Bioinformatics study of bortezomib resistance-related proteins and signaling pathways in mantle cell lymphoma
Highlight box
Key findings
• We conducted a comprehensive bioinformatics analysis and identified 11 key differentially expressed gene (DEGs) linked to bortezomib (BTZ) resistance in mantle cell lymphoma (MCL). Midkine (MDK) was discovered to be a key gene involved in BTZ resistance. MDK serum levels differed significantly between the resistant and sensitive groups of patients.
What is known, and what is new?
• Previous research has shown the complexity of BTZ resistance mechanisms in MCL, which involve various genes and signaling pathways.
• The present study identified specific genes and pathways. Notably, it showed that MDK was a central mediator of resistance and provided validated clinical data to support bioinformatics predictions.
What is the implication, and what should change now?
• Identifying MDK as a central gene in BTZ resistance suggests new avenues for targeted therapy in MCL.
• Clinical strategies should consider monitoring MDK levels to predict and manage BTZ resistance, which could improve the therapeutic outcomes of MCL patients.
Introduction
Mantle cell lymphoma (MCL) is a rare subtype of non-Hodgkin’s lymphoma of B-cell non-Hodgkin lymphoma (NHL), and accounts for approximately 3–10% of all new NHL cases (1). MCL typically affects individuals between 60 and 70 years and is more common in men than women (2). The disease is often diagnosed at an advanced stage, specifically stage III or IV (3). The lymph nodes are most often affected, followed by the bone marrow and spleen (3). The physiological heterogeneity of MCL and its diverse clinical presentations present significant challenges in its treatment. Additionally, the advanced age of most patients precludes the use of high-dose chemotherapy regimens (4).
MCL patients who have not received any form of treatment, who are not suitable candidates for high-dose chemotherapy, or who are not eligible for autologous stem cell transplantation may be treated with the rituximab, cyclophosphamide, doxorubicin, vincristine, and prednisone (R-CHOP) regimen, which has been observed to yield a complete response rate of up to 42% (5). However, it should be noted that the median progression-free survival (PFS) of patients who receive this regimen is only approximately 16.6 months (5,6). Use of molecularly targeted medicines, such as the proteasome inhibitor bortezomib (BTZ), with R-CHOP can substantially enhance response rates (7).
BTZ inhibits the proteasomal complex and is predominantly employed as a first-line therapeutic intervention for multiple myeloma (8). Studies have shown that BTZ also exerts significant anti-tumor effects in patients with relapsed/refractory MCL and diffuse large B-cell lymphoma (9,10). However, its efficacy is suboptimal for some patients, and resistance can occur (11,12). Therefore, it is crucial to systematically analyze the key genes and related mechanisms of BTZ resistance in MCL through bioinformatics methods for the treatment and prognosis of MCL.
This study used the Gene Expression Omnibus (GEO) database, which is a rich resource of gene expression data. Specifically, using advanced bioinformatics tools, we explored the differentially expressed genes (DEGs) associated with BTZ resistance in MCL. We identified these genes, mapped the disease-related signaling pathways, and constructed protein-protein interaction (PPI) networks to understand protein communication and molecular regulation. Our goal was to predict the roles of key genes in drug resistance development, providing a theoretical basis for understanding BTZ resistance. To ensure the robustness and clinical relevance of our findings, we integrated real-world data from MCL patients at the Affiliated Tumor Hospital of Nantong University to enhance the credibility and applicability of our results. By combining computational biology with empirical patient data, we seek to improve the diagnostic strategies and therapeutic interventions for BTZ-resistant MCL. We present this article in accordance with the STREGA reporting checklist (available at https://tcr.amegroups.com/article/view/10.21037/tcr-24-1482/rc).
Methods
Data sourcing
Gene chip datasets containing MCL BTZ-resistant and normal control cohorts (GSE20915 and GSE51371) were obtained from the GEO database (https://www.ncbi.nlm.nih.gov/geo/). The datasets included in this study had to meet the following inclusion criteria: (I) comprise whole-genome messenger RNA expression microarray data; (II) include MCL BTZ-resistant and normal control cohorts; and (III) have undergone standardized processing.
Combining DEG selection with data processing
The R programming language (https://www.r-project.org/) was used to perform the principal component analysis (PCA) of specimens derived from the two datasets to examine the distribution among various cohorts. GEO2R (https://www.ncbi.nlm.nih.gov/geo/geo2r/) was used to analyze the DEGs in the datasets. The selection criteria for the DEGs were as follows: an absolute value of the logarithm of the fold change ≥0.5 and a P value <0.05. Some common DEGs obtained from the two (datasets) were inconsistently regulated (i.e., either upregulated or downregulated). A Venn diagram was generated to show all the intersecting typical DEGs, and a bioinformatics analysis of the related pathways was performed. To eliminate the influence of false positives in the identification of common DEGs and to screen for predictive targets that can serve as predictors for clinical diagnosis and prognosis, upregulated genes in clinical applications often represent excessively active or abnormally enhanced biological processes (BP), which may be key drivers of disease occurrence and progression. Therefore, we chose to analyze only the upregulated genes among the common DEGs. Heatmaps and volcano plots were also generated using the CHDTEPDB (13) to visualize the DEGs identified in the two datasets. The Venn diagram showed the intersecting upregulated DEGs from both datasets, revealing a set of consistently upregulated DEGs associated with BTZ resistance in MCL.
Gene Ontology (GO), Kyoto Encyclopedia of Genes and Genomes (KEGG), and gene set enrichment analysis (GSEA) pathway enrichment assessments
The biological roles of the DEGs in BTZ-resistant MCL were investigated by GO and KEGG assessments. The GO technique is a strong bioinformatics technique used to determine the BP, cellular components (CC), and molecular functions (MF) associated with DEGs. A GSEA was conducted to assess the possible related mechanistic pathways.
Analysis of PPI networks of frequently expressed DEGs
The PPI networks of the common DEGs were studied using the Search Tool for the Retrieval of Interacting Genes/Proteins (STRING) (https://string-db.org/). The results were subsequently loaded into the Cytoscape (https://cytoscape.org/) software to facilitate viewing and correlation analysis. The Molecular Complex Detection (MCODE) plugin was used to identify crucial protein expression molecules.
Clinical data
The study cohort comprised 40 patients with relapsed or refractory MCL who were admitted to the Department of Hematology and Lymphoma at the Affiliated Tumor Hospital of Nantong University between June 2020 to December 2023. To be eligible for inclusion in this study, the patients had to meet the following inclusion criteria: (I) be aged older than 18 years; (II) have received a diagnosis of MCL based on an analysis of their imaging data and a histological examination; (III) have received BTZ as second-line treatment; and (IV) have adhered to follow-up and treatment. Patients were excluded from the study if they met any of the following exclusion criteria: (I) had incomplete clinical data; and/or (II) had other concurrent malignant tumors.
The therapeutic response of the patients after treatment was evaluated using computed tomography, magnetic resonance imaging, or positron emission tomography-computed tomography following the Revised Response Criteria for Lymphoma (14). Patients exhibiting a partial response, chemotherapy-related recurrence/progression, or uncertain complete response followed by recurrence/death within 6 months of beginning treatment were classified as the resistant cohort, while the remaining patients were classified as the sensitive cohort.
The study was conducted in accordance with the Declaration of Helsinki (as revised in 2013). The study was approved by the ethics committee of the Affiliated Tumor Hospital of Nantong University (No. 2023-40), and informed consent was taken from all the patients.
ELISA for detecting the expression levels of the DEG midkine (MDK) in the serum of the patients in the resistant and sensitive cohorts
The 96-well response plates were coated with diluted polyclonal antibodies against human MDK, specifically sheep anti-human MDK antibodies. Each well received 100 µL of the antibody solution. The plates were incubated at 37 ℃ for 0.5 hours and then incubated overnight at 4 ℃. Following the removal of the coating solution, the plates underwent a washing process using phosphate-buffered saline solution augmented with 0.05% Tween 20 (PBST). The blocking solution was added to each well, which was 300 µL in volume and contained 1% fetal bovine serum mixed with PBST. The plates were then incubated for 1 hour at room temperature, after which they were washed again with PBST. The serum samples and standard solutions were diluted using a dilution solution, and 100 µL of each diluted sample was introduced into the individual reaction wells, including a blank control. The plates were incubated at ambient temperature for 60 minutes, after which they were washed with PBST. Subsequently, 100 µL of rabbit anti-human MDK monoclonal antibody (0.1 mg/L) was added to each well, and the plates were incubated at an ambient temperature for 1 hour, and then washed with PBST. Next, 50 µL of secondary antibody labeled with horseradish peroxidase was added to each well and allowed to incubate at ambient temperature for 1 hour. Following the process of PBST washing the initiation of color development was carried out. Subsequently, the plates were incubated at room temperature in a light-restricted environment for 10 minutes. The experiment was terminated, and the optical density values were recorded at a specific wavelength of 450 nm using an enzyme-linked immunosorbent assay (ELISA) reader.
Statistical analysis
SPSS 26.0 (IBM Corporation, USA) and GraphPad Prism 7.0 (GraphPad Software Inc. USA) were used for the graphing and statistical analysis. T-tests were applied to generate the P values and adjusted P values with the latter being modified by the false discovery rate. The serum MDK levels are presented as the median (M) and quartile (P25, P75) for the non-normally distributed quantitative data. The quantitative data of both cohorts were compared using the Mann-Whitney U test for independent samples. A P value <0.05 was considered statistically significant.
Results
Selection of DEGs linked to MCL BTZ resistance
Gene chip datasets containing MCL BTZ-resistant cohorts and normal control cohorts (GSE20915 and GSE51371) were selected from the GEO database. Volcano plots, PCA plots, heatmaps, and normalized box plots of the samples were obtained for the DEGs based on the selection criteria (Figure 1A,1B). Among the results, particular emphasis was placed on the upregulated DEGs. In GSE20915, 144 upregulated DEGs were identified, while in GSE51371, 219 upregulated DEGs were identified. Using a Venn diagram to compare the elevated DEGs from both datasets (Figure 1C), 11 DEGs linked to MCL BTZ resistance were identified. These genes are set out in Table 1.
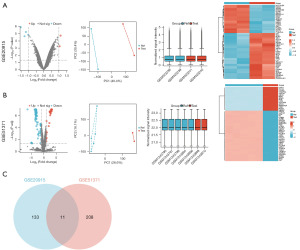
Table 1
PTPRN2 | CDKN1C | HIPK2 | GBE1 |
DENND1B | MDK | RHOBTB1 | BIK |
TNFAIP3 | BTNL9 | CDKN1A |
DEGs, differentially expressed genes; PTPRN2, protein tyrosine phosphatase, receptor type N2; CDKN1C, cyclin-dependent kinase inhibitor 1C; HIPK2, homeodomain interacting protein kinase 2; GBE1, glucan (1,4-alpha-), branching enzyme 1; DENND1B, DENN domain containing 1B; MDK, midkine; RHOBTB1, Rho-related BTB domain containing 1; BIK, BCL-2-interacting killer; TNFAIP3, TNF alpha induced protein 3; BTNL9, butyrophilin like 9; CDKN1A, cyclin-dependent kinase inhibitor 1A.
KEGG enrichment analysis with GO
An enrichment analysis of the identified DEGs was conducted using the Database for Annotation, Visualization and Integrated Discovery (DAVID). This analysis aimed to acquire enrichment information related to GO in the context of Homo sapiens. The upregulated DEGs were found to be enriched in a variety of BP, including the negative regulation of transferase activity, the intrinsic apoptotic signaling pathway in response to DNA damage by p53 class mediator, the negative regulation of cyclin-dependent serine/threonine kinase activity, and protein phosphorylation (Figure 2A). In relation to the MF, the DEGs were found to be associated with several activities, including protein kinase inhibitor activity, Lys63-specific deubiquitinase activity, transmembrane receptor protein tyrosine phosphatase activity, and protein tyrosine kinase activity (Figure 2B). The KEGG pathway enrichment analysis revealed that the elevated DEGs were predominantly linked to the cell cycle, cellular senescence, nuclear factor kappa-B (NF-κB) signaling pathway, interleukin 17 (IL-17) signaling network, and p53 signaling pathway (Figure 2C). The top five outcomes are presented in Table 2.
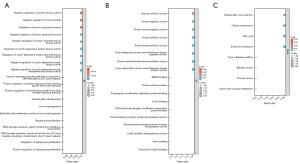
Table 2
Ontology | ID | Descriptions | Gene ratio | Bg ratio | P value | Adjusted P value |
---|---|---|---|---|---|---|
BP | GO:0045736 | Cyclin-dependent protein serine/threonine kinase activity is negatively regulated | 3/11 | 33/18800 | 8.05e−07 | 8.05e−07 |
BP | GO:1904030 | Cyclin-dependent protein kinase activity is negatively regulated | 3/11 | 33/18800 | 8.05e−07 | 8.05e−07 |
BP | GO:2000347 | Hepatocyte proliferation is positively regulated | 2/11 | 10/18800 | 1.4e−05 | 1.4e−05 |
BP | GO:0000079 | Control of protein serine/threonine kinase activity that is cyclin-dependent | 3/11 | 98/18800 | 2.2e−05 | 2.2e−05 |
BP | GO:1904029 | Control of the activity of cyclin-dependent protein kinase | 3/11 | 101/18800 | 2.41e−05 | 2.41e−05 |
MF | GO:0004861 | Activity inhibiting cyclin-dependent protein serine/threonine kinase | 2/11 | 12/18410 | 2.14e−05 | 2.14e−05 |
MF | GO:0030291 | Inhibition of protein serine/threonine kinase activity | 2/11 | 33/18410 | <0.001 | <0.001 |
MF | GO:0016538 | Regulator activity of cyclin-dependent protein serine/threonine kinase | 2/11 | 50/18410 | <0.001 | <0.001 |
MF | GO:0004860 | Protein kinase inhibitor activity | 2/11 | 70/18410 | <0.001 | <0.001 |
MF | GO:0019210 | Kinase inhibitor activity | 2/11 | 74/18410 | <0.001 | <0.001 |
KEGG | hsa01522 | Endocrine resistance | 2/8 | 98/8164 | 0.004 | 0.004 |
KEGG | hsa04110 | Cell cycle | 2/8 | 126/8164 | 0.006 | 0.006 |
KEGG | hsa04218 | Cellular senescence | 2/8 | 156/8164 | 0.009 | 0.009 |
KEGG | hsa05169 | Epidemic of Epstein-Barr virus | 2/8 | 202/8164 | 0.02 | 0.02 |
KEGG | hsa00500 | Metabolism of sucrose and starch | 1/8 | 36/8164 | 0.03 | 0.03 |
GO, Gene Ontology; KEGG, Kyoto Encyclopedia of Genes and Genomes; BP, biological process; MF, molecular function.
Building a PPI network, evaluating the module, and selecting the core genes of the common DEGs
The PPI network of DEGs was constructed using the STRING database. The acquired findings were loaded into the Cytoscape software to facilitate the viewing and study of the PPI/s. The Network Analyzer tool in Cytoscape was used to compute the undirected scores for each node in the PPI and determine the degree values for the corresponding nodes. Node size was representative of the degree value and the node color which ranged from red to green, signifying each node’s varying levels of neighborhood connectivity. The thickness of the edges indicated the combined score value, and an attribute circle layout was used to arrange all the protein nodes. The nodes with a degree value ≥4 were positioned in the inner layer (Figure 3A). The MCODE plugin was also used to perform the cluster analysis of the significant protein molecules, employing default parameters such as a node score cut-off value of 0.2, a K-core of 2, and maximum depth of 100. This analysis revealed one highly scored cluster (Figure 3B). The candidate key genes included cyclin dependent kinase inhibitor 1A (CDKN1A), CDKN1C, MDK, and TNF alpha induced protein 3 (TNFAIP3). Since CDKN1A, CDKN1C, and TNFAIP3 all function as “tumor suppressor genes” in lymphoma or MCT, which is contrary to the upregulation of these target genes observed in the BTZ-resistant group in this study, we believe that MDK may be the optimal key gene.
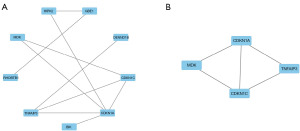
Patient cohorts
Based on the follow-up results, the patients were allocated to the following two cohorts: the resistant cohort (n=26); and the sensitive cohort (n=14). The resistant cohort comprised 19 males and 7 females with a mean age of 64.34±5.53 years and a mean body mass index (BMI) of 22.00±4.42 kg/m². The sensitive cohort’ comprised 10 males and 4 females with a mean age of 62.10±6.24 years and a mean BMI of 22.33±2.59 kg/m2. No statistically significant differences were observed between the two cohorts in terms of gender, age, and BMI (all P>0.05).
Comparison of serum MDK levels between the two cohorts of patients
The resistant cohort had a significantly higher serum concentration of MDK [1,539 (1,212, 2,023) ng/L] than the sensitive cohort [1,175 (786, 1,502) ng/L] (Figure 4). This difference was found to be statistically significant (P<0.05).
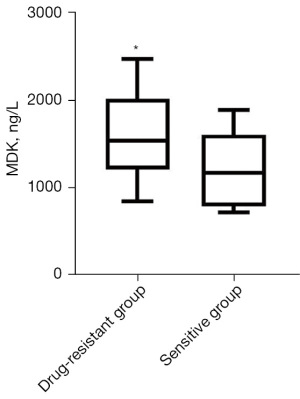
Discussion
BTZ exerts potent anti-tumor effects by inhibiting proteasome activity, leading to the accumulation of abnormal proteins and apoptosis in tumor cells (15). It has shown promise in treating relapsed and refractory MCL, significantly extending progression-free survival when combined with chemotherapy, and is FDA-approved as a second-line therapy (16,17). However, some MCL patients develop resistance to BTZ, further worsening their prognosis (18). Therefore, the identification of the key genes involved in BTZ resistance in MCL is crucial for the development of new therapeutic targets for BTZ-resistant MCL. In recent years, gene chips have become extensively used in illness research (19). The use of gene chips provides a high-throughput method for screening DEGs and allows for the identification of specific proteins and signaling pathways involved in resistance mechanisms. By integrating bioinformatics analysis, gene chips enable us to detect potential therapeutic targets and biomarkers for overcoming BTZ resistance, offering valuable insights into personalized treatment strategies for MCL.
This study employed bioinformatics tools to analyze records about BTZ resistance in MCL obtained from the GEO database. The research identified 11 commonly elevated DEGs. The KEGG analysis revealed that the enriched pathways primarily relate to the cell cycle, cellular senescence, the p53 signaling pathway, the NF-κB signaling pathway, and the IL-17 signaling pathway. The mechanisms mentioned above have been previously documented in the context of MCL. For example, research has shown that deferasirox can initiate the breakdown of cyclin D1 and cause apoptosis in MCL cells through a process dependent on reactive oxygen species and GSK3β (glycogen synthase kinase 3 beta) (20). The direct downregulation of CDC20 (cell division cycle 20) by p53 exhibits anti-tumor activity in MCL (21). The mitoses of natural killer lymphoma cells induced by BTZ leads to cell death (22). The inhibition of KDM6B histone demethylase by GSK-J4 modulates NF-κB signaling, preventing adhesion between MCL cells and stromal cells (23). Such findings implicitly indicate the accuracy and scientific significance of our study’s findings.
In constructing the PPI network and conducting the module analysis of the shared DEGs, we discovered a single cluster and four potential candidate genes (i.e., CDKN1A, CDKN1C, MDK, and TNFAIP3). Previous study on lymphoma and BTZ have shown that the simultaneous inhibition of EZH1/2 (enhancer of Zeste 1/2Polycomb repressive complex 2 subunit) effectively overcomes resistance to ibrutinib in MCL by explicitly targeting the growth-inhibitory effects mediated by CDKN1C (24). The reactivation of EZH1/2 in MCL leads to the re-expression of p57CDKN1C and TP53INP1, resulting in cell-cycle arrest and the inhibition of cell growth (25). The TNFAIP3/A20 gene has been identified as a newly discovered tumor suppressor in several subtypes of NHL, such as MCL (26). Additionally, recent study has shown the potential effectiveness of newer histone deacetylase inhibitors in lymphoma models, emphasizing the significance of CDKN1A expression in facilitating the anti-tumor response of these inhibitors (27). Since CDKN1A, CDKN1C, and TNFAIP3 are recognized as tumor suppressor genes in lymphoma or MCL, their upregulation in the BTZ-resistant group observed in this study contradicts their typical tumor-suppressive role. In contrast, MDK is known to function as an oncogene, and its upregulation aligns with its role in promoting tumor progression and resistance mechanisms. Therefore, based on this differential behavior, we believe that MDK may be the optimal key gene contributing to BTZ resistance in MCL. Further in vivo and in vitro experiments need to be conducted to verify and explore the role of MDK in the future (28).
To further explore the expression of MDK in BTZ-resistant MCL patients, we examined 40 MCL patients who underwent second-line treatment with BTZ. After six months of observation, the patients were classified into resistant and sensitive cohorts based on their treatment response. The findings showed that the serum MDK concentration of the resistant cohort was significantly higher than that of the sensitive cohort. This outcome further emphasized the potential importance of MDK as a key gene influencing the mechanism of BTZ resistance in MCL patients. The role of MDK in tumor resistance is primarily linked to its anti-apoptotic and anti-senescence effects and its mediation of intercellular resistance signaling (29,30). Thus, blocking the MDK-mediated resistance pathway could improve patient treatment outcomes.
Conclusions
Many factors are believed to drive the emergence of resistance to BTZ in MCL. The identification of the key gene MDK and its corresponding signaling pathways extends our understanding of the underlying molecular mechanisms involved in this phenomenon. Moreover, this study established a theoretical framework for prospective specialized treatment interventions. Nevertheless, it is important to acknowledge this study’s limitations, including a relatively small sample size and the absence of in vitro experimental validation. These factors might have generated biases in the selection and analysis of the data. To evaluate and delve deeper into the findings, future research needs to be conducted, including multicenter, large-sample, prospective investigations.
Acknowledgments
Funding: This study was supported by
Footnote
Reporting Checklist: The authors have completed the STREGA reporting checklist. Available at https://tcr.amegroups.com/article/view/10.21037/tcr-24-1482/rc
Data Sharing Statement: Available at https://tcr.amegroups.com/article/view/10.21037/tcr-24-1482/dss
Peer Review File: Available at https://tcr.amegroups.com/article/view/10.21037/tcr-24-1482/prf
Conflicts of Interest: All authors have completed the ICMJE uniform disclosure form (available at https://tcr.amegroups.com/article/view/10.21037/tcr-24-1482/coif). The authors have no conflicts of interest to declare.
Ethical Statement: The authors are accountable for all aspects of the work in ensuring that questions related to the accuracy or integrity of any part of the work are appropriately investigated and resolved. The study was conducted in accordance with the Declaration of Helsinki (as revised in 2013). The study was approved by the ethics committee of the Affiliated Tumor Hospital of Nantong University (No. 2023-40), and informed consent was taken from all the patients.
Open Access Statement: This is an Open Access article distributed in accordance with the Creative Commons Attribution-NonCommercial-NoDerivs 4.0 International License (CC BY-NC-ND 4.0), which permits the non-commercial replication and distribution of the article with the strict proviso that no changes or edits are made and the original work is properly cited (including links to both the formal publication through the relevant DOI and the license). See: https://creativecommons.org/licenses/by-nc-nd/4.0/.
References
- Skarbnik AP, Goy AH. Lenalidomide for mantle cell lymphoma. Expert Rev Hematol 2015;8:257-64. [Crossref] [PubMed]
- Silkenstedt E, Linton K, Dreyling M. Mantle cell lymphoma - advances in molecular biology, prognostication and treatment approaches. Br J Haematol 2021;195:162-73. [Crossref] [PubMed]
- Ye H, Desai A, Zeng D, et al. Frontline Treatment for Older Patients with Mantle Cell Lymphoma. Oncologist 2018;23:1337-48. [Crossref] [PubMed]
- Yavorkovsky LL. Mantle Cell Lymphoma-Time to Dismantle the Treatment Paradox. JAMA Oncol 2018;4:626-7. [Crossref] [PubMed]
- Robak T, Huang H, Jin J, et al. Bortezomib-based therapy for newly diagnosed mantle-cell lymphoma. N Engl J Med 2015;372:944-53. [Crossref] [PubMed]
- Joyson P, Karanvir S, Sumit P, et al. An Update on Recently Developed Analytical and Bio-analytical Methods for Some Anticancer Drugs. Curr Pharm Anal 2023;19:117-35. [Crossref]
- Novak U, Fehr M, Schär S, et al. Combined therapy with ibrutinib and bortezomib followed by ibrutinib maintenance in relapsed or refractory mantle cell lymphoma and high-risk features: a phase 1/2 trial of the European MCL network (SAKK 36/13). EClinicalMedicine 2023;64:102221. [Crossref] [PubMed]
- Rajkumar SV. Multiple myeloma: 2022 update on diagnosis, risk stratification, and management. Am J Hematol 2022;97:1086-107. [Crossref] [PubMed]
- Fricker LD. Proteasome Inhibitor Drugs. Annu Rev Pharmacol Toxicol 2020;60:457-76. [Crossref] [PubMed]
- Davies AJ, Barrans S, Stanton L, et al. Differential Efficacy From the Addition of Bortezomib to R-CHOP in Diffuse Large B-Cell Lymphoma According to the Molecular Subgroup in the REMoDL-B Study With a 5-Year Follow-Up. J Clin Oncol 2023;41:2718-23. [Crossref] [PubMed]
- Luanpitpong S, Janan M, Yosudjai J, et al. Bcl-2 Family Members Bcl-xL and Bax Cooperatively Contribute to Bortezomib Resistance in Mantle Cell Lymphoma. Int J Mol Sci 2022;23:14474. [Crossref] [PubMed]
- Zeng G, Yu Q, Zhuang R, et al. Recent advances and future perspectives of noncompetitive proteasome inhibitors. Bioorg Chem 2023;135:106507. [Crossref] [PubMed]
- Song Z, Yu J, Wang M, et al. CHDTEPDB: Transcriptome Expression Profile Database and Interactive Analysis Platform for Congenital Heart Disease. Congenit Heart Dis 2023;18:693-701. [Crossref]
- Cheson BD, Pfistner B, Juweid ME, et al. Revised response criteria for malignant lymphoma. J Clin Oncol 2007;25:579-86. [Crossref] [PubMed]
- Alwahsh M, Farhat J, Talhouni S, et al. Bortezomib advanced mechanisms of action in multiple myeloma, solid and liquid tumors along with its novel therapeutic applications. EXCLI J 2023;22:146-68. [PubMed]
- Bond DA, Martin P, Maddocks KJ. Relapsed Mantle Cell Lymphoma: Current Management, Recent Progress, and Future Directions. J Clin Med 2021;10:1207. [Crossref] [PubMed]
- Pan D, Kaufman JL, Htut M, et al. Filanesib plus bortezomib and dexamethasone in relapsed/refractory t(11;14) and 1q21 gain multiple myeloma. Cancer Med 2022;11:358-70. [Crossref] [PubMed]
- Gonzalez-Santamarta M, Quinet G, Reyes-Garau D, et al. Resistance to the Proteasome Inhibitors: Lessons from Multiple Myeloma and Mantle Cell Lymphoma. Adv Exp Med Biol 2020;1233:153-74. [Crossref] [PubMed]
- Afable MG 2nd, Wlodarski M, Makishima H, et al. SNP array-based karyotyping: differences and similarities between aplastic anemia and hypocellular myelodysplastic syndromes. Blood 2011;117:6876-84. [Crossref] [PubMed]
- Samara A, Shapira S, Lubin I, et al. Deferasirox induces cyclin D1 degradation and apoptosis in mantle cell lymphoma in a reactive oxygen species- and GSK3β-dependent mechanism. Br J Haematol 2021;192:747-60. [Crossref] [PubMed]
- Chen Y, Yang P, Wang J, et al. p53 directly downregulates the expression of CDC20 to exert anti-tumor activity in mantle cell lymphoma. Exp Hematol Oncol 2023;12:28. [Crossref] [PubMed]
- Shen L, Au WY, Wong KY, et al. Cell death by bortezomib-induced mitotic catastrophe in natural killer lymphoma cells. Mol Cancer Ther 2008;7:3807-15. [Crossref] [PubMed]
- Sadeghi L, Wright APH. GSK-J4 Inhibition of KDM6B Histone Demethylase Blocks Adhesion of Mantle Cell Lymphoma Cells to Stromal Cells by Modulating NF-κB Signaling. Cells 2023;12:2010. [Crossref] [PubMed]
- Kagiyama Y, Fujita S, Shima Y, et al. CDKN1C-mediated growth inhibition by an EZH1/2 dual inhibitor overcomes resistance of mantle cell lymphoma to ibrutinib. Cancer Sci 2021;112:2314-24. [Crossref] [PubMed]
- Li W, Bi C, Han Y, et al. Targeting EZH1/2 induces cell cycle arrest and inhibits cell proliferation through reactivation of p57(CDKN1C) and TP53INP1 in mantle cell lymphoma. Cancer Biol Med 2019;16:530-41. [Crossref] [PubMed]
- Honma K, Tsuzuki S, Nakagawa M, et al. TNFAIP3/A20 functions as a novel tumor suppressor gene in several subtypes of non-Hodgkin lymphomas. Blood 2009;114:2467-75. [Crossref] [PubMed]
- Mensah AA, Kwee I, Gaudio E, et al. Novel HDAC inhibitors exhibit pre-clinical efficacy in lymphoma models and point to the importance of CDKN1A expression levels in mediating their anti-tumor response. Oncotarget 2015;6:5059-71. [Crossref] [PubMed]
- Seyfinejad B, Jouyban A. Importance of Method Validation in the Analysis of Biomarker. Curr Pharm Anal 2022;18:567-9. [Crossref]
- Yu X, Zhou Z, Tang S, et al. MDK induces temozolomide resistance in glioblastoma by promoting cancer stem-like properties. Am J Cancer Res 2022;12:4825-39. [PubMed]
- Saikia M, Cheung N, Singh AK, et al. Role of Midkine in Cancer Drug Resistance: Regulators of Its Expression and Its Molecular Targeting. Int J Mol Sci 2023;24:8739. [Crossref] [PubMed]