis coupled electron transport” indicating the important roles these genes play in cellular energy metabolism. Mitochondria, as the “energy factory” of the cell, are closely associated with electron transport chains and ATP synthesis in cellular energy generation. The changes in these DEGs may reflect alterations in cellular energy metabolism. Secondly, in terms of cellular component construction and modification. Enrichment of GO terms such as “positive regulation of cellular component biogenesis”, “histone modification” and “peptidyl-lysine modification” suggests that these DEGs regulate processes involved in cellular component construction, chromatin structure, and protein modification. These processes are crucial for cell growth, differentiation, and functional execution. Lastly, in terms of mitochondrial-related components. In the cellular component category, DEGs mainly enrich in mitochondrial-related components such as “mitochondrial protein complex” and “inner mitochondrial membrane protein complex”. This further emphasizes the importance of mitochondria in the functionality of DEGs, possibly related to mitochondrial dysfunction or metabolic abnormalities.
The KEGG signaling pathway is related to energy metabolism and diseases. In terms of energy metabolism, DEGs are mainly involved in pathways such as “Thermogenesis”, “Oxidative phosphorylation” and “Lysine degradation”, indicating a potential association between DEG alterations and energy metabolism abnormalities. In terms of diseases, related pathways cover neurodegenerative diseases such as “Parkinson’s disease” and “Huntington’s disease” as well as metabolic diseases like “Non-alcoholic fatty liver disease (NAFLD)”. It’s worth mentioning NAFLD, as a common chronic liver disease, its pathogenesis is complex and involves changes in multiple metabolic pathways and gene expressions.
The production and release of PLAT in the body are strictly regulated, and as a key molecule in the fibrinolytic system, it plays an important role in regulating the tumor microenvironment and immune microenvironment. From the perspective of the fibrinolytic system, it can regulate the dissolution of blood fibrinogen, maintain normal fibrinolysis balance and vascular function, and is crucial for maintaining normal blood circulation (11,12). From the microenvironment perspective, it can maintain extracellular matrix homeostasis and regulate the post-translational activation of growth factors (11,13). Changes in fibrinolytic activity may affect the degradation of the extracellular matrix, thereby influencing the migration and distribution of immune cells. Additionally, TPA may also affect immune cell infiltration and tumor nutrition supply by regulating angiogenesis. Furthermore, it has been reported to be associated with neuroprotection, learning and memory systems, the progression of certain kidney diseases, and peritoneal adhesions (14-18).
The process by which PLAT regulates the fibrinolysis system in the blood can be summarized as follows: when conditions such as thrombosis or vascular injury occur, it can convert plasminogen (an inactive precursor) to plasmin. This is an enzyme that plays a crucial role in the process of blood clot dissolution. It specifically cleaves peptide bonds between arginine and lysine, recognizes and binds to fibrin, degrades fibrin into soluble products, and has a specific thrombolytic effect, inhibiting thrombus formation (19). Due to its unique role in anticoagulation, TPA is widely used in clinical practice to treat diseases such as myocardial infarction, stroke, and deep vein thrombosis (20). TPA can help restore normal blood flow to the affected area by dissolving the obstructed blood clots. In addition, the correlation between TPA and the microenvironment provides new ideas and directions for future drug development. One possible goal for future drug development is to optimize the immune microenvironment by regulating the activity of TPA, thereby enhancing the effectiveness of immunotherapy or reducing its side effects.
Angiogenesis refers to the process of new blood vessel formation, which occurs in various situations such as growth and development, wound healing, disease progression, and tumor growth. Angiogenesis is a complex process involving interactions between multiple cell types, including endothelial cell proliferation, migration, differentiation, extracellular matrix degradation, and tube formation (21). There is a close connection between TPA and angiogenesis. TPA can degrade the microvascular basement membrane and extracellular matrix by activating proteolytic cascades, thereby inhibiting microvessel formation (22). Tissue-type plasminogen activator contains five structural domains, two of which are kringles that show significant sequence identity with the domain of angiostatin, an angiogenesis inhibitor (17,21,23). Previous studies have shown that kringle domains exhibit anti-angiogenic activity after enzymatic cleavage or expression as a recombinant domain (21,23,24). The recombinant kringle domain of TPA has an inhibitory effect on endothelial cell proliferation. In vitro experiments have demonstrated that human and murine endothelial cell migration is suppressed by fragments containing kringle 2 of TPA (23). Reteplase is a variant of tissue-type plasminogen activator that contains kringle 2 and the enzyme binding site of human tissue-type plasminogen activator. This drug has a significant impact on endothelial cell proliferation, migration, and angiogenesis (23). The recombinant kringle domain of TPA is a selective inhibitor of endothelial cell growth and is considered a novel target for anti-angiogenesis therapy (21,23). Plasminogen activator inhibitor-1 (PAI-1) is the primary inhibitor of TPA (25). PAI-1 is a complex regulator of angiogenesis, and its absence may result in inhibited endothelial cell movement and weakened neovascularization of tumors and other types of cells. In PAI-1-deficient mice tumors, angiogenesis and the expression of vascular endothelial growth factor (VEGF) are reduced. The expression of VEGF is significantly decreased, leading to decreased tumor angiogenesis and neovascularization (26). These studies indicate that TPA plays an important regulatory role in the angiogenesis process and likely exhibits negative regulation of vascular neogenesis.
PPGLs are distinguished from other tumors of the urogenital system by their rich blood supply. Through multi-omics analysis of over 100 cases of PPGLs, researchers have found that this tumor is driven by a wide variety of driver mutations, primarily arising from a single mutation (somatic mutation, germline mutation, or fusion gene) (27). Our team’s investigation into the vascular formation of PPGLs has never ceased (8,28,29). In this study, we conducted transcriptome sequencing analysis on PLAT overexpressed PC-12 cells and control group cells, resulting in the identification of 2,231 DEGs. GO functional enrichment analysis and KEGG pathway enrichment revealed that these genes were significantly enriched in mitochondrial respiratory chain and energy metabolism functions or pathways. Mitochondria are important organelles that act as the “energy factory” of the cells (30). It is one of the main sites of reactive oxygen species (ROS) production in the body and participate in regulating cell apoptosis (30). The mitochondrial respiratory chain is located on the inner mitochondrial membrane and consists of five large protein complexes, including NADH: ubiquinone oxidoreductase (Complex I) (31). There is a connection between the mitochondrial respiratory chain and angiogenesis. The mitochondrial respiratory chain oxidizes organic molecules such as glucose, releasing energy and ultimately generating ATP. This process produces a series of intracellular signaling molecules, such as reactive nitrogen species (RNS) and ROS. ROS, which are small molecular metabolites generated by the normal cell mitochondrial respiratory chain or membrane-bound NADPH oxidase, play important roles in angiogenesis both in vivo and in vitro studies. ROS can transmit signals by activating the PI3K/AKT pathway and the hypoxia-inducible factor-1 (HIF-1) signaling molecule, promoting tumor neovascularization (32,33). Additionally, ROS can enhance endothelial cell proliferation, decomposition and migration, regulate factors involved in angiogenesis such as pAKT, NF-κB, MAPK, and matrix metalloproteinases (MMPS) (31). ROS also contribute to the regulation of vascular tone and vascular structure through the modulation of Ang II. The energy metabolism processes also influence tumor initiation and growth. When intracellular oxidative phosphorylation is inhibited, tumor cells can obtain energy by promoting glycolysis, resulting in the production of more lactic acid (34,35). Lactic acid is modified by monocarboxylate transporters and carbonic anhydrase to acidify the extracellular environment, further promoting cancer cell proliferation (36). PLAT primarily functions in the process of fibrinolysis, and its relationship with energy metabolism has not been fully elucidated. Although they are not directly connected, there may be indirect effects under certain pathological conditions. For example, abnormal PLAT activity leading to thrombus formation or embolism may affect vascular permeability and microcirculation, thereby influencing the supply of oxygen and nutrients to tissues and the exchange of nutrients, indirectly impacting the energy metabolism in that area. Future research can further explore this potential relationship and its mechanisms.
The strengths of this study lie in providing preliminary insights into the role of PLAT in pheochromocytoma and guiding further research. By using RNA sequencing and bioinformatics methods to identify DEGs associated with PLAT regulation, the study offers clues for unraveling the molecular mechanisms through which PLAT participates in regulating pheochromocytoma development and angiogenesis. Additionally, the study utilizes PC-12 cells overexpressing PLAT as an experimental model, which is a commonly used cell line aiding researchers in better understanding PLAT’s function in tumor cells. Moreover, transcriptome sequencing analysis, being a high-throughput technique, furnishes comprehensive gene expression data, thereby providing researchers with extensive research avenues. However, the study also has its limitations. Firstly, it relies solely on one cell line for experimentation, lacking validation on other tumor cell lines or pathological samples in vivo. This might constrain the generalizability of the findings, failing to fully reflect the actual in vivo biological context. Secondly, while transcriptome sequencing analysis yields abundant gene expression data, there remains some controversy regarding the certainty and reliability of the research findings, necessitating further validation through functional experiments. Additionally, the study does not delve into the specific mechanisms through which PLAT regulates pheochromocytoma development and angiogenesis but merely offers preliminary clues, thus requiring further research to validate and delve deeper into these findings. Overall, the study serves as a crucial reference for understanding the role of PLAT in pheochromocytoma, yet further experimentation and research are warranted to confirm and expand upon these results.
Regarding the mentioned limitations above of this study, future work will focus on several aspects. Firstly, expanding the range of experimental samples. We will introduce a wider range of tumor cell lines and conduct in vivo experiments through the design and implementation of animal model experiments for validation. Secondly, in-depth analysis and validation of transcriptome sequencing data will be conducted, including functional experiments to validate the roles of key genes and pathways. This will involve designing and implementing corresponding functional experiments such as gene knockout and overexpression to validate their roles in pheochromocytoma. Lastly, a thorough exploration of the specific mechanisms by which PLAT regulates the development of pheochromocytoma and angiogenesis will be conducted, including research on signaling pathways and interacting proteins. Based on the results of mechanism exploration, potential therapeutic targets will be identified to provide new avenues and methods for the treatment of pheochromocytoma.
Conclusions
This work represents an advance in biomedical science. It provides some insights into the role of PLAT in pheochromocytoma and suggests directions for further research on its involvement in tumor development and angiogenesis. However, the specific regulatory mechanisms still require further validation.
Acknowledgments
Funding: This work was supported by
Footnote
Reporting Checklist: The authors have completed the MDAR reporting checklist. Available at https://tcr.amegroups.com/article/view/10.21037/tcr-24-326/rc
Data Sharing Statement: Available at https://tcr.amegroups.com/article/view/10.21037/tcr-24-326/dss
Peer Review File: Available at https://tcr.amegroups.com/article/view/10.21037/tcr-24-326/prf
Conflicts of Interest: All authors have completed the ICMJE uniform disclosure form (available at https://tcr.amegroups.com/article/view/10.21037/tcr-24-326/coif). The authors have no conflicts of interest to declare.
Ethical Statement: The authors are accountable for all aspects of the work in ensuring that questions related to the accuracy or integrity of any part of the work are appropriately investigated and resolved.
Open Access Statement: This is an Open Access article distributed in accordance with the Creative Commons Attribution-NonCommercial-NoDerivs 4.0 International License (CC BY-NC-ND 4.0), which permits the non-commercial replication and distribution of the article with the strict proviso that no changes or edits are made and the original work is properly cited (including links to both the formal publication through the relevant DOI and the license). See: https://creativecommons.org/licenses/by-nc-nd/4.0/.
References
- Szatko A, Glinicki P, Gietka-Czernel M. Pheochromocytoma/paraganglioma-associated cardiomyopathy. Front Endocrinol (Lausanne) 2023;14:1204851. [Crossref] [PubMed]
- Lam AK. Update on Adrenal Tumours in 2017 World Health Organization (WHO) of Endocrine Tumours. Endocr Pathol 2017;28:213-27. [Crossref] [PubMed]
- Zhong X, Su T, Yang Y, et al. Platelet-Lymphocyte and Neutrophil-Lymphocyte Ratios Are Prognostic Markers for Pheochromocytomas and Paragangliomas. J Clin Endocrinol Metab 2023;108:2230-9. [Crossref] [PubMed]
- Jain A, Baracco R, Kapur G. Pheochromocytoma and paraganglioma-an update on diagnosis, evaluation, and management. Pediatr Nephrol 2020;35:581-94. [Crossref] [PubMed]
- Goeke CM, Zhang X, Hashimoto JG, et al. Astrocyte tissue plasminogen activator expression during brain development and its role in pyramidal neuron neurite outgrowth. Neurosci Lett 2022;769:136422. [Crossref] [PubMed]
- Ruikar K, Khode V, Shetty SS, et al. Association of pro-fibrinolytic receptor AnnexinA2 with tissue plasminogen activator/Inhibitor-1 in pre-eclampsia. Afr Health Sci 2023;23:309-19. [Crossref] [PubMed]
- Wang X, Xue D, Zhu X, et al. Low Expression of PLAT in Breast Cancer Infers Poor Prognosis and High Immune Infiltrating Level. Int J Gen Med 2021;14:10213-24. [Crossref] [PubMed]
- Sun F, Zhuo R, Ma W, et al. From clinic to mechanism: Proteomics-based assessment of angiogenesis in adrenal pheochromocytoma. J Cell Physiol 2019;234:22057-70. [Crossref] [PubMed]
- Greene LA, Tischler AS. Establishment of a noradrenergic clonal line of rat adrenal pheochromocytoma cells which respond to nerve growth factor. Proc Natl Acad Sci U S A 1976;73:2424-8. [Crossref] [PubMed]
- Ohnuma K, Hayashi Y, Furue M, et al. Serum-free culture conditions for serial subculture of undifferentiated PC12 cells. J Neurosci Methods 2006;151:250-61. [Crossref] [PubMed]
- Hu K, Yang J, Tanaka S, et al. Tissue-type plasminogen activator acts as a cytokine that triggers intracellular signal transduction and induces matrix metalloproteinase-9 gene expression. J Biol Chem 2006;281:2120-7. [Crossref] [PubMed]
- Lemarchant S, Pruvost M, Hébert M, et al. tPA promotes ADAMTS-4-induced CSPG degradation, thereby enhancing neuroplasticity following spinal cord injury. Neurobiol Dis 2014;66:28-42. [Crossref] [PubMed]
- Kruithof EK, Dunoyer-Geindre S. Human tissue-type plasminogen activator. Thromb Haemost 2014;112:243-54. [Crossref] [PubMed]
- Elmi S, Sahu G, Malavade K, et al. Role of tissue plasminogen activator and plasminogen activator inhibitor as potential biomarkers in psychosis. Asian J Psychiatr 2019;43:105-10. [Crossref] [PubMed]
- Hua Q, Cheng H, Yang YQ, et al. Role of tPA in Corticosterone-Induced Apoptosis of Mouse Mural Granulosa and Oviductal Epithelial Cells. Cells 2023;12:455. [Crossref] [PubMed]
- Obiang P, Macrez R, Jullienne A, et al. GluN2D subunit-containing NMDA receptors control tissue plasminogen activator-mediated spatial memory. J Neurosci 2012;32:12726-34. [Crossref] [PubMed]
- Seillier C, Hélie P, Petit G, et al. Roles of the tissue-type plasminogen activator in immune response. Cell Immunol 2022;371:104451. [Crossref] [PubMed]
- Yildiz MK, Okan I, Dursun N, et al. Effect of orally administered simvastatin on prevention of postoperative adhesion in rats. Int J Clin Exp Med 2014;7:405-10. [PubMed]
- Summaria L, Hsieh B, Robbins KC. The specific mechanism of activation of human plasminogen to plasmin. J Biol Chem 1967;242:4279-83. [Crossref] [PubMed]
- Dong Y, Yang L, Ren J, et al. Intravenous Tissue Plasminogen Activator Can Be Safely Given without Complete Blood Count Results Back. PLoS One 2015;10:e0131234. [Crossref] [PubMed]
- Kim HK, Lee SY, Oh HK, et al. Inhibition of endothelial cell proliferation by the recombinant kringle domain of tissue-type plasminogen activator. Biochem Biophys Res Commun 2003;304:740-6. [Crossref] [PubMed]
- Ismail AA, Shaker BT, Bajou K. The Plasminogen-Activator Plasmin System in Physiological and Pathophysiological Angiogenesis. Int J Mol Sci 2021;23:337. [Crossref] [PubMed]
- Carroll VA, Nikitenko LL, Bicknell R, et al. Antiangiogenic activity of a domain deletion mutant of tissue plasminogen activator containing kringle 2. Arterioscler Thromb Vasc Biol 2005;25:736-41. [Crossref] [PubMed]
- Cao Y, Cao R, Veitonmäki N. Kringle structures and antiangiogenesis. Curr Med Chem Anticancer Agents 2002;2:667-81. [Crossref] [PubMed]
- Jasmiad NB, Abd Ghani RB, Agarwal R, et al. Relationship between serum and tear levels of tissue plasminogen activator and plasminogen activator inhibitor-1 in diabetic retinopathy. BMC Ophthalmol 2022;22:357. [Crossref] [PubMed]
- Gutierrez LS, Schulman A, Brito-Robinson T, et al. Tumor development is retarded in mice lacking the gene for urokinase-type plasminogen activator or its inhibitor, plasminogen activator inhibitor-1. Cancer Res 2000;60:5839-47. [PubMed]
- Fishbein L, Leshchiner I, Walter V, et al. Comprehensive Molecular Characterization of Pheochromocytoma and Paraganglioma. Cancer Cell 2017;31:181-93. [Crossref] [PubMed]
- Mao Y, Ma W, Zhuo R, et al. COX4I2 is a novel biomarker of blood supply in adrenal tumors. Transl Androl Urol 2021;10:2899-909. [Crossref] [PubMed]
- Mao Y, Zhuo R, Ma W, et al. Fibroblasts mediate the angiogenesis of pheochromocytoma by increasing COX4I2 expression. Front Oncol 2022;12:938123. [Crossref] [PubMed]
- Yang M, Liu C, Jiang N, et al. Mitochondrial homeostasis: a potential target for delaying renal aging. Front Pharmacol 2023;14:1191517. [Crossref] [PubMed]
- Sharma LK, Lu J, Bai Y. Mitochondrial respiratory complex I: structure, function and implication in human diseases. Curr Med Chem 2009;16:1266-77. [Crossref] [PubMed]
- Groeger G, Quiney C, Cotter TG. Hydrogen peroxide as a cell-survival signaling molecule. Antioxid Redox Signal 2009;11:2655-71. [Crossref] [PubMed]
- Fruehauf JP, Meyskens FL Jr. Reactive oxygen species: a breath of life or death? Clin Cancer Res 2007;13:789-94. [Crossref] [PubMed]
- Warburg O. On the Origin of Cancer Cells. Science 1956;123:309-14. [Crossref] [PubMed]
- Gatenby RA, Gillies RJ. Why do cancers have high aerobic glycolysis? Nat Rev Cancer 2004;4:891-9. [Crossref] [PubMed]
- Sonveaux P, Copetti T, De Saedeleer CJ, et al. Targeting the lactate transporter MCT1 in endothelial cells inhibits lactate-induced HIF-1 activation and tumor angiogenesis. PLoS One 2012;7:e33418. [Crossref] [PubMed]