Heterogeneity of tumor microenvironment cell groups in inflammatory and adenomatous polyposis coli mutant colorectal cancer based on single cell sequencing
Highlight box
Key findings
• The study utilized single-cell RNA sequencing to analyze the tumor microenvironment (TME) of two types of colorectal cancer (CRC): inflammatory and adenomatous polyposis coli (APC) mutant CRC. Significant differences were observed in the composition and gene expression profiles of immune cells between two types of CRC, particularly in macrophages and T cells.
What is known and what is new?
• Macrophages play crucial roles in both inflammation and cancer, with plasticity and diverse functions in the TME. T cells are pivotal in anti-tumor responses, and their subsets exhibit functional differences that influence tumor progression and prognosis in CRC.
• The manuscript provides a detailed single-cell characterization of the TME in two types of CRC, highlighting distinct immune cell compositions and gene expression patterns. It identifies specific macrophage subgroups (Mγ) associated with worse prognosis in inflammatory CRC, linked to APOE and BNIP3 expression and shows that APC CRC has a higher proportion of T cells, particularly Tβ, associated with tumor suppression and better prognosis.
What is the implication, and what should change now?
• The findings suggest potential targets for therapeutic interventions aimed at modulating the TME in CRC. Further research is warranted to validate the prognostic significance of identified biomarkers like APOE and BNIP3 in clinical settings. Understanding the functional differences in immune cell subsets, particularly macrophage and T cells, could lead to targeted therapies that enhance anti-tumor immune responses in CRC.
Introduction
Colorectal cancer (CRC) is the third most common cancer worldwide, and it etiologically arises from malignant transformation within the gastrointestinal tract (1). However, the pathogenesis of CRC is complex, it hypothesizes contributors include dietary exposures, genetic predispositions, concomitant gastrointestinal diseases, chemical carcinogens, lifestyle factors, and infections. Advances in cancer screening and treatments have reduced overall CRC mortality in recent years (2), and current data also indicate that the subtype associated with inflammatory bowel disease (IBD) confers worse prognosis (3).
IBD-associated CRC (IBD-CRC), often associated with chronic inflammation in the colon, displays a tumor microenvironment (TME) enriched with immune cells such as tumor-associated macrophages (TAMs), dendritic cells, and T cells (4). Furthermore, the presence of cytokine-secreting immune cells and activated inflammatory pathways underscores the role of inflammation in driving tumor progression and therapy resistance in this subtype (5). In contrast, adenomatous polyposis coli (APC) mutant CRC, characterized by mutations in the APC gene, exhibits distinct features in its TME. One study has revealed alterations in key signaling pathways related to Wnt/β-catenin, leading to dysregulated cellular proliferation and differentiation within the tumor (6). Moreover, APC mutant CRC TMEs are characterized by an increased presence of cancer-associated fibroblasts and alterations in extracellular matrix composition, contributing to tumor growth and invasion (7). In addition, the immune microenvironment of the two subtypes of CRC typically exhibits different immune cell infiltration, indicating differences in the immune evasion mechanisms employed by these subtypes (8).
Single-cell RNA sequencing (scRNA-seq) has revealed the complex cellular composition of the TME at high resolution (9), and it enables unbiased, high-throughput, and high-resolution transcriptomic analysis of individual cells (10). To date, a consensus has coming into being that scRNA-seq is a powerful tool for elucidating biological mechanisms at the cellular level (11). The development of scRNA-seq technology provides some new perspectives for research on health and diseases. The differences in the TME caused by IBD-CRC and APC mutant CRC have not been described at the single-cell level, while scRNA-seq allows us to observe unique alterations in IBD-CRC at the cell population level and gain deeper insights into IBD-CRC pathogenesis. Thus, studying azoxymethane/dextran sodium sulfate (AOM/DSS) and APC-mutant CRC TME differences at a single-cell resolution may aid development of more effective therapeutic strategies.
In this study, we initiated our research with the Gene Expression Omnibus (GEO) database and selected the GSE5956400 (AOM/DSS) and GSE5956401 (ApcMin/+) datasets from the GSE198758 collection for analysis. The AOM/DSS dataset represents an inflammation-induced mouse model of CRC, while the ApcMin/+ dataset corresponds to a mouse model of CRC resulting from APC gene mutations. We analyzed the heterogeneity of the TME in these two types of tumors and further examined the differences in macrophages, T cells, and their subsets. Moreover, we validated normal and tumor tissues in human intestines using immunohistochemistry and immunofluorescence staining. Collectively, our data reveal heterogeneity between the two types of CRC, and we anticipate that these findings will inform future treatment strategies for CRC. We present this article in accordance with the MDAR reporting checklist (available at https://tcr.amegroups.com/article/view/10.21037/tcr-24-689/rc).
Methods
Patient information and data acquisition
This study was conducted in accordance with the Declaration of Helsinki (as revised in 2013). The single-cell 3'mRNA sequencing data for this study was obtained from GEO (available at https://www.ncbi.nlm.nih.gov/geo/) under accession number GSE198758, in which GSE5956400 (AOM/DSS) and GSE5956401 (ApcMin/+) datasets were included in this study for further comparison and analysis. The AOM/DSS group of mice in this dataset were treated with AOM (10 mg/kg) for 1 week and then treated with 2.5% DSS for 5 days. After that, they were treated with H2O for 2 weeks. This cycle was repeated for three times. Animals in the ApcMin/+ group were C57BL/6 mice with APC mutation (12). For validation of the screening results of this study, we collected tumor and adjacent normal tissues from 5 patients with CRC, the details of the participants are listed in Table S1. The subject population was all CRC patients seeking medical treatment at Tangshan Gongren Hospital Affiliated to Hebei Medical University and all the included patients have signed informed consent forms. This study was approved by the Institutional Review Board of the Tangshan Gongren Hospital Affiliated to Hebei Medical University (IRB-2023145).
scRNA sequencing data processing
The scRNA sequencing data was processed and analyzed using the R package Seurat (13). High quality cells were selected based on the following criteria: (I) number of genes detected per cell between 200 and 3,000; (II) percentage of mitochondrial gene expression <5% per cell, an indicator of cell apoptosis status. As a result, 5,714 cells in AOM and 2,780 cells in APC were included in the downstream analyses. Gene expression data was then normalized using the Seurat package and normalization method “LogNormalize” to reduce the magnitude of discrete gene expression counts. Highly variable genes (hvg) then generated using the Seurat “FindVariableFeatures” function, and the top 2,000 hvg were selected for principal component analysis. Batch effects between samples were removed using Harmony, and the first 8 significant principal components were used for t-distributed stochastic neighbor embedding (t-SNE) analysis. Clustering of cells was performed using the “FindClusters” function (resolution =0.2) and visualized by t-SNE. Subclustering analysis was performed using the “FindClusters” function (resolution =0.3) and visualized by t-SNE.
Identification of marker genes and differentially expressed genes (DEGs)
We used Seurat’s “FindAllMarkers” function to identify marker genes for each cluster. Marker genes identified by “FindAllMarkers” were required to have an average expression [average log2(fold change)] higher than other clusters by >0.25-fold and detectable expression in >25% of cells within that cluster. We used Seurat’s “FindMarkers” function and Wilcoxon rank sum test based on default parameters to compute DEGs between cell subgroups. Cutoff values were set as absolute average log2(fold change) value ≥0.25 and P value <0.05.
Cell annotation
A total of 7 cell types were annotated based on known markers (14,15). Two unknown cell types named UN1 and UN2, UN1 cells were annotated by Csf3r, UN2 cells by Retnlg, macrophages (M) by Ctss, T cells by Cd3g, enterocyte (En) by Epcam, B cells by Ccr7, and fibroblasts (Fb) by Col4a1.
Enrichment analysis and survival analysis
The R package ClusterProfiler was used to perform Gene Ontology (GO) analysis (16), with gene sets with P value <0.05 considered significantly enriched. Online Kyoto Encyclopedia of Genes and Genomes (KEGG) pathway analysis was performed using R software, with pathways of adjusted P value <0.05 considered significantly altered. Online survival analysis was performed using GEPIA2 database, with P value <0.05 considered statistically significant.
Hematoxylin-eosin staining
According to the manufacturer’s instructions, the entire colon was washed with phosphate-buffered saline (PBS) and fixed in 4% paraformaldehyde, and paraffin sections were made, followed by hematoxylin and eosin (HE) staining.
Immunohistochemistry and immunofluorescence staining
The general processing method for colorectal tissue slices is to dewaxing and antigen recovery the colorectal tissue in citrate buffer, and to block endogenous peroxidase by incubating it in 3% (v/v) H2O2 for 25 minutes. Subsequently, the colorectal tissue was incubated with 5% bovine serum albumin (BSA) for 30 minutes. Immunohistochemistry: after slice processing, the specific primary antibodies (APOE, 1:200, ZEN BIO, Chengdu, China, Cat No. R381129; BNIP3, 1:200, ZEN BIO, Cat No. R381756) were incubated overnight again. After rinsing with PBS three times, goat anti rabbit immunoglobulin G (IgG) antibodies conjugated with biotin were reacted at a dilution of 1:200 for 1 hour. An appropriate volume of diaminobenzidine (DAB) was applied to tissues for signal development, where the nucleus was stained blue with hematoxylin and representative images are collected using a LeiCa SP8 laser confocal microscope (Leica Microscope GmbH, Germany). Immunofluorescence staining: after slice processing, the specific primary antibodies (APOE, 1:200; BNIP3, 1:200; CD68, 1:200, ZEN BIO, Cat No.250019) were incubated overnight again. After rinsing with PBS three times, goat anti rabbit and mouse IgG fluorescent antibodies conjugated with biotin were reacted at a dilution of 1:200 for 1 hour. Then, the nucleus was stained with 4',6-diamidino-2-phenylindole (DAPI) and representative images were collected using the ImageXpress® Micro Confocal system (Molecular Devices, USA). Values were analyzed by Image J software (NIH, USA).
Statistical analysis
Unpaired two-tailed Wilcoxon rank sum test was used to compare cell distributions between two groups. Unpaired two-tailed Student’s t-test was employed to compare gene expression or features between groups. Pathway activity correlation was determined by Spearman correlation test. P value <0.05 was considered statistically significant.
Results
Single-cell landscape of AOM/DSS (AOM) and APC-mutant (APC) CRC tumors
To characterize the TME of CRC in different etiology, we collected 8,544 high-quality single cells from AOM/DSS (AOM) and APC-mutant (APC) CRC types for scRNA-seq (Figure 1A). Quality control metrics confirmed the reliability of these cells (Figure 1B). Through unsupervised clustering and t-SNE analysis, 8,544 cells from the AOM and APC groups were clustered into 7 clusters. We then named the 7 cell clusters (macrophages, T cells, B cells, fibroblasts, enterocyte, and 2 unknown cell types named UN1 and UN2) according to the specific markers (Figure 1C). We displayed the cell fraction of the 7 cell types in the AOM and APC groups (Figure 1D), with T cells accounting for 69%. The composition of each cell type in the AOM and APC groups is shown (Figure 1E,1F). Violin plots showing marker genes for each cell cluster are presented (Figure 1G). Our data indicate macrophages and T cells differ greatly between the AOM and APC groups. Marker genes for each cell population demonstrate the reliability of our cell clustering (Figure 1G). Next, we show the DEGs for the 7 cell types (Figure 1H). Our data uncovered the heterogeneity between AOM and APC TMEs and highlighted significant differences in tumor-infiltrating immune cells between AOM and APC groups.
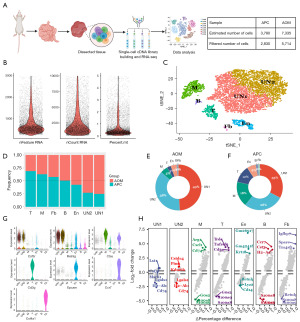
Macrophages exhibit enhanced pro-tumoral effects in IBD-CRC
Macrophages are highly plastic and multifunctional immune cells that mediate developmental, homeostatic, debris scavenging, pathogen clearance, and inflammatory-regulating effects (17). Both resident and recruited macrophages are present during acute inflammation and oncogenesis in almost all tissues (17-19). We therefore investigated differences between macrophages in the AOM and APC groups (Figure 2A). We observed a higher fraction of macrophages in the APC group (Figure 2B). We display volcano plots of macrophage DEGs between the AOM and APC groups (Figure 2C). We validate the reliability of our cell clustering by showing specific marker genes for macrophage subgroups (Figure 2D). To understand the biological functions of macrophage in more depth, we performed GO and KEGG pathway enrichment analysis on the DEGs (Figure 2E). GO enrichment analysis revealed DEGs were significantly enriched in cell-cell adhesion and cytokine activity. KEGG pathway analysis showed DEGs were enriched in pathways in various diseases, including rheumatoid arthritis, tuberculosis, and leishmaniasis. Aberrant cytokine regulation is an important mechanism in tumorigenesis and progression (20,21). Cell-cell adhesion is a key factor promoting cancer progression, facilitating cancer development including immune evasion and metastatic dissemination (22,23). In summary, macrophages in the AOM/DSS group likely exhibit enhanced pro-tumoral effects.
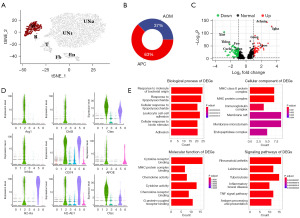
Worse prognosis of IBD-CRC is associated with APOE and BNIP3 expression
To elucidate macrophage differences, we performed subclustering analysis, identifying 3 subgroups named Mα, Mβ, and Mγ (Figure 3A). We show the cell fractions of the 3 cell types in the AOM and APC groups CRC types (Figure 3B) and their proportions in the AOM and APC groups (Figure 3C,3D). We found cluster Mγ differed specially between the AOM and APC groups. Specific marker genes for cluster Mγ are shown (Figure S1). GO and KEGG pathway enrichment analysis was performed on DEGs in cluster Mγ (Figure 3E). GO analysis revealed cluster Mγ is associated with leukocyte migration, chemokine activity, and cytokine activity. KEGG analysis showed cluster Mγ is not only related to pathways in various diseases, but also interleukin (IL)-17 signaling, cytokine-cytokine receptor interaction, and chemokine signaling. Upregulation of leukocyte migration and chemokines are important factors promoting tumor progression and metastasis (24). Cluster Mγ is enriched in expression of multiple genes including APOE and BNIP3. Moreover, APOE is a specific marker gene for Mγ. Overall survival analysis in the GEPIA2 database indicates these genes predict worse prognosis (Figure 3F). In summary, IBD-CRC worse prognosis may be related to APOE and BNOP3 gene.
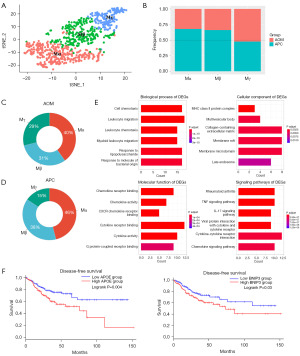
Higher proportion of T cells in APC dataset
T cells are major immune cell types that regulate anti-tumor responses, so we compared differences between T cells in the AOM and APC groups (Figure 4A). We observed T cells were more prevalent in the APC group compared to the AOM group (Figure 4B). Volcano plots of T cell DEGs between the AOM and APC groups are shown, indicating differences exist (Figure 4C). We validated the reliability of our cell clustering and T cell subclustering by showing specific T cell marker genes (Figure 4D). Next, we performed GO and KEGG pathway enrichment analysis on the T cell DEGs (Figure 4E). GO analysis revealed the DEGs were significantly enriched in lymphocyte and leukocyte proliferation and differentiation. KEGG pathway analysis showed the DEGs were enriched in Th17 cell differentiation and nuclear factor kappaB (NF-κB) signaling pathways. The NF-κB pathway is associated with tumor cytotoxicity, inhibiting cancer progression (25-27). In summary, APC T cells exhibit stronger tumor suppressive effects and further exploration of precise T cell subset functional alterations in IBD-CRC is warranted.
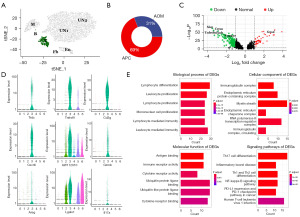
Tβ subgroup exhibits greater tumor suppressive effects
To illustrate T cell differences between the AOM and APC groups in more depth, we performed subclustering analysis on the T cells. The 3 T cell subgroups were named Tα, Tβ, and Tγ (Figure 5A). We show the cell fractions of the 3 cell types in the AOM and APC CRC types (Figure 5B) and their proportions in the AOM and APC groups (Figure 5C,5D). We found each T cell subgroup was less prevalent in AOM, with cluster Tβ differing most between the AOM and APC groups. Specific marker genes for cluster Tβ are shown (Figure S2). GO and KEGG pathway enrichment analysis was performed on DEGs in cluster Tβ (Figure 5E). GO analysis revealed cluster Tβ is associated with transcription repressors and myeloid cell differentiation. KEGG pathway analysis showed cluster Tβ is related to multiple pathways including IL-17 signaling, tumor necrosis factor (TNF) signaling, and Th17 cell differentiation. Upregulation of TNF signaling indicates good prognosis (28), and the IL-17 pathway is involved in promoting inflammation and protection against extracellular pathogens (29). PPP1CC and IFNGR1 in Tβ predict good prognosis (Figure 5F). These pieces of evidence indicate Tβ is associated with tumor suppression, further illustrating CRC driven by APC-mutation confers better prognosis compared to IBD-CRC.
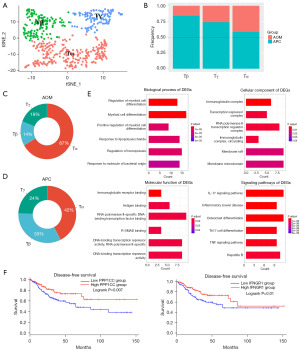
APOE and BNIP3 expression is associated with macrophage
Tumor associated macrophages have anti-inflammatory and pro tumor progression functions, and we found that the M1 subgroup expresses a higher inflammatory signaling pathway. Since APOE and BNIP3 were associated with CRC recurrence, and worse prognosis, we examined whether these genes are co-expressed in macrophages by immunofluorescence and immunohistochemical (Figure 6). We differentiate tumor tissue and adjacent normal tissue through HE staining (Figure 6A). We found that APOE and BNIP3 in 5 tumor/adjacent normal tissue pairs were co-localized with macrophage markers CD68 (Figure 6C,6D). Moreover, the expression of APOE, BNIP3 and CD68 were elevated in adjacent normal tissue compared with tumor tissue (Figure 6G-6I). However, we performed complementary validation of APOE and BNIP3 through immunohistochemical staining, consistent with immunofluorescence results (Figure 6B,6E,6F).
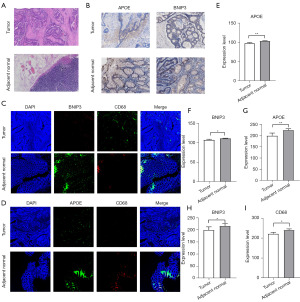
Discussion
The incidence rate and mortality of IBD-CRC gradually increase, and the worse prognosis of IBD-CRC remains unresolved. Recent research has increased understanding of IBD-CRC through various methods, including mouse models demonstrating IBD-CRC development (30-32). However, mechanisms underlying worse IBD-CRC prognosis compared to other subtybes CRC have not been elucidated at the single-cell level. Our scRNA-seq analysis of AOM/DSS disease and APCMin+ datasets provide insights into IBD-CRC heterogeneity to improve prognosis.
In this study, we analyzed the related scRNA data from GSE198758. Initial analysis uncovered fewer immune cells overall in AOM, while AOM displayed increased pro-tumoral properties, suggesting IBD-CRC prognosis may relate to diminished immunity. Comparing major immune subsets, macrophages exhibited enhanced pro-tumoral functions in AOM. APOE and BNIP3 expression in a Mγ subset associated with worse prognosis. Furthermore, a T cell subgroup with tumor suppressive properties was less prevalent in AOM. Our scRNA-seq characterization of CRC types elucidate unique immune landscapes underlying differential IBD-CRC and APC-CRC outcomes.
Macrophages exhibited marked heterogeneity between AOM and APC datasets. AOM had lower macrophage numbers and proportions, consistent with depletion worsening prognosis (33), and enrichment analysis implicated stronger pro-tumoral AOM macrophage effects. Subclustering revealed increased Mγ in AOM, so we analyzed this subset. Mγ displayed enriched expression of worse prognostic genes including APOE, a potential IBD-CRC recurrence biomarker. One study showed TREM2/APOE/C1q macrophage infiltration may be a potential prognostic biomarker for renal clear cell carcinoma recurrence, this is consistent with our results (28), and another study have shown that reducing APOE expression decreases thyroid cancer growth (34). TAMs promote gastric cancer migration by promoting APOE metastasis (35). Multiple studies have confirmed correlations between tumor metastasis and APOE expression (28,34-36). The Mγ subgroup displays another gene with poor prognosis, BNIP3, which plays an important role in cancer progression. Prior studies found BNIP3 promotes CRC under hypoxia, and drug resistance to cisplatin in CRC patients may be associated with abnormal Bnip3 expression (29,37). Mγ’s inflammatory profile suggests possible TAM identity, and TAMs facilitate cancer progression through instigating immunosuppression, invasion, and metastasis (17). Our scRNA-seq links APOE/BNIP3 macrophage expression to IBD-CRC prognosis. Immunofluorescence validated increased APOE/BNIP3 expression in patient adjacent normal versus tumor tissue, confirming the relationship with progression. Moreover, our mouse and patient data indicate AOM/IBD-CRC microenvironments harbor enhanced pro-tumoral properties, partly attributable to specific TAM activation, providing mechanistic insights into worse IBD-CRC differentiation and prognosis. Targeting the Mγ subset represents a potential therapeutic approach for improving outcomes.
T cells were more abundant in APC versus AOM datasets. Some studies have shown that the low response to immune checkpoint therapy in patients with metastatic castration-resistant prostate cancer is due to the paucity of T cells in the TME (38). Enrichment in proliferation/differentiation and NF-κB signaling implies stronger tumor inhibitory effect of APC T cells, consistent with IBD-CRC worser prognosis and higher recurrence (39-41). Subclustering revealed a tumor-suppressive Tβ subset concentrated in APC datasets. Tβ pathway analysis suggested inflammatory effects aiding tumor suppression (42,43). Our scRNA-seq thus provides insights into APC-mutant CRC versus IBD-CRC prognostic differences to guide proactive treatment approaches. These findings may guide development of prognosis-improving, subtype-tailored immunotherapies for CRC patients. Future patient sample validation and mechanistic investigations are warranted.
There are still some limitations of this study, including verifying relevant cell subpopulations in a large patient cohort and conducting in vivo and in vitro experiments to confirm the role of relevant cell subpopulations. In conclusion, our scRNA-seq analysis of mouse CRC datasets provide a high-resolution immune landscape characterization illuminating unique IBD-CRC properties underlying inferior outcomes. These findings lay the foundation for future patient sample validation studies and mechanistic investigations to tailor immunotherapeutic strategies for improving IBD-CRC prognosis.
Conclusions
In summary, our study revealed single-cell heterogeneity distinguishing inflammatory and APC-mutant CRC dataset microenvironments. IBD-CRC contained fewer enriched immune cells, including increased APOE+ pro-tumoral macrophages versus APC-mutant CRC. The IBD-CRC TME exhibited enhanced tumor-promoting properties. Our scRNA-seq analysis enriches theoretical knowledge of IBD-CRC pathogenesis at a high resolution, these results provide valuable insights into mechanisms underlying inferior IBD-CRC prognosis to guide development of tailored therapeutic strategies for improving outcomes. Overall, characterization of the intricate immune landscape differences between CRC subtypes lays the foundation for future translational research efforts to stratify and optimize immunotherapeutic approaches for CRC patients.
Acknowledgments
We would like to thank the GEO and GEPIA 2 databases, as well as the data providers for the free use of their data.
Funding: None.
Footnote
Reporting Checklist: The authors have completed the MDAR reporting checklist. Available at https://tcr.amegroups.com/article/view/10.21037/tcr-24-689/rc
Data Sharing Statement: Available at https://tcr.amegroups.com/article/view/10.21037/tcr-24-689/dss
Peer Review File: Available at https://tcr.amegroups.com/article/view/10.21037/tcr-24-689/prf
Conflicts of Interest: All authors have completed the ICMJE uniform disclosure form (available at https://tcr.amegroups.com/article/view/10.21037/tcr-24-689/coif). The authors have no conflicts of interest to declare.
Ethical Statement: The authors are accountable for all aspects of the work in ensuring that questions related to the accuracy or integrity of any part of the work are appropriately investigated and resolved. The study was conducted in accordance with the Declaration of Helsinki (as revised in 2013). This study was approved by the Institutional Review Board of the Tangshan Gongren Hospital Affiliated to Hebei Medical University (IRB-2023145). All patients have signed informed consent forms.
Open Access Statement: This is an Open Access article distributed in accordance with the Creative Commons Attribution-NonCommercial-NoDerivs 4.0 International License (CC BY-NC-ND 4.0), which permits the non-commercial replication and distribution of the article with the strict proviso that no changes or edits are made and the original work is properly cited (including links to both the formal publication through the relevant DOI and the license). See: https://creativecommons.org/licenses/by-nc-nd/4.0/.
References
- Sung H, Ferlay J, Siegel RL, et al. Global Cancer Statistics 2020: GLOBOCAN Estimates of Incidence and Mortality Worldwide for 36 Cancers in 185 Countries. CA Cancer J Clin 2021;71:209-49. [Crossref] [PubMed]
- Li GM, Xiao GZ, Qin PF, et al. Single-Cell RNA Sequencing Reveals Heterogeneity in the Tumor Microenvironment between Young-Onset and Old-Onset Colorectal Cancer. Biomolecules 2022;12:1860. [Crossref] [PubMed]
- Birch RJ, Burr N, Subramanian V, et al. Inflammatory Bowel Disease-Associated Colorectal Cancer Epidemiology and Outcomes: An English Population-Based Study. Am J Gastroenterol 2022;117:1858-70. [Crossref] [PubMed]
- Zegarra Ruiz DF, Kim DV, Norwood K, et al. Microbiota manipulation to increase macrophage IL-10 improves colitis and limits colitis-associated colorectal cancer. Gut Microbes 2022;14:2119054. [Crossref] [PubMed]
- Bose S, Saha P, Chatterjee B, et al. Chemokines driven ovarian cancer progression, metastasis and chemoresistance: Potential pharmacological targets for cancer therapy. Semin Cancer Biol 2022;86:568-79. [Crossref] [PubMed]
- Surun A, Varlet P, Brugières L, et al. Medulloblastomas associated with an APC germline pathogenic variant share the good prognosis of CTNNB1-mutated medulloblastomas. Neuro Oncol 2020;22:128-38. [Crossref] [PubMed]
- Chesnokova V, Zonis S, Zhou C, et al. Growth hormone is permissive for neoplastic colon growth. Proc Natl Acad Sci U S A 2016;113:E3250-9. [Crossref] [PubMed]
- Wang D, Xie B. Prognostic and tumor immunity implication of inflammatory bowel disease-associated genes in colorectal cancer. Eur J Med Res 2022;27:91. [Crossref] [PubMed]
- Chen H, Ye F, Guo G. Revolutionizing immunology with single-cell RNA sequencing. Cell Mol Immunol 2019;16:242-9. [Crossref] [PubMed]
- Kolodziejczyk AA, Kim JK, Svensson V, et al. The technology and biology of single-cell RNA sequencing. Mol Cell 2015;58:610-20. [Crossref] [PubMed]
- Yamada S, Nomura S. Review of Single-Cell RNA Sequencing in the Heart. Int J Mol Sci 2020;21:8345. [Crossref] [PubMed]
- Tian Y, Wang X, Cramer Z, et al. APC and P53 mutations synergise to create a therapeutic vulnerability to NOTUM inhibition in advanced colorectal cancer. Gut 2023;72:2294-306. [Crossref] [PubMed]
- Satija R, Farrell JA, Gennert D, et al. Spatial reconstruction of single-cell gene expression data. Nat Biotechnol 2015;33:495-502. [Crossref] [PubMed]
- Lee HO, Hong Y, Etlioglu HE, et al. Lineage-dependent gene expression programs influence the immune landscape of colorectal cancer. Nat Genet 2020;52:594-603. [Crossref] [PubMed]
- Liu Y, Zhang Q, Xing B, et al. Immune phenotypic linkage between colorectal cancer and liver metastasis. Cancer Cell 2022;40:424-437.e5. [Crossref] [PubMed]
- Yu G, Wang LG, Han Y, et al. clusterProfiler: an R package for comparing biological themes among gene clusters. OMICS 2012;16:284-7. [Crossref] [PubMed]
- Anderson NR, Minutolo NG, Gill S, et al. Macrophage-Based Approaches for Cancer Immunotherapy. Cancer Res 2021;81:1201-8. [Crossref] [PubMed]
- Zigmond E, Varol C, Farache J, et al. Ly6C hi monocytes in the inflamed colon give rise to proinflammatory effector cells and migratory antigen-presenting cells. Immunity 2012;37:1076-90. [Crossref] [PubMed]
- Morias Y, Abels C, Laoui D, et al. Ly6C- Monocytes Regulate Parasite-Induced Liver Inflammation by Inducing the Differentiation of Pathogenic Ly6C+ Monocytes into Macrophages. PLoS Pathog 2015;11:e1004873. [Crossref] [PubMed]
- Kim DS, Scherer PE. Obesity, Diabetes, and Increased Cancer Progression. Diabetes Metab J 2021;45:799-812. [Crossref] [PubMed]
- Garten A, Schuster S, Penke M, et al. Physiological and pathophysiological roles of NAMPT and NAD metabolism. Nat Rev Endocrinol 2015;11:535-46. [Crossref] [PubMed]
- Läubli H, Borsig L. Altered Cell Adhesion and Glycosylation Promote Cancer Immune Suppression and Metastasis. Front Immunol 2019;10:2120. [Crossref] [PubMed]
- Tong Y, Cheng PSW, Or CS, et al. Escape from cell-cell and cell-matrix adhesion dependence underscores disease progression in gastric cancer organoid models. Gut 2023;72:242-55. [Crossref] [PubMed]
- Chow MT, Luster AD. Chemokines in cancer. Cancer Immunol Res 2014;2:1125-31. [Crossref] [PubMed]
- Hoesel B, Schmid JA. The complexity of NF-κB signaling in inflammation and cancer. Mol Cancer 2013;12:86. [Crossref] [PubMed]
- Patel M, Horgan PG, McMillan DC, et al. NF-κB pathways in the development and progression of colorectal cancer. Transl Res 2018;197:43-56. [Crossref] [PubMed]
- Zhang M, Liu ZZ, Aoshima K, et al. CECR2 drives breast cancer metastasis by promoting NF-κB signaling and macrophage-mediated immune suppression. Sci Transl Med 2022;14:eabf5473. [Crossref] [PubMed]
- Obradovic A, Chowdhury N, Haake SM, et al. Single-cell protein activity analysis identifies recurrence-associated renal tumor macrophages. Cell 2021;184:2988-3005.e16. [Crossref] [PubMed]
- Qureshi-Baig K, Kuhn D, Viry E, et al. Hypoxia-induced autophagy drives colorectal cancer initiation and progression by activating the PRKC/PKC-EZR (ezrin) pathway. Autophagy 2020;16:1436-52. [Crossref] [PubMed]
- Rajamäki K, Taira A, Katainen R, et al. Genetic and Epigenetic Characteristics of Inflammatory Bowel Disease-Associated Colorectal Cancer. Gastroenterology 2021;161:592-607. [Crossref] [PubMed]
- Gobert AP, Latour YL, Asim M, et al. Protective Role of Spermidine in Colitis and Colon Carcinogenesis. Gastroenterology 2022;162:813-827.e8. [Crossref] [PubMed]
- Gong Y, Liu Z, Yuan Y, et al. PUMILIO proteins promote colorectal cancer growth via suppressing p21. Nat Commun 2022;13:1627. [Crossref] [PubMed]
- van der Bij GJ, Bögels M, Otten MA, et al. Experimentally induced liver metastases from colorectal cancer can be prevented by mononuclear phagocyte-mediated monoclonal antibody therapy. J Hepatol 2010;53:677-85. [Crossref] [PubMed]
- Huang J, Sun W, Wang Z, et al. FTO suppresses glycolysis and growth of papillary thyroid cancer via decreasing stability of APOE mRNA in an N6-methyladenosine-dependent manner. J Exp Clin Cancer Res 2022;41:42. [Crossref] [PubMed]
- Zheng P, Luo Q, Wang W, et al. Tumor-associated macrophages-derived exosomes promote the migration of gastric cancer cells by transfer of functional Apolipoprotein E. Cell Death Dis 2018;9:434. [Crossref] [PubMed]
- Liu H, Sun Y, Zhang Q, et al. Pro-inflammatory and proliferative microglia drive progression of glioblastoma. Cell Rep 2021;36:109718. [Crossref] [PubMed]
- Vianello C, Cocetta V, Catanzaro D, et al. Cisplatin resistance can be curtailed by blunting Bnip3-mediated mitochondrial autophagy. Cell Death Dis 2022;13:398. [Crossref] [PubMed]
- Subudhi SK, Siddiqui BA, Aparicio AM, et al. Combined CTLA-4 and PD-L1 blockade in patients with chemotherapy-naïve metastatic castration-resistant prostate cancer is associated with increased myeloid and neutrophil immune subsets in the bone microenvironment. J Immunother Cancer 2021;9:e002919. [Crossref] [PubMed]
- Ramsey M, Krishna SG, Stanich PP, et al. Inflammatory Bowel Disease Adversely Impacts Colorectal Cancer Surgery Short-term Outcomes and Health-Care Resource Utilization. Clin Transl Gastroenterol 2017;8:e127. [Crossref] [PubMed]
- Renz BW, Thasler WE, Preissler G, et al. Clinical outcome of IBD-associated versus sporadic colorectal cancer: a matched-pair analysis. J Gastrointest Surg 2013;17:981-90. [Crossref] [PubMed]
- Lu C, Schardey J, Zhang T, et al. Survival Outcomes and Clinicopathological Features in Inflammatory Bowel Disease-associated Colorectal Cancer: A Systematic Review and Meta-analysis. Ann Surg 2022;276:e319-30. [Crossref] [PubMed]
- Amatya N, Garg AV, Gaffen SL. IL-17 Signaling: The Yin and the Yang. Trends Immunol 2017;38:310-22. [Crossref] [PubMed]
- Guo W, Zhang C, Wang X, et al. Resolving the difference between left-sided and right-sided colorectal cancer by single-cell sequencing. JCI Insight 2022;7:e152616. [Crossref] [PubMed]